Imaging features and deep learning for prediction of pulmonary epithelioid hemangioendothelioma in CT images
Highlight box
Key findings
• Our study confirmed the heterogeneity of the imaging findings of pulmonary epithelioid hemangioendothelioma (PEH) and revealed some previously undescribed types and features. The deep learning model based on computed tomography (CT) to distinguish PEH has clinical application potential.
What is known and what is new?
• The imaging findings of PEH are varied and lack typical clinical findings.
• This study confirmed heterogeneity in the imaging findings of PEH and showed some previously undescribed types and features. This study is the first attempt to use deep learning to predict PEH, which indicates its potential value for clinical application and needs further optimization.
What is the implication, and what should change now?
• The deep learning model based on CT to distinguish PEH needs further exploration in the future.
Introduction
Epithelioid hemangioendothelioma (EHE) is a rare vasogenic tumour with an estimated incidence of less than 1 per million people (1). EHE is characterized by the presence of epithelioid or histiocytoid endothelial cells and mainly occurs in the liver, lung and bone (2,3). Pulmonary epithelioid hemangioendothelioma (PEH) was originally named intravascular bronchiolar alveolar tumour (IVBAT), representing 19% of all EHE cases (4). The clinical and imaging features associated with PEH have been reported in a small number of previous studies with small sample sizes (5). The most common manifestation of PEH is bilateral multiple nodules (6), which may appear in many lung diseases and may be easily misdiagnosed as metastatic cancer (7), but the imaging presentation of PEH is diverse and remains unclear (8-10). In the meantime, the clinical diagnosis and management of PEH are difficult due to the lack of typical clinical presentation. The diagnosis of PEH is based on the histopathological diagnosis after a lung biopsy and a positive immunohistochemical staining for vascular endothelial markers such as CD31 (7). Histopathological examination can not only be used to diagnose PEH, but also help to determine its adverse effects (6). Nevertheless, lung biopsy may increase the risk of tumor-related bleeding in patients with PEH, especially for peripheral lesions (7). These may contribute to delayed diagnosis. Thus, there is an urgent need to gain insight into the imaging features and imaging classification of PEH, as well as to try to establish a new complementary diagnostic tool that can accurately identify PEH from imaging data to enable early cancer diagnosis.
Deep learning, a representative technique of artificial intelligence (AI), has shown great promise for detecting some common diseases based on clinical images, especially in medical image-related disease diagnosis and outcome prediction (11). Deep learning techniques have been utilized in image recognition of thoracic tumors (12). Deep learning models based on computed tomography (CT) to identify lung cancer have been demonstrated with certain advantages (13) and high accuracy at 80–90% (14,15). Therefore, AI-assisted systems may provide support for clinical decision-making on images of suspected PEH, improving diagnostic rates.
The objective of this study is to describe the imaging features of PEH in depth via a retrospective cohort and published cases. On this basis, we further explore the imaging findings that have not clearly been defined and analyze the correlation between imaging features and prognosis. Meanwhile, we attempted to explore the potential value of deep learning models based on CT findings for identifying PEH. We present this article in accordance with the STROBE reporting checklist (available at https://jtd.amegroups.com/article/view/10.21037/jtd-23-455/rc).
Methods
Patients
We retrospectively evaluated patients who with were diagnosed PEH and underwent CT at Guangzhou Institute of Respiratory Health from September 2011 to December 2021. The inclusion criteria were as follows: (I) each patient was examined by histopathology and diagnosed according to the EHE consensus (16); (II) the site of primary lesions was limited to lung, with or without extrathoracic metastases; (III) patients with complete clinical and imaging data [CT and/or positron emission tomography (PET)/CT]. Moreover, the exclusion criteria were as follows: (I) subjects with a history of other thoracic tumors (lung cancer, pleural mesothelioma, etc.); (II) poor-quality or postoperative chest CT images to observe and assess PEH lesions. In addition, several pulmonary diseases with similar imaging findings to PEH were also included for differentiation, such as central lung cancer, diffuse alveolar hemorrhage, multiple ground-glass nodules, lung metastases, viral and hypersensitivity pneumonitis, and disseminated tuberculosis. Those diseases were diagnosed according to current clinical practice guidelines (17-19) and were defined as the control groups (Figure S1). The study was conducted in accordance with the Declaration of Helsinki (as revised in 2013). The study was approved by the Ethics Board of The First Affiliated Hospital of Guangzhou Medical University (No. ES-2023-028-01) and individual consent for this retrospective analysis was waived.
CT image acquisition
All the patients underwent unenhanced chest CT with a multidetector CT scanner (TOSHIBA Aquilion, Tokyo, Japan; SIEMENS Definition AS+, Munich, Germany; GE Revolution 256, GE HealthCare, Chicago, IL, USA; NMS NEuViz 128, Shenyang, China; GE Discovery ST). The CT protocol included the following parameters: tube voltage of 120 kVp, the field of view (FOV) of 320 mm by a matrix of 512×512 and the tube-current with automated modulation. Lung images were reconstructed with a slice thickness of 1.0 mm and were observed at both the lung window (level, −600 HU; width, 1,500 HU) and mediastinal window (level, 40 HU; width, 350 HU).
