Identification of prognostic biomarkers of smoking-related lung cancer
Highlight box
Key findings
• SCGB3A1 exhibits a positive correlation with patient prognosis in lung cancer.
What is known and what is new?
• Smoking is one of the causes of lung cancer, but it is not the initial carcinogenic factor. The molecular mechanisms leading to lung cancer may differ depending on whether it is caused by smoking.
• We identified three genes, BPIFA1, SLPI, and SCGB3A1, as being associated with lung cancer in smokers, with SCGB3A1 demonstrating a positive correlation with patient prognosis.
What is the implication, and what should change now?
• Our study demonstrated the association of BPIFA1, SLPI, and SCGB3A1 with lung cancer in smokers, with SCGB3A1 revealing a notable correlation with patient prognosis. These findings offer potential new targets for the treatment of lung cancer.
• Therefore, we will conduct clinical trials to further verify whether the differential expression of SCGB3A1 impacts the prognosis of lung cancer patients. Concurrently, foundational research will be undertaken to elucidate how SCGB3A1 modulates patient prognosis, aiming to uncover the mechanisms through which it extends patient survival.
Introduction
Lung cancer is one of the most severe malignant tumors affecting humans. According to the latest World Cancer Report [2022], lung cancer ranks first in both incidence and mortality among males and second in incidence and first in mortality among females (1,2). Based on the different cell types that form lung cancer, it can be divided into small-cell lung cancer (SCLC) (approximately 15% of cases) and non-small cell lung cancer (NSCLC) (about 85% of cases). NSCLC can further be classified into three types: lung adenocarcinoma (LUAD) (30–40% of cases), lung squamous cell carcinoma (LUSC) (20–25% of cases), and large-cell carcinoma (LCC) (15–20% of cases) (3). Since early-stage lung cancer often lacks obvious symptoms, about 40% of patients with NSCLC are diagnosed with metastasis during disease progression (4). Moreover, early diagnosis and effective prognostic treatment measures for lung cancer are still limited, leading to a 5-year survival rate of less than 15% for these patients (5,6). Therefore, further investigation into the mechanisms of lung cancer formation and its impact on prognosis is needed.
The occurrence of lung cancer is a complex process involving multiple factors and stages. Among these, smoking is one of the causes of lung cancer, but it is not the initial carcinogenic factor. The molecular mechanisms leading to lung cancer may differ depending on whether it is caused by smoking (7-9). Studies have found that nicotine, a major component of tobacco, can affect the expression of the Bcl-2 family proteins in lung cancer cells, promoting cancer cell growth and enhancing drug resistance (10,11). Tobacco activates the Notch signaling pathway to induce lung cancer and regulates cell apoptosis by increasing survivin expression, thereby promoting the malignant transformation of bronchial epithelial cells (12). Vellichirammal et al. reported a positive correlation between smoking and fusion frequency in lung adenocarcinoma and found that as a fusion gene associated with cigarette smoke exposure, downregulation of the P53 pathway resulted in higher gene fusion formation in lung adenocarcinoma (13). Furthermore, smoking generates carcinogens during the combustion process, damaging bronchial epithelial cells through different mechanisms and activating oncogenes, leading to mutations and inactivating tumor-suppressor genes, ultimately causing carcinogenesis (14,15).
Research has shown that compared to normal tissues, the genome of cancer tissue undergoes significant changes, such as gene structural abnormalities, including gene copy number variations, gene expression profiles changes and epigenetic modifications (16). Moreover, different types of cancer have various genomic alterations, which are related to the patient’s genetic expression and inducing factors. In clinical practice, gene mutations are used for cancer typing and treatment, allowing for personalized diagnosis, treatment, and prevention for different patients (17,18). Smoking is a factor in patients with lung cancer, and various genetic changes may also occur within their cancer tissues. Identifying unique differential genes for patients with smoking-related lung cancer can provide targeted guidance for clinical diagnosis, treatment, and prevention. Advances in gene sequencing and bioinformatics have made this approach possible. The specific mechanisms through which smoking induces and regulates lung cancer remain unclear, and there is limited research on the relationship between related genes and the prognosis of patients with lung cancer who smoke. Smoking is one of the causes of lung cancer. Therefore, there is an urgent need to provide new theoretical basis and potential therapeutic targets for the formation mechanism of smoking-related lung cancer.
