Artificial intelligence (AI)-assisted chest computer tomography (CT) insights: a study on intensive care unit (ICU) admittance trends in 78 coronavirus disease 2019 (COVID-19) patients
Highlight box
Key findings
• Our study used artificial intelligence (AI)-supported LungCTAnalyzer software to analyze chest computed tomography (CT) scans of 78 coronavirus disease 2019 (COVID-19) patients, revealing a significant correlation between lung involvement and intensive care unit (ICU) admission needs.
What is known and what is new?
• Prior research identified chest CT as an important tool in managing COVID-19. We took this a step further by quantitatively analyzing CT scans with AI for infiltrations and lung collapse in order to forecast ICU requirements, demonstrating a high correlation between AI and radiologist assessments.
• This manuscript offers empirical evidence of the effectiveness and accuracy of computerized lung CT analysis in pandemics such as COVID-19.
What is the implication, and what should change now?
• AI-driven computer analysis should be integrated into clinical practice for better decision-making and resource allocation, potentially improving patient outcomes in the ongoing pandemic and in future health crises.
Introduction
The coronavirus disease 2019 (COVID-19) pandemic is caused by severe acute respiratory syndrome coronavirus 2 (SARS-CoV-2), which was first identified in December 2019 in Wuhan, China (1). The virus quickly became a global pandemic, with hundreds of millions of cases and almost seven million deaths worldwide (2,3).
Chest computed tomography (CT) imaging plays an important role in the diagnosis and management of COVID-19, particularly in cases where reverse transcription polymerase chain reaction (RT-PCR) testing is not available or inconclusive (4,5). CT imaging reveals characteristic features of the disease, provides information for diagnosis and staging, and can also help to monitor disease progression and assess the response to treatment (6).
Automated analysis of COVID-19-related chest CT scans would be very desirable for several reasons. Most importantly, the infiltrations and consolidations could be expressed as affected volume and directly quantified. The distribution of affected tissue in the sides of the lungs, lobes, and lung regions could be analyzed. Automatic processing could help to reduce the workload of radiologists and other healthcare professionals, enabling them to focus on patient care and other important tasks. Automation also has the potential to be more consistent than manual review, if it can be shown to be robust to differences across multiple clinical sites. Open-source availability of all software components would enable identical computations on computer hardware around the world which would support future pandemic preparedness.
We recently developed a free and open-source software—LungCTAnalyzer (7)—for the medical imaging software 3D Slicer (8). The software is using artificial intelligence (AI)-tools for lung and lobe segmentation. It is designed specifically for the analysis of lung CT scans, offering a comprehensive set of tools for the segmentation, quantification, and visualization of lung structures. By leveraging the power of 3D Slicer and building upon its functionalities, LungCTAnalyzer provides a user-friendly interface and a robust set of features that streamline the process of lung image analysis for the detection of emphysema, normal lung tissue, infiltrated and collapsed parenchyma.
As an open-source COVID dataset with radiologist scores has been published (9), the present study aims to quantitatively analyze the data to determine the accordance of computer vs. human analysis to demonstrate the feasibility of computer analysis with LungCTAnalyzer and to elaborate on the existing differences in lung involvement between the severity-scored groups, to evaluate whether tissue affection in severe cases could be attributed to the side of lungs, lung regions or lung lobes and to predict patients requiring intensive care unit (ICU)-treatment. We present this article in accordance with the MDAR reporting checklist (available at https://jtd.amegroups.com/article/view/10.21037/jtd-23-1150/rc).
Methods
Ethical statement
All ethical statements have been included in the dataset paper (9). The study was conducted according to the guidelines of the Declaration of Helsinki (as revised in 2013).
