Exploring diverse programmed cell-death patterns to develop a novel gene signature for predicting the prognosis of lung adenocarcinoma patients
Highlight box
Key findings
• A 9-gene signature to predict prognosis of lung adenocarcinoma (LUAD) based on programmed cell death (PCD) was developed and validated by RNA sequencing and immunohistochemical assays.
What is known and what is new?
• PCD plays a critical role in cancer progression, metastasis and anti-tumor immunity.
• This study aimed to identify survival-related genes and develop a novel gene signature based on 12 forms of PCD for predicting the prognosis of LUAD.
What is the implication, and what should change now?
• The cell death index in this article could serve as a valuable prognostic indicator and guide personalized therapeutic strategies for LUAD.
Introduction
Lung cancer is the leading cause of cancer-related deaths worldwide, accounting for nearly 1.3 million deaths per year (1). Among them, lung adenocarcinoma (LUAD) is the most common type of lung cancer and accounts for 50% of all cases. Although great advances have been made in early low-dose computed tomography (CT) screening together with chemotherapy and targeted therapies, overall survival (OS) is still low for most LUAD patients (2). The high risk of acquired chemoresistance and metastatic relapse after excision are the main reasons. At present, the commonly used indicators to predict the prognosis of LUAD include tumor size and metastasis (3), which hardly match the high heterogeneity of lung cancer. Therefore, there is an urgent need to explore more accurate and effective prognostic indicators to facilitate the personalized diagnosis and appropriate therapeutic intervention for LUAD patients.
Programmed cell death (PCD) is tightly controlled by a specific molecular machinery. Cells that are incapable of killing themselves may accumulate further genetic damage that advances neoplastic transformation (4). And the resistance of cancer cells to undergo PCD is closely associated with cancer development and chemotherapeutic resistance. PCD consists of apoptosis, necroptosis, ferroptosis, pyroptosis, netotic cell death, entotic cell death, lysosome-dependent cell death, parthanatos, autophagy-dependent cell death, oxeiptosis, cuproptosis and alkaliptosis (5). Apoptosis is a well-ordered process of cellular control, where initially the integrity of the cell membrane is maintained, but later stages involve membrane blebbing and loss of membrane integrity (6). Cancer cells often show some level of apoptotic resistance, a trait they share with artificially generated “undead” cells (7). Programmed necrosis is typically associated with nuclear degradation and the release of factors like high mobility group box 1 (HMGB1), triggering a potent inflammatory response (8). Ferroptosis, a form of cell death characterized by iron-dependent lipid peroxidation, is implicated in carcinogenesis and is considered distinct from other regulated cell death forms (9). Pyroptosis is an immunogenic form of cell death that releases pro-inflammatory cytokines, influencing the infiltration of immune cells into the tumor microenvironment (TME) (10). Netotic cell death is driven by the release of neutrophil extracellular traps (NETs) (5). In cancer, NETs can influence tumor progression, metastasis, and the TME (11). The engulfment and killing of live cells by entosis may serve as a mechanism to eliminate cancer cells, acting as a suppressor of tumor growth (12). Lysosomal cell death is mediated by hydrolytic enzymes released into the cytosol after lysosomal membrane permeabilization (5). Parthanatos is a form of cell death that depends on the overactivation of poly ADP-ribose polymerase-1 (PARP1). In cancer, PARP1 plays a complex role, both promoting DNA repair and stimulating DNA fragmentation (11). Autophagy can mend the aptness of metastatic cells under stressful conditions by counteracting apoptosis and necroptosis, but on the other hand, autophagy reduces metastasis by inducing the death of metastasizing cells (13). Oxeiptosis is a reactive oxygen species (ROS)-sensitive, caspase-independent, non-inflammatory regulated cell death pathway (14). Cuproptosis is associated with mitochondrial metabolism and affects tumor cell proliferation, metastasis, and drug resistance (15). Alkaliptosis, being an intracellular alkalinization-dependent form of regulated necrosis, offers a novel avenue for cancer treatment (16). In conclusion, increasing research on PCD has revealed its complex impact on cancer progression, metastasis and anti-tumor immunity.
Despite the critical role of PCD in the development of malignant tumors, the relationship between all the 12 types of PCD and the survival outcomes of LUAD requires further research. Therefore, the aim of this study is to bridge this knowledge gap and identify survival-related genes by integrating array-based databases to construct an effective prognostic indicator. In recent years, prognostic signature based on multiple genes has been widely studied and used to predict the prognosis and treatment of various tumors (2). Thus, we also employed least absolute shrinkage and selection operator (LASSO) regression-based multi-gene signature to establish a novel survival indicator for LUAD and verified its prognostic value on protein level. Furthermore, we explored the correlation between the expression of diverse PCD genes and tumor immune infiltration in LUAD. We present this article in accordance with the TRIPOD reporting checklist (available at https://jtd.amegroups.com/article/view/10.21037/jtd-23-1275/rc).