In this study, we obtained gene chip datasets for patients with lung cancer who smoke from the Gene Expression Omnibus (GEO) (https://www.ncbi.nlm.nih.gov/geo/) and The Cancer Genome Atlas (TCGA) (https://portal.gdc.cancer.gov) databases, analyzed the differential gene expression in their tissues, and determined the correlation of these selected genes with clinical factors and their prognostic analysis. The aim of this study is to provide new theoretical evidence and potential therapeutic targets for the mechanisms of smoking-related lung cancer formation. We present this article in accordance with the REMARK reporting checklist (available at https://jtd.amegroups.com/article/view/10.21037/jtd-23-1890/rc).
Methods
Dataset selection
The microarray data and corresponding clinical data of smokers and nonsmokers with lung cancer were obtained from the GEO and TCGA databases. The messenger RNA (mRNA) expression profile data from the GSE12428 dataset were downloaded from the GEO database. GSE12428 contains mRNA expression level data of 28 cases (12 smokers and 16 ex-smokers) of cartilaginous bronchial tissue and 35 cases (19 current smokers and 16 ex-smokers) of primary lung cancer tissue samples, totaling 63 lung cancer and normal tissue pairs. The smokers are patients who were still smoking when they were diagnosed with lung cancer. Ex-smokers have a history of smoking but had quit smoking when they were diagnosed with lung cancer. TCGA dataset was analyzed from TCGA and includes 483 cases of LUAD and 347 cases of normal tissue as well as 486 cases of LUSC and 338 cases of normal tissue. The study was conducted in accordance with the Declaration of Helsinki (as revised in 2013).
Identification of differentially expressed genes
The linear model for microarray data in the “limma” package in R software (version 3.40.6, The R Foundation of Statistical Computing, Vienna, Austria) was used to analyze differential genes between samples. The criteria for selecting differentially expressed genes were an adjusted P value ≤0.05 and |log2(fold change)| ≥2. The results were visualized with volcano plots and heatmaps, and the common differentially expressed genes among datasets (GSE12428) were selected for further study. The clinical information about the sample is in the https://cdn.amegroups.cn/static/public/jtd-23-1890-1.xlsx.
Enrichment analysis of differentially expressed genes
Enrichment analysis of differentially expressed genes among smokers and nonsmokers with LUAD was performed using the online Database for Annotation, Visualization and Integrated Discovery (DAVID) 6.8 (https://david.ncifcrf.gov). Gene Ontology (GO) gene function analysis was conducted based on human genes. The differentially expressed mRNAs related to smoking-related lung cancer were analyzed using Kyoto Encyclopedia of Genes and Genomes (KEGG) pathway analysis to identify the biological pathways enriched by the differentially expressed genes. P value ≤0.05 and |log2(fold change)| ≥2 indicated statistical significance.
Expression levels of differentially expressed genes under different clinical factors
Gene Expression Profiling Interactive Analysis (GEPIA) (http://gepia.cancer-pku.cn/index.html) online data analysis was used to compare the differential gene mRNA expression levels obtained from lung cancer (LUAD and LUSC) tissues and normal tissues. P value ≤0.05 indicated statistical significance. The same samples were grouped according to tumor stage (stages I, II, III, and IV), and the expression levels of the selected genes during different stages of the tumor were analyzed. P value ≤0.05 indicated statistical significance.
Prognostic analysis of differentially expressed genes
The Kaplan-Meier plotter (https://kmplot.com/analysis/) was used for online analysis of the relationship between the differential gene mRNA expression levels obtained from lung cancer (LUAD and LUSC) tissues and normal tissues and survival data. The Kaplan-Meier plotter includes multiple GEO datasets, which can identify and validate differentially expressed genes, including mRNA and microRNA (miRNA) that can significantly affect prognosis.
Statistical analysis
R software was used for statistical analysis, and the threshold for identifying differentially expressed genes was set at P≤0.05 and |log2(fold change)| ≥2. The Kaplan-Meier plotter was used for testing the survival data. P value ≤0.05 indicated statistical significance.
