Transcriptomic analysis reveals the potential crosstalk genes and immune relationship between Crohn’s disease and atrial fibrillation
Highlight box
Key findings
• Our study identifies CXCL16 and HLA-DPB1 as pivotal genes associated with the development of both disorders, highlighting the significant involvement of immune responses in the complex interplay between atrial fibrillation (AF) and Crohn’s disease (CD).
What is known and what is new?
• AF and CD are believed to involve inflammatory and autoimmune processes.
• Through the integration of least absolute shrinkage and selection operator and support vector machine algorithms, we pinpointed CXCL16 and HLA-DPB1 as shared genes, and our immune cell infiltration analysis demonstrated increased levels of CD4+ T cells and CD8+ T cells, as well as dendritic cells, in individuals with both AF and CD.
What is the implication, and what should change now?
• This study uncovers CXCL16 and HLA-DPB1 as shared diagnostic genes, shedding light on the genetic interplay between AF and CD, emphasizing the involvement of CD4+ and CD8+ T cells, and dendritic cells, providing potential targets for immunomodulatory therapies and opening avenues for further research to enhance patient outcomes.
Introduction
CD is a multifaceted inflammatory bowel disorder characterized by chronic inflammation of the gastrointestinal tract. Its pathogenesis is a complex interplay of genetic susceptibility, environmental factors, and aberrant immune responses (1). Recent genome-wide association studies have identified multiple susceptibility loci, including genes that play a role in regulating immune response, intestinal barrier function, and autophagy, all of which contribute to the development and progression of CD (2). Moreover, dysbiosis of the gut microbiome has also been implicated in the pathogenesis of CD, with changes in the composition and function of the microbiota contributing to the persistence of chronic inflammation (3).
CD exhibits a diverse global epidemiology, with a higher incidence reported in North America and Europe (4). Various risk factors have been identified for CD, including family history of inflammatory bowel disease, smoking, urban living, and exposure to certain infections (5). Furthermore, CD has been associated with an increased susceptibility to other diseases, such as osteoporosis, anemia, and malignancies like lymphoma and colorectal cancer (6). Despite the significant progress in understanding the pathogenesis of CD, the management of this complex disorder remains a challenge.
Atrial fibrillation (AF) is a prevalent cardiac arrhythmia characterized by the irregular and rapid contractions of the atria, which can manifest in symptoms such as palpitations, fatigue, and dyspnea (7). The pathophysiology of AF is multifaceted, involving complex structural and electrical remodeling of the atria. Structural remodeling, encompassing fibrosis and hypertrophy, induces electrical inhomogeneities, slows conduction velocity, and heightens vulnerability to reentry (8). Meanwhile, electrical remodeling, which is defined by changes in ion channel expression and function, may alter action potential duration and conduction velocity, ultimately leading to the initiation and perpetuation of AF (9). Moreover, evidence suggests that inflammation, oxidative stress, and autonomic nervous system imbalance may also contribute to the pathogenesis of AF (10,11).
Recent investigations have unveiled a potential correlation between AF and CD. One of these inquiries reported that individuals affected by CD confronted a higher likelihood of developing AF than the general population, with a corresponding increase in risk proportionate to the severity and duration of the disease (12). Another study has demonstrated an elevated prevalence of AF among patients with inflammatory bowel disease, including CD (13). Although the mechanisms underlying this association are not fully understood, it has been posited that chronic inflammation and oxidative stress may contribute to the pathogenesis of both AF and CD (14,15). Additionally, some of the pharmaceutical agents prescribed for the treatment of CD, such as corticosteroids and anti-tumor necrosis factor (TNF) agents, have been linked to an augmented risk of AF (16,17). Thus, further investigations are warranted to unravel the precise relationship between AF and CD and to identify potential therapeutic modalities for these patients.
With the advent of advanced high-throughput sequencing, gene expression profiling, and network analysis, bioinformatics has garnered considerable interest in the study of disease pathogenesis, diagnosis, and treatment. In the study, we utilized bioinformatics methodologies to identify potential crosstalk genes that link AF and CD, and to analyze their interaction with infiltrating immune cells. Our primary aim was to gain an enhanced understanding of the complex pathophysiological mechanisms underlying the relationship between AF and CD. We present this article in accordance with the STREGA reporting checklist (available at https://jtd.amegroups.com/article/view/10.21037/jtd-23-1078/rc).
Methods
The flow diagram of the analysis process is presented in Figure 1. The study was conducted in accordance with the Declaration of Helsinki (as revised in 2013).