Methods
Collection of PCD related genes and LUAD datasets
The workflow of our study is shown in Figure 1. The gene set of regulatory genes of the 12 patterns of PCD mentioned above were collected from multiple resources, including GSEA gene sets, GeneCards website, KEGG, public data center of FerrDb (www.zhounan.org/ferrdb) and manual collection from relevant review articles (5,13,15). Ultimately, 1,933 genes were selected as our gene set, including 504 ferroptosis genes, 101 necroptosis genes, 427 apoptosis genes, 403 lysosome-dependent cell death genes, 388 autophagy genes, 52 pyroptosis genes, 15 entotic cell death genes, 14 cuproptosis genes, 9 parthanatos genes, 8 netotic cell death genes, 7 alkaliptosis genes, and 5 oxeiptosis genes. The gene list in detail is exhibited at table available at https://cdn.amegroups.cn/static/public/jtd-23-1275-1.doc.
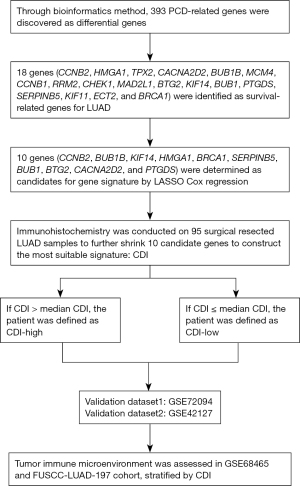
In this study, patients who met the following criteria were selected: (I) histopathological diagnosed with invasive LUAD; (II) with complete OS data; (III) with accessible mRNA expression profile covering all candidate genes; (IV) received standard treatment.
According to the former collection criteria, the mRNA expression matrix and relevant clinical characteristics [including OS, age, gender and tumor node metastasis (TNM) stage] of 476 LUAD patients were acquired from The Cancer Genome Atlas (TCGA) database. Likewise, we included the transcriptome data and survival statistics of a total of 398 qualified LUAD samples from GSE72094 dataset, 443 LUAD samples from GSE68465 dataset, and 173 LUAD samples from GSE42127 dataset, respectively. The “org.Hs.eg.db” R package was subsequently applied to convert ensemble ids to gene symbols.
Furthermore, the gene expression profiles and clinical information of 197 LUAD patients who underwent surgery between September 2011 and May 2016 at the Department of Thoracic Surgery, Fudan University Shanghai Cancer Center (FUSCC-LUAD-197 cohort) were acquired for tumor immune infiltration analysis. The study was conducted in accordance with the Declaration of Helsinki (as revised in 2013). The study was approved by the institutional review board of Fudan University Shanghai Cancer Center (IRB No. 2008223-9). Informed consents were waived due to the retrospective nature of the study.
Screening of differentially expressed genes (DEGs) and functional enrichment analysis
The raw mRNA counts data for DEGs analysis was acquired from the TCGA-LUAD datasets involving 585 LUAD tissues and normal tissues. Subsequently, we applied “DESeq2” R package to screen out DEGs between cancer group and normal group with the following criteria: P<0.05 and |log2FC| >1 (17). Functional enrichment analysis was performed using the “clusterProfiler” (18) and “enrichplot” R packages to identify biological signal pathways significantly associated with the DEGs of the TCGA-LUAD cohort.
Establishment of a PCD-related gene signature and a prognostic index (named CDI)
Kaplan-Meier survival analysis was used to evaluate whether these genes were closely associated with the OS status of LUAD in TCGA-LUAD, GSE68465, GSE72094 cohorts. There were some criteria to meet for PCD-related genes to be admitted to the list of survival-related genes: (I) log-rank P<0.1 in all three cohorts; (II) log-rank P<0.02 in at least two cohorts. We further utilized the LASSO Cox regression and Cox regression in GSE72094 and our cohort, respectively, with the help of R package “glmnet” and the indication of “lambda. min” value (19), as to concentrate on the candidate model genes to establish the optimal prognostic gene signature. By applying Cox regression, we ultimately constructed a prognostic index [cell death index (CDI)] for the precise prediction of LUAD prognosis, combining the regression coefficient (β) with the level of gene expression. CDI = ∑ (βi * expression level of Gene i).
Kaplan-Meier survival curve, survival ROC curve and independent prognostic factor assessment of CDI
Kaplan-Meier survival curves and ROC curves were performed via “survminer” and “timeROC” (20) R package respectively to validate the predictive efficacy of our gene signature on OS in GSE42127 and GSE72094 cohorts. Furthermore, to demonstrate the prognostic value of CDI was independent of other clinical characteristics including age, gender, smoking status and p-TNM stage, we applied univariate and multivariate Cox regression with R in GSE72094 cohort. Hazard ratios (HRs) and 95% confidence intervals (CIs) of each variable were measured simultaneously and P<0.05 was considered as statistical significance.
