Establishment and verification of a prediction model based on clinical characteristics and computed tomography radiomics parameters for distinguishing benign and malignant pulmonary nodules
Highlight box
Key findings
• A clinical-radiomics nomogram combining radiomic computed tomography (CT) features, carcinoembryonic antigen, and CYFRA21-1 showed good discrimination for predicting malignant vs benign lung nodules, with area of curve 0.85 and 0.76 in training and validation cohorts. The nomogram outperformed models using clinical factors or radiomics alone, as well as other common prediction models like Mayo, Veterans Affairs, and Brock.
What is known and what is new?
• Qualitative CT assessment alone is limited in distinguishing malignant vs benign lung nodules. Previous prediction models for lung nodule malignancy exist but have limitations.
• This study develops and validates a clinical-radiomics nomogram to predict lung nodule malignancy. It demonstrates the additive value of combining clinical variables with a radiomic signature extracted from CT images. The model outperforms other common prediction models.
What is the implication, and what should change now?
• Radiomics can quantify imaging features undetectable to the human eye and improve diagnostic accuracy when combined with clinical data. The clinical-radiomics nomogram could help reduce unnecessary invasive procedures on benign nodules. With further validation, this approach could be implemented in clinical practice to guide management of lung nodules.
• This study provides evidence to incorporate radiomics analysis into lung cancer screening programs and evaluation of indeterminate nodules. If validated externally, the nomogram could be adopted clinically to predict malignancy non-invasively and guide management. Radiomics should be further assessed as a tool to enhance quantitative imaging and precision medicine.
Introduction
Within the realm of malignancies in China, lung cancer reigns supreme, boasting the highest incidences and mortality rates. A staggering 70% of afflicted individuals are diagnosed at advanced stages, and the five-year survival rate is a meager 16% (1). A principal cause for the dismal survival rates among lung cancer patients can be attributed to the insidious nature of the disease, which often eludes early detection, thereby forfeiting optimal therapeutic opportunities (2). Furthermore, the heterogeneity in the morphology and dimensions of pulmonary nodules further inflates the incidence of misdiagnosis during initial stages of lung cancer. Most early-stage lung cancers manifest as solitary pulmonary nodules with a diameter of less than 3 cm, posing a significant challenge in the differential diagnosis of benign versus malignant nodules within the realm of radiographic lung cancer detection methods (3). If malignant pulmonary nodules could be identified and surgically excised at an early stage, it would substantially enhance the survival and cure rates among lung cancer patients. Consequently, the radiographic classification of pulmonary nodules into benign versus malignant categories is a pivotal component of early lung cancer diagnosis (4).
The wide application of low-dose computed tomography (LDCT) in clinic could distinguish benign and malignant pulmonary nodules, and promote the survival rate (5). Spiculation and lobulation are common computed tomography (CT) manifestations of malignant pulmonary nodules, however, research suggests that individual radiographic features or quantitative parameters are insufficient for an accurate differentiation diagnosis of pulmonary nodules (6). Thus, a multivariate prediction model is likely to improve diagnostic performance. Previous prediction models for distinguishing benign from malignant lung nodules include the Mayo (7), Veterans Affairs (VA) (8), Brock (9) models and so on. However, those predictive models still have some limitations. Firstly, they were developed using data from specific patient populations and may not be generalizable to other populations. This can lead to inaccurate predictions if the patient being evaluated differs from the population used to create the model. Secondly, they do not consider individual patient characteristics such as age, smoking history, and commodities, which can impact the likelihood of malignancy.
Radiomics is a method that involves extracting many different features from medical images using data-characterization algorithms, which has the potential to uncover tumor patterns and characteristics that are not visible to the human eye (10). In contrast to conventional visual image features, radiomics can extract substantially greater numbers of nodule features with much better reproducibility. Since the concept of radiomics has been introduced, it has been applied extensively in the identification, grading, efficacy evaluation, and prognostication of diverse neoplastic conditions (11).
Thus, the aim of the present study was to build and verify a prediction model based on high-throughput features extracted by radiomics from CT imaging and clinical characteristics to distinguish malignant from pulmonary nodules. Besides, we aimed to evaluate the incremental efficacy of this model in comparison to traditional pulmonary nodule prediction models such as Mayo, VA, and Brock. We present this article in accordance with the TRIPOD reporting checklist (available at https://jtd.amegroups.com/article/view/10.21037/jtd-23-1400/rc).