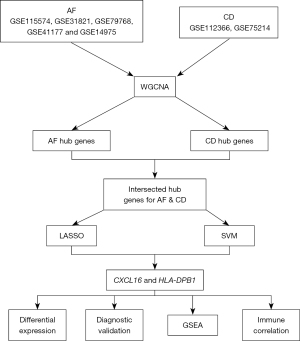
Acquisition of datasets
We employed the “GEOquery” R program to retrieve two CD datasets, namely GSE112366 and GSE75214, as well as five AF datasets, including GSE115574, GSE31821, GSE79768, GSE41177, and GSE14975, from the Gene Expression Omnibus (GEO; https://www.ncbi.nlm.nih.gov/geo/) database. The GSE112366 dataset comprises 362 CD samples and 26 normal samples, while GSE75214 includes 75 CD samples and 22 control samples. The AF datasets collectively encompass 56 sinus rhythm (SR) samples and 83 AF samples. To eliminate batch effects and create a unified GEO dataset, the Bioconductor “sva” R software was applied.
WGCNA
The weighted gene co-expression network analysis (WGCNA) is a popular systems biology approach for analyzing high-throughput gene expression data (18). In this study, we employed the WGCNA R software package to construct a co-expression network. To begin with, hierarchical clustering, a method for grouping samples or genes based on their similarity, was performed using the standard R function “Hclust”. This step aimed to identify any potential outliers that might affect downstream analyses. To retrieve a scale-free network, which is characterized by a few highly connected hub genes and many poorly connected peripheral genes, we utilized the “pickSoftThreshold” function. This function helps to determine an appropriate soft thresholding power β, which transforms the gene expression similarity matrix into a weighted adjacency matrix. The adjacency function was then applied to transform the similarity matrix into an adjacency matrix, utilizing the soft-thresholding parameter β. This adjacency matrix was used to construct a topological overlap matrix (TOM), which captures both direct and indirect correlations between genes while minimizing the impact of noise and false associations. We used hierarchical clustering and the dynamic tree cut algorithm to detect modules, which are groups of genes that are co-expressed and biologically related. Finally, we conducted Pearson correlation tests to examine the correlation between the identified modules and the disease status of patients.
Machine learning
Two machine learning algorithms, least absolute shrinkage and selection operator (LASSO) and support vector machine (SVM), were employed to identify the optimal diagnostic genes. LASSO regression, a popular variable selection technique, was performed using the “glmnet” language package in R to identify the best predictors of AF and CD from the intersected genes obtained from WGCNA analysis. LASSO regression uses a penalty term to shrink the regression coefficients of irrelevant variables to zero, while keeping relevant variables. SVM, a widely used supervised learning algorithm, was also used to establish a threshold between the two classes of AF and CD. SVM seeks to find the best hyperplane that separates the data points of different classes with the largest margin, allowing for accurate classification based on one or several feature vectors.
Evaluating the immune cell infiltration
The 23 types of immune cells were calculated using single-sample gene set enrichment analysis (ssGSEA). The immune populations that exhibited zero score in over 80% sample were removed from further study. Then the immunologic features of all samples were evaluated using the ssGSEA technique using the “GSVA” R package (19).
Statistical analysis
Data analysis and statistical analyses were conducted by R 4.2.2. The statistical difference between the two groups was assessed using the Wilcox test. The Pearson correlation was employed to examine the association between the expression levels of biomarkers linked to immune cells, with statistical significance set at a P value of 0.05.
Results
Merging GEO data
For the AF study, we integrated five GEO datasets, namely GSE115574, GSE31821, GSE79768, GSE41177, and GSE14975, by eliminating the batch effects with the aid of the “sva” package implemented in R software. The merged datasets were then normalized using the “normalizeBetweenArrays” function of the “limma” package in R software, which adjusted the expression values of genes to obtain similar distributions across the entire set of arrays. Principal component analysis (PCA) plots validated the successful removal of batch effects (Figure S1A,S1B).
WGCNA network construction and module identification
To identify the subpopulation of genes associated with the specific disease status in AF and CD, we herein used the method of WGCNA to dig out the hub genes. To guarantee a scale-free network, we computed the scale-free fit index and mean connectivity. The power of β=6 was chosen for the soft thresholding for AF dataset and the power of β=11 was chosen for CD dataset (Figure S2A,S2B). Then the genes of both datasets were clustered based on a dissimilarity measure (1 − TOM) as visualized by dendrogram (Figure 2A,2B). To retrieve the subpopulation of genes associated with clinical diseases, we performed Pearson regression to investigate the correlation between gene modules with clinical traits. In AF dataset (Figure 2C), nine modules were obtained by co-expression network based on AF samples and we obtained two modules including MEyellow and MEblue due to their strong positive correlating with AF (R=0.37, P<0.001 and R=0.38, P<0.001). In CD dataset, 11 modules were obtained by co-expression network based on CD samples (Figure 2D). The MEtan had the highest positive correlation with CD (R=0.8, P<0.001) while MEgreenyellow had the strongest negative correlation with CD (R=−0.6, P<0.001). To sum up, 348 genes of MEyellow and MEblue in AF dataset were selected as AF hub genes and 701 genes from MEtan and MEgreenyellow in CD dataset were selected as CD hub genes. After intersection of AF and CD hub genes, we finally obtained 30 genes that were potentially involved in the pathological progression of both AF and CD diseases (Figure 2E).