Group analysis of TME based on CDI level
A group analysis of the TME based on CDI levels was conducted. Gene expression data from the GSE68465 and FUSCC-LUAD-197 cohorts were collected, and the LM22 signatures (21) were downloaded. We assessed whether there was a significant difference in the cell fraction of the tumor immune microenvironment between the high CDI group and the low CDI group, using the median CDI value as the cutoff, with the assistance of the online platform CIBERSORTx (https://cibersortx.stanford.edu/).
Correlation analysis between clinical features and the prognostic signature
Correlation analysis was performed to examine the relationship between clinical features and the prognostic signature. The Wilcoxon test and t-test were employed to evaluate the statistical differences in CDI levels between groups with different p-TNM stages and survival status. All statistical analyses were conducted using R version 4.2.0.
Immunohistochemistry (IHC) staining on tissue microarray and evaluation of immunostaining intensity
Tissue microarrays were constructed using 4-µm-thick sections sliced from tissue blocks. For IHC staining, the sections were incubated with primary polyclonal antibodies for KIF14 (rabbit polyclonal, 26000-1-AP, Proteintech, Wuhan, China; dilution 1:400), CCNB2 (rabbit polyclonal, 21644-1-AP, Proteintech, dilution 1:200), HMGA1 (rabbit polyclonal, 29895-1-AP, Proteintech, dilution 1:400), BubR1 (rabbit polyclonal, 11504-2-AP, Proteintech, dilution 1:400), BTG2 (rabbit polyclonal, 22339-1-AP, Proteintech, dilution 1:400), BUB1 (rabbit polyclonal, 13330-1-AP, Proteintech, dilution 1:100), PTGDS (rabbit polyclonal, 10754-2-AP, Proteintech, dilution 1:400), Maspin (rabbit polyclonal, 11722-1-AP, Proteintech, dilution 1:400), BRCA1 (rabbit polyclonal, 22362-1-AP, Proteintech, dilution 1:100), CACNA2D2 (rabbit polyclonal, 46384-1, Sabbiotech, Nanjing, China; dilution 1:150). The total set of tissue specimens was processed and immunostained in one time to guarantee the objective comparison among samples. The whole process of IHC was performed on the LUAD tissue samples of our cohort (FUSCC-LUAD) and conducted in accordance to the guidance of “An Introduction to the Performance of Immunohistochemistry” (22). The antigenic repair was performed in a microwave using Tris-EDTA (dilution 1:50) and tissue sections were treated with 3% hydrogen peroxide to remove endogenous peroxidase.
IHC-staining was assessed by the immunoreactivity score (IRS). The intensity of staining was defined in four categories (no staining =0, weak staining =1, moderate staining =2 and strong staining =3) and multiplied by the proportion of positive cells (no =0, <10% =1, 10–50% =2, 51–80% =3 and >80% =4) (23). Each section was scored by person who was blinded to the patients’ outcomes.
Statistical analysis
All statistical analyses in this study were conducted via R software (v.2022.12.0 Build 353). Survival curves were conducted by Kaplan-Meier plots and compared via the log-rank test. Student’s t-test or Wilcoxon test was used to analyze differences between groups. P<0.05 was considered statistically significant.
Results
Identification of PCD-related DEGs in LUAD
PCD-related genes were obtained from the table available at https://cdn.amegroups.cn/static/public/jtd-23-1275-1.doc of a previously published article and public data centers such as the FerrDb database and Gene Cards website. Non-coding genes were excluded, resulting in a selection of 1,933 candidate genes for this study. Subsequently, we identified a total of 393 PCD-related DEGs (table available at https://cdn.amegroups.cn/static/public/jtd-23-1275-2.doc) from the TCGA-LUAD cohort using the “DESeq2” R package, comparing cancer and normal groups. The volcano plot (Figure 2A) displays these DEGs.
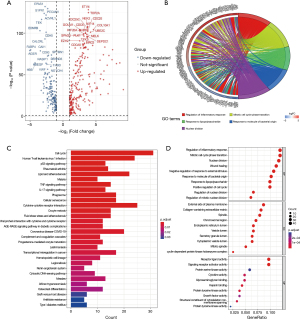
To gain further insights into the biological functions of the DEGs, we performed Kyoto Encyclopedia of Genes and Genomes (KEGG) and Gene Ontology (GO) enrichment analyses. Furthermore, we conducted GO clustering analysis of the DEGs based on the underlying functional signaling pathways, as depicted in Figure 2B. Figure 2C,2D presents the top 30 functional enrichment pathways resulting from the KEGG and GO analyses, respectively. The results revealed that these DEGs were involved in diverse biological pathways, including the cell cycle, p53 signaling pathway, TNF signaling pathway, IL-17 signaling pathway (Figure 2C), as well as the regulation of inflammatory response, mitotic cell cycle phase transition, nuclear division (Figure 2D) and execution phase of apoptosis (P<0.01, GO analysis) which is not shown in Figure 2, etc. The darker red modules or bubbles indicate a closer correlation between biological pathways and DEGs.