Patient population and open-source dataset
The dataset used in this study has been recently published and expanded (9). In short, it includes data from 81 COVID-19 patients (diagnoses confirmed by positive RT-PCR tests), 50 during the first phase of COVID pandemic between April 2020 and May 2021 and 31 during the second between December 2020 and May 2021. They underwent non-contrast chest CT scans at Azienda Ospedaliera Pugliese-Ciaccio (Catanzaro, Italy). These scans feature volume reconstructions with 0.3 to 1 mm slice thickness. The average age of the patients was 56 years, ranging from 20 to 83, and the male-to-female ratio was 42:39. Two distinct scanners were utilized at the clinical facility: Siemens Somatom Go. now (Siemens Healthineers GmbH, Erlangen, Germany) and Toshiba Aquilion ONE (Canon Medical System Europe B.V., Zoetermeer, The Netherlands). Among the patients, 81 underwent a single CT scan for diagnostic purposes, while 12 received additional scans for follow-up, resulting in a total of 93 CT volumes in the database. To facilitate data sharing and processing, the DICOM files were anonymized and converted into NRRD format (http://teem.sourceforge.net/nrrd/, accessed on 15 February 2021) using 3D Slicer (8).
Visual assessment
Primary findings regarding COVID-19 in CT images involve atypical pneumonia and encompass two macroscopic lung tissue abnormalities: ground-glass opacity (GGO), which is an area of increased X-ray attenuation in the lung with preserved bronchial and vascular markings, and consolidation, referring to the filling of the pulmonary tree with material that attenuates X-rays more than the surrounding lung parenchyma.
An expert radiologist (Aldo Marzulla), one of the authors of the dataset paper (9), with over 20 years of experience) was tasked with visually evaluating the CT images and assigning a clinical score, based on the extent of lung involvement with the aforementioned manifestations. The radiologist navigated through the volume on his radiological workstation in axial, sagittal, and coronal planes, assigning a lung involvement classification scale ranging from 0, indicating no lung involvement (0%), to 5, representing severe lung involvement (greater than 75%), with intermediate levels covering minimal (less than 5%), mild (5% to 25%), moderate (26% to 50%), and significant (51% to 75%) involvement. This took about 2 to 3 minutes per case. These scores are included in the open dataset alongside each image volume.
In an effort to appraise the potential for interobserver bias through a comparison between human evaluators, a second radiological specialist (N.K.B., a co-author of this study) undertook an independent visual assessment using an identical scoring system. Intriguingly, out of the 81 patients evaluated, she deemed three as “not classifiable” (one patient with incomplete CT and two patients with pneumothorax). In addition, in 19 patients we noted possible preexisting lung disease (11 patients with possible fibrosis, 2 patients with COPD, 4 patients with non-COVID type interstitial lung alterations, 1 patient with a lung tumor and 1 patient with atelectasis of a lung lobe).
We made a deliberate decision to exclude the three unclassifiably patients from the study and to retain the other three patients with possible lung disease in the dataset for the sake of providing a comprehensive reference.
Automated segmentation of the lungs and lung pathologies
This study employed an automated approach for the segmentation of the lungs and lung pathologies using the free and open-source medical imaging software 3D Slicer (8), for which we wrote a special extension in the programming language Python: LungCTAnalyzer (7) using the same software license. The program can be run in batch processing mode, without manual interaction. CT data were imported into 3D Slicer as NRRD files.
Data calibration
During a preliminary scan of all datasets, it became evident that the CT scans exhibited some heterogeneity in HU range, quality, windowing, and the presence of artifacts. Utilizing the TotalSegmentator [no-new-U-Net (nnU-Net)] (10) for segmentation, we were able to automatically generate segments corresponding to the trachea and the left erector spinae muscle in the CT datasets. We then evaluated the median Hounsfield units (HU) of both segmented structures in both scanners [Toshiba: trachea, −931.6; muscle, 22.68 (mean HU). Siemens: trachea, −958.2; muscle, 24.25 (mean HU)]. A Python function then standardized CT scans by calibrating HU values for air and muscle. It takes a 3D NumPy array, mean HU values for air and muscle, and returns a standardized CT scan. The function calculates a linear transformation to map input HU values to desired output values (−1,000 HU for air, 30 HU for muscle, Figure 1). For the Toshiba scanner, we found a mean slope of 1.08 [standard deviation (SD), 0.06] and a mean intercept of 5.72 (SD, 16.46). For the Siemens scanner, we found a mean slope of 1.06 (SD, 0.06) and a mean intercept of 5.12 (SD, 16.98). The transformation is applied, and the adjusted CT scan is returned for further analysis. This calibration is intended to ensure consistency across datasets and facilitate further analysis.