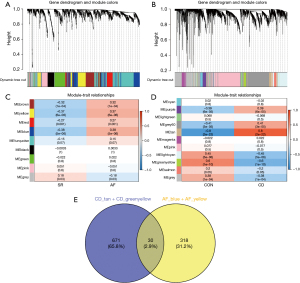
Enrichment of the hub genes identified by WGCNA
To decipher the function of the hub genes in AF and CD, we conducted Gene Ontology (GO) enrichment analysis on the key gene modules derived from WGCNA. In the blue module of AF, the hub genes were significantly enriched in heart contraction, heart processes and muscle contraction, with considerable proportion of genes located on contractile fiber (Figure 3A). Intriguingly, genes in yellow module were enriched in immune related pathways such as leukocyte migration and leukocyte mediated immunity, suggesting that the activation of immune system may potentially participated in the pathology of AF (Figure 3B). In the Tan module of CD, immune related GO terms were significantly enriched such as responses to IFN gamma and defense response to virus (Figure 3C). While in yellowgreen module which negatively correlated with CD, we found that respiration and mitochondrial related pathways were significantly enriched (Figure 3D). Notably, in the 30 intersected genes, major histocompatibility complex (MHC) related pathways and antigen processing related pathways were enriched, suggesting that they are activated in both diseases (Figure 3E). Figure 3F illustrates a network of gene-pathway associations that depict the pathways connected to commonly differentially expressed genes (DEGs).
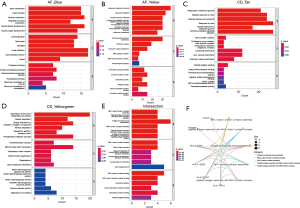
Identification of diagnostic genes via machine learning
In an effort to identify the potential diagnostic genes in both AF and CD, we combined LASSO and SVM algorithms to obtain the most robust diagnostic genes. Briefly, LASSO analysis in AF datasets identified five out of 26 hub cross genes under the most appropriate λ=0.0629 (Figure 4A). SVM screened 25 genes in AF (Figure 4B). In CD, LASSO analysis obtained five genes under the most appropriate λ=0.0754 and SVM obtained 22 genes as diagnostic hub genes for CD (Figure 4C,4D). Finally, intersection of two algorithms calculated based on two diseases retrieved two overlapping genes, namely CXCL16 and HLA-DPB1 (Figure 4E).
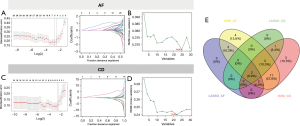
The diagnostic validation and expression of CXCL16 and HLA-DPB1 in AF and CD
CXCL16 and HLA-DPB1 were selected as shared diagnostic biomarkers in both AF and CD. In test cohort of CD and AF, both markers showed wonderful diagnostic values as indicated by receiver operating characteristic (ROC) curves (Figure 5A,5B). Then their expression pattern was visualized by boxplot, which showed that CXCL16 and HLA-DPB1 were both significantly upregulated in both CD and AF (Figure 5C,5D). The diagnostic efficacy of CXCL16 and HLA-DPB1 in CD was further validated in validation cohort GSE112366. In AF, the diagnostic efficacy of CXCL16 and HLA-DPB1 was validated in GSE41177, GSE115574, GSE79768, GSE31821, and GSE14975. These observations showed that CXCL16 and HLA-DPB1 could perform robustly in both AF and CD diseases.
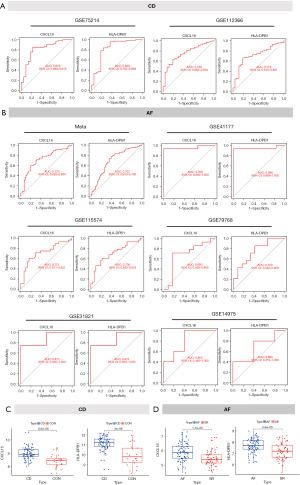
GSEA analysis of CXCL16 and HLA-DPB1 in AF and CD
To decipher the molecular function of CXCL16 and HLA-DPB1 in AF and CD, we additionally performed GSEA analysis. In AF, patients with high expression of CXCL16 were enriched in Kyoto Encyclopedia of Genes and Genomes (KEGG) term associated with immunity such as chemokine signaling and cytokine-cytokine receptor interaction (Figure 6A). Likewise, high expression of HLA-DPB1 was associated with cell adhesion molecules and chemokine signaling (Figure 6B). In parallel, similar results was obtained in CD patients (Figure 6C,6D), suggesting that these two markers were strongly associated with immune activation.