Establishment of the gene signature to predict the prognosis of LUAD
Based on the PCD-related DEGs, we downloaded survival information of LUAD patients and performed Kaplan-Meier survival analysis for primary screening of survival-related genes in the GSE68465, GSE72094, and TCGA-LUAD cohorts. We identified 102 survival-related genes in GSE68465, 97 genes in TCGA-LUAD, and 94 genes in GSE72094, all meeting the cut-off of log-rank P<0.1 in Kaplan-Meier analysis (Tables S1,S2). The intersection of prognostic genes in the three cohorts yielded a total of 39 genes (Figure 3A). To ensure a more significant association with LUAD prognosis and to reduce statistical omission, we retained genes that exhibited log-rank P<0.02 in at least two cohorts. Consequently, 18 genes (CCNB2, HMGA1, TPX2, CACNA2D2, BUB1B, MCM4, CCNB1, RRM2, CHEK1, MAD2L1, BTG2, KIF14, BUB1, PTGDS, SERPINB5, KIF11, ECT2, BRCA1) were determined as the survival-related genes for constructing the LUAD prognostic model. Then we applied LASSO Cox regression in GSE72094 using RNA-Seq data. We determined −4.7 as the best cutoff value for log λ, corresponding to the minimum partial likelihood deviance and 10 genes (CCNB2, BUB1B, KIF14, HMGA1, BRCA1, SERPINB5, BUB1, BTG2, CACNA2D2, and PTGDS) were enrolled as candidates for our gene signature (Figure 3B,3C). Next, we investigated the correlation between each candidate model gene on RNA-Seq level in GSE72094 cohort. Overall, there were significant correlations between each candidate gene (Figure 3D). And their respective association with OS of LUAD was verified by Kaplan-Meier survival analysis (Figure S1).
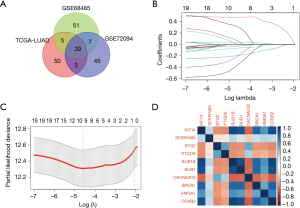
Next, we performed IHC of the 10 candidate genes on 95 surgically resected LUAD samples of our cohort (FUSCC-LUAD), in order to further shrink candidate genes to construct the most suitable signature. Different degrees of immunostaining intensity are shown as Figure 4. The immunostaining results are presented in Figures S2,S3. Then we randomly divided FUSCC-LUAD cohort into training set and validation set on a 3:1 ratio and applied Cox regression in training set using IRS of the 10 candidate genes and OS data. According to the coefficients table of Cox regression (Table S3) followed by mathematical approximation, we finally built a 9-gene signature as our prognostic model. Based on all the aforementioned analyses, we generated the formula for the CDI as a prognostic score: CDI = (0.3033 × expression of CCNB2) + (0.4775 × expression of HMGA1) + (−0.1952 × expression of CACNA2D2) + (−0.3531 × expression of BUB1B) + (−0.0663 × expression of BTG2) + (0.0627 × expression of KIF14) + (−0.0291 × expression of PTGDS) + (0.4000 × expression of SERPINB5) + (−0.2029 × expression of BRCA1). Based on the formula above and the RNA-Seq data of the 9 PCD-related genes, CDI was calculated for each patient in our cohort (FUSCC-LUAD). Based on median CDI, patients in training set were divided into the high-CDI group (n=36) and low-CDI group (n=36). Receiver operating characteristic (ROC) analyses were further conducted in the validation set of FUSCC-LUAD cohort to evaluate the prognostic value of CDI as a continuous variable. The results showed that CDI had excellent predictive efficiency for the OS of LUAD [24-month area under the curve (AUC): 0.773, 36-month AUC: 0.750, 60-month AUC: 0.809; Figure 5A]. The Kaplan-Meier analysis curve of the training set demonstrated a significant difference in survival status between the two groups (P<0.0001, Figure 5B).