Methods
Clinical data
In this single-center retrospective study, we screened a total of 342 patients with pulmonary nodules who underwent high-resolution CT (HRCT) examination in our hospital from May 2016 to May 2021. This study was conducted in accordance with the Declaration of Helsinki (as revised in 2013). This study was approved by the institutional ethics committee of the Affiliated Hospital of Nantong University (No. 2018-L100), and written informed consent for this retrospective analysis was waived.
The inclusion criteria were as follows: (I) the patient’s clinical data were complete and the patient had undergone HRCT with satisfactory image quality; (II) the diameter of pulmonary nodule was ≤30 mm; (III) the surgery was completed within one month after HRCT examination and had definite pathological diagnosis after operation. The exclusion criteria were as follows: (I) the patient’s information was incomplete; (II) the image quality was low; (III) patients received anti-tumor therapy previously. After screening, it was found that there was a total of 342 patients that met the criteria. Using a 7:3 random sampling method, 239 patients (76 benign and 163 malignant) were selected as the training set, while 103 patients (26 benign and 77 malignant) were chosen as the validation set.
Clinical measurements
The clinical characteristics of the enrolled patients were recorded, including patient gender, age, smoking history, years since smoking cessation, previous medical history (such as hypertension, diabetes, coronary heart disease), personal tumor history, family tumor history, as well as hematological indicators such as preoperative carcinoembryonic antigen (CEA), cytokeratin-19-fragment (CYFRA21-1), adenosine deaminase (ADA), lactate dehydrogenase (LDH), neutrophil lymphocyte ratio (NLR), lymphocyte monocyte ratio (LMR), and platelet lymphocyte ratio (PLR).
Instruments and imaging method
The examinations were conducted using a 256-slice CT scanner (Brilliance iCT, Philips Healthcare, Cleveland, OH, USA) with the following parameters: The CT protocol included an automatic tube current range of 100–400 mA and section thickness of 5 mm. The collimation was set at 0.625 mm and pitch at 0.914 matrix with a resolution of 512×512 pixels. Breath-hold technique was used during full inspiration for image acquisition. Lung smooth reconstruction kernel with a thickness of 1 mm was applied to obtain images that were captured in both lung (window width: 1,500 HU; window level: −600 HU) and mediastinal (window width: 350 HU; window level: 50 HU) settings. Scanning started from thorax entrance up to posterior costal angle region.
All images were examined and recorded by two radiologists with more than five years of work experience and without knowing the pathology. When their opinions were not unified, they would discuss together to reach a consensus.
Region of interest (ROI) segmentation and data preprocessing
Dicom images were viewed with the software RadiAnt DICOM Viewer (version 4.6.5) and Image J (version v1.52j) and exported in tiff-format. The maximum boundary of the nodules was manually and semi-automatically delineated layer by layer using ITK-SNAP software (Version 3.4.0, available at http://www.itksnap.org/), by two radiologists with a collective experience of five years. Blood vessels and bronchus were avoided. The ROI segmentation was checked by one senior radiologist (Figure 1). The images and data underwent preprocessing through the application of image binarization and data normalization techniques. Image binarization was utilized to convert each pixel’s gray value in the images into either 0 or 1, resulting in binary images that were more suitable for further processing. This approach eliminated extraneous image information beyond the ROI to prevent noise introduction.
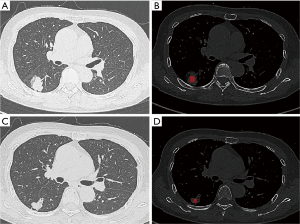
Radiomics feature extraction and selection
There were 1,781 tumor characteristics quantified by extracted features from the lesion segmented from patients’ CT images using Pyradiomics 2.2.0 (in Python software 3.6; https://www.python.org) (12). Briefly, the radiomic features consisted of four feature groups: 186 volume and shape features, 107 first-order features, 651 texture features, and 837 wavelet features. Texture features included the common gray level dependence matrix (GLDM), gray-level cooccurrence matrix (GLCM), gray level run-length matrix (GLRLM), gray-level size zone matrix (GLSZM), neighborhood gray tone difference matrix (NGTDM), and neighborhood gray-level difference matrix (NGLDM).