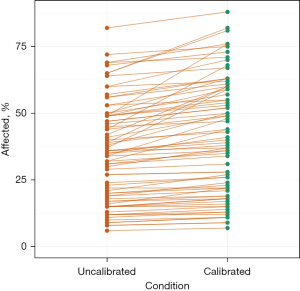
Lung and lobe segmentation
Next, the segmentation process began using the Lung CT Segmenter (LCTS) module. Trachea, left iliopsoas muscle, lungs, and lobes were segmented involving the 3D Slicer TotalSegmentator extension (11), which involves a tool for robust segmentation of 104 important anatomical structures in CT images with a well-trained neural network: nnU-Net (10). Mean radiodensity in HU of the trachea and iliopsoas muscle was automatically calculated (see above) for radiodensity evaluation. Each lung and lobe segmentation took about 114 s on the computer system described below.
Lung analysis segmentation
The calibrated scalar volume was then loaded into LungCTAnalyzer and lung analysis segments were generated for emphysema (−2,000 to −949 HU), normal lung (−950 to −749 HU), infiltrated lung/GGO (−750 to −399 HU), collapsed lung (−400 to 0 HU), and vessels (>0 HU). The volume of each of these segments was expressed in mm3. These segments were created for each lung, lobe, and the upper and lower half of each lung, as well as the anterior and posterior halves of each lung. To accomplish that, we calculated the centroid for each segmented lung and constructed a matching quadrant of markups which was then used to crop away the posterior half of the lung if the anterior half was to be preserved (and vice versa). The same technique was used for isolating the upper and lower half of each lung.
Thresholds were not changed throughout the complete study. Each lung analysis segmentation took 187 s.
The complete analysis of 78 datasets takes [(114+187) ×78]/60=391.3 minutes, with 5 minutes per case. This includes data loading and saving.
Percentage of COVID-affected lung
The 3D Slicer Segment Statistics tool was employed to analyze all generated lung structures and pathologies. The percentage of affected areas within both lungs, individual lungs, all lobes, and regions of interest were evaluated using the following formula: percent affected = [infiltrated volume (mL) + collapsed volume (mL)] ×100/organ volume (mL).
Vessel volumes were excluded from the total volumes, while airways were not considered. Ultimately, the findings were recorded in a CSV text file for further analysis and interpretation.
All processing was performed on the following systems:
- Device: DESKTOP Windows 11;
- Processor: 11th Gen Intel(R) Core(TM) i7-11700F @ 2.50GHz, 2.50 GHZ;
- Installed RAM: 32.0 GB;
- System type: 64-bit operating system, x64-based processor;
- GPU: NVIDIA GeForce RTX 3070 Ti, Driver version: 527.37, 8 GB dedicated video RAM;
- 3D Slicer 5.2.2;
- LungCTAnalyzer 2.65;
- TotalSegmentator 1.5.3;
- Pytorch 2.0.0+cu118.
The automated approach facilitated efficient and accurate segmentation of the lungs and lung pathologies, providing valuable insights into the disease progression and aiding in diagnosis and treatment planning.
Statistical analysis
The statistical analysis was conducted using R version 4.2.3 and RStudio version 2023.03.0 Build 386. The ggplot2 package was used to generate figures.
The Shapiro-Wilk normality test showed that the % COVID affection was not normally distributed between lungs and lobes (P=0.02375).
Thus, for group comparisons of COVID severity the Kruskal-Wallis test for group comparisons was used and the Wilcoxon rank sum test with Benjamini-Hochberg adjustment was added.
The reliability of the computed volume was evaluated using the intraclass correlation coefficient in the two-way random-effects model for intra- and inter-reader/retest assessments. Statistical significance was defined as a P value less than 0.05.
Results
Figure 2 illustrates a representative case of moderate COVID-19 manifestations, showcasing the automatic segmentation of lungs, lobes, and trachea without user intervention, using 3D Slicer’s LungCTSegmenter. Figure 3 demonstrates the automatic volumetric analysis of the same patient, applying the previously described thresholds through the 3D Slicer LungCTAnalyzer.