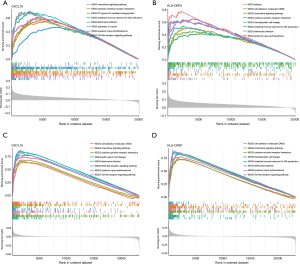
Immune profile study and correlation with diagnostic markers
Given the strong immune associated nature of two biomarkers, we next explored their expression with immune infiltration. We first quantified the infiltration of various immune cells and found that some adaptive immune population such as CD4+ and CD8+ T cells and innate immune populations such as dendritic cells were significantly upregulated in AF patients (Figure 7A). In CD patients, we observed that almost all immune cells were significantly upregulated, which may be due to the inflammatory nature of CD (Figure 7B). Pearson correlation study showed that the two biomarkers were strongly correlated with most types of immune cells in both AF and CD (Figure 7C,7D).
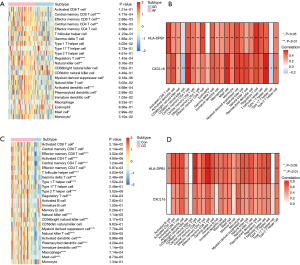
Discussion
This study endeavored to investigate potential shared genes, biological pathways, and associated immune cells between AF and CD by employing bioinformatics and machine learning techniques. Specifically, we utilized WGCNA for the first time to construct a gene co-expression network, and subsequently applied machine learning algorithms to identify two key genes: CXCL16 and HLA-DPB1. Additionally, our immune infiltration analysis highlighted the possible critical roles of CD4+ and CD8+ T cells, as well as dendritic cells, in the pathogenesis of AF and CD.
Our study aimed to explore the enrichment of MHC-related and antigen processing-related pathways among the 30 intersecting genes. The MHC region contains a cluster of genes involved in the immune system, with the MHC-related pathway referring to the molecular pathways that are regulated by MHC genes and their products. These pathways play a crucial role in immune recognition and the subsequent activation of immune responses against pathogens, tumor cells, and self-antigens. Dysregulation of the MHC-related pathway can lead to autoimmune diseases, allergies, and infections (20). Although the mechanisms underlying MHC involvement in autoimmune diseases are not fully understood, one long-standing hypothesis suggests that the loss of immune tolerance to self-antigens is attributed to anomalies in the presentation of self or foreign peptides to self-reactive T lymphocytes resulting from aberrant class II expression. Hence, the targeting of specific self-antigens could be determined by distinct MHC class II (MHCII) alleles, potentially resulting in disease-specific associations. A recent high-density mapping of the MHC region has revealed the involvement of HLA-DRB1*01:03 and HLA-C*06:02 in CD among European populations (21). MHC molecules may also play a role in regulating the immune response to gut microbiota (22). Various investigations have demonstrated that specific genetic variations in the MHC region are correlated with the pathogenesis of CD. The IBD3 region, one of the most polymorphic regions in the MHC, harbors several candidate genes, including TNF, LTA, and NFKB1 (23). The proteins encoded by TNF and LTA genes are significant players in the inflammatory response and cell apoptosis, and their expression levels are closely associated with the onset and progression of CD (24-26). The protein encoded by the NFKB1 gene is a fundamental constituent of the NF-κB signaling pathway and is also implicated in the pathogenesis of IBD. Moreover, MHC class I and class II molecules are key regulators of IBD onset. Class I molecules are predominantly expressed on intestinal epithelial cells and are vital for mounting an immune response and clearing intestinal bacteria, while class II molecules are primarily expressed on immune cells and modulate T cell and B cell immune responses (27,28). Recent research has suggested that specific subsets of immune cells, such as T-lymphocytes and macrophages, may contribute to the pathogenesis of AF. These cells can infiltrate the atrial tissue and produce pro-inflammatory cytokines, ultimately leading to the development of atrial fibrosis and electrical remodeling (29,30). Additionally, the gut-heart axis has emerged as a potential mechanism linking the gut microbiota to the development of AF, whereby gut dysbiosis can result in increased levels of circulating inflammatory markers and subsequent atrial remodeling (31). In conclusion, the MHC-related pathway plays a crucial role in immune response by presenting antigens to T cells, which are a fundamental component of the immune system. Moreover, this pathway also participates in the regulation of gut bacteria. Consequently, dysregulation of immune response and gut bacteria may be implicated in the pathogenesis of AF and CD.