PET/CT imaging was performed using a GE Discovery ST-8 PET/CT scanner. 18F-FDG was produced and synthesized by the GE Mini Trace cyclotron via an automatic synthesis module, with a radiochemical purity of 95% or greater. Patients were fasted for a minimum of 6 hours before being injected 18F-FDG, at a dose of 3.70–5.55 MBq/kg. The whole body PET scans were acquired in 2-D mode and ranged from the head down to the root of the thigh. The obtained PET data were reconstructed using an ordered subset expectation maximization algorithm (6-bed positions; 3.5 minutes/bed; 128×128 matrix). All images were exported in DICOM format for feature extraction and further analysis.
Imaging features extraction and algorithm training
Two experienced radiologists independently analyzed the chest CT images, and discrepancies were resolved by consensus. The following imaging features of the PEH were evaluated: lesions size (largest diameter), distribution (single or multiple; uni- or bilaterality), presence of necrosis and calcification, pleural effusion and regional lymph nodes involvement (hilar and mediastinal). Furthermore, the imaging findings in patients with PEH were classified according to the previous expert consensus (16).
Deep learning algorithms were also attempted to identify PEH based on CT images, and distinguish PEH from other pulmonary diseases as described above. Moreover, the images of PEH and other pulmonary diseases were classified using an 18-layer deep residual neural network (ResNet 18), including four residual modules and a fully connected layer. The loss function for the model training was the cross entropy as previously described (20). Prior to training the network, the data were displayed in lung window images with a maximum value of 0 and a minimum of −1,024. Finally, the intensity value of the images was normalized between 0 and 255 (Figure 1). Dropout and L2 regularization were adopted to achieve model optimization and avoid overfitting. The ResNet is implemented with PyTorch, and the model is optimized with Stochastic Gradient Descent (SGD) algorithm. The batch size of the training data is 5. The learning rate is 1e−4. Model discrimination was assessed using machine learning evaluation metrics, including accuracy, precision, recall, and F1 score.
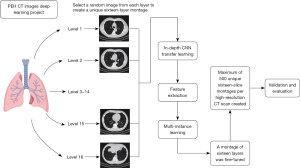
Systematic review of published cases
A systematic literature search was conducted in PubMed between 2010 and 2022, using the following keywords: “pulmonary epithelioid hemangioendothelioma” and “intravascular bronchioloalveolar tumor” or both alone to identify articles. This search was restricted to human studies in the English language. Eligible studies were included for a pooled analysis if they met the following inclusion criteria: (I) studies that included patients with a pathologically confirmed diagnosis of PEH; (II) studies that reported relevant clinical and/or radiological data of patients with PEH. Specifically, the following data from individual case reports or series were extracted and analyzed: demographic and clinical features (age, sex, symptom, etc.), imaging characteristics, pathological findings and prognosis (Figure S2). For cases with CT images, their imaging features were summarized based on the evaluation by our radiologists and the results reported in the literature. If not, summary analysis is mainly conducted according to the description of imaging features provided in the literature.
Statistical analysis
The quantitative data were presented as mean ± standard deviation (SD) or median (interquartile range, 25–75%) according to distribution. The clinical characteristics, imaging features and laboratory parameters were compared using Mann-Whitney or t-test for continuous variables and Continuous variables for categorical variables. The overall diagnostic accuracy was estimated by calculating the area under the receiver operating characteristic curve (AUC) with 95% confidence interval (CI). Overall survival of patients with different imaging findings was compared via the Kaplan-Meier analysis with the Log-rank test. Differences with a P≤0.05 were considered statistically significant. All statistical analyses were performed in R (version 4.2.0).
Results
Clinical characteristics of patients with PEH
Twenty-five patients with PEH ultimately met our inclusion criteria. Fourteen patients (56%) underwent surgical lung biopsy, 7 (28%) underwent percutaneous lung biopsy and 4 (16%) underwent transbronchial lung biopsy. The mean age of these patients was 41.9±14.6 years, 15 of them were male and the mean duration was 4.8±7.6 years. Of the 25 patients, 23 (92%) had one or more of the following symptoms: 15 patients (60%) presented with cough, 13 (52%) with expectoration and 11 (44%) with dyspnea. A few patients also presented with hemoptysis (4, 16%) and weight loss (6, 24%). In terms of tumor markers, the correlation analysis showed mediastinal and hilar lymph node enlargement was associated with elevated carcinoembryonic antigen (P<0.05). The detailed characteristics of these patients are shown in Table 1.