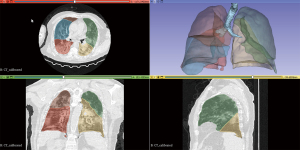
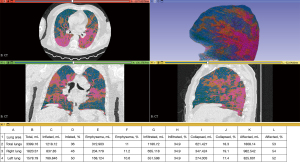
No significant difference between COVID-affected lung was found between the biological sexes (Figure 4). Within the 78 patients, a strong correlation was observed between the analysis of Expert 1 and computer analysis (R=0.86, P<2.2e−16, Figure 5). Consequently, the median percentage of COVID-19-affected areas in each expert evaluation score exhibited significant differences (P<0.05) from other scores, except for score classes 1 and 2, which were similar (Figure 6).
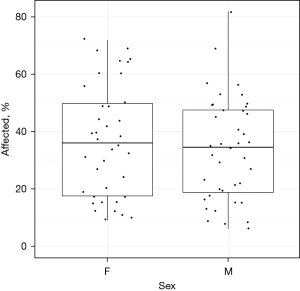
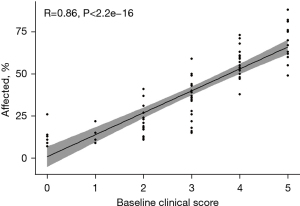
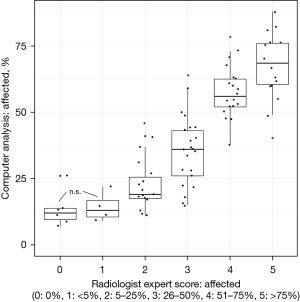
The correlation between expert and machine improved when using self-calibrated data [Akaike Information Criterion (AIC) Model 2, Bayesian Information Criterion (BIC) Model 2], as evidenced by the AIC and BIC values (AIC 1 vs. 2: 602.5 vs. 586.4, BIC 1 vs. 2: 609.5 vs. 593.5). The impact of data calibration is depicted in Figure 1.
With the participation of Expert 2, we observed a notable, statistically significant interobserver bias (Figure 7, stronger than anticipated), as evidenced by the Wilcoxon signed-rank test with continuity correction (P<0.05).
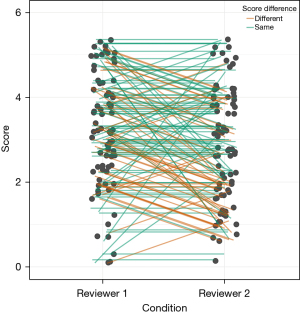
Total lung affection is shown in Table 1, and there were no significant differences between the right and left lungs.
Table 1
Structure | Mean | Median | SD | Q1 | Q3 | IQR | Comparison | P |
---|---|---|---|---|---|---|---|---|
Total lungs | 35.2 | 35 | 18.8 | 18 | 49 | 31 | – | – |
Right lung | 35.5 | 34 | 19.0 | 19 | 48 | 29 | RL vs. LL | n.s. |
Left lung | 35.9 | 36 | 19.0 | 18 | 49 | 31 | LL vs. RL | n.s. |
Right upper lobe | 30.6 | 26 | 19.7 | 12.5 | 42 | 29.5 | RUL vs. ML | n.s. |
Right middle lobe | 28.1 | 21 | 19.5 | 11 | 44 | 33 | ML vs. RUL | n.s. |
Right lower lobe | 44.8 | 47 | 22.4 | 27 | 60 | 33 | RLL vs. RUL | 0.00012 |
RLL vs. ML | 2.8e−05 | |||||||
Left upper lobe | 29.7 | 28 | 18.2 | 13 | 41 | 28 | LUL vs. LLL | 0.00011 |
Left lower lobe | 44.4 | 43 | 23.0 | 23 | 62 | 39 | LLL vs. LUL | 0.00011 |
Anterior | 36.0 | 35 | 19.9 | 19 | 51 | 32 | A vs. P | 0.00019 |
Posterior | 41.2 | 42 | 21.2 | 21 | 58 | 37 | P vs. A | 0.00019 |
Upper half | 31.4 | 29.5 | 18.6 | 14.2 | 44 | 29.8 | UH vs. LH | 0.02001 |
Lower half | 39.2 | 39.5 | 20.2 | 20.2 | 54 | 34 | LH vs. UH | 0.02001 |
Differences were evaluated with the Kruskal-Wallis test for group comparisons and the Wilcoxon rank sum test with Benjamini-Hochberg adjustment. Taking into account both lungs: A = anterior half; P = posterior half; UH = upper half; LH = lower half. COVID, coronavirus disease; SD, standard deviation; Q1, first quartile; Q3, third quartile; IQR, interquartile range; RL, right lung; LL, left lung; RUL, right upper lobe; ML, right middle lobe; RLL, right lower lobe; LUL, left upper lobe; LLL, left lower lobe; n.s., not significant.