Results
Differential gene expression analysis and screening
In the GSE12428 dataset, we used the R software package “limma” (version 3.40.6) to perform differential expression analysis based on the screening criteria. The samples were grouped into lung cancer tissues and normal tissues (available online: https://cdn.amegroups.cn/static/public/jtd-23-1890-2.xlsx), and the analysis revealed 225 upregulated genes and 565 downregulated genes in lung cancer tissues compared to normal tissues (Figure 1A). The top 10 upregulated and downregulated mRNA differences are shown in the heatmap (Figure 1B). Further grouping based on smoking status in the GSE12428 dataset and differential expression analysis according to the screening criteria indicated one upregulated gene and four downregulated genes in smokers (Figure 1C) (available online: https://cdn.amegroups.cn/static/public/jtd-23-1890-3.xlsx). The heatmap in Figure 1D depicts the differential expression levels of mRNA in the upregulated and downregulated genes in smokers. We then intersected the upregulated mRNA in lung cancer tumor tissues with the upregulated mRNA in smoking patients and found no intersecting mRNA (Figure 1E). However, when we intersected the downregulated mRNA in lung cancer tumor tissues with the downregulated mRNA in smoking patients, four mRNAs were downregulated in both smoking and nonsmoking patients with lung cancer: CSH1, BPIFA1, SLPI, and SCGB3A1 (Figure 1F).
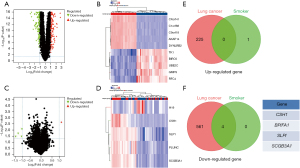
Functional analysis of genes
GO enrichment analysis was performed for the selected genes, and significantly enriched GO annotations (P≤0.05) are presented in the bar chart in Figure 2A. The results indicated that the differentially expressed genes were enriched in molecular functions such as antibacterial humoral response, antimicrobial humoral response, negative regulation of multiorganism process, regulation of symbiosis, response to external stimulus, humoral immune response, defense response to bacterium, and regulation of multiorganism process. The cellular components included extracellular space, extracellular region part, and extracellular region. The enriched biological pathways included antibiotic function and antimicrobial function (Table 1). KEGG pathway enrichment analysis of the selected genes revealed that they were involved in nine significantly enriched pathways, including innate immune response, innate immunity, immunity, antibacterial humoral response, extracellular region, extracellular space, secretion, antibiotic process, and antimicrobial process, as shown in the bubble chart in Figure 2B (the colors of the circles represent the correlation between the genes and pathways, and the size of the circles represents the enrichment multiple). The relevant enriched pathways are listed in Table 2.
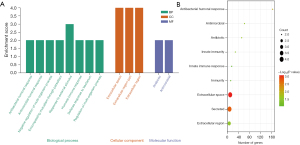
Table 1
Term | Count | Percent (%) | P value | FDR |
---|---|---|---|---|
Biological process | ||||
Antibacterial humoral response | 2 | 50 | 0.011 | 0.05 |
Antimicrobial humoral response | 2 | 50 | 0.025 | 0.05 |
Negative regulation of multi-organism process | 2 | 50 | 0.031 | 0.05 |
Regulation of symbiosis | 2 | 50 | 0.048 | 0.05 |
Response to external stimulus | 3 | 75 | 0.046 | 0.05 |
Humoral immune response | 2 | 50 | 0.048 | 0.05 |
Defense response to bacterium | 2 | 50 | 0.042 | 0.05 |
Regulation of multiorganism process | 2 | 50 | 0.07 | 0.05 |
Cellular component | ||||
Extracellular space | 4 | 100 | 0.001 | 0.039 |
Extracellular region part | 4 | 100 | 0.0072 | 0.14 |
Extracellular region | 4 | 100 | 0.013 | 0.16 |
Molecular function | ||||
Antibiotic function | 2 | 50 | 0.024 | 0.086 |
Antimicrobial function | 2 | 50 | 0.029 | 0.086 |
FDR, false-discovery rate.