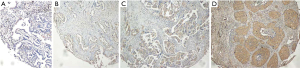
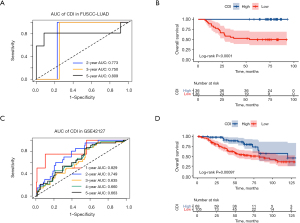
External validation of the prognosis predictive value of the nine-gene signature
We used the GSE72094 and GSE42127 as validation cohorts to confirm the predictive efficiency of the nine-gene signature. The AUCs of the GSE42127 cohort for the 1-, 2-, 3-, and 5-year OS rates were 0.829, 0.749, 0.635, and 0.663, respectively (Figure 5C). Based on the median CDI of GSE72094 cohort, 328 LUAD patients in GSE72094 were divided into high-CDI and low-CDI groups. As for 173 patients in GSE42127, we chose CDI =−0.23 as the cutpoint indicated by the “survminer” package in R. Consistent with the results of the Kaplan-Meier analysis from the training cohort, patients in the validation group also showed significantly lower OS rates in the high-CDI group compared to the low-CDI group (P<0.001 in GSE42127, Figure 5D). And the AUCs of the GSE72094 cohort for the 1-, 2-, 3-, and 5-year OS rates were 0.646, 0.724, 0.717, and 0.737, respectively (Figure 6A). Patients in GSE72094 cohort showed significantly lower OS rates in the high-CDI group than in the low-CDI group (P<0.0001 in GSE72094, Figure 6B). Consequently, CDI was confirmed as an outstanding indicator for the survival outcome of LUAD patients.
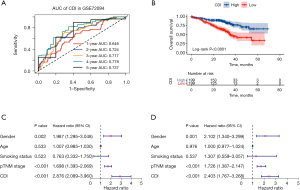
To provide a more convincing assessment of CDI’s predictive value for the prognosis of LUAD patients, we included CDI and other clinical characteristics of GSE72094 cohort in Cox regression analyses. Univariable analyses demonstrated that high CDI (HR =2.876, 95% CI: 2.089–3.960, P<0.001) and advanced pTNM stage (HR =1.698, 95% CI: 1.393–2.069, P<0.001) were closely associated with worse OS in LUAD patients (Figure 6C). Multivariable analyses further verified that CDI (HR =2.403, 95% CI: 1.767–3.268, P<0.001) and pTNM stage (HR =1.726, 95% CI: 1.387–2.147, P<0.001) were independent predictors of OS in LUAD patients (Figure 6D).
Correlation investigation between clinical characteristics and CDI level
To investigate the association of traditional clinical features with CDI levels, the patients in the training cohort were divided into two groups based on their vital status (alive or dead). The alive group exhibited significantly lower CDI than the dead group, as demonstrated by the Wilcoxon test (P<0.0001, Figure 7A). Likewise, the patients were divided into four groups according to p-TNM stage (I, II, III, or IV). T-test showed significant differences in CDI levels among stage I, stage II, stage III and stage IV groups (Figure 7B). Overall, the evidence above indicated that CDI has a close association with classic clinical features, including survival status and p-TNM stage.
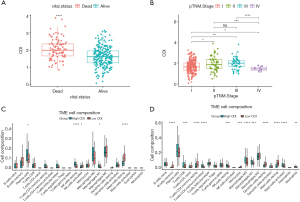
Investigation of immune cell infiltration in CDI-high versus CDI-low
Firstly, we divided the GSE68465 and FUSCC-LUAD-197 cohorts into high-CDI and low-CDI groups based on the median CDI in each cohort. Then the fractions of 22 types of immune cells in the TME of each group were calculated using the online platform CIBERSORTx, using LM22 expression signatures as a reference. Remarkably, the resting NK-cells in both cohorts were significantly higher in the high-CDI group than in the low-CDI group. Conversely, the resting mast cells in both cohorts were significantly lower in the high-CDI group than in the low-CDI group (Figure 7C,7D, FUSCC-LUAD-197 cohort and GSE68465 cohort). Interestingly, the high-CDI group exhibited significantly higher M1-like macrophages in both cohorts.
Discussion
Recent evidence suggests that PCD plays a critical role in diverse biological processes and significantly affects the development and metastasis of cancer (24). Therefore, PCD-related genes have the potential to serve as prognostic indicators for malignant tumors, which is consistent with the results of the prognostic model we constructed in this study. To our knowledge, this is the first time a PCD-related gene signature has been proposed based on comprehensive exploration stream mechanisms related to the analysis of all the twelve forms of PCD. In conclusion, the proposed PCD-related gene signature shows great potential in predicting the OS and recurrence-free survival of invasive LUAD patients, opening new avenues for evaluating the clinical outcomes of LUAD patients. Furthermore, we analyzed the correlation of CDI with clinical features, the TME, to investigate possible downstream mechanisms related to the prognosis of LUAD.
