Bioinformatics analysis of the prognostic role of alternative splicing data in lung adenocarcinoma
Highlight box
Key findings
• This study used bioinformatics techniques to reveal the value of some alternative splicing events (ASEs) in the prognosis of lung adenocarcinoma (LUAD) patients. And because of this, it may provide a new idea for revealing potential prognostic biomarkers and treatment targets of LUAD patients.
What is known and what is new?
• In the past few decades, the existence of alternative splicing (AS) has been identified as a driver of cancer by many studies, and has become a new biomarker for other cancers. Studies have shown that AS is connected with the proliferation and invasion of cancer cells. What’s more, studies have shown that different types of ASEs and polarization of macrophages can promote metastasis of lung cancer.
• This study integrated the ASE spectrum and clinical data of LUAD patients, and identified ASEs related to LUAD. This study may show that ASEs can be used as a new biomarker related to the prognosis of LUAD patients. We even established the model to evaluate the relationship between ASEs and the prognosis of LUAD. The study also showed that ASE risk score could be used as an independent predictor of prognosis in LUAD patients, and splicing factors could well predict the survival of LUAD patients.
What is the implication, and what should change now?
• The prediction model may provide ideas for finding specific targets to improve the survival rate of LUAD. However, the specific mechanism of the role of AS on the prognosis of LUAD patients remains to be further explored.
Introduction
Global cancer statistics in 2020 showed that in 2020, the number of new cases of lung cancer accounted for the second of all new cancer cases, and its new deaths accounted for the first of all cancer deaths, which is one of the main causes of cancer deaths (1). Lung cancer is divided into non-small cell lung cancer (NSCLC) and small cell lung cancer (SCLC). Lung adenocarcinoma (LUAD) is the most common histological type of NSCLC (2). According to the World Health Organization (WHO), it has five subtypes. Recently, however, researchers have proposed that Cribri-p is a sixth pattern (3). Treatments options for LUAD include first-line therapy and the current hot immunological and targeted therapies. Although great progress has been made in the treatment of LUAD (4-6), the mortality rate is still high due to individual differences, epidermal growth factor receptor (EGFR) mutations, and drug resistance to chemotherapeutic drugs. Existing biomarkers such as BRAF can no longer meet current needs, so there is an urgent need to research new biomarkers to provide new targets for the treatment of LUADs (7).
Alternative splicing (AS), also known as selective splicing, enables the precursor messenger RNA (mRNA) to produce different mRNA splicing isomers, resulting in different proteins (8). As a post-transcriptional regulatory mechanism, AS is connected with a range of pathophysiological processes, and is especially related to the occurrence, progression, metastasis, and drug resistance of cancer (9). Exploring abnormal ASEs will help us to study the underlying developmental and prognostic mechanisms in LUAD patients. In addition, according to relevant research, ASEs have become an essential part of the formation of tumor microenvironment (10).
The tumor immune microenvironment (TIME) is a dynamic system that provides the necessary conditions for tumor survival. It includes endothelial cells, fibroblasts, pericytes, and immune infiltrating cells (10). Among them, B cells, regulatory T (Treg) cells, and tumor-associated macrophage (TAM) cells play a role in promoting LUAD. ASEs change the TIME through immune infiltrating cells, thus predicting the development and prognosis of gastric cancer, bowel cancer, and other tumors (11,12). In this study, LUAD RNA sequencing (RNAseq) data and TCGASpliceSeq data were downloaded from The Cancer Genome Atlas (TCGA) database to screen the AS data related to the prognosis of LUAD patients, and to explore the mechanism affecting the prognosis of LUAD patients. We present this article in accordance with the TRIPOD reporting checklist (available at https://jtd.amegroups.com/article/view/10.21037/jtd-24-6/rc).