The presence of excessive and repetitive irrelevant data is often observed in high-dimensional information. Consequently, this can lead to overfitting and significantly impair the performance of the learning algorithm. Hence, conducting a feature selection procedure becomes imperative. The redundant features were reduced and the optimal radiomics features were selected using methods such as variance threshold, analysis of variance (ANOVA), and least absolute shrinkage and selection operator (LASSO) logistic regression model with 10-fold cross-validation. A threshold value of 0.8 was applied to the variance threshold method to eliminate eigenvalues with smaller variances. The ANOVA method included all features that exhibited significant differences (P<0.05) between benign and malignant lesions. The LASSO algorithm determined the optimal LASSO alpha parameter through five-fold cross validation, ultimately selecting radiomics features with non-zero coefficients from the training cohort (13,14). The Rad-score for each lesion was determined by combining specific features using their corresponding coefficients.
Development and validation of the clinical-radiomics nomogram
A training cohort dataset was analyzed using multivariate logistic regression to determine the clinic characteristics and Rad-score that independently predict whether pulmonary nodules are benign or malignant. Subsequently, a clinical-radiomics nomogram was created based on these factors. The nomogram’s ability to predict outcomes was evaluated by analyzing receiver operating characteristic (ROC) curves and calculating the area under the curve (AUC) in both the training and validation groups. The sensitivity, specificity, positive predictive value (PPV), and negative predictive value (NPV) were determined by optimizing the Youden index to identify its optimal threshold. The calibration curves were used to assess how well the predicted probabilities matched the observed results. To assess the practical value of the nomogram, decision curve analysis (DCA) was performed in the validation group to measure the net benefit across various threshold probabilities. Net benefit referred to the weighted discrepancy between true positive proportion and false positive proportion, considering the relative risk linked with false positive and false negative outcomes.
Statistical analysis
The statistical analyses were carried out using R software (version 3.5.1, https://www.r-project.org/). The LASSO regression model was constructed with the “glmnet” package and ROC curve analysis was performed using the “pROC” package. Categorical variables were analyzed in terms of frequencies and proportions, while continuous variables were described using mean and standard deviation. The Chi-square test was utilized to compare categorical variables, whereas the independent t-test was employed for continuous variables comparison. The DeLong method was employed to assess the importance of the distinction between the clinical-radiomics nomogram and alternative models (15,16). A P value less than 0.05 was considered statistically significant.
Results
Patient features
In this study, all 342 patients had available pathological results. The histopathological examination revealed 102 cases (30%) of benign nodules (27 cases of pulmonary sequestration, 37 cases of tuberculoma, 20 cases of chronic inflammation of lung tissue, 18 cases of sclerosing pneumocytoma). The 240 (70%) malignant solitary pulmonary nodules included 208 cases of adenocarcinoma, 29 cases of squamous cell carcinoma, and 3 cases of small cell lung cancer. The included patients were divided into the training and validation cohorts (239 in the training cohort and 103 in the validation cohort). The baseline characteristics of the cohort are listed in Table 1. No significant difference in age, gender, smoking, family history of cancer, nodule size, and hematological indicators were noted between the training cohort and validation cohort groups (all P>0.05). Univariate and multivariate logistic regression analyses of clinical risk factors concluded that age, CEA and CYFRA21-1 were independent influencing factors of malignant pulmonary nodule [odds ratio (OR) 95% confidence interval (CI) =1.05 (1.02–1.08), 1.45 (1.15–1.90), 1.42 (1.06–2.06), respectively]. Multivariate logistic regression was used to establish a clinical prediction model (baseline model), which was as follows:
Table 1
Characteristic | Training cohort, N=239 | Validation cohort, N=103 | P value |
---|---|---|---|
Gender | 0.71 | ||
Male | 95 (39.7) | 38 (36.9) | |
Female | 144 (60.3) | 65 (63.1) | |
Age, years | 59.74±10.80 | 60.34±9.92 | 0.63 |
Smoking | 18 (7.5) | 12 (11.7) | 0.3 |
Family history of cancer | 1 (0.4) | 1 (1.0) | 1 |
Nodule size (mm) | 16.01±5.11 | 16.28±4.91 | 0.74 |
Hematological indicators | |||
CEA (μg/L) | 3.36±6.37 | 2.79±2.19 | 0.38 |
CYFRA21-1 (ng/mL) | 1.74±0.87 | 1.62±0.83 | 0.24 |
NLR | 2.30±1.76 | 1.89±0.82 | 0.03 |
LMR | 4.29±1.71 | 4.64±1.74 | 0.08 |
PLR | 122.41±47.39 | 118.07±43.52 | 0.43 |
LDH (U/L) | 188.86±37.82 | 182.46±39.62 | 0.16 |
ADA (U/L) | 9.28±2.98 | 8.59±2.36 | 0.04 |
Data are presented as n (%) or mean ± standard deviation. CEA, carcinoembryonic antigen; CYFRA21-1, cytokeratin-19-fragment; NLR, neutrophil to lymphocyte ratio; LMR, lymphocyte to monocyte ratio; PLR, platelet to lymphocyte ratio; LDH, lactate dehydrogenase; ADA, adenosine deaminase.