Table 2
Term | Enrichment | Count | Percent (%) | P value | FDR |
---|---|---|---|---|---|
Innate immune response | 16.127 | 2 | 50 | 0.05 | 1 |
Innate immunity | 28.982 | 2 | 50 | 0.035 | 0.069 |
Immunity | 12.416 | 2 | 50 | 0.081 | 0.081 |
Antibacterial humoral response | 163.186 | 2 | 50 | 9.2E−4 | 0.229 |
Extracellular region | 7.219 | 3 | 75 | 0.03 | 0.121 |
Extracellular space | 10.574 | 4 | 100 | 8.4E−5 | 6.8E−4 |
Secretion | 8.263 | 4 | 100 | 1.8E−4 | 1.7E−3 |
Antibiotic function | 52.031 | 2 | 50 | 0.024 | 0.086 |
Antimicrobial function | 61.995 | 2 | 50 | 0.029 | 0.086 |
FDR, false-discovery rate.
Expression of genes in lung cancer tissues and normal tissues
To validate the expression levels of the selected genes (CSH1, BPIFA1, SLPI, and SCGB3A1) in lung cancer, we performed online analysis using TCGA database. The expression levels of these genes were analyzed in 483 cases of LUAD and 347 cases of normal tissues as well as 486 cases of LUSC and 338 cases of normal tissues. The results showed that CSH1 and BPIFA1 had lower expression levels of LUAD and LUSC compared with normal tissues (Figure 3A,3B). SLPI was significantly downregulated trend in both LUAD and LUSC, and the difference was statistically significant (P≤0.05) (Figure 3C). SCGB3A1 was downregulated in both LUAD and LUSC tissues compared with normal tissues, with a statistically significant difference in LUSC (P≤0.05) (Figure 3D). Overall, BPIFA1, SLPI, and SCGB3A1 were downregulated in lung cancer tissues, which was consistent with the analysis of the GSE12428 dataset.
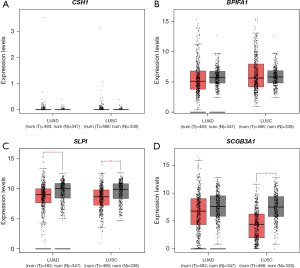
Correlation of genes with tumor stage
Using TCGA database, we further analyzed the expression levels of the four genes (CSH1, BPIFA1, SLPI, and SCGB3A1) in lung cancer tissues according to tumor stage. The results showed that CSH1 had low expression levels in tissues from all stages of lung cancer, making it difficult to draw comparisons (Figure 4A). BPIFA1 had higher expression in stage III or IV lung cancer tissues than in stage I or II tissues (Figure 4B). SLPI had a lower expression in stage II lung cancer tissues than in stage I, III, and IV tumor tissues, but the difference was not significant (Figure 4C). SCGB3A1 had a significantly higher expression in stage I tumor tissues than in stage II, III, and IV tumor tissues, indicating a decreased expression with tumor progression (Figure 4D). These results suggest that SCGB3A1 could be one of the markers for lung cancer staging.
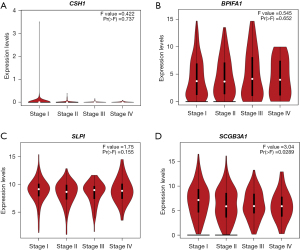
Kaplan-Meier plotter survival analysis
The Kaplan-Meier plotter was used to evaluate the effects of CSH1, BPIFA1, SLPI, and SCGB3A1 on overall survival in smokers with LUAD. As shown in Figure 5, high CSH1, SLPI, and SCGB3A1 expression was associated with improved patient survival rates, indicating that the high expression of these three genes was related to improved overall survival. However, CSH1 and SLPI did not show significant differences (P>0.05), while SCGB3A1 did show a significant difference (P≤0.05). On the other hand, low expression of BPIFA1 was associated with increased patient survival, but the increase was not significant (P>0.05). Overall, high expression of SCGB3A1 could indicate a better prognosis, as an increase in SCGB3A1 mRNA expression was associated with improved patient outcomes.