Overfitting is a pervasive issue in clinical biomarker discovery studies, whereby a model becomes overly intricate and fits the data too closely, resulting in poor generalizability to new data. To mitigate this issue, it is critical to appropriately select an adequate number of samples for training and testing the model (32). The area under the ROC curve (AUC) is a widely accepted metric for evaluating the performance of a biomarker or diagnostic test. It quantifies the ability of the test to discriminate between true positives and true negatives across a range of cutoff values. A higher AUC value indicates better overall test performance in terms of sensitivity and specificity (33). In this study, we employed ROC analysis to assess the diagnostic capability of CXCL16 and HLA-DPB1 in predicting CD and AF. The results indicated that CXCL16 achieved an AUC of 0.818 and 0.720 for predicting CD and AF, respectively, while HLA-DPB1 showed an AUC of 0.829 and 0.702 for predicting CD and AF, respectively. Both markers exhibited excellent diagnostic value in the test cohort of CD and AF, as corroborated by the ROC curves.
CXCL16 is a chemokine that plays a crucial role in immune cell recruitment and inflammation. This protein is expressed in various cell types, including endothelial cells, smooth muscle cells, and macrophages, and functions as a chemoattractant for immune cells expressing its cognate receptor, recombinant chemokine C-X-C-motif receptor 6 (CXCR6) (34). CXCL16 has been implicated in the pathogenesis of CD, an inflammatory bowel disease characterized by chronic inflammation and fibrosis in the intestinal mucosa (35). Numerous studies have elucidated the multifaceted role of CXCL16 in CD, highlighting its involvement in the regulation of immune responses, fibrosis promotion, and inflammation modulation in the intestinal mucosa. Notably, a recent investigation revealed that CXCL16 expression levels are elevated in both the serum and intestinal mucosa of individuals afflicted with CD, and that CXCL16 promotes the recruitment of immune cells to inflamed intestinal tissue, suggesting a potential contributory role in the chronic inflammation characteristic of this disease (36). Another study has demonstrated that CXCL16 promotes fibroblast migration, which may contribute to fibrosis in animal models of renal fibrosis (37). Fibrosis, a hallmark feature of CD, is believed to be a factor in disease progression and complications. Additionally, a study investigating the CXCL16 p. Ala181Val polymorphism found that carriers of this variant have a higher risk of developing severe CD and fistulizing disease (38). Collectively, these findings underscore the importance of CXCL16 in CD, particularly through its involvement in regulating immune response, promoting fibrosis, and regulating inflammation in the intestinal mucosa. Furthermore, recent research has demonstrated that CXCL16 is released from activated platelets in the inflammatory state of AF and may amplify platelet activation, leading to a vicious cycle of inflammation and thrombosis (39). Furthermore, CXCL16 may exacerbate AF by stimulating cardiac fibroblast proliferation, disrupting collagen synthesis, enhancing extracellular matrix remodeling, and contributing to atrial structural remodeling (40). Elevated levels of CXCL16 and other chemokines have been associated with the progression of AF by promoting the inflammatory response process, triggering arrhythmia and matrix formation, and activating the coagulation system. Additionally, the medication therapy, including ARBs, warfarin, and statins, may affect the relationship between CXCL16 and prognosis in AF patients through their impact on the concentration of CXCL16, inflammatory response, and anticoagulant status (41-43). Recent research has also identified extensive areas of atrial fibrosis in patients with lone AF through histological examination of atrial biopsies (29). Therefore, CXCL16’s ability to promote fibrosis could also contribute to the development of AF.
Our study has identified HLA-DPB1 as an important gene involved in the crosstalk between AF and CD, albeit with limited research currently available on this gene. HLA-DPB1 encodes the human leukocyte antigen DPβ1 chain, which thereby produces the MHCII molecule that is essential for presenting antigens to T cells. T cells, in turn, are an integral component of the immune response, which has been implicated in the pathogenesis of both AF and CD. By conducting KEGG enrichment analysis on these two factors, CXCL16 and HLA-DPB1, it was found that they are mainly associated with the immune system. Given the strong association of these two biomarkers with the immune system, we further investigated their expression in relation to immune infiltration.
This study found a significant difference in the immune pattern between the control group and both AF and CD groups, with CD4+ and CD8+ T cells and innate immune populations being elevated in both AF and CD samples. Previous studies suggest that AF is linked to increased infiltration of immune cells, including CD4+ and CD8+ T cells, macrophages, dendritic cells, and mast cells (10,44). These cells produce pro-inflammatory cytokines, chemokines, and growth factors that contribute to atrial remodeling, fibrosis, and electrical instability in AF. CD4+ T cells activate fibroblasts and release cytokines like interleukin (IL)-17, IL-6, and TNF-α (10) that facilitate inflammation and fibrosis, while CD8+ T cells promote fibrosis and atrial remodeling by inducing apoptosis of cardiomyocytes and producing transforming growth factor (TGF)-β. The activation and infiltration of CD8 T cells into the atrial tissue can lead to the release of pro-inflammatory cytokines and chemokines, promoting atrial remodeling and electrical disturbances. This immune response mediated by CD8 T cells may contribute to the perpetuation of AF and its progression to a more chronic and persistent state (45,46). Macrophages contribute to the pathogenesis of AF by releasing pro-inflammatory cytokines such as TNF-α, IL-1β, and IL-6, as well as phagocytosing apoptotic cardiomyocytes, which can lead to fibrosis (47). Dendritic cells promote inflammation by presenting antigens to T cells and producing cytokines like IL-6 and IL-1β (48). Mast cells release histamine, which can cause atrial fibrosis and remodeling, as well as activate fibroblasts and myofibroblasts (48). In summary, CD4+ and CD8+ T cells, along with innate immune populations, play a critical role in the promotion of inflammation, fibrosis, and electrical instability in the atria, thereby exacerbating the pathogenesis of AF.