Table 1
Characteristics | N/(mean ± SD) |
---|---|
Number of patients | 25 |
Age (year) | 41.9±14.6 |
Course of disease (year) | 4.8±7.6 |
Sex | |
Male | 15 |
Female | 10 |
Smoking history | |
Smoker | 6 |
Non-smoker | 14 |
Ex-smoker | 3 |
Unknown | 2 |
Main symptom | |
Cough | 15 |
Expectoration | 13 |
Dyspnea | 11 |
Weight loss | 6 |
Hemoptysis | 4 |
Laboratory examination of tumor marker | |
Neuron specific enolase (ng/mL) | 37.9±27.2 |
Carcinoembryonic antigen (ng/mL) | 1.2±0.6 |
CA125 (U/mL) | 28.1±23.0 |
CA153 (U/mL) | 11.0±6.0 |
Neuron-specific enolase | 2.1±0.8 |
Immunological detection (CD31/CD34) | 25 |
Both positive | 18 |
Single CD31 positive | 6 |
Single CD34 positive | 1 |
PEH, pulmonary epithelioid hemangioendothelioma; SD, standard deviation.
Imaging findings on CT
The imaging findings based on different classification criteria were summarized in Table 2. Radiologically, a majority of patients had multiple lesions (n=24), the distribution of which appeared bilateral (n=19), unilateral (n=5) or unilateral single (n=1). According to the location of lesions, 7 cases were classified as central type and 16 cases presented with peripheral type. In terms of its composition, the lesions were divided into consolidations (n=21) and ground-glass opacities (n=4). Three types identified by the size of the lesions included nodules (≤3 cm, n=8), masses (>3 cm, n=2) and mixed types with both nodules and masses (n=11). Most of the largest lesions (n=17) in the 25 patients had clear boundaries, and the mean diameter of the largest lesions was 18.36±23.39 mm. Moreover, imaging signs of PEH were also measured and shown in Table 2.
Table 2
Characteristics | N/(mean ± SD)/median (IQR) |
---|---|
Classification based on distribution | |
Single nodule in one lobe | 1 |
Multiple nodules in one lobe | 5 |
Multiple nodules in the whole lung | 19 |
Classification of the site of tumor | |
Central | 7 |
Peripheral | 16 |
Unknown | 2 |
Classification based on lesions components | |
Consolidations | 21 |
Mass type | 2 |
Nodular type | 8 |
Mixed | 11 |
Ground glass opacities | 4 |
The largest lesion | |
Clear boundaries | 17 |
Unclear boundaries | 8 |
Mean diameter (mm) | 18.36±23.39 |
Classification based on consensus | |
Multiple pulmonary nodules | 5 |
Reticular nodular shadow | 2 |
Diffuse pleural thickening | 4 |
Nodules or masses invade the pleura | 4 |
Mixed type | 4 |
Other | 6 |
Imaging signs | |
Calcification | 5 |
Pleural thickening | 8 |
Pleural effusion | 15 |
Mediastinal lymph node enlargement | 8 |
Hilar lymph nodes enlargement | 10 |
PET/CT examination | |
Negative | 2 |
Positive | 8 |
SUVmax | 10.1 (6.4–11.2) |
Mixed, mass and nodular type. The other types of classification included solitary pulmonary nodes and endobronchial lesions. PEH, pulmonary epithelioid hemangioendothelioma; SD, standard deviation; IQR, interquartile range; PET, positron emission tomography/computed tomography; SUVmax, maximum standardized uptake value.
In accordance with the imaging classification of the consensus, four imaging patterns of PEH were found in our center (Figure 2), including diffuse pleural thickening (n=4), reticular nodular shadow (n=2), multiple pulmonary nodules (n=5) and mass invasion of the pleura (n=4). Mixed types containing two or more of those types were also observed in the other four patients. In addition to the above, solitary pulmonary nodules and endobronchial nodule were presented in 3 and 1 cases, respectively. Nevertheless, two patients with undefined imaging findings were unable to adequately observe the distribution of their lesions due to atelectasis.
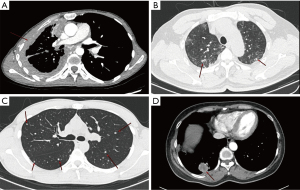
Imaging findings on PET/CT
Ten patients underwent F18-FDG PET/CT examinations, and the standardized uptake value (SUV) of tumor was measured. A positive lesion was defined as a lesion with a radiological uptake above the mediastinal blood pool. Positive lesions were found in 8 of the 10 patients in our study. The median SUVmax value was 10.1 (interquartile range, 6.4–11.2). In addition, extrapulmonary involvement was observed in 1 case presenting with metastases to the sternum, clavicle and spine.
