Clinical-radiomics nomogram for the risk prediction of esophageal fistula in patients with esophageal squamous cell carcinoma treated with intensity-modulated radiation therapy or volumetric-modulated arc therapy
Highlight box
Key findings
• The clinical-radiomics model had the best performance in predicting esophageal fistula (EF) in patients with esophageal squamous cell carcinoma (ESCC), with an area under the receiver operating characteristic curve value of 0.896.
What is known and what is new?
• Previous studies have established EF prediction models using clinical characteristics, such as, but not limited to, T4, N3, maximum thickness of the tumor, ulcerative type, and stenosis. However, the predictive value of radiomics in EF has been less reported. Few studies have reported the risk factors for EF in patients with ESCC treated by intensity-modulated radiation therapy (IMRT) or volumetric-modulated arc therapy (VMAT).
• In this study, we analyze the predictive factors of EF in patients with ESCC who received IMRT or VMAT and establish a prediction model for EF based on clinical factors and radiomics features.
What is the implication, and what should change now?
• This is the first study to establish a clinical-radiomics model for predicting EF in patients with ESCC treated by IMRT or VMAT. For the patients with the high risk of esophageal fistula, strong consideration for chemotherapy alone may be a preferred treatment choice.
Introduction
Esophageal cancer (EC) is the sixth most common cancer and ranks fifth in tumor-related death among all cancers in China (1). About 90% of EC cases in China are esophageal squamous cell carcinoma (ESCC) (2). Radiotherapy with or without chemotherapy is the current standard treatment for unresectable locally advanced EC (3). Esophageal fistula (EF) is a serious adverse event occurring during or after radiotherapy in patients with EC. The incidence of EF in patients with EC treated with radiotherapy is about 4–24% (4-7) with a median survival of 5.3–11.0 months (7-12) when one occurs.
Previous studies have established EF prediction models using T4, N3, maximum thickness of the tumor, ulcerative type, stenosis, and low body mass index (BMI) (7-17). In addition to clinical factors, quantitative imaging features might provide additional information for risk prediction. Radiomics, a technology used to efficiently mine quantitative image features from standard medical imaging, has been widely used in prognosis prediction (18,19). Previous studies showed that computed tomography (CT)-based radiomics could predict survival in patients with EC (20-24), while the effectiveness of this procedure to identify the risk factors for EF after intensity-modulated radiation therapy (IMRT) or volumetric-modulated arc therapy (VMAT) remained debate (11,25,26).
This study thus aimed to analyze the predictive factors of EF in patients with unresectable locally advanced ESCC that underwent IMRT or VMAT and to further establish a prediction model for EF based on clinical factors and radiomics features. We present this article in accordance with the TRIPOD reporting checklist (available at https://jtd.amegroups.com/article/view/10.21037/jtd-24-191/rc).
Methods
Study design
It was a retrospective single institution study. The data of all consecutive patients with ESCC treated at Xijing Hospital from January 2013 to December 2020 were evaluated. We included in the analysis the data of patients: (I) with a histological diagnosis of ESCC; (II) not surgical candidate due to the local extension of disease; and (III) underwent IMRT or VMAT. Patients were excluded for any of the following reasons: (I) underwent surgery for management of esophageal cancer and/or of other thoracic tumors; (II) underwent previous thoracic radiotherapy; (III) with EF occurred before IMRT or VMAT; (IV) with a histological diagnosis of esophageal tumor different from squamous cell carcinoma; or (V) with incomplete clinical data or incomplete follow-up. Patients were divided into two study groups including EF group and non-EF group based if they developed or not an EF after IMRT or VMAT, respectively. The intergroup differences were statistically compared (I) to identify the predictive factors for EF, and (II) to establish a prediction model for EF based on clinical factors and radiomics features (end-points of the study). EF was diagnosed via barium esophagram, CT scan, magnetic resonance imaging, or endoscopy.
The study was conducted in accordance with the Declaration of Helsinki (as revised in 2013), and it was approved by the local ethics committee of Xijing Hospital (No. KT20172035-1). Informed consent was waived due to the retrospective nature of the study.
Clinical data collection
All data were collected from electronic medical records and the radiotherapy system. Clinical characteristics, hematological parameters, and treatment characteristics were recorded and analyzed. The basic clinical characteristics included age at diagnosis, gender, Eastern Cooperative Oncology Group performance status, smoking history, drinking history, and BMI. Tumor characteristics included the location of tumor, T stage, N stage, tumor type, stenosis grade, longitudinal length of tumor, tumor axial maximum thickness, and tumor volume. Treatment characteristics included induction chemotherapy, concurrent chemotherapy, chemotherapy regimen, IMRT or VMAT, total radiation dose, single radiation dose, and radiation fields. Hematological parameters included leukocyte count, neutrophil count, lymphocyte count, lymphocyte rate (LR), hemoglobin level, platelet count, and serum albumin level. All hematological parameters were collected within 1 week before radiotherapy, and the neutrophil to lymphocyte ratio (NLR), hemoglobin to lymphocyte ratio (HLR), and platelet to lymphocyte ratio (PLR) were calculated according to the collected data. The Onodera prognostic nutritional index (PNI) was calculated as follows: PNI = serum albumin (g/L) + 5 × total number of peripheral blood lymphocytes (×109/L). Tumor staging was performed according to the seventh edition of the American Joint Committee on Cancer (AJCC) staging criteria. The type of EC was classified according to findings on barium esophagram as determined by at least two senior radiologists. The stenosis grade was determined according to the ratio of the diameter of the stenosis segment and the normal segment of the esophagus on the barium meal image, as reported in Gui et al. (27). We used this method to divide the esophageal stenosis into four grades: grade I, 0–24%; grade II, 25–49%; grade III, 50–74%; and grade IV, 75–100%. For continuous variables that were in accordance with normal distribution, the missing values were imputed using the mean