In regard to the lobes (Table 1), a higher degree of infiltration and collapse was observed in the left lower lobe compared to the upper lobe (P<0.05). A similar pattern was found in the right lower lobes, with increased infiltration or collapse relative to the upper lobes (P<0.05). No significant difference was detected in the COVID-19-related involvement when comparing the left and right lower lobes to each other. The right middle lobes demonstrated lower involvement compared to the right lower lobes (P<0.05).
Upon examining the lung regions, significant differences in COVID-19 involvement were found when comparing the anterior and posterior halves of the lungs as the posterior regions showed higher COVID-19 involvement (Table 1). Comparing the upper and lower halves of both lungs, the lower halves of the lungs exhibited significantly greater COVID-19 involvement than the upper halves (Table 1).
It was particularly interesting that, according to computer analysis, patients who required admission to the ICU during their inpatient treatment demonstrated significantly higher COVID-19 lung involvement (Figure 8), as compared to patients who could remain in general wards. Patients with ≥40% COVID-19 involvement (n=31) were predominantly treated in intensive care (n=24, 77%).
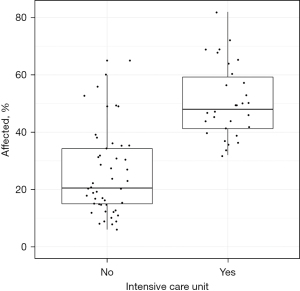
Discussion
The present study demonstrated the potential of 3D Slicer computer analysis as a reliable and reproducible tool for assessing lung involvement in patients with COVID-19. Our validation of computer analysis against expert radiological judgment revealed a high correlation between the two approaches in detecting COVID-19 affections. This finding is supported by a recent study (12) that suggests that computer analysis can effectively complement expert evaluation and contribute to more accurate and objective assessments of lung involvement.
In this study, the LungCTAnalyzer module uses a nnU-Net (13) TotalSegmentator AI tool (10), which enables autonomous computer analysis including lung lobes without human intervention, however, our results clearly show that a gold standard has not been defined. The use of AI-powered tools like the nnU-Net TotalSegmentator can provide several benefits, including reduced time spent on image analysis, increased consistency in assessments, and potentially improved patient outcomes due to faster and more accurate diagnosis. Furthermore, autonomous computer analysis could alleviate the workload of radiologists and healthcare professionals, especially during times of increased demand, such as the COVID-19 pandemic.
However, there are also potential dangers associated with relying solely on autonomous computer analysis, but the design of this study included AI only for the segmentation of lung masks and lung lobes.
The present study employs a two-way strategy for computer analysis of lung CT: first, anatomical structures are segmented using AI, and then the results are refined with conventional image processing methods, such as thresholding, smoothing, and volumetry. This approach is especially sound because autonomous deep-learning computer systems for detecting COVID-19 alone are on the horizon, but still have limitations (14,15).
Our results have shown that automatic calibration of CT data is an elegant, efficient and feasible solution that may be particularly valuable for analyzing heterogeneous or artifact-laden datasets. The time required to perform a complete segmentation using 3D Slicer compared to manual delineation times (5 vs. 2–3 minutes) still favors the human observer, however, it has to be noted that LungCTAnalyzer as well as its AI tool has not been optimized for speed yet. This, in combination with better hardware, will certainly reduce program execution times. In addition, the automated analysis could seamlessly be integrated into the data transfer process from the scanner, ensuring that it will have been automatically processed by the time the radiologist is ready to review the case. Thus, in a practical setting, any potential delay becomes negligible.