Strengths and limitations
This study represents a groundbreaking application of bioinformatics tools to explore the genetic relationship between CD and AF. By employing LASSO regression algorithm and SVM, we identified potential shared diagnostic genes, which enhances the overall comprehensiveness and innovation of this investigation. Additionally, through validation of external datasets, we have demonstrated improved precision of predictions, further validating the significance of these results. However, it is important to note that this study has some limitations, such as the lack of consideration for regional, racial, and age-specific patient data. Therefore, future research should aim to evaluate these factors, which could significantly contribute to the development of AF.
Conclusions
Our study signifies an endeavor in the application of bioinformatics tools to thoroughly explore the genetic association between AF and CD. As a result, we have identified the CXCL16 and HLA-DPB1 genes as fundamental components in the interplay between these two pathological conditions. By highlighting the crucial role of CD4+ and CD8+ T cells and dendritic cells in the pathogenesis of AF and CD, our findings offer significant potential for immunomodulatory therapies to manage patients with both conditions. Furthermore, our findings pave the way for further research into the dynamic relationship between AF and CD, potentially identifying novel therapeutic targets for their treatment. In conclusion, this study serves as a foundational stepping stone towards a deeper understanding of the complex interplay between genetics and immune responses in the development of AF and CD, ultimately leading to improved patient outcomes.
Acknowledgments
Funding: None.
Footnote
Reporting Checklist: The authors have completed the STREGA reporting checklist. Available at https://jtd.amegroups.com/article/view/10.21037/jtd-23-1078/rc
Peer Review File: Available at https://jtd.amegroups.com/article/view/10.21037/jtd-23-1078/prf
Conflicts of Interest: All authors have completed the ICMJE uniform disclosure form (available at https://jtd.amegroups.com/article/view/10.21037/jtd-23-1078/coif). The authors have no conflicts of interest to declare.
Ethical Statement: The authors are accountable for all aspects of the work in ensuring that questions related to the accuracy or integrity of any part of the work are appropriately investigated and resolved. The study was conducted in accordance with the Declaration of Helsinki (as revised in 2013).
Open Access Statement: This is an Open Access article distributed in accordance with the Creative Commons Attribution-NonCommercial-NoDerivs 4.0 International License (CC BY-NC-ND 4.0), which permits the non-commercial replication and distribution of the article with the strict proviso that no changes or edits are made and the original work is properly cited (including links to both the formal publication through the relevant DOI and the license). See: https://creativecommons.org/licenses/by-nc-nd/4.0/.
References
- Baumgart DC, Sandborn WJ. Inflammatory bowel disease: clinical aspects and established and evolving therapies. Lancet 2007;369:1641-57. [Crossref] [PubMed]
- Franke A, McGovern DP, Barrett JC, et al. Genome-wide meta-analysis increases to 71 the number of confirmed Crohn's disease susceptibility loci. Nat Genet 2010;42:1118-25. [Crossref] [PubMed]
- Marchesi JR, Adams DH, Fava F, et al. The gut microbiota and host health: a new clinical frontier. Gut 2016;65:330-9. [Crossref] [PubMed]
- Molodecky NA, Soon IS, Rabi DM, et al. Increasing incidence and prevalence of the inflammatory bowel diseases with time, based on systematic review. Gastroenterology 2012;142:46-54.e42; quiz e30. [Crossref] [PubMed]
- Ng SC, Shi HY, Hamidi N, et al. Worldwide incidence and prevalence of inflammatory bowel disease in the 21st century: a systematic review of population-based studies. Lancet 2017;390:2769-78. [Crossref] [PubMed]
- Bernstein CN, Blanchard JF, Kliewer E, et al. Cancer risk in patients with inflammatory bowel disease: a population-based study. Cancer 2001;91:854-62. [Crossref] [PubMed]