Radiomics feature selection and radiomics score construction
For this study, we extracted various features including volume and shape features, first-order features, texture features, and wavelet features for each case. A total of 1,781 radiomic features were obtained. The two experienced radiologists achieved good consistency between their observations with an interobserver coefficient exceeding 0.80. Then λ=0.0427 with log (λ)=−1.369 was chosen as the optimal value, and finally, the optimal λ resulted in 24 non-zero coefficients (Figure 2). The 24 non-zero coefficients are shown in Table 2. Then the results of the multiple logistic regression analysis showed that original_shape_Sphericity, exponential_glcm_Maximum Probability, log_sigma_2_0_mm_3D_glcm_Maximum Probability, and logarithm_firstorder_90 Percentile were independent risk factors for malignant pulmonary nodules [OR (95% CI) =0.34 (0.23–0.49), 1.44 (1.10–2.476), 0.42 (0.20–0.74), 0.60 (0.39–0.89), respectively]. The Rad-score of each patient was calculated by the following calculation formula:
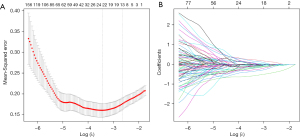
Table 2
Radiomics features | Coefficients |
---|---|
original_shape_Sphericity | −0.926 |
exponential_glcm_MaximumProbability | 0.749 |
log_sigma_2_0_mm_3D_glcm_MaximumProbability | −0.838 |
logarithm_firstorder_90Percentile | −1.059 |
wavelet_LLL_ngtdm_Coarseness | −0.439 |
wavelet_HHL_ngtdm_Coarseness | −0.447 |
wavelet_LHL_ngtdm_Coarseness | −0.658 |
exponential_glcm_ClusterProminence | −0.501 |
log_sigma_2_0_mm_3D_firstorder_90Percentile | 0.589 |
log_sigma_1_0_mm_3D_firstorder_90Percentile | 0.532 |
wavelet_HLH_firstorder_Mean | −0.469 |
log_sigma_1_0_mm_3D_glszm_LargeAreaHighGrayLevelEmphasis | 0.996 |
wavelet_HLL_glcm_SumEntropy | −0.534 |
logarithm_glcm_Imc2 | 0.448 |
wavelet_LLL_glcm_SumEntropy | −0.457 |
log_sigma_1_0_mm_3D_glcm_Id | 0.227 |
gradient_glcm_MaximumProbability | −0.231 |
log_sigma_3_0_mm_3D_gldm_GrayLevelVariance | 0.194 |
log_sigma_5_0_mm_3D_glcm_Contrast | −0.112 |
wavelet_LHH_glcm_SumEntropy | 0.246 |
wavelet_LHL_glcm_SumEntropy | −0.087 |
gradient_gldm_GrayLevelVariance | −0.033 |
wavelet_LLL_firstorder_90Percentile | −0.034 |
wavelet_LHH_glcm_MCC | 0.002 |
Development of the clinical-radiomics nomogram
Multivariate logistic regression results are shown in Table 3. Multivariate logistic regression showed that CEA [OR (95% CI): 1.40 (1.09–1.88)], CT rad score [OR (95% CI): 2.74 (2.03–3.85)], and CYFRA21-1 [OR (95% CI): 1.80 (1.14–2.94)] were independent influencing factors of malignant pulmonary nodule (all P<0.05). Then, we constructed a clinical-radiomics nomogram, integrating CEA, CT rad-score, and CYFRA21-1, according to the Akaike Information Criterion (AIC) in the training cohort (Figure 3). The nomogram formula is as follows:
Table 3
Variables | Coefficient | OR | 95% CI | P |
---|---|---|---|---|
CEA | 0.34 | 1.40 | 1.09–1.88 | 0.01* |
CYFRA21-1 | 0.59 | 1.80 | 1.14–2.94 | 0.02* |
CT rad-score | 1.01 | 2.74 | 2.03–3.85 | <0.001* |
*, P<0.05. OR, odds ratio; CI, confidence interval; CEA, carcinoembryonic antigen; CYFRA21-1, cytokeratin-19-fragment; CT, computed tomography.