The prognostic gene signature we created in the present study consists of 9 PCD-related genes (CACNA2D2, BUB1B, BTG2, KIF14, PTGDS, SERPINB5, CCNB2, HMGA1, BRCA1), some of which have been widely reported on its function and some not. Our study found that the relatively higher expression of CACNA2D2 was associated with a better prognosis. The CACNA2D2 gene, a new subunit of the Ca2+-channel complex, has been identified in the homozygous deletion region of chromosome 3p21.3 in human lung cancer. Previous study showed that overexpression of CACNA2D2 have effects on intracellular Ca2+ contents, mitochondria homeostasis, cell proliferation, and apoptosis (25). BUB1B is a critical mitotic checkpoint protein which plays a central role in spindle assembly checkpoint signaling and stable attachment of kinetochores to spindle microtubules (26). It has been reported that BUB1B suppression inhibits primary tumor growth and reduces metastasis to the lung and lymph nodes, which is consistent with its indicating capacity of poor prognosis in our study. Next, BTG2 plays an important role in cell proliferation, differentiation, apoptosis and DNA damage repair. Overexpression of BTG2 can inhibit the proliferation and invasion of some tumors, including lung cancer cells (27). We found the expression of SerpinB5 was much lower in LUAD samples than normal tissue, which may be explained by the fact that SerpinB5 can inhibit tumor cell infiltration and metastasis, promote tumor cell apoptosis, and inhibit tumor vascular growth (28). KIF14 is potentially oncogenic and can act as a chromokinesin via binding to microtubules and chromatin during the bipolar spindle formation (28). CCNB2 belongs to type B cell cycle family protein, which is located on chromosome 15q22. One study has shown that overexpression of CCNB2 is associated with the development and deterioration of colorectal and lung cancer (29). Our study also verified that the expression of CCNB2 is a risk factor for LUAD. Glycoprotein prostaglandin D2 synthase (PTGDS) is a member of the lipocalin superfamily and plays dual roles in prostaglandins metabolism and lipid transport. PTGDS has been involved in various cellular processes including the tumorigenesis of solid tumors (29). Previous study has delineated some germline mutations, including BRCA1/2 mutation. BRCA1 protein is required for maintenance of chromosomal stability, thereby protecting the genome from damage (30). New data also show that BRCAs transcriptionally regulate some genes involved in DNA repair, the cell cycle, and apoptosis (31). HMGA1 is an architectural transcription factor involved in the processes of DNA transcription, replication, recombination, and repair (32). HMGA1 is highly expressed in a variety of cancers, including early-stage lung cancer. We also observed that HMGA1 expression was extremely higher in LUAD tissues.
Tumor cells can survive because the TME allows them to escape immune surveillance and drug interference (33). M1-like and M2-like macrophages are closely associated with inflammatory responses, with M1-like macrophages participating mainly in pro-inflammatory responses and M2-like macrophages mainly involved in anti-inflammatory responses (34). Interestingly, our study revealed a negative correlation between the M2-like/M1-like macrophage ratio and CDI score in both cohorts, indicating that pro-inflammatory responses are stronger in the high-CDI group, which had a poorer prognosis. It is important to note that while M1-like macrophages possess the ability to directly kill tumor cells by releasing cytotoxic molecules and ROS, the TME can present inhibitory factors, such as suppressive factors released by tumor cells, which may hinder the anti-tumor activity of M1-like macrophages (35). Therefore, we hypothesize that a high CDI level is associated with the activated release of immune-suppressive factors from LUAD tumor cells, such as transforming growth factor beta, programmed cell death ligand 1, indoleamine 2,3-dioxygenase, which requires further study. Additionally, we found that resting mast cells are significantly lower in the high-CDI group, implying a stronger immune function of mast cells in the TME. Previous studies have shown that mast cells contribute to tumor progression, particularly through their ability to promote tumor vascularization and secrete proteases that facilitate vascular invasion and accelerate metastatic spread (36,37). In conclusion, it has been suggested that mast cells contribute, at least partially, to the micrometastasis that occurs at early stages of tumor development and to the angiogenesis, which may account for the poor survival of LUAD patients in the high-CDI group.
Although the model exhibited good predictive accuracy for the prognosis of patients with LUAD, the study still has several limitations. Firstly, the IHC results are dependent on the kind of antibodies, and the assessments of IHC might be subjective as the immunostaining intensity could be judged individually by different observers. Secondly, the validation of our model was primarily based on the patients from public database and a single institution. Therefore, future prospective studies in multiple institutions are required to confirm the effectiveness of the prognostic model.
Conclusions
In our study, a 9-gene signature (CCNB2, BUB1B, KIF14, HMGA1, BRCA1, SERPINB5, BUB1, BTG2, CACNA2D2, and PTGDS) based on PCD-related genes was identified and validated to accurately predict the prognosis of LUAD patients. A higher CDI score indicates a poorer prognosis. The CDI could serve as a valuable prognostic indicator and guide personalized therapeutic strategies for LUAD.
Acknowledgments
The authors thank the subjects for their participation in this study.