Deep learning-based analysis of CT images
We proceeded to build a deep learning model to distinguish PEH from pulmonary diseases with similar CT presentation (control group). The demographic characteristics of the control group are shown in Table S1. After screening and eligibility assessment, 61 CT images of 25 PEH patients and 243 CT images of control groups were included in the model (Figure S1). The data set was divided into training and testing sets. For the differentiation of PEH and controls, the algorithm converged to a stable result within 50–200 iterations. The differentiation accuracies of training and testing sets were 100% and 74%, respectively (Figure 3A,3B). The deep learning model achieved an AUC value of 0.71 (95% CI: 0.69–0.72) (Figure 3C). However, the training loss and accuracy reached convergence at an early stage (less than 50 iterations) due to the limited sample size. Meanwhile, the significant deviation in performance between the training group and testing group indicated that the model was overfitting. In subgroup analysis, several radiographic subtypes of PEH were further distinguished from the other diseases with the most similar imaging findings. Nevertheless, valid and robust models were not successfully established since the sample size was small (Figure S3). The models discrimination performance including accuracy, precision, recall, F1 score were also been evaluated. The accuracy is 73.4%, the precision is 18.7%, the recall is 18.4%, and the F1 score is 18.5%, respectively.
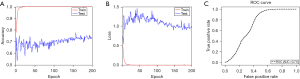
Review and pooled analysis of published cases
A total of 54 case reports or series encompassing 71 patients with PEH met all the criteria and were included in pooled analysis (Table S2). Baseline clinical characteristics are shown in Table S3. Most patients had multiple solid lesions of both lungs (n=49), and only a few patients showed ground glass opacities (n=8). In patients with solid lesions, the majority was the nodular (n=36), followed by the mixed type (n=16) and the mass type (n=10). Concerning lesion characteristics, most of the lesions showed an irregular morphology, unclear boundary and heterogeneous density. In terms of imaging signs, 13 patients showed lesion calcification, and pleural effusion was observed in 14 patients, hilar and mediastinal lymph node involvement was present in 8 patients, respectively (Table 3).
Table 3
Characteristics | N |
---|---|
Number of patients | 71 |
Distribution | |
Single nodule in one lobe | 12 |
Multiple nodules in one lobe | 10 |
Multiple nodules in the whole lung | 49 |
Classification based on lesions components | |
Consolidations* | |
Mass type | 10 |
Nodular type | 36 |
Mixed | 16 |
Ground glass opacities | 8 |
Imaging signs | |
Calcification | 13 |
Pleural effusion | 14 |
Mediastinal lymph node enlargement | 8 |
Hilar lymph nodes enlargement | 8 |
*, the imaging features of 1 patient were not available. Mixed type contained both mass and nodular types. PEH, pulmonary epithelioid hemangioendothelioma.
Moreover, we further explored the differences in survival outcomes between different imaging findings. During the mean follow-up period of 28.4±35.2 months, 21 patients died and the causes of death were summarized in Table S3. The Kaplan-Meier curves with Cox regression analysis revealed that pleural effusion was significantly associated with poor overall survival in patients with PEH (P<0.001, hazard ratio: 5.13, 95% CI: 2.2–13.14) (Figure 4). On the contrary, none of the other imaging features were prognostic factors for survival.
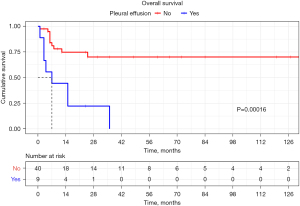
Discussion
The present study confirmed the significant heterogeneity in the imaging findings of PEH through a retrospective analysis of 25 patients and a pooled analysis of 71 published cases. In addition to the four typical patterns, the mixture of patterns and types of solitary pulmonary nodules were also observed in our center. The pooled analysis suggested that some features (e.g., pleural effusion) may have potential prognostic value. Furthermore, this study was the first to apply the deep learning method to the identification of PEH and differentiation from other diseases with similar imaging manifestations. The current model shows potential for clinical application, though the issue of overfitting needs further improvement. This study may provide preliminary evidence for imaging assessment and development of AI model for PEH.
In general, PEH occurs mainly in the young and middle-aged populations and presents with asymptomatic or non-specific respiratory symptoms (i.e., cough, dyspnoea), as seen in our results (21,22). However, the proportion of male patients in this study was 60% (15/25), in contrast to the findings of previous reports in which PEH is four times more common in women (23). Radiologically, our results showed that PEH mainly presented as multiple bilateral nodules/masses, and the largest nodules were less than 2 cm in diameter in most patients. These findings are consistent with previous reports that the most common CT presentation of PEH is multiple perivascular lesions in both lungs (7,24). Meanwhile, most of the lesions showed a solid component and clear borders with regular morphology, which is in line with previous studies (25,26). A small number of lesions, however, are characterized by ground-glass opacities and irregular margins, which may be attributed to tumour infiltration of bronchioles and small vessels (26,27).
In our study, punctate calcification was observed in nodules in 20% (5/25) of cases, a feature also reported by other studies (23,28). Interestingly, two studies reported an increase in calcification during follow-up, suggesting that this feature appears to be related to the long-term evolution of the tumour (28). Moreover, enlargement of the hilar or mediastinal lymph nodes was found in 10 cases, which has rarely been described in previous case studies. It has been suggested that enlargement of adjacent lymph nodes may indicate the presence of metastatic spread and was considered to be a poor prognostic factor (2). Overall, the imaging features of PEH demonstrated in this study were generally consistent with previous reports, while some features that are not widely recognized have also been identified. These results increased the awareness of imaging findings of PEH and the clinical significance and revolution of the findings need to be further explored.