Discussion
Lung cancer is one of most common malignant tumors, but the molecular mechanisms related to its occurrence are diverse. Smoking may increase lung cancer incidence and mortality (19,20). Even for the same type of lung cancer tissue, tumors may have different molecular mechanisms based on whether smoking is a factor. Studies have shown that cigarette smoke can stimulate lung epithelial and cancer cells by activating myristoylated alanine-rich C kinase substrate (MARCKS) and subsequently the nuclear factor κB (NF-κB) signaling pathway. Smoking induces phosphorylation of MARCKS (p-MARCKS), which is positively correlated with the phosphorylation of NF-κB (p-65), leading to the upregulation of proinflammatory cytokines and promoting epithelial-mesenchymal transition and stem cell properties (21,22). Although the relationship between smoking and lung cancer is well known, much work remains to fully elucidate the risk factors associated with lung cancer among smokers and non-smokers (23).
Many diseases’ physiological and pathological processes can be discerned at the mRNA and protein levels. With the rapid development and application of high-throughput sequencing technology, bioinformatics analysis of molecular biological functions and disease processes has become increasingly insightful (24,25). Zhang et al. identified MYH7 as a novel biomarker for heavy smoking-related LUAD, and it is significantly associated with the prognosis of lung cancer and closely related to the survival rate of patients with this disease (26). Zhang et al. found that compared with NSCLC patients who smoked, non-smoking patients were more sensitive to EGFR tyrosine kinase inhibitors and had better prognosis. In addition, it was found that non-smoking patients had a higher maximum standardized uptake value of primary tumors and a lower incidence of EGFR mutations (27).
Numerous biomedical databases support data mining and bioinformatics analysis, extracting potentially helpful data and providing valuable information for clinical and disease mechanism research. The GEO database and TCGA database are popular and widely used biomedical information repositories, covering nearly all genomics, transcriptomics, proteomics, epigenetics, and other omics data related to organs, tissues, and cells. They are the largest and most comprehensive tumor gene information databases globally (28,29) and thus have greatly improved the early diagnosis and prevention of cancer by providing support for the in-depth understanding of cancer’s pathogenic factors and mechanisms from molecular and genetic perspectives (30,31). In recent years, researchers have integrated and analyzed data to uncover the pathogenic mechanisms of related tumors. Through TCGA and GEO database analysis, Jin and Yang identified hub genes (SPP1, POSTN, COL1A2, FN1, IGFBP3, APP, MMP3, MMP13, CXCL8, and CXCL12) that could serve as potential diagnostic markers for head and neck squamous cell carcinoma (HNSCC) (32). The relative expression of FN1, APP, SPP1, and POSTN might be associated with the prognosis of patients with HNSCC. Through bioinformatics analysis, Zhao et al. found three genes (COL1A1, PLEK2, and GPX3) to be related to the prognosis of LUAD. The risk scores of patients with LUAD were significantly correlated with survival rates in three independent GEO datasets and the LUAD TCGA dataset (33). There is still controversy over whether smoking induces lung cancer, and there is limited research on the relationship between related genes and the prognosis of patients with lung cancer who smoke.
In this study, lung cancer-related mRNA expression profile datasets were analyzed through bioinformatics analysis and integration of the GEO database and multiple online databases. The results identified 790 genes with statistically significant differences in cancer tissue, including 225 upregulated genes and 565 downregulated genes. Among the smokers with lung cancer, four genes were downregulated: CSH1, BPIFA1, SLPI, and SCGB3A1. GO and KEGG pathway enrichment analysis of the selected genes revealed that they are primarily associated with antimicrobial responses, humoral immune responses, and responses to external stimuli. Among these genes, BPIFA1, SLPI, and SCGB3A1 showed low expression levels in lung cancer tissue, with SCGB3A1 exhibiting significant differences. High expression of SCGB3A1 was associated with a favorable prognosis for smokers with lung cancer, suggesting SCGB3A1 may be one of the molecular markers related to the pathogenesis and prognosis of smoking-related lung cancer.