Funding: This work was supported by
Footnote
Reporting Checklist: The authors have completed the TRIPOD reporting checklist. Available at https://jtd.amegroups.com/article/view/10.21037/jtd-23-1275/rc
Data Sharing Statement: Available at https://jtd.amegroups.com/article/view/10.21037/jtd-23-1275/dss
Peer Review File: Available at https://jtd.amegroups.com/article/view/10.21037/jtd-23-1275/prf
Conflicts of Interest: All authors have completed the ICMJE uniform disclosure form (available at https://jtd.amegroups.com/article/view/10.21037/jtd-23-1275/coif). The authors have no conflicts of interest to declare.
Ethical Statement: The authors are accountable for all aspects of the work in ensuring that questions related to the accuracy or integrity of any part of the work are appropriately investigated and resolved. The study was conducted in accordance with the Declaration of Helsinki (as revised in 2013). The study was approved by the institutional review board of Fudan University Shanghai Cancer Center (IRB No. 2008223-9). Informed consents were waived due to the retrospective nature of the study.
Open Access Statement: This is an Open Access article distributed in accordance with the Creative Commons Attribution-NonCommercial-NoDerivs 4.0 International License (CC BY-NC-ND 4.0), which permits the non-commercial replication and distribution of the article with the strict proviso that no changes or edits are made and the original work is properly cited (including links to both the formal publication through the relevant DOI and the license). See: https://creativecommons.org/licenses/by-nc-nd/4.0/.
References
- Chen Y, Tang L, Huang W, et al. Identification and validation of a novel cuproptosis-related signature as a prognostic model for lung adenocarcinoma. Front Endocrinol (Lausanne) 2022;13:963220. [Crossref] [PubMed]
- Zhou J, Wang X, Li Z, et al. Construction and analysis of a novel ferroptosis-related gene signature predicting prognosis in lung adenocarcinoma. FEBS Open Bio 2021;11:3005-18. [Crossref] [PubMed]
- Chan TA, Yarchoan M, Jaffee E, et al. Development of tumor mutation burden as an immunotherapy biomarker: utility for the oncology clinic. Ann Oncol 2019;30:44-56. [Crossref] [PubMed]
- Strasser A, Vaux DL. Cell Death in the Origin and Treatment of Cancer. Mol Cell 2020;78:1045-54. [Crossref] [PubMed]
- Tang D, Kang R, Berghe TV, et al. The molecular machinery of regulated cell death. Cell Res 2019;29:347-64. [Crossref] [PubMed]
- Yang Y, Chen Y, Wu JH, et al. Targeting regulated cell death with plant natural compounds for cancer therapy: A revisited review of apoptosis, autophagy-dependent cell death, and necroptosis. Phytother Res 2023;37:1488-525. [Crossref] [PubMed]
- Fuchs Y, Steller H. Programmed cell death in animal development and disease. Cell 2011;147:742-58. [Crossref] [PubMed]
- Sun Y, Peng ZL. Programmed cell death and cancer. Postgrad Med J 2009;85:134-40. [Crossref] [PubMed]
- Gong L, Huang D, Shi Y, et al. Regulated cell death in cancer: from pathogenesis to treatment. Chin Med J (Engl) 2023;136:653-65. [Crossref] [PubMed]
- Liu J, Hong M, Li Y, et al. Programmed Cell Death Tunes Tumor Immunity. Front Immunol 2022;13:847345. [Crossref] [PubMed]
- Qi X, Li Q, Che X, et al. Application of Regulatory Cell Death in Cancer: Based on Targeted Therapy and Immunotherapy. Front Immunol 2022;13:837293. [Crossref] [PubMed]
- Krishna S, Overholtzer M. Mechanisms and consequences of entosis. Cell Mol Life Sci 2016;73:2379-86. [Crossref] [PubMed]
- Mishra AP, Salehi B, Sharifi-Rad M, et al. Programmed Cell Death, from a Cancer Perspective: An Overview. Mol Diagn Ther 2018;22:281-95. [Crossref] [PubMed]
- Pallichankandy S, Thayyullathil F, Cheratta AR, et al. Targeting oxeiptosis-mediated tumor suppression: a novel approach to treat colorectal cancers by sanguinarine. Cell Death Discov 2023;9:94. [Crossref] [PubMed]
- Tong X, Tang R, Xiao M, et al. Targeting cell death pathways for cancer therapy: recent developments in necroptosis, pyroptosis, ferroptosis, and cuproptosis research. J Hematol Oncol 2022;15:174. [Crossref] [PubMed]
- Liu J, Kuang F, Kang R, et al. Alkaliptosis: a new weapon for cancer therapy. Cancer Gene Ther 2020;27:267-9. [Crossref] [PubMed]
- Tang B, Xu W, Wang Y, et al. Identification of critical ferroptosis regulators in lung adenocarcinoma that RRM2 facilitates tumor immune infiltration by inhibiting ferroptotic death. Clin Immunol 2021;232:108872. [Crossref] [PubMed]
- Wu T, Hu E, Xu S, et al. clusterProfiler 4.0: A universal enrichment tool for interpreting omics data. Innovation (Camb) 2021;2:100141. [Crossref] [PubMed]
- Friedman J, Hastie T, Tibshirani R. Regularization Paths for Generalized Linear Models via Coordinate Descent. J Stat Softw 2010;33:1-22.