In our study, all four types of imaging findings based on consensus were observed in a majority of patients, presenting with one or more of these types (16). Moreover, solitary pulmonary nodules and endobronchial lesions were found in 4 cases and 1 case, respectively, which are rarely reported. Unfortunately, the pattern of bilateral multifocal areas of reticulonodular was initially misdiagnosed as diffuse alveolar hemorrhage in 2 cases of our study, as reported in previous studies (29,30). Similarly, the pattern of bilateral multiple pulmonary nodules was misdiagnosed with lung metastases or lung cancer, which is consistent with previous studies (31-33). In addition, previous studies have suggested that both diffuse pleural thickening and pleural infiltration types could easily be mistaken for malignant mesothlioma (24,34). This evidence demonstrated that misdiagnosis of PEH remains common. Overall, the current imaging patterns of PEH are nonspecific, and more subtypes may be classified in the future. Meanwhile, several pulmonary diseases (i.e., pneumonia, metastases and tuberculosis) were also provided as potential differential diagnoses and were further incorporated into the deep-learning model for classification. Hence, both adequate consideration of existing types and comprehensive comparison with similar diseases are both necessary for assessing CT images of suspected PEH.
Currently, the prognosis of PEH remains unclear due to the lack of definitive predictors and available staging systems. Our pooled analysis found that pleural effusion was a significant prognostic factor for poor survival in patients with PEH. In our center, of the nine patients who presented with diffuse pleural thickening or pleural infiltration, eight had pleural effusion. There was considerable evidence confirmed that patients with pleural thickening and effusion have a poor prognosis, which was similar to our results (34,35). Similarly, several studies showed that fibrinous pleuritis and extrapleural proliferation of tumor cells and pleural invasion were indicators of poor outcomes (6,36,37). These indicated that pleural involvement might correlate with more aggressive behaviour of tumor, suggesting a later stage of disease progression (38). Hence, the dynamic assessment on severity of pleural involvement might be helpful in predicting the prognosis and disease progression of patients with PEH. In addition, the pattern of multiple pulmonary nodules was found to be associated with favorable survival (21). Furthermore, it has been revealed that an increased uptake value of FDG PET/CT was an effective marker of malignant PEH lesions which worsen the prognosis (39). But more research with larger samples is still needed to verify these findings. Overall, continuous imaging monitoring could be considered for clinical decision-making and personalized management for patients with PEH. The prognostic value of more imaging features may be worthy of further studies.
Our study also attempted to use CT-based AI algorithms for the identification and discrimination of PEH, referring to previous applications of deep learning in the field of thoracic oncology (40,41). Despite the occurrence of overfitting, the current model showed potential for clinical application. Specifically, there was a tendency for the accuracy and loss to improve in the later stage of test sets, suggesting that the model was still possible to obtain useful information from these data. These preliminary results suggested that the development and validation of an AI model for image recognition of PEH remains a viable and promising proposal.
Previous studies have successfully constructed deep learning models for rare diseases, such as idiopathic pulmonary fibrosis, pleural mesothelioma, and interstitial lung disease (42-44). These studies demonstrated robust and efficient performance of models with AUC ranging from 0.75–0.92 (41-44). It is well known that commonly used metrics for evaluating machine learning algorithms are accuracy, precision, and recall (45). In large-sample machine learning in general recall and precision show a significant negative correlation (46). However, the challenges brought by the limited sample size of rare diseases for neural network training could not be ignored. In our study, probably due to the insufficient sample size, our metrics such as precision, recall, and F1 score are lower compared to other studies (47,48). Notably, the significant heterogeneity in the radiological presentation of PEH may increase the difficulty of the training and the risk of overfitting. Overall, the most fundamental approach to improving the problem of overfitting is still to expand the sample capacity. Thus, it is worthy to conduct clinical registries for the continuous collection of cases with PEH, in preparation for large-scale studies in the future (49).
In recent years, groundbreaking advances in model training methods for small sample size images have been developed. The following methods might be attempted to address the limitations of the data volume for adequate learning of image features in PEH. Firstly, the transfer learning strategy makes it possible to implement deep learning on relatively small datasets (50). Specifically, transfer learning allows the model to transfer some of the knowledge learned on large datasets (e.g., normal CT images) to similar tasks, making it easier to learn the characteristics of the target disease (51). Secondly, data augmentation can also be used to extend the training dataset by resizing, rotating and reflecting the images of the dataset (52). Furthermore, it is promising that transformer methods with their adaptive feature have been increasingly accepted, specifically for diseases with heterogeneous imaging finding (49,52). Despite their advantages, one of the biggest obstacles to the development of highly accurate PEH recognition and classification algorithms is the lack of large imaging datasets for algorithm training. Therefore, if imaging biomarkers are to be successfully developed using these technologies, regional as well as international collaboration is needed to develop a centralised imaging repository for PEH.