- Chugh SS, Havmoeller R, Narayanan K, et al. Worldwide epidemiology of atrial fibrillation: a Global Burden of Disease 2010 Study. Circulation 2014;129:837-47. [Crossref] [PubMed]
- Nattel S, Dobrev D. Controversies About Atrial Fibrillation Mechanisms: Aiming for Order in Chaos and Whether it Matters. Circ Res 2017;120:1396-8. [Crossref] [PubMed]
- Voigt N, Heijman J, Wang Q, et al. Cellular and molecular mechanisms of atrial arrhythmogenesis in patients with paroxysmal atrial fibrillation. Circulation 2014;129:145-56. [Crossref] [PubMed]
- Hu YF, Chen YJ, Lin YJ, et al. Inflammation and the pathogenesis of atrial fibrillation. Nat Rev Cardiol 2015;12:230-43. [Crossref] [PubMed]
- Korantzopoulos P, Letsas KP, Tse G, et al. Inflammation and atrial fibrillation: A comprehensive review. J Arrhythm 2018;34:394-401. [Crossref] [PubMed]
- Choi YJ, Choi EK, Han KD, et al. Increased risk of atrial fibrillation in patients with inflammatory bowel disease: A nationwide population-based study. World J Gastroenterol 2019;25:2788-98. [Crossref] [PubMed]
- Pattanshetty DJ, Anna K, Gajulapalli RD, et al. Inflammatory bowel "Cardiac" disease: Point prevalence of atrial fibrillation in inflammatory bowel disease population. Saudi J Gastroenterol 2015;21:325-9. [Crossref] [PubMed]
- Takei Y, Ichikawa M, Kijima Y. Oral direct renin inhibitor aliskiren reduces in vivo oxidative stress and serum matrix metalloproteinase-2 levels in patients with permanent atrial fibrillation. J Arrhythm 2015;31:76-7. [Crossref] [PubMed]
- Negi S, Sovari AA, Dudley SC Jr. Atrial fibrillation: the emerging role of inflammation and oxidative stress. Cardiovasc Hematol Disord Drug Targets 2010;10:262-8. [Crossref] [PubMed]
- Larsson SC, Lee WH, Burgess S, et al. Plasma Cortisol and Risk of Atrial Fibrillation: A Mendelian Randomization Study. J Clin Endocrinol Metab 2021;106:e2521-6. [Crossref] [PubMed]
- Holubar SD, Holder-Murray J, Flasar M, et al. Anti-Tumor Necrosis Factor-α Antibody Therapy Management Before and After Intestinal Surgery for Inflammatory Bowel Disease: A CCFA Position Paper. Inflamm Bowel Dis 2015;21:2658-72. [Crossref] [PubMed]
- Langfelder P, Horvath S. WGCNA: an R package for weighted correlation network analysis. BMC Bioinformatics 2008;9:559. [Crossref] [PubMed]
- Hänzelmann S, Castelo R, Guinney J. GSVA: gene set variation analysis for microarray and RNA-seq data. BMC Bioinformatics 2013;14:7. [Crossref] [PubMed]
- Tumer G, Simpson B, Roberts TK. Genetics, Human Major Histocompatibility Complex (MHC). In: StatPearls. Treasure Island: StatPearls Publishing; 2023.
- Goyette P, Boucher G, Mallon D, et al. High-density mapping of the MHC identifies a shared role for HLA-DRB1*01:03 in inflammatory bowel diseases and heterozygous advantage in ulcerative colitis. Nat Genet 2015;47:172-9. [Crossref] [PubMed]
- Newman B, Silverberg MS, Gu X, et al. CARD15 and HLA DRB1 alleles influence susceptibility and disease localization in Crohn's disease. Am J Gastroenterol 2004;99:306-15. [Crossref] [PubMed]
- Muro M, López-Hernández R, Mrowiec A. Immunogenetic biomarkers in inflammatory bowel diseases: role of the IBD3 region. World J Gastroenterol 2014;20:15037-48. [Crossref] [PubMed]
- Bradley JR. TNF-mediated inflammatory disease. J Pathol 2008;214:149-60. [Crossref] [PubMed]
- Matsukura H, Ikeda S, Yoshimura N, et al. Genetic polymorphisms of tumour necrosis factor receptor superfamily 1A and 1B affect responses to infliximab in Japanese patients with Crohn’s disease. Aliment Pharmacol Ther 2008;27:765-70. [Crossref] [PubMed]
- Ferguson LR, Huebner C, Petermann I, et al. Single nucleotide polymorphism in the tumor necrosis factor-alpha gene affects inflammatory bowel diseases risk. World J Gastroenterol 2008;14:4652-61. [Crossref] [PubMed]
- López-Hernández R, Valdés M, Lucas D, et al. Association analysis of MICA gene polymorphism and MICA-129 dimorphism with inflammatory bowel disease susceptibility in a Spanish population. Hum Immunol 2010;71:512-4. [Crossref] [PubMed]