SCGB3A1, also known as secretoglobin family 3A member 1, is distributed in the extracellular region and highly expressed in the lungs, regulating cell growth (34,35). Mazumdar et al. demonstrated that SCGB3A1 inhibits tumor growth in NSCLC by targeting hypoxia-inducible factor 2α (HIF-2α) and inhibiting the Ak strain transforming (AKT) signaling pathway (36). The direct correlation between SCGB3A1 and HIF-2α was validated in approximately 70% of patients with NSCLC in Mazumdar et al.’s study, suggesting that SCGB3A1 functions as a tumor-suppressor gene (36). Additionally, Palalı et al. found that SCGB3A1 has a relieving protective effect on nasal polyposis (37). Li et al. found that SCGB3A1 expression is correlated positively with prognosis and promotes antitumor immunity in LUAD, which may serve as immune-related therapeutic target for LUAD (38). In our study, smokers with lung cancer who had a high expression of SCGB3A1 had a favorable prognosis, possibly because smoking stimulates the nasal, respiratory, and lung tissues, upregulating SCGB3A1 expression, which inhibits tumor progression or deterioration. Therefore, it may be predicted by judging the expression level of SSCGB3A1 to prognostic characteristics of smoking-related lung cancer. SCGB3A1 is essential in the pathogenesis and prognosis of smoking-related lung cancer. Certainly, key molecules that can be used as therapeutic targets for lung cancer can be found through gene and molecular target research, immunotherapy, clinical trials and drug development in future research.
In light of these findings, some limitations to this study should also be noted. First, there was lack of clinical validation. Moreover, there is need for more data from basic studies to elucidate the regulatory mechanisms by which SCGB3A1 prolongs patient prognosis. In subsequent research, we will conduct clinical experiment to verify whether the differential expression of SCGB3A1 affects the prognosis of patients with lung cancer. We will also conduct basic studies to identify the regulatory mechanism of SCGB3A1 in prolonging the prognosis of patients.
Conclusions
In conclusion, by exploring the pathogenic mechanisms of smoking-related lung cancer through bioinformatics, we identified the expression of SCGB3A1 as being associated with the clinical staging and prognosis of patients, supporting its potential as a biomarker for the prognosis of smokers with lung cancer. It may play a significant role in the occurrence and development of tobacco-related lung cancer and may represent a potential new target in lung cancer treatment.
Acknowledgments
We thank the public repositories for providing data from the GSE12428 dataset.
Funding: None.
Footnote
Reporting Checklist: The authors have completed the REMARK reporting checklist. Available at https://jtd.amegroups.com/article/view/10.21037/jtd-23-1890/rc
Peer Review File: Available at https://jtd.amegroups.com/article/view/10.21037/jtd-23-1890/prf
Conflicts of Interest: All authors have completed the ICMJE uniform disclosure form (available at https://jtd.amegroups.com/article/view/10.21037/jtd-23-1890/coif). The authors have no conflicts of interest to declare.
Ethical Statement: The authors are accountable for all aspects of the work in ensuring that questions related to the accuracy or integrity of any part of the work are appropriately investigated and resolved. The study was conducted in accordance with the Declaration of Helsinki (as revised in 2013).
Open Access Statement: This is an Open Access article distributed in accordance with the Creative Commons Attribution-NonCommercial-NoDerivs 4.0 International License (CC BY-NC-ND 4.0), which permits the non-commercial replication and distribution of the article with the strict proviso that no changes or edits are made and the original work is properly cited (including links to both the formal publication through the relevant DOI and the license). See: https://creativecommons.org/licenses/by-nc-nd/4.0/.