Methods
Data collection and organization
The RNAseq data used in this review were obtained from TCGA database and then processed data. Clinical data included 486 patients with complete age, sex, definite TNM stage, and follow-up time. In addition, seven types of AS event (ASE) data are provided in TCGASpliceSeq database [alternating acceptor (AA), alternating donor (AD), alternating promoter (AP), alternating terminator (AT), exon skipping (ES); mutually exclusive exon (ME), and retained intron (RI)]. The study was conducted in accordance with the Declaration of Helsinki (as revised in 2013).
Construction of an independent predictive model
An UpSet plot was used to directly show the relationship between ASEs and genes. The total lifetime of LUAD was downloaded from the TCGA database. To screen for ASEs associated with survival, we divided patients into two groups based on the median PSI value in each ASE and performed a univariate Cox analysis. Splicing events with expression P value <0.05 were selected as survival-related ASEs. A volcano plot was used to show ASEs related and not related to prognosis. The top 20 overall survival-related splicing events (OS-SEs) for each splicing mode were filtered out based on the P value and displayed in a bubble chart. Furthermore, in order to keep off over-fitting of the prognostic model, least absolute shrinkage and selection operator (LASSO) regression and multivariate Cox regression were used to analyze the relationship between each AS in LUAD and prognosis. Then, on the basis of the median risk score, the samples were separated into a high-risk group and a low-risk group. The survival curve of each group was shown by Kaplan-Meier survival analysis, and the receiver operating characteristic (ROC) curve was used to verify the accuracy of the model. After that, the effectiveness of the model was verified under different methods.
Statistical analysis
The correlation between the prognosis of LUAD and AS was analyzed by Spearman correlation analysis. Kaplan-Meier curve was used to draw OS diagrams among different groups, and ROC curves were used to verify the reliability of this model. Then, univariate and multivariate Cox regression analyses were carried out to determine the independent predictors. All P values were calculated on the strength of bilateral statistical testing, and P<0.05 was deemed to be statistically significant.
Results
Discovery of prognosis-related ASEs and OS-SEs from the dataset
An enzyme can occur in various types of genes and a gene can be localized in various types of splicing patterns (Figure 1A). ASEs associated with prognosis and their P values were shown in volcano and forest plots, respectively (Figure 1B and Figure S1). The bubble map showed the top 20 ASEs in seven splicing patterns (Figure 1C). Among all ASEs, KIAA0895L-36956-AA, INTS2-42894-AD, SH3KBP1-88643-AP, BEST3-23330-A, IL1R1-54779-ES, TPM2-86278-ME, and C10orf32-12982-RI were the most remarkable events for AA, AD, AP, AT, ES, ME, and RI, respectively.
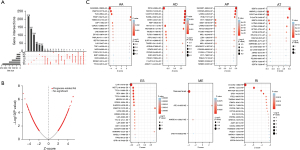
Construction and analysis of the independent prognostic model
The prognostic marker was constructed for LUAD using several of the top 20 OS-SEs. To avoiding over-fitting, a LASSO plot and Lambda plot were performed (Figure 2A). The whole sample was divided into two groups, and the survival probability survival of each group was shown by the results of survival analysis (Figure 2B), which indicated that there was a huge difference between them. Compared with low-risk patients, the OS of high-risk patients was significantly worse. The Kaplan-Meier curve supported the reliability of the model (P<0.001). The time-dependent ROC curve showed that the area under the curve (AUC) values of characteristic long non-coding RNA (lncRNA) for 1-, 3-, and 5-year survival rates were 0.806, 0.752, and 0.746, respectively (Figure 2C). The time-dependent ROC curve showed that AUC value of related genes was 0.806, which was much better than the age (AUC =0.555), gender (AUC =0.604), and stage (AUC =0.718) in predicting the prognosis of LUAD (Figure 2D). The concordance index verified the consistency between the predicted results and the actual results, which showed that predicted results were reliable (Figure 2E).