This study has several limitations. First, this study was retrospectively performed in a single institution, which may result in unavoidable selection bias. Second, the longitudinal observation of long-term changes in imaging features of PEH was not performed due to the lack of follow-up CT images. Another limitation of the present study is the relatively small sample size since PEH is a rare disease, especially for building a machine-learning model. Despite these limitations, to our knowledge, this study is the first to explore the possibility of using CT-based deep learning to identify PEH.
Conclusions
Overall, the imaging of PEH is heterogeneous. Multiple pulmonary nodules are predominant, and the presence of a mixture of types is equally noteworthy. Some features may be associated with a poor prognosis, such as pleural effusion. This study is the first attempt to use deep learning in predicting PEH and indicates its potential value for clinical application, which needs to be further optimised in the future. Increased understanding of the imaging presentation of PEH and the development of AI-assisted systems may be of value in improving the accuracy of early diagnosis of PEH.
Acknowledgments
Funding: This work was financially supported by
Footnote
Reporting Checklist: The authors have completed the STROBE reporting checklist. Available at https://jtd.amegroups.com/article/view/10.21037/jtd-23-455/rc
Data Sharing Statement: Available at https://jtd.amegroups.com/article/view/10.21037/jtd-23-455/dss
Peer Review File: Available at https://jtd.amegroups.com/article/view/10.21037/jtd-23-455/prf
Conflicts of Interest: All authors have completed the ICMJE uniform disclosure form (available at https://jtd.amegroups.com/article/view/10.21037/jtd-23-455/coif). The authors have no conflicts of interest to declare.
Ethical Statement: The authors are accountable for all aspects of the work in ensuring that questions related to the accuracy or integrity of any part of the work are appropriately investigated and resolved. The study was conducted in accordance with the Declaration of Helsinki (as revised in 2013). The study was approved by the Ethics Board of The First Affiliated Hospital of Guangzhou Medical University (No. ES-2023-028-01) and individual consent for this retrospective analysis was waived.
Open Access Statement: This is an Open Access article distributed in accordance with the Creative Commons Attribution-NonCommercial-NoDerivs 4.0 International License (CC BY-NC-ND 4.0), which permits the non-commercial replication and distribution of the article with the strict proviso that no changes or edits are made and the original work is properly cited (including links to both the formal publication through the relevant DOI and the license). See: https://creativecommons.org/licenses/by-nc-nd/4.0/.
References
- Lavacchi D, Voltolini L, Comin CE, et al. Primary pleural epithelioid hemangioendothelioma: case report and review of the literature. Anticancer Drugs 2021;32:1131-7. [Crossref] [PubMed]
- Lau K, Massad M, Pollak C, et al. Clinical patterns and outcome in epithelioid hemangioendothelioma with or without pulmonary involvement: insights from an internet registry in the study of a rare cancer. Chest 2011;140:1312-8. [Crossref] [PubMed]
- Guo Q, Xue J, Xu L, et al. The clinical features of epithelioid hemangioendothelioma in a Han Chinese population: A retrospective analysis. Medicine (Baltimore) 2017;96:e7345. [Crossref] [PubMed]
- Ye B, Li W, Feng J, et al. Treatment of pulmonary epithelioid hemangioendothelioma with combination chemotherapy: Report of three cases and review of the literature. Oncol Lett 2013;5:1491-6. [Crossref] [PubMed]
- Mao X, Liang Z, Chibhabha F, et al. Clinico-radiological features and next generation sequencing of pulmonary epithelioid hemangioendothelioma: A case report and review of literature. Thorac Cancer 2017;8:687-92. [Crossref] [PubMed]
- Kitaichi M, Nagai S, Nishimura K, et al. Pulmonary epithelioid haemangioendothelioma in 21 patients, including three with partial spontaneous regression. Eur Respir J 1998;12:89-96. [Crossref] [PubMed]
- Mesquita RD, Sousa M, Trinidad C, et al. New Insights about Pulmonary Epithelioid Hemangioendothelioma: Review of the Literature and Two Case Reports. Case Rep Radiol 2017;2017:5972940. [Crossref] [PubMed]