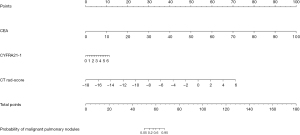
Validation of the clinical-radiomics nomogram
The diagnostic efficiency of each model in the training and verification cohorts is showed in Table 4. The AUCs of clinical model, CT rad-score, and clinical-radiomics nomogram for predicting malignant pulmonary nodule in the training cohort were 0.74 (95% CI: 0.68–0.81), 0.81 (95% CI: 0.74–0.87) and 0.85 (95% CI: 0.80–0.91), respectively. The AUCs of clinical model, CT rad-score, and clinical-radiomics nomogram for predicting malignant pulmonary nodule in the validation cohort were 0.64 (95% CI: 0.53–0.76), 0.75 (95% CI: 0.64–0.85) and 0.76 (95% CI: 0.66–0.86) respectively (Figure 4). Clinical-radiomics nomogram shows significant advantage over CT rad-score alone and clinical model for predicting malignant pulmonary nodule. The sensitivity, specificity of clinical-radiomics nomogram for predicting malignant pulmonary nodule were 81.2% and 75.9% in the training cohort; 84.3%, and 63.6% in the internal validation cohort, respectively. Compared with three common models (Mayo, VA, Brock), the clinical-radiomics nomogram still had the highest AUC both in the training cohort [0.85 (95% CI: 0.80–0.89) vs. 0.72 (95% CI: 0.66–0.78) vs. 0.72 (95% CI: 0.66–0.77), vs. 0.72 (95% CI: 0.66–0.77), respectively] and validation cohort [0.76 (95% CI: 0.67–0.84), 0.74 (95% CI: 0.64–0.82), 0.63 (95% CI: 0.53–0.72), 0.71 (95% CI: 0.61–0.79), respectively]. The clinical-radiomics nomogram’s calibration curves exhibited strong agreement between predicted outcomes and observations in both the training and validation groups (Figure 5). The DCA indicated that clinical-radiomics nomogram could add more net benefits than “all treatment” or “none treatment” with the threshold probability range from 0 to 1.0 in the validation cohort (Figure 6).
Table 4
Cohort | Models | AUC (95% CI) | Sensitivity (%) | Specificity (%) | PPV (%) | NPV (%) |
---|---|---|---|---|---|---|
Training cohort | Clinical model | 0.74 (0.68–0.81) | 77.1 | 62.3 | 83.4 | 52.4 |
CT rad-score | 0.81 (0.74–0.87) | 79.4 | 73.9 | 88.2 | 59.3 | |
Clinical-radiomics nomogram | 0.85 (0.80-0.91) | 81.2 | 75.9 | 90.9 | 57.7 | |
Validation cohort | Clinical model | 0.64 (0.53–0.76) | 72.9 | 63.6 | 89.0 | 52.5 |
CT rad-score | 0.75 (0.64–0.85) | 82.9 | 63.6 | 82.9 | 63.6 | |
Clinical-radiomics nomogram | 0.76 (0.66–0.86) | 84.3 | 63.6 | 83.1 | 65.6 |
AUC, area under the curve; CI, confidence interval; PPV, positive predictive value; NPV, negative predictive value; CT, computed tomography.
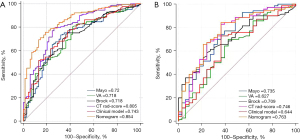
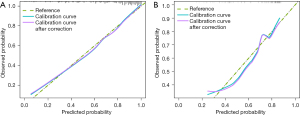
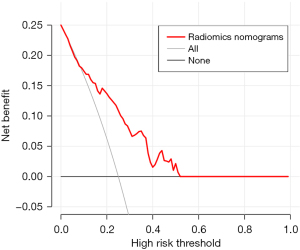
Discussion
The article discussed a non-invasive diagnostic method for distinguishing benign pulmonary nodules from malignant pulmonary nodules. The results of this study showed that CEA, CYFRA21-1 and CT rad-score were important predictors for predicting the malignant pulmonary nodules.