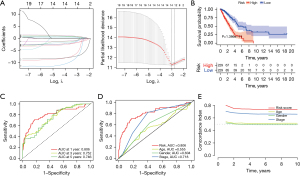
Results of the independent prognostic model
In order to verify the independence of risk score, univariate and multivariate Cox regression analysis was carried out on age, sex, stage, and so on. Since the P value of risk scores in both analyses was smaller than 0.001, the risk ratios were 1.011 [95% confidence interval (CI): 1.007–1.015] and 1.011 (95% CI: 1.007–1.015), respectively. The risk score was thus shown to be a good prediction model (Figure 3A,3B). Then, we use signatures and traditional clinicopathological features including sex, age, and staging constructing the nomogram. The results showed that the nomogram using signature risk scores is reliable and accurate (Figure 3C). The nomogram was basically consistent with the actual predicted OS, which further showed that the prediction model is reliable (Figure 3D). Therefore, SFs can be used as a predictor of survival.
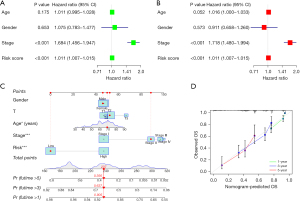
Prognostic analysis
Based on the previous data, we established a prognostic model. Then, we analyzed the prognosis of LUAD in different stages. As shown in Figure 4A, among patients with LUADs in stage I–II, low-risk patients have better OS than high-risk patients. Coincidentally, this phenomenon also occurs in patients with LUAD in stage III–IV (Figure 4B). Later, we divided the samples into three groups, and SFTPA1 had the greatest influence on gene expression (Figure 4C).
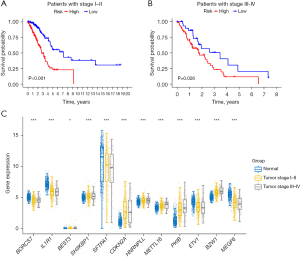
Gene mutations in LUAD patients in the high- and low-risk groups
Mutated genes were analyzed in order to comprehend the biological characteristics of related genes. Among these two separate groups, the first 20 genes had the highest mutation rate (Figure 5), and the number of mutations in the high-risk group was significantly higher than that in the low-risk group. The mutation rate of each gene was more than 10% in both high- and low-risk groups. Missense mutation was identified as the most common kind of mutation, followed by nonsense mutation.
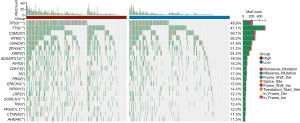
Expression of immune-related genes
The expression of different immune-related genes was analyzed. The heat map showed that there was less ASE expression in the low-risk group and more in the high-risk group (Figure 6).
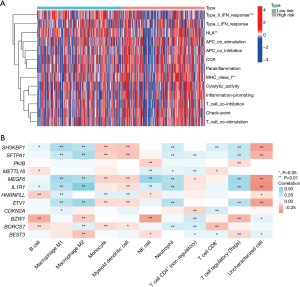
Discussion
Lung cancer is the most common malignant tumor in the respiratory system, and its high morbidity and mortality have been the focus of global attention. According to the data released by the Global Cancer Observatory (GLOBOCAN) 2020, lung cancer ranks first in the cause of death among malignant tumors in the world and in China (1). The pathogenesis of lung cancer is not very clear, and it is related to many factors. A recent meta-analysis showed that in addition to smoking, cooking habits, diet, smoking, cancer and lung health history, as well as female reproductive history are associated with lung cancer in non-smoking women (13). In addition, age, race, socioeconomic status, geographic location, and hormone replacement therapy may also be associated with the incidence of lung cancer (14,15). LUAD is the most common type of lung cancer, and in recent years, combination therapy has made substantial progress, but the prognosis of LUAD patients is still poor, and the 5-year survival rate is less than 20% (16). Therefore, the identification of new prognostic markers is very important for personalized treatment and prognosis of patients with LUAD.