Our results also highlighted the anatomical and regional differences in COVID-19 lung affections. We found a higher degree of infiltration and collapse in the lower lobes, the lower halves of the lungs, and the posterior lungs. These differences provide valuable insights into the distribution and extent of lung involvement in advanced COVID-19.
A significant insight from our research is the capability of the 3D Slicer computer analysis method to provide indicators for potential ICU treatment needs. Our data showed that patients with more pronounced lung involvement (>40%) were predominantly treated in intensive care settings. While this underscores the utility of gauging lung involvement for resource allocation, especially in a pandemic scenario with constrained ICU resources, it’s imperative to acknowledge that ICU admission decisions are multi-faceted and not solely predicated on CT lung involvement.
However, it is important to note that there were seven patients with high pulmonary affections who did not require ICU admission. This phenomenon has been a common observation during the COVID-19 pandemic and highlights the multifactorial nature of the disease, in which deterioration of lung function may occur very late despite already significant pulmonary radiological affections (16,17). This observation emphasizes the need for further investigation to better understand the factors that may influence ICU admission requirements, such as comorbidities (18), patient age (19), and overall clinical presentation (20).
The limitations of the study should also be acknowledged. Our sample size was relatively small, which may limit the generalizability of our findings. Moreover, the study design was retrospective, and prospective studies with larger sample sizes are needed to confirm and expand upon these observations. Additionally, while the high correlation between expert and computer analysis is promising, further research is required to refine and optimize the 3D Slicer computer analysis tool, including the nnU-Net TotalSegmentator AI component, and validate its utility in different clinical settings. In addition, our method for calibration of the CT HU values could be improved, ideally through the use of calibration phantoms as part of the scanning protocol for any future prospective study. Similarly, our use of hard threshold values to define the areas of emphysema and otherwise characterize features could potentially be improved through the use of a soft threshold technique, but it is questionable if a computer program will ever be able to mitigate these different nuances.
A particular constraint of the initial study is that it included only a single radiological expert as a human control. Nevertheless, this limitation was an inherent component of the design in the preceding study. To address this, we introduced an additional reviewer who utilized the same scoring system (refer to Figure 8). With the participation of two radiological experts, a notable, statistically significant interobserver bias was detected. This finding underscores the necessity for an automated, reproducible computer evaluation to mitigate such biases.
Another limitation of our study pertains to the variable “affected lung involvement”, whose role as an independent predictor for ICU admission remains to be proven. In a more comprehensive study, this variable would ideally be incorporated into a multivariate regression analysis. This analysis would assess if “affected lung involvement” can hold its significance against well-established confounders, such as age and comorbidities. Unfortunately, the dataset used in our study does not include these variables, preventing us from performing this analysis at this time. Future prospective studies incorporating these data points will be necessary to conduct a more robust assessment.
Lastly, patterns, GGO, or “tinted signs” (20) may not be correctly detected by the threshold-based computer algorithms yet and would require further development of AI detection methods.
Despite these limitations, our study contributes valuable insights to the existing literature on lung involvement in COVID-19 and highlights the potential of 3D Slicer computer analysis as a reliable, reproducible, and complementary tool for expert radiological evaluation. The anatomical and regional differences in lung affections, as well as the potential to predict ICU treatment requirements, have important implications for clinical practice and resource allocation during a pandemic.
The availability of automatic volumetry of COVID affections could also be extremely helpful during follow-up.
In this discussion of a science paper, it is important to emphasize that the methodology employed, using the 3D Slicer computer analysis, has broader applications beyond just COVID-19. This work demonstrates that a previously challenging image analysis problem, detailed segmentation of lung anatomy in patients with severe disease, is now routinely solvable using freely available software with reliability suitable to explore novel anatomical correlates of disease severity. The paper has effectively showcased how this approach can be used for the quantitative assessment of lung pathologies in the context of COVID-19. However, its utility extends to a wide range of pulmonary conditions, including fibrosis, emphysema, and other infectious lung diseases.
The versatility and potential of the 3D Slicer-based LungCTAnalyzer, alongside the nnU-Net TotalSegmentator AI component, make it a promising tool for future research and clinical practice, always keeping in mind that computer software will probably be an adjunct not a substitute to humans. The fact that the software is open-source and freely available to researchers worldwide further bolsters its potential impact, as it encourages collaboration and continuous improvement within the scientific community.