CEA serves as a widely employed biomarker in clinical practice for early detection and monitoring of lung cancer. CYFRA21-1, on the other hand, is a frequently utilized tumor marker in clinical settings and is often employed as a primary adjunctive diagnostic tool for squamous cell carcinoma of the lung. Several studies have indicated a widespread elevation of serum CEA in patients with non-small cell lung cancer, suggesting its potential utility as a candidate biomarker for the diagnosis of non-small cell lung cancer. Additionally, the level of serum CYFRA21-1 exhibits a strong correlation with tumor staging and disease progression, thereby serving as a viable means to monitor treatment efficacy (17,18).
Radiomics refers to the artificial intelligence technology that extracts shape, intensity, texture, and wavelet features of lesions based on imaging images [such as CT, magnetic resonance imaging (MRI), and positron emission tomography (PET)-CT], converts them into high-dimensional quantifiable quantitative feature data to further reflect the biological information of lesions, which is a process that transforms the subjective evaluation of images into objective quantitative data. It can provide relevant information for diagnosing diseases, prognostic evaluation and predicting treatment efficacy (19). It was reported that radiomics is valuable in predicting the benign and malignant effects of pulmonary nodules, but different imaging omics studies extract different features (10). Hawkins et al. extracted 219 features from CT images of 600 pulmonary nodules, and ultimately selected 23 stable features to distinguish between benign and malignant nodules with an accuracy rate of 80% and a false positive rate of 9%. However, the study had inconsistent standards for collecting CT images with varying slice thicknesses, limited clinical data integration, and used support vector machine (SVM) algorithms that consume large amounts of memory and are sensitive to missing data, which limits its discriminatory potential (20). Feng et al. developed clinical risk factor models, radiomics models, and a comprehensive model combining radiomics features and clinical risk factors to predict the malignancy of 426 pulmonary nodules. The radiomics model utilized the LASSO logistic regression algorithm to reduce the β coefficients of the classifier, decrease classifier variance, and prevent overfitting. The results showed that the diagnostic accuracy of the comprehensive model was higher than that of the individual models, with AUC values of 0.97, 0.93, and 0.91 for training set, internal validation, and external validation cohorts, respectively. The diagnostic accuracy, sensitivity, and specificity for the external validation cohort were 0.82, 0.79, and 0.95, indicating that the combination of radiomics features and clinical risk factors has significant value in distinguishing between benign and malignant pulmonary nodules (21).
In the present study, it was concluded that original_shape_Sphericity, exponential_glcm_Maximum Probability, log_sigma_2_0_mm_3D_glcm_Maximum Probability, and logarithm_firstorder_90 Percentile are independent radiomic characteristics for malignant pulmonary nodules. The feature “original_shape_Sphericity” in pulmonary nodules represents the degree of sphericity or roundness of the nodule’s shape. It provides information about how closely the shape of the nodule resembles a sphere. Malignant nodules often exhibit more irregular and non-spherical shapes compared to benign nodules, which tend to be more spherical. Therefore, a lower value of “original_shape_Sphericity” may indicate a higher likelihood of malignancy, while a higher value may suggest a lower probability of malignancy (22). The feature “exponential_glcm_Maximum Probability” in pulmonary nodules represents the maximum probability value obtained from the gray-level co-occurrence matrix (GLCM) after applying an exponential transformation, which can be useful in quantifying the dominant or most prominent texture pattern within the nodule. log_sigma_2_0_mm_3D_glcm_MaximumProbability provides information about the texture or spatial arrangement of gray-level intensities within the nodule volume at a particular scale. The “logarithm_firstorder_90Percentile” feature is useful for characterizing the overall intensity distribution and heterogeneity within the nodule. Different types of nodules may exhibit distinct intensity patterns due to variations in tissue composition, vascularity, or necrosis. The clinical-radiomics nomogram model we constructed provides a comprehensive description of the malignant pulmonary nodule by extracting more statistical features.