- Oda N, Maeda Y, Kiura K, et al. Pulmonary epithelioid haemangioendothelioma mimicking lung cancer. BMJ Case Rep 2021;14:e240152. [Crossref] [PubMed]
- Aung TT, Chu A, Kondapi D, et al. A Case of Pulmonary Epithelioid Hemangioendothelioma with Literature Review. Case Rep Oncol Med 2020;2020:8048056. [Crossref] [PubMed]
- Abramian O, Singhal S, Stephen MJ A. 49-Year-Old Man With Cough and Hand, Wrist, and Knee Pain. Chest 2020;157:e47-51. [Crossref] [PubMed]
- Mishra S. Deep Transfer Learning-Based Framework for COVID-19 Diagnosis Using Chest CT Scans and Clinical Information. SN Comput Sci 2021;2:390. [Crossref] [PubMed]
- Lu MT, Raghu VK, Mayrhofer T, et al. Deep Learning Using Chest Radiographs to Identify High-Risk Smokers for Lung Cancer Screening Computed Tomography: Development and Validation of a Prediction Model. Ann Intern Med 2020;173:704-13. [Crossref] [PubMed]
- Trajanovski S, Mavroeidis D, Swisher CL, et al. Towards radiologist-level cancer risk assessment in CT lung screening using deep learning. Comput Med Imaging Graph 2021;90:101883. [Crossref] [PubMed]
- Huang P, Lin CT, Li Y, et al. Prediction of lung cancer risk at follow-up screening with low-dose CT: a training and validation study of a deep learning method. Lancet Digit Health 2019;1:e353-62. [Crossref] [PubMed]
- Jacobs C, Setio AAA, Scholten ET, et al. Deep Learning for Lung Cancer Detection on Screening CT Scans: Results of a Large-Scale Public Competition and an Observer Study with 11 Radiologists. Radiol Artif Intell 2021;3:e210027. [Crossref] [PubMed]
- Stacchiotti S, Miah AB, Frezza AM, et al. Epithelioid hemangioendothelioma, an ultra-rare cancer: a consensus paper from the community of experts. ESMO Open 2021;6:100170. [Crossref] [PubMed]
- Ettinger DS, Wood DE, Aisner DL, et al. Non-Small Cell Lung Cancer, Version 3.2022, NCCN Clinical Practice Guidelines in Oncology. J Natl Compr Canc Netw 2022;20:497-530. [Crossref] [PubMed]
- Fernández Pérez ER, Travis WD, Lynch DA, et al. Diagnosis and Evaluation of Hypersensitivity Pneumonitis: CHEST Guideline and Expert Panel Report. Chest 2021;160:e97-e156. [Crossref] [PubMed]
- WHO Guidelines Approved by the Guidelines Review Committee. WHO consolidated guidelines on drug-resistant tuberculosis treatment. Geneva: World Health Organization; 2019.
- Wu N, Phang J, Park J, et al. Deep Neural Networks Improve Radiologists' Performance in Breast Cancer Screening. IEEE Trans Med Imaging 2020;39:1184-94. [Crossref] [PubMed]
- Woo JH, Kim TJ, Lee KS, et al. Epithelioid hemangioendothelioma in the thorax: Clinicopathologic, CT, PET, and prognostic features. Medicine (Baltimore) 2016;95:e4348. [Crossref] [PubMed]
- Nguyen T, Chagani F, Khasawneh M, et al. Epithelioid Hemangioendothelioma Presenting as Necrotizing Pneumonia. Cureus 2023;15:e39328. [Crossref] [PubMed]
- Cronin P, Arenberg D. Pulmonary epithelioid hemangioendothelioma: an unusual case and a review of the literature. Chest 2004;125:789-93. [Crossref] [PubMed]
- Kim EY, Kim TS, Han J, et al. Thoracic epithelioid hemangioendothelioma: imaging and pathologic features. Acta Radiol 2011;52:161-6. [Crossref] [PubMed]
- Epelboym Y, Engelkemier DR, Thomas-Chausse F, et al. Imaging findings in epithelioid hemangioendothelioma. Clin Imaging 2019;58:59-65. [Crossref] [PubMed]
- Liu H, Wang J, Lang J, et al. Pulmonary Epithelioid Hemangioendothelioma: Imaging and Clinical Features. J Comput Assist Tomogr 2021;45:788-94. [Crossref] [PubMed]
- Dail DH, Liebow AA, Gmelich JT, et al. Intravascular, bronchiolar, and alveolar tumor of the lung (IVBAT). An analysis of twenty cases of a peculiar sclerosing endothelial tumor. Cancer 1983;51:452-64. [Crossref] [PubMed]
- Jinghong X, Lirong C. Pulmonary epithelioid hemangioendothelioma accompanied by bilateral multiple calcified nodules in lung. Diagn Pathol 2011;6:21. [Crossref] [PubMed]
- Briens E, Caulet-Maugendre S, Desrues B, et al. Alveolar haemorrhage revealing epithelioid haemangioendothelioma. Respir Med 1997;91:111-4. [Crossref] [PubMed]
- Struhar D, Sorkin P, Greif J, et al. Alveolar haemorrhage with pleural effusion as a manifestation of epithelioid haemangioendothelioma. Eur Respir J 1992;5:592-3.