As our methodology has proven effective in the present case study of COVID-19 and has also been used in the quantification of lung changes in chest CT of patients with mutations of the novel coronavirus 2 with success (21), future research should explore its applications in the context of other lung pathologies, both infectious and non-infectious. This would not only provide additional insights into the value and limitations of this approach but also help optimize patient care and outcomes across a variety of pulmonary conditions. By doing so, the scientific community can work together to refine and expand the use of AI-powered tools, ensuring that they complement and enhance expert human judgment in the assessment and management of lung diseases.
Conclusions
In conclusion, our research illustrates the pattern and degree of lung involvement in COVID-19 patients. It suggests a potential correlation between lung involvement and the consideration for ICU admission, without asserting a direct causal link. Notably, computer-assisted detection of COVID-19 manifestations in the lung correlated highly with evaluations made by radiological experts. This indicates that computer-based analysis might offer valuable insights for assessing lung involvements in clinical settings. These preliminary findings warrant further exploration, ideally with larger cohorts, to validate and delve deeper into the observations. In the broader scope, enhancing our grasp of lung involvement not only in COVID-19, paired with refining diagnostic tools, has the potential to elevate patient care, follow up and outcomes.
Acknowledgments
As the primary author is a non-native English speaker, he utilized the ChatGPT-GPT-4 model (LLM, a Large Language Model is an advanced machine learning model that is capable of processing vast amounts of language data and generating human-like responses to queries) as an editing tool during the creation of this article to: review and amend spelling and grammatical errors; ensure linguistic consistency and coherence; test and fine-tune the wording of the article; standardize the format of the Annotated Bibliography.
Funding: This research was supported by
Footnote
Reporting Checklist: The authors have completed the MDAR reporting checklist. Available at https://jtd.amegroups.com/article/view/10.21037/jtd-23-1150/rc
Peer Review File: Available at https://jtd.amegroups.com/article/view/10.21037/jtd-23-1150/prf
Conflicts of Interest: All authors have completed the ICMJE uniform disclosure form (available at https://jtd.amegroups.com/article/view/10.21037/jtd-23-1150/coif). R.S.J.E. reports grants from the National Heart, Lung, and Blood Institute (NHLBI) for the present manuscript, with no time limit specified for this item; contract with Lung Biotechnology and Insmed to serve as Image Core for a study; sponsored research agreement with Boehringer Ingelheim; consulting fees from Leuko Labs and Mount Sinai; payment/honoraria Chiesi; patent pending in the area of lung cancer risk assessment using machine learning technology; he is co-founder and stockholder of Quantitative Imaging Solutions, a company specializing in imaging analytics in the lung cancer space. S.P. is a full-time employee of Isomics, Inc. and participated in the research as part of his normal work activities. The other authors have no conflicts of interest to declare.
Ethical Statement: The authors are accountable for all aspects of the work in ensuring that questions related to the accuracy or integrity of any part of the work are appropriately investigated and resolved. The study was conducted according to the guidelines of the Declaration of Helsinki (as revised in 2013).
Open Access Statement: This is an Open Access article distributed in accordance with the Creative Commons Attribution-NonCommercial-NoDerivs 4.0 International License (CC BY-NC-ND 4.0), which permits the non-commercial replication and distribution of the article with the strict proviso that no changes or edits are made and the original work is properly cited (including links to both the formal publication through the relevant DOI and the license). See: https://creativecommons.org/licenses/by-nc-nd/4.0/.