References
- Siegel RL, Miller KD, Wagle NS, et al. Cancer statistics, 2023. CA Cancer J Clin 2023;73:17-48. [Crossref] [PubMed]
- Miller KD, Nogueira L, Devasia T, et al. Cancer treatment and survivorship statistics, 2022. CA Cancer J Clin 2022;72:409-36. [Crossref] [PubMed]
- Brambilla E, Travis WD, Colby TV, et al. The new World Health Organization classification of lung tumours. Eur Respir J 2001;18:1059-68. [Crossref] [PubMed]
- El Rassy E, Botticella A, Kattan J, et al. Non-small cell lung cancer brain metastases and the immune system: From brain metastases development to treatment. Cancer Treat Rev 2018;68:69-79. [Crossref] [PubMed]
- Wang Z, Xu Y, Tian L, et al. A Multi-Task Convolutional Neural Network for Lesion Region Segmentation and Classification of Non-Small Cell Lung Carcinoma. Diagnostics (Basel) 2022;12:1849. [Crossref] [PubMed]
- Fassina A, Cappellesso R, Fassan M. Classification of non-small cell lung carcinoma in transthoracic needle specimens using microRNA expression profiling. Chest 2011;140:1305-11. [Crossref] [PubMed]
- Hou W, Hu S, Li C, et al. Cigarette Smoke Induced Lung Barrier Dysfunction, EMT, and Tissue Remodeling: A Possible Link between COPD and Lung Cancer. Biomed Res Int 2019;2019:2025636. [Crossref] [PubMed]
- Gibbons DL, Byers LA, Kurie JM. Smoking, p53 mutation, and lung cancer. Mol Cancer Res 2014;12:3-13. [Crossref] [PubMed]
- Liu X, Lin XJ, Wang CP, et al. Association between smoking and p53 mutation in lung cancer: a meta-analysis. Clin Oncol (R Coll Radiol) 2014;26:18-24. [Crossref] [PubMed]
- Shrestha T, Takahashi T, Li C, et al. Nicotine-induced upregulation of miR-132-5p enhances cell survival in PC12 cells by targeting the anti-apoptotic protein Bcl-2. Neurol Res 2020;42:405-14. [Crossref] [PubMed]
- Mosadegh M, Hasanzadeh S, Razi M. Nicotine-induced damages in testicular tissue of rats; evidences for bcl-2, p53 and caspase-3 expression. Iran J Basic Med Sci 2017;20:199-208. [Crossref] [PubMed]
- Yesupatham ST, Dayanand CD, Azeem Mohiyuddin SM, et al. An Insight into Survivin in Relevance to Hematological, Biochemical and Genetic Characteristics in Tobacco Chewers with Oral Squamous Cell Carcinoma. Cells 2023;12:1444. [Crossref] [PubMed]
- Vellichirammal NN, Albahrani A, Guda C. Fusion gene recurrence in non-small cell lung cancers and its association with cigarette smoke exposure. Transl Lung Cancer Res 2022;11:2022-39. [Crossref] [PubMed]
- Thirlway F, Bauld L, McNeill A, et al. Tobacco smoking and vulnerable groups: Overcoming the barriers to harm reduction. Addict Behav 2019;90:134-5. [Crossref] [PubMed]
- Bittoun R, Barone M, Mendelsohn CP, et al. Promoting positive attitudes of tobacco-dependent mental health patients towards NRT-supported harm reduction and smoking cessation. Aust N Z J Psychiatry 2014;48:954-6. [Crossref] [PubMed]
- Aburatani H. Epigenomics and epigenetic therapy of cancer. Nihon Rinsho 2012;70:287-93.
- Hellyer JA, White MN, Gardner RM, et al. Impact of Tumor Suppressor Gene Co-Mutations on Differential Response to EGFR TKI Therapy in EGFR L858R and Exon 19 Deletion Lung Cancer. Clin Lung Cancer 2022;23:264-72. [Crossref] [PubMed]
- Deng T, Tang J, Zhou L, et al. Effective targeted therapy based on dynamic monitoring of gene mutations in non-small cell lung cancer. Transl Lung Cancer Res 2019;8:532-8. [Crossref] [PubMed]
- Aredo JV, Luo SJ, Gardner RM, et al. Tobacco Smoking and Risk of Second Primary Lung Cancer. J Thorac Oncol 2021;16:968-79. [Crossref] [PubMed]
- Simba H, Menya D, Mmbaga BT, et al. The contribution of smoking and smokeless tobacco to oesophageal squamous cell carcinoma risk in the African oesophageal cancer corridor: Results from the ESCCAPE multicentre case-control studies. Int J Cancer 2023;152:2269-82. [Crossref] [PubMed]