AS, also known as selective splicing, enables a single gene to produce multiple mRNA, which provides a mechanism for proteome diversity for cells and plays a key role in the occurrence or maintenance of malignant tumors (17). In the past few decades, the existence of AS has been identified as a driver of cancer by many studies, and has become a new biomarker (17,18). A study has shown that AS is connected with the proliferation and invasion of cancer cells (19). Different types of ASEs and polarization of macrophages can promote metastasis of lung cancer (20). High expression of COLIA1 can promote the proliferation and metastasis of cervical cancer cells (21). Androgen receptor splice variant 7 (AR-V7) is a structurally active transcription factor. AR-V7 expression is associated with drug resistance and poor clinical prognosis of androgen receptor signaling inhibitors (ARSi) (22). In addition to participating in the pathway of chemotherapeutic resistance, AS can also affect the efficacy of chemotherapeutic drugs through abnormal splicing of molecular targets (23-25). Surprisingly, AS could provide new insights into anti-cancer metabolic therapy (26). Based on this, this review hypothesizes that AS may be a potential molecular marker for predicting the prognosis of patients with LUAD.
This study integrated the ASE spectrum and clinical data of LUAD patients, and identified ASEs related to LUAD. The differences of seven ASEs related to prognosis were KIAA0895L (AA), INTS2 (AD), SH3KBP1 (AP), BEST3 (AT), IL1R1 (ES), TPM2 (ME), and C10orf32 (RI). Among them, SH3KBP1 is highly expressed in glioblastoma stem cells, resulting in poor survival of glioma patients, affecting tumor cell proliferation, migration, and germline stem cells (GSCs) self-renewal ability (27). In addition, TPM2 is associated with the prognosis of patients with breast, colorectal, and prostate cancer, and is involved in tumor progression and chemotherapy resistance (28-30). In this study, the correlation between ASEs and the prognosis of LUAD patients showed that ASEs can be used as a new biomarker related to the prognosis of LUAD patients. In order to further evaluate the relationship between ASEs and the prognosis of LUAD, a prognostic model was established, which could clearly distinguish the OS rate of patients with high risk and low risk of LUAD. The AUC was 0.536, which shows the reliability of the risk model for prediction. The concordance index further illustrated the reliability of the model. Furthermore, univariate and multivariate Cox regression analyses were conducted between the risk score in the prognostic model and the clinical parameters of LUAD patients (age, sex, and stage). The results showed that ASE risk score could be used as an independent predictor of prognosis in LUAD patients, and SFs could well predict the survival of LUAD patients. As the main upstream regulator of ASEs, SFs play a role in the progression of cancer by mediating the AS process. The advantage of the prediction model in this study is that it may provide ideas for finding specific targets to improve the survival rate of LUAD. However, the specific mechanism of as affecting the prognosis of LUAD needs to be further studied. Moreover, the biggest problem facing the study is that it is entirely based on biological analysis and lacks some clinical data and in vitro experiments to further verify.
Conclusions
This study used bioinformatics techniques to screen survival-related ASEs in LUAD patients, revealed the value of some ASEs in the prognosis of LUAD patients, and provided a new idea for revealing potential prognostic biomarkers and treatment targets of LUAD patients.
Acknowledgments
Funding: The present study was supported by
Footnote
Reporting Checklist: The authors have completed the TRIPOD reporting checklist. Available at https://jtd.amegroups.com/article/view/10.21037/jtd-24-6/rc
Peer Review File: Available at https://jtd.amegroups.com/article/view/10.21037/jtd-24-6/prf
Conflicts of Interest: All authors have completed the ICMJE uniform disclosure form (available at https://jtd.amegroups.com/article/view/10.21037/jtd-24-6/coif). The authors have no conflicts of interest to declare.
Ethical Statement: The authors are accountable for all aspects of the work in ensuring that questions related to the accuracy or integrity of any part of the work are appropriately investigated and resolved. The study was conducted in accordance with the Declaration of Helsinki (as revised in 2013).