Qualitative diagnosis of pulmonary nodules is crucial for early diagnosis and treatment. However, relying solely on HRCT for qualitative diagnosis can be very difficult. Our results showed that this clinical-radiomics nomogram combining CEA, CYFRA21 and CT rad-score had good preoperative prediction performance of pulmonary nodule classification. The results showed that the clinical-radiomics nomogram had higher AUC values and accuracy than the common models (Mayo, VA, Brock). The calibration curves indicated a high consistency between the predicted probability of nomograms and the actual observation, and our model achieved high predictive performance. The DCA demonstrated that the clinical-radiomics nomogram was clinically valuable.
There are several limitations in the present study. Firstly, the study population is sourced from a single institution, thus limiting the sample size. This restriction in the number of validation cohort participants may potentially impact the validation of the proposed models. When mispredictions occur in a small number of lesions, it can result in a significant discrepancy. While our results hold encouraging potential, a more extensive, multicenter study with equitably distributed samples is necessitated to conclusively affirm the resilience of the proposed nomogram. Secondly, the extraction of radiomics features was conducted on two-dimensional (2D) ROIs. While 2D features may potentially omit crucial information required for a comprehensive characterization of the entire lesion, comparative studies have revealed that 2D features exhibit superior performance to their three-dimensional (3D) counterparts in the context of lung cancer (23). Thirdly, patients who underwent surgical procedures were disproportionately inclined to be those with a confirmed diagnosis of malignant neoplasms. Lack of external validation was the fourth flaw.
Conclusions
The clinical-radiomics nomogram combined of CT-based radiomics signature, along with CYFRA21-1 and CEA, demonstrated strong predictive ability, calibration, and clinical usefulness in distinguishing between benign and malignant pulmonary nodules. The use of CT-based radiomics has the potential to assist clinicians in making informed decisions prior to biopsy or surgery while avoiding unnecessary treatment for non-cancerous lesions.
Acknowledgments
Funding: None.
Footnote
Reporting Checklist: The authors have completed the TRIPOD reporting checklist. Available at https://jtd.amegroups.com/article/view/10.21037/jtd-23-1400/rc
Data Sharing Statement: Available at https://jtd.amegroups.com/article/view/10.21037/jtd-23-1400/dss
Peer Review File: Available at https://jtd.amegroups.com/article/view/10.21037/jtd-23-1400/prf
Conflicts of Interest: All authors have completed the ICMJE uniform disclosure form (available at https://jtd.amegroups.com/article/view/10.21037/jtd-23-1400/coif). The authors have no conflicts of interest to declare.
Ethical Statement: The authors are accountable for all aspects of the work in ensuring that questions related to the accuracy or integrity of any part of the work are appropriately investigated and resolved. This study was conducted in accordance with the Declaration of Helsinki (as revised in 2013). This study was approved by the institutional ethics committee of the Affiliated Hospital of Nantong University (No. 2018-L100), and written informed consent for this retrospective analysis was waived.
Open Access Statement: This is an Open Access article distributed in accordance with the Creative Commons Attribution-NonCommercial-NoDerivs 4.0 International License (CC BY-NC-ND 4.0), which permits the non-commercial replication and distribution of the article with the strict proviso that no changes or edits are made and the original work is properly cited (including links to both the formal publication through the relevant DOI and the license). See: https://creativecommons.org/licenses/by-nc-nd/4.0/.
References
- Sung H, Ferlay J, Siegel RL, et al. Global Cancer Statistics 2020: GLOBOCAN Estimates of Incidence and Mortality Worldwide for 36 Cancers in 185 Countries. CA Cancer J Clin 2021;71:209-49. [Crossref] [PubMed]
- Gould MK, Donington J, Lynch WR, et al. Evaluation of individuals with pulmonary nodules: when is it lung cancer? Diagnosis and management of lung cancer, 3rd ed: American College of Chest Physicians evidence-based clinical practice guidelines. Chest 2013;143:e93S-e120S.