- Glas J, Martin K, Brünnler G, et al. MICA, MICB and C1_4_1 polymorphism in Crohn’s disease and ulcerative colitis. Tissue Antigens 2001;58:243-9. [Crossref] [PubMed]
- Frustaci A, Chimenti C, Bellocci F, et al. Histological substrate of atrial biopsies in patients with lone atrial fibrillation. Circulation 1997;96:1180-4. [Crossref] [PubMed]
- Issac TT, Dokainish H, Lakkis NM. Role of inflammation in initiation and perpetuation of atrial fibrillation: a systematic review of the published data. J Am Coll Cardiol 2007;50:2021-8. [Crossref] [PubMed]
- Rashid S, Noor TA, Saeed H, et al. Association of gut microbiome dysbiosis with the progression of atrial fibrillation: A systematic review. Ann Noninvasive Electrocardiol 2023;28:e13059. [Crossref] [PubMed]
- Hernández B, Parnell A, Pennington SR. Why have so few proteomic biomarkers "survived" validation? (Sample size and independent validation considerations). Proteomics 2014;14:1587-92. [Crossref] [PubMed]
- Nahm FS. Receiver operating characteristic curve: overview and practical use for clinicians. Korean J Anesthesiol 2022;75:25-36. [Crossref] [PubMed]
- Ma Z, Jin X, He L, et al. CXCL16 regulates renal injury and fibrosis in experimental renal artery stenosis. Am J Physiol Heart Circ Physiol 2016;311:H815-21. [Crossref] [PubMed]
- Gordon IO, Bettenworth D, Bokemeyer A, et al. International consensus to standardise histopathological scoring for small bowel strictures in Crohn's disease. Gut 2022;71:479-86. [Crossref] [PubMed]
- Lehrke M, Konrad A, Schachinger V, et al. CXCL16 is a surrogate marker of inflammatory bowel disease. Scand J Gastroenterol 2008;43:283-8. [Crossref] [PubMed]
- Izquierdo MC, Martin-Cleary C, Fernandez-Fernandez B, et al. CXCL16 in kidney and cardiovascular injury. Cytokine Growth Factor Rev 2014;25:317-25. [Crossref] [PubMed]
- Pu Q, Lin P, Gao P, et al. Gut Microbiota Regulate Gut-Lung Axis Inflammatory Responses by Mediating ILC2 Compartmental Migration. J Immunol 2021;207:257-67. [Crossref] [PubMed]
- Borst O, Münzer P, Gatidis S, et al. The inflammatory chemokine CXC motif ligand 16 triggers platelet activation and adhesion via CXC motif receptor 6-dependent phosphatidylinositide 3-kinase/Akt signaling. Circ Res 2012;111:1297-307. [Crossref] [PubMed]
- Dahl CP, Husberg C, Gullestad L, et al. Increased production of CXCL16 in experimental and clinical heart failure: a possible role in extracellular matrix remodeling. Circ Heart Fail 2009;2:624-32. [Crossref] [PubMed]
- Clancy P, Koblar SA, Golledge J. Angiotensin receptor 1 blockade reduces secretion of inflammation associated cytokines from cultured human carotid atheroma and vascular cells in association with reduced extracellular signal regulated kinase expression and activation. Atherosclerosis 2014;236:108-15. [Crossref] [PubMed]
- Kumagai N, Nusser JA, Inoue H, et al. Effect of Addition of a Statin to Warfarin on Thromboembolic Events in Japanese Patients With Nonvalvular Atrial Fibrillation and Diabetes Mellitus. Am J Cardiol 2017;120:230-5. [Crossref] [PubMed]
- Smith C, Halvorsen B, Otterdal K, et al. High levels and inflammatory effects of soluble CXC ligand 16 (CXCL16) in coronary artery disease: down-regulatory effects of statins. Cardiovasc Res 2008;79:195-203. [Crossref] [PubMed]
- Liu Y, Shi Q, Ma Y, et al. The role of immune cells in atrial fibrillation. J Mol Cell Cardiol 2018;123:198-208. [Crossref] [PubMed]
- Friebel J, Witkowski M, Wegner M, et al. Cytotoxic CD8(+) T Cells Are Involved in the Thrombo-Inflammatory Response during First-Diagnosed Atrial Fibrillation. Cells 2022;12:141. [Crossref] [PubMed]
- Chang G, Chen Y, Liu Z, et al. The PD-1 with PD-L1 Axis Is Pertinent with the Immune Modulation of Atrial Fibrillation by Regulating T Cell Excitation and Promoting the Secretion of Inflammatory Factors. J Immunol Res 2022;2022:3647817. [Crossref] [PubMed]
- Yamashita T, Sekiguchi A, Iwasaki YK, et al. Recruitment of immune cells across atrial endocardium in human atrial fibrillation. Circ J 2010;74:262-70. [Crossref] [PubMed]
- Liu L, Lee J, Fu G, et al. Activation of peripheral blood CD3(+) T-lymphocytes in patients with atrial fibrillation. Int Heart J 2012;53:221-4. [Crossref] [PubMed]