Open Access Statement: This is an Open Access article distributed in accordance with the Creative Commons Attribution-NonCommercial-NoDerivs 4.0 International License (CC BY-NC-ND 4.0), which permits the non-commercial replication and distribution of the article with the strict proviso that no changes or edits are made and the original work is properly cited (including links to both the formal publication through the relevant DOI and the license). See: https://creativecommons.org/licenses/by-nc-nd/4.0/.
References
- Sung H, Ferlay J, Siegel RL, et al. Global Cancer Statistics 2020: GLOBOCAN Estimates of Incidence and Mortality Worldwide for 36 Cancers in 185 Countries. CA Cancer J Clin 2021;71:209-49. [Crossref] [PubMed]
- Bade BC, Dela Cruz CS. Lung Cancer 2020: Epidemiology, Etiology, and Prevention. Clin Chest Med 2020;41:1-24. [Crossref] [PubMed]
- Nakajima N, Yoshizawa A, Rokutan-Kurata M, et al. Prognostic significance of cribriform adenocarcinoma of the lung: validation analysis of 1,057 Japanese patients with resected lung adenocarcinoma and a review of the literature. Transl Lung Cancer Res 2021;10:117-27. [Crossref] [PubMed]
- Jia K, Wu Y, Huang J, et al. Survival-Associated Alternative Splicing Events in Pan-Renal Cell Carcinoma. Front Oncol 2019;9:1317. [Crossref] [PubMed]
- Wan Q, Sang X, Jin L, et al. Alternative Splicing Events as Indicators for the Prognosis of Uveal Melanoma. Genes (Basel) 2020;11:227. [Crossref] [PubMed]
- Lin X, Zhou T, Hu S, et al. Prognostic significance of pyroptosis-related factors in lung adenocarcinoma. J Thorac Dis 2022;14:654-67. [Crossref] [PubMed]
- Dora D, Vörös I, Varga ZV, et al. BRAF RNA is prognostic and widely expressed in lung adenocarcinoma. Transl Lung Cancer Res 2023;12:27-41. [Crossref] [PubMed]
- Raveh E, Matouk IJ, Gilon M, et al. The H19 Long non-coding RNA in cancer initiation, progression and metastasis - a proposed unifying theory. Mol Cancer 2015;14:184. [Crossref] [PubMed]
- Zhang Y, Yao X, Zhou H, et al. OncoSplicing: an updated database for clinically relevant alternative splicing in 33 human cancers. Nucleic Acids Res 2022;50:D1340-7. [Crossref] [PubMed]
- Ma S, Zhu J, Wang M, et al. A comprehensive characterization of alternative splicing events related to prognosis and the tumor microenvironment in lung adenocarcinoma. Ann Transl Med 2022;10:479. [Crossref] [PubMed]
- Kim EK, Yoon SO, Jung WY, et al. Implications of NOVA1 suppression within the microenvironment of gastric cancer: association with immune cell dysregulation. Gastric Cancer 2017;20:438-47. [Crossref] [PubMed]
- Wan L, Yu W, Shen E, et al. SRSF6-regulated alternative splicing that promotes tumour progression offers a therapy target for colorectal cancer. Gut 2019;68:118-29. [Crossref] [PubMed]
- Huang J, Yue N, Shi N, et al. Influencing factors of lung cancer in nonsmoking women: systematic review and meta-analysis. J Public Health (Oxf) 2022;44:259-68. [Crossref] [PubMed]
- MacRosty CR, Rivera MP. Lung Cancer in Women: A Modern Epidemic. Clin Chest Med 2020;41:53-65. [Crossref] [PubMed]