- Sasaki A, Egashira H, Sugimoto H, et al. CT-guided Biopsy for the Diagnosis of Pulmonary Epithelioid Hemangioendothelioma Mimicking Metastatic Lung Cancer. Intern Med 2018;57:3631-5. [Crossref] [PubMed]
- Haro A, Saitoh G, Tamiya S, et al. Four-year natural clinical course of pulmonary epithelioid hemangioendothelioma without therapy. Thorac Cancer 2015;6:544-7. [Crossref] [PubMed]
- Xiong W, Wang Y, Ma X, et al. Multiple bilateral pulmonary epithelioid hemangioendothelioma mimicking metastatic lung cancer: case report and literature review. J Int Med Res 2020;48:300060520913148. [Crossref] [PubMed]
- Bahrami A, Allen TC, Cagle PT. Pulmonary epithelioid hemangioendothelioma mimicking mesothelioma. Pathol Int 2008;58:730-4. [Crossref] [PubMed]
- Stacchiotti S, Simeone N, Lo Vullo S, et al. Activity of sirolimus in patients with progressive epithelioid hemangioendothelioma: A case-series analysis within the Italian Rare Cancer Network. Cancer 2021;127:569-76. [Crossref] [PubMed]
- Bagan P, Hassan M, Le Pimpec Barthes F, et al. Prognostic factors and surgical indications of pulmonary epithelioid hemangioendothelioma: a review of the literature. Ann Thorac Surg 2006;82:2010-3. [Crossref] [PubMed]
- Anderson T, Zhang L, Hameed M, et al. Thoracic epithelioid malignant vascular tumors: a clinicopathologic study of 52 cases with emphasis on pathologic grading and molecular studies of WWTR1-CAMTA1 fusions. Am J Surg Pathol 2015;39:132-9. [Crossref] [PubMed]
- Shibayama T, Makise N, Motoi T, et al. Clinicopathologic Characterization of Epithelioid Hemangioendothelioma in a Series of 62 Cases: A Proposal of Risk Stratification and Identification of a Synaptophysin-positive Aggressive Subset. Am J Surg Pathol 2021;45:616-26. [Crossref] [PubMed]
- Nizami I, Mohammed S, Abouzied Mel D. Pulmonary epitheloid hemangioendothelioma PET CT findings and review of literature. Ann Saudi Med 2014;34:447-9. [Crossref] [PubMed]
- Jiang B, Li N, Shi X, et al. Deep Learning Reconstruction Shows Better Lung Nodule Detection for Ultra-Low-Dose Chest CT. Radiology 2022;303:202-12. [Crossref] [PubMed]
- Park YJ, Choi D, Choi JY, et al. Performance Evaluation of a Deep Learning System for Differential Diagnosis of Lung Cancer With Conventional CT and FDG PET/CT Using Transfer Learning and Metadata. Clin Nucl Med 2021;46:635-40. [Crossref] [PubMed]
- Walsh SLF, Calandriello L, Silva M, et al. Deep learning for classifying fibrotic lung disease on high-resolution computed tomography: a case-cohort study. Lancet Respir Med 2018;6:837-45. [Crossref] [PubMed]
- Galateau Salle F, Le Stang N, Tirode F, et al. Comprehensive Molecular and Pathologic Evaluation of Transitional Mesothelioma Assisted by Deep Learning Approach: A Multi-Institutional Study of the International Mesothelioma Panel from the MESOPATH Reference Center. J Thorac Oncol 2020;15:1037-53. [Crossref] [PubMed]
- Choe J, Hwang HJ, Seo JB, et al. Content-based Image Retrieval by Using Deep Learning for Interstitial Lung Disease Diagnosis with Chest CT. Radiology 2022;302:187-97. [Crossref] [PubMed]
- Park SY, Kim YW, Song YR, et al. Compound-level identification of sasang constitution type-specific personalized herbal medicine using data science approach. Heliyon 2023;9:e13692. [Crossref] [PubMed]
- Liang Y, Wang H, Yang J, et al. A Deep Learning Framework to Predict Tumor Tissue-of-Origin Based on Copy Number Alteration. Front Bioeng Biotechnol 2020;8:701. [Crossref] [PubMed]
- Tian G, Wang Z, Wang C, et al. A deep ensemble learning-based automated detection of COVID-19 using lung CT images and Vision Transformer and ConvNeXt. Front Microbiol 2022;13:1024104. [Crossref] [PubMed]
- Wang SH, Nayak DR, Guttery DS, et al. COVID-19 classification by CCSHNet with deep fusion using transfer learning and discriminant correlation analysis. Inf Fusion 2021;68:131-48. [Crossref] [PubMed]
- Kpodonu J, Tshibaka C, Massad MG. The importance of clinical registries for pulmonary epithelioid hemangioendothelioma. Chest 2005;127:1870-1; author reply 1871. [Crossref] [PubMed]
- Shi F, Chen B, Cao Q, et al. Semi-Supervised Deep Transfer Learning for Benign-Malignant Diagnosis of Pulmonary Nodules in Chest CT Images. IEEE Trans Med Imaging 2022;41:771-81. [Crossref] [PubMed]
- Son JW, Hong JY, Kim Y, et al. How Many Private Data Are Needed for Deep Learning in Lung Nodule Detection on CT Scans? A Retrospective Multicenter Study. Cancers (Basel) 2022;14:3174. [Crossref] [PubMed]
- Gao R, Zhao S, Aishanjiang K, et al. Deep learning for differential diagnosis of malignant hepatic tumors based on multi-phase contrast-enhanced CT and clinical data. J Hematol Oncol 2021;14:154. [Crossref] [PubMed]