- Hansell DM, Bankier AA, MacMahon H, et al. Fleischner Society: glossary of terms for thoracic imaging. Radiology 2008;246:697-722. [Crossref] [PubMed]
- Morgan L, Choi H, Reid M, et al. Frequency of Incidental Findings and Subsequent Evaluation in Low-Dose Computed Tomographic Scans for Lung Cancer Screening. Ann Am Thorac Soc 2017;14:1450-6. [Crossref] [PubMed]
- Siegel RL, Miller KD, Fuchs HE, et al. Cancer Statistics, 2021. CA Cancer J Clin 2021;71:7-33. [Crossref] [PubMed]
- Harzheim D, Eberhardt R, Hoffmann H, et al. The Solitary Pulmonary Nodule. Respiration 2015;90:160-72. [Crossref] [PubMed]
- Zhang R, Wei Y, Wang D, et al. Deep learning for malignancy risk estimation of incidental sub-centimeter pulmonary nodules on CT images. Eur Radiol 2023; Epub ahead of print. [Crossref]
- Zhao HC, Xu QS, Shi YB, et al. Clinical-radiological predictive model in differential diagnosis of small (≤ 20 mm) solitary pulmonary nodules. BMC Pulm Med 2021;21:281. [Crossref] [PubMed]
- Baldwin DR. Management of pulmonary nodules according to the 2015 British Thoracic Society guidelines. Key messages for clinical practice. Pol Arch Med Wewn 2016;126:262-74. [Crossref] [PubMed]
- Wilson R, Devaraj A. Radiomics of pulmonary nodules and lung cancer. Transl Lung Cancer Res 2017;6:86-91. [Crossref] [PubMed]
- Javaid M, Javid M, Rehman MZ, et al. A novel approach to CAD system for the detection of lung nodules in CT images. Comput Methods Programs Biomed 2016;135:125-39. [Crossref] [PubMed]
- van Griethuysen JJM, Fedorov A, Parmar C, et al. Computational Radiomics System to Decode the Radiographic Phenotype. Cancer Res 2017;77:e104-7. [Crossref] [PubMed]
- Qiu J, Peng S, Yin J, et al. A Radiomics Signature to Quantitatively Analyze COVID-19-Infected Pulmonary Lesions. Interdiscip Sci 2021;13:61-72. [Crossref] [PubMed]
- Mao B, Ma J, Duan S, et al. Preoperative classification of primary and metastatic liver cancer via machine learning-based ultrasound radiomics. Eur Radiol 2021;31:4576-86. [Crossref] [PubMed]
- DeLong ER, DeLong DM, Clarke-Pearson DL. Comparing the areas under two or more correlated receiver operating characteristic curves: a nonparametric approach. Biometrics 1988;44:837-45.
- Zou GY, Yue L. Using confidence intervals to compare several correlated areas under the receiver operating characteristic curves. Stat Med 2013;32:5077-90. [Crossref] [PubMed]
- Trulson I, Holdenrieder S. Prognostic value of blood-based protein biomarkers in non-small cell lung cancer: A critical review and 2008-2022 update. Tumour Biol 2023; Epub ahead of print. [Crossref]
- Chen Z, Liu X, Shang X, et al. The diagnostic value of the combination of carcinoembryonic antigen, squamous cell carcinoma-related antigen, CYFRA 21-1, neuron-specific enolase, tissue polypeptide antigen, and progastrin-releasing peptide in small cell lung cancer discrimination. Int J Biol Markers 2021;36:36-44. [Crossref] [PubMed]
- Song F, Song X, Feng Y, et al. Radiomics feature analysis and model research for predicting histopathological subtypes of non-small cell lung cancer on CT images: A multi-dataset study. Med Phys 2023;50:4351-65. [Crossref] [PubMed]
- Hawkins S, Wang H, Liu Y, et al. Predicting Malignant Nodules from Screening CT Scans. J Thorac Oncol 2016;11:2120-8. [Crossref] [PubMed]
- Feng B, Chen X, Chen Y, et al. Radiomics nomogram for preoperative differentiation of lung tuberculoma from adenocarcinoma in solitary pulmonary solid nodule. Eur J Radiol 2020;128:109022. [Crossref] [PubMed]
- Cai Q, Du SY, Gao S, et al. A model based on CT radiomic features for predicting RT-PCR becoming negative in coronavirus disease 2019 (COVID-19) patients. BMC Med Imaging 2020;20:118. [Crossref] [PubMed]
- Shen C, Liu Z, Guan M, et al. 2D and 3D CT Radiomics Features Prognostic Performance Comparison in Non-Small Cell Lung Cancer. Transl Oncol 2017;10:886-94. [Crossref] [PubMed]