- Jin C, Lang B. Hormone replacement therapy and lung cancer risk in women: a meta-analysis of cohort studies: Hormone replacement therapy and lung cancer risk. Medicine (Baltimore) 2019;98:e17532. [Crossref] [PubMed]
- Chen Z, Fillmore CM, Hammerman PS, et al. Non-small-cell lung cancers: a heterogeneous set of diseases. Nat Rev Cancer 2014;14:535-46. [Crossref] [PubMed]
- Urbanski LM, Leclair N, Anczuków O. Alternative-splicing defects in cancer: Splicing regulators and their downstream targets, guiding the way to novel cancer therapeutics. Wiley Interdiscip Rev RNA 2018;9:e1476. [Crossref] [PubMed]
- Cerasuolo A, Buonaguro L, Buonaguro FM, et al. The Role of RNA Splicing Factors in Cancer: Regulation of Viral and Human Gene Expression in Human Papillomavirus-Related Cervical Cancer. Front Cell Dev Biol 2020;8:474. [Crossref] [PubMed]
- Soubise B, Jiang Y, Douet-Guilbert N, et al. RBM22, a Key Player of Pre-mRNA Splicing and Gene Expression Regulation, Is Altered in Cancer. Cancers (Basel) 2022;14:643. [Crossref] [PubMed]
- Zhao Y, Li M, Wu W, et al. Downregulated ESRP1/2 promotes lung metastasis of bladder carcinoma through altering FGFR2 splicing and macrophage polarization. Front Immunol 2023;14:1161273. [Crossref] [PubMed]
- Huang X, Shi H, Shi X, et al. LncRNA FBXL19-AS1 promotes proliferation and metastasis of cervical cancer through upregulating COL1A1 as a sponge of miR-193a-5p. J Biol Res (Thessalon) 2021;28:20. [Crossref] [PubMed]
- Melnyk JE, Steri V, Nguyen HG, et al. The splicing modulator sulfonamide indisulam reduces AR-V7 in prostate cancer cells. Bioorg Med Chem 2020;28:115712. [Crossref] [PubMed]
- de Fraipont F, Gazzeri S, Cho WC, et al. Circular RNAs and RNA Splice Variants as Biomarkers for Prognosis and Therapeutic Response in the Liquid Biopsies of Lung Cancer Patients. Front Genet 2019;10:390. [Crossref] [PubMed]
- Wojtuszkiewicz A, Assaraf YG, Maas MJ, et al. Pre-mRNA splicing in cancer: the relevance in oncogenesis, treatment and drug resistance. Expert Opin Drug Metab Toxicol 2015;11:673-89. [Crossref] [PubMed]
- Chen QW, Cai QQ, Yang Y, et al. LncRNA BC promotes lung adenocarcinoma progression by modulating IMPAD1 alternative splicing. Clin Transl Med 2023;13:e1129. [Crossref] [PubMed]
- Ma Z, Chen H, Xia Z, et al. Energy stress-induced circZFR enhances oxidative phosphorylation in lung adenocarcinoma via regulating alternative splicing. J Exp Clin Cancer Res 2023;42:169. [Crossref] [PubMed]
- Song H, Wang Y, Shi C, et al. SH3KBP1 Promotes Glioblastoma Tumorigenesis by Activating EGFR Signaling. Front Oncol 2020;10:583984. [Crossref] [PubMed]
- Zhang J, Zhang J, Xu S, et al. Hypoxia-Induced TPM2 Methylation is Associated with Chemoresistance and Poor Prognosis in Breast Cancer. Cell Physiol Biochem 2018;45:692-705. [Crossref] [PubMed]
- Liu S, Zeng F, Fan G, et al. Identification of Hub Genes and Construction of a Transcriptional Regulatory Network Associated With Tumor Recurrence in Colorectal Cancer by Weighted Gene Co-expression Network Analysis. Front Genet 2021;12:649752. [Crossref] [PubMed]
- Varisli L. Identification of new genes downregulated in prostate cancer and investigation of their effects on prognosis. Genet Test Mol Biomarkers 2013;17:562-6. [Crossref] [PubMed]