- Blanche P, Dartigues JF, Jacqmin-Gadda H. Estimating and comparing time-dependent areas under receiver operating characteristic curves for censored event times with competing risks. Stat Med 2013;32:5381-97. [Crossref] [PubMed]
- Newman AM, Liu CL, Green MR, et al. Robust enumeration of cell subsets from tissue expression profiles. Nat Methods 2015;12:453-7. [Crossref] [PubMed]
- Magaki S, Hojat SA, Wei B, et al. An Introduction to the Performance of Immunohistochemistry. Methods Mol Biol 2019;1897:289-98. [Crossref] [PubMed]
- Kahlmeyer A, Stöhr CG, Hartmann A, et al. Expression of PD-1 and CTLA-4 Are Negative Prognostic Markers in Renal Cell Carcinoma. J Clin Med 2019;8:743. [Crossref] [PubMed]
- Su Z, Yang Z, Xu Y, et al. Apoptosis, autophagy, necroptosis, and cancer metastasis. Mol Cancer 2015;14:48. [Crossref] [PubMed]
- Carboni GL, Gao B, Nishizaki M, et al. CACNA2D2-mediated apoptosis in NSCLC cells is associated with alterations of the intracellular calcium signaling and disruption of mitochondria membrane integrity. Oncogene 2003;22:615-26. [Crossref] [PubMed]
- Chen H, Lee J, Kljavin NM, et al. Requirement for BUB1B/BUBR1 in tumor progression of lung adenocarcinoma. Genes Cancer 2015;6:106-18. [Crossref] [PubMed]
- Yang W, Wei C, Cheng J, et al. BTG2 and SerpinB5, a novel gene pair to evaluate the prognosis of lung adenocarcinoma. Front Immunol 2023;14:1098700. [Crossref] [PubMed]
- Wang H, Tang F, Tang P, et al. Noncoding RNAs-mediated overexpression of KIF14 is associated with tumor immune infiltration and unfavorable prognosis in lung adenocarcinoma. Aging (Albany NY) 2022;14:8013-31. [Crossref] [PubMed]
- Li MJ, Yan SB, Chen G, et al. Upregulation of CCNB2 and Its Perspective Mechanisms in Cerebral Ischemic Stroke and All Subtypes of Lung Cancer: A Comprehensive Study. Front Integr Neurosci 2022;16:854540. [Crossref] [PubMed]
- Fu F, Tao X, Jiang Z, et al. Identification of Germline Mutations in East-Asian Young Never-Smokers with Lung Adenocarcinoma by Whole-Exome Sequencing. Phenomics 2023;3:182-9. [Crossref] [PubMed]
- De Luca P, De Siervi A. Critical role for BRCA1 expression as a marker of chemosensitivity response and prognosis. Front Biosci (Elite Ed) 2016;8:72-83. [Crossref] [PubMed]
- Saed L, Jeleń A, Mirowski M, et al. Prognostic Significance of HMGA1 Expression in Lung Cancer Based on Bioinformatics Analysis. Int J Mol Sci 2022;23:6933. [Crossref] [PubMed]
- Zou Y, Zheng S, Deng X, et al. The Role of Circular RNA CDR1as/ciRS-7 in Regulating Tumor Microenvironment: A Pan-Cancer Analysis. Biomolecules 2019;9:429. [Crossref] [PubMed]
- Pan Y, Yu Y, Wang X, et al. Tumor-Associated Macrophages in Tumor Immunity. Front Immunol 2020;11:583084. [Crossref] [PubMed]
- Simiczyjew A, Dratkiewicz E, Mazurkiewicz J, et al. The Influence of Tumor Microenvironment on Immune Escape of Melanoma. Int J Mol Sci 2020;21:8359. [Crossref] [PubMed]
- Ranieri G, Ammendola M, Patruno R, et al. Tryptase-positive mast cells correlate with angiogenesis in early breast cancer patients. Int J Oncol 2009;35:115-20. [Crossref] [PubMed]
- Ribatti D, Finato N, Crivellato E, et al. Angiogenesis and mast cells in human breast cancer sentinel lymph nodes with and without micrometastases. Histopathology 2007;51:837-42. [Crossref] [PubMed]