References
- Li Q, Guan X, Wu P, et al. Early Transmission Dynamics in Wuhan, China, of Novel Coronavirus-Infected Pneumonia. N Engl J Med 2020;382:1199-207. [Crossref] [PubMed]
- Wu F, Zhao S, Yu B, et al. A new coronavirus associated with human respiratory disease in China. Nature 2020;579:265-9. [Crossref] [PubMed]
- Dong E, Du H, Gardner L. An interactive web-based dashboard to track COVID-19 in real time. Lancet Infect Dis 2020;20:533-4. [Crossref] [PubMed]
- Ai T, Yang Z, Hou H, et al. Correlation of Chest CT and RT-PCR Testing for Coronavirus Disease 2019 (COVID-19) in China: A Report of 1014 Cases. Radiology 2020;296:E32-40. [Crossref] [PubMed]
- Fang Y, Zhang H, Xie J, et al. Sensitivity of Chest CT for COVID-19: Comparison to RT-PCR. Radiology 2020;296:E115-7. [Crossref] [PubMed]
- Pan F, Ye T, Sun P, et al. Time Course of Lung Changes at Chest CT during Recovery from Coronavirus Disease 2019 (COVID-19). Radiology 2020;295:715-21. [Crossref] [PubMed]
- Bumm R, Lasso A. Lung CT Analyzer. Lung CT Analyzer on GitHub. Available online: https://github.com/rbumm/SlicerLungCTAnalyzer
- Kikinis R, Pieper SD, Vosburgh KG. 3D Slicer: A Platform for Subject-Specific Image Analysis, Visualization, and Clinical Support. In: Jolesz FA, editor. Intraoperative Imaging and Image-Guided Therapy. New York, NY: Springer; 2014:277-89.
- Zaffino P, Marzullo A, Moccia S, et al. An Open-Source COVID-19 CT Dataset with Automatic Lung Tissue Classification for Radiomics. Bioengineering (Basel) 2021;8:26. [Crossref] [PubMed]
- Wasserthal J, Breit HC, Meyer MT, et al. TotalSegmentator: Robust Segmentation of 104 Anatomic Structures in CT Images. Radiol Artif Intell 2023;5:e230024. [Crossref] [PubMed]
- Lasso A. TotalSegmentator extension for 3D Slicer [Internet]. 2023 [cited 2023 May 6]. Available online: https://github.com/lassoan/SlicerTotalSegmentator
- Risoli C, Nicolò M, Colombi D, et al. Different Lung Parenchyma Quantification Using Dissimilar Segmentation Software: A Multi-Center Study for COVID-19 Patients. Diagnostics (Basel) 2022;12:1501. [Crossref] [PubMed]
- Isensee F, Jaeger PF, Kohl SAA, et al. nnU-Net: a self-configuring method for deep learning-based biomedical image segmentation. Nat Methods 2021;18:203-11. [Crossref] [PubMed]
- Aslani S, Jacob J. Utilisation of deep learning for COVID-19 diagnosis. Clin Radiol 2023;78:150-7. [Crossref] [PubMed]
- Li Z, Zhong Z, Li Y, et al. From community-acquired pneumonia to COVID-19: a deep learning-based method for quantitative analysis of COVID-19 on thick-section CT scans. Eur Radiol 2020;30:6828-37. [Crossref] [PubMed]
- Zhou F, Yu T, Du R, et al. Clinical course and risk factors for mortality of adult inpatients with COVID-19 in Wuhan, China: a retrospective cohort study. Lancet 2020;395:1054-62. [Crossref] [PubMed]
- Guan WJ, Ni ZY, Hu Y, et al. Clinical Characteristics of Coronavirus Disease 2019 in China. N Engl J Med 2020;382:1708-20. [Crossref] [PubMed]
- Yang X, Yu Y, Xu J, et al. Clinical course and outcomes of critically ill patients with SARS-CoV-2 pneumonia in Wuhan, China: a single-centered, retrospective, observational study. Lancet Respir Med 2020;8:475-81. [Crossref] [PubMed]
- Dowd JB, Andriano L, Brazel DM, et al. Demographic science aids in understanding the spread and fatality rates of COVID-19. Proc Natl Acad Sci U S A 2020;117:9696-8. [Crossref] [PubMed]
- Petrilli CM, Jones SA, Yang J, et al. Factors associated with hospital admission and critical illness among 5279 people with coronavirus disease 2019 in New York City: prospective cohort study. BMJ 2020;369:m1966. [Crossref] [PubMed]
- Kottlors J, Fervers P, Geißen S, et al. Morphological appearance of the B.1.1.7 mutation of the novel coronavirus 2 (SARS-CoV-2) in chest CT. Quant Imaging Med Surg 2023;13:1058-70. [Crossref] [PubMed]