- Liu J, Chen SJ, Hsu SW, et al. MARCKS cooperates with NKAP to activate NF-kB signaling in smoke-related lung cancer. Theranostics 2021;11:4122-36. [Crossref] [PubMed]
- Chen CH, Statt S, Chiu CL, et al. Targeting myristoylated alanine-rich C kinase substrate phosphorylation site domain in lung cancer. Mechanisms and therapeutic implications. Am J Respir Crit Care Med 2014;190:1127-38. [Crossref] [PubMed]
- Hanash S. Lung cancer susceptibility beyond smoking history: opportunities and challenges. Transl Lung Cancer Res 2022;11:1230-2. [Crossref] [PubMed]
- Sun G, Ye H, Yang Q, et al. Using Proteome Microarray and Gene Expression Omnibus Database to Screen Tumour-Associated Antigens to Construct the Optimal Diagnostic Model of Oesophageal Squamous Cell Carcinoma. Clin Oncol (R Coll Radiol) 2023;35:e582-92. [Crossref] [PubMed]
- Song AY, Mu L, Dai XY, et al. Analysis of Significant Genes and Pathways in Esophageal Cancer Based on Gene Expression Omnibus Database. Chin Med Sci J 2023;38:20-8. [Crossref] [PubMed]
- Zhang Y, Wang Q, Zhu T, et al. Identification of Cigarette Smoking-Related Novel Biomarkers in Lung Adenocarcinoma. Biomed Res Int 2022;2022:9170722. [Crossref] [PubMed]
- Zhang X, Guo X, Gao Q, et al. Association between cigarette smoking history, metabolic phenotypes, and EGFR mutation status in patients with non-small cell lung cancer. J Thorac Dis 2023;15:5689-99. [Crossref] [PubMed]
- Wang Z, Jensen MA, Zenklusen JC. A Practical Guide to The Cancer Genome Atlas (TCGA). Methods Mol Biol 2016;1418:111-41. [Crossref] [PubMed]
- Rasti A, Abazari O, Dayati P, et al. Identification of Potential Key Genes Linked to Gender Differences in Bladder Cancer Based on Gene Expression Omnibus (GEO) Database. Adv Biomed Res 2023;12:157. [Crossref] [PubMed]
- Young IC, Brabletz T, Lindley LE, et al. Multi-cancer analysis reveals universal association of oncogenic LBH expression with DNA hypomethylation and WNT-Integrin signaling pathways. Cancer Gene Ther 2023;30:1234-48. [Crossref] [PubMed]
- Turi M, Anilkumar Sithara A, Hofmanová L, et al. Transcriptome Analysis of Diffuse Large B-Cell Lymphoma Cells Inducibly Expressing MyD88 L265P Mutation Identifies Upregulated CD44, LGALS3, NFKBIZ, and BATF as Downstream Targets of Oncogenic NF-κB Signaling. Int J Mol Sci 2023;24:5623. [Crossref] [PubMed]
- Jin Y, Yang Y. Identification and analysis of genes associated with head and neck squamous cell carcinoma by integrated bioinformatics methods. Mol Genet Genomic Med 2019;7:e857. [Crossref] [PubMed]
- Zhao J, Guo C, Ma Z, et al. Identification of a novel gene expression signature associated with overall survival in patients with lung adenocarcinoma: A comprehensive analysis based on TCGA and GEO databases. Lung Cancer 2020;149:90-6. [Crossref] [PubMed]
- Reynolds SD, Reynolds PR, Pryhuber GS, et al. Secretoglobins SCGB3A1 and SCGB3A2 define secretory cell subsets in mouse and human airways. Am J Respir Crit Care Med 2002;166:1498-509. [Crossref] [PubMed]
- Niimi T, Copeland NG, Gilbert DJ, et al. Cloning, expression, and chromosomal localization of the mouse gene (Scgb3a1, alias Ugrp2) that encodes a member of the novel uteroglobin-related protein gene family. Cytogenet Genome Res 2002;97:120-7. [Crossref] [PubMed]
- Mazumdar J, Hickey MM, Pant DK, et al. HIF-2alpha deletion promotes Kras-driven lung tumor development. Proc Natl Acad Sci U S A 2010;107:14182-7. [Crossref] [PubMed]
- Palalı M, Murat Özcan K, Ozdaş S, et al. Investigation of SCGB3A1 (UGRP2) gene arrays in patients with nasal polyposis. Eur Arch Otorhinolaryngol 2014;271:3209-14. [Crossref] [PubMed]
- Li Z, Feng Y, Li P, et al. CD1B is a Potential Prognostic Biomarker Associated with Tumor Mutation Burden and Promotes Antitumor Immunity in Lung Adenocarcinoma. Int J Gen Med 2022;15:3809-26. [Crossref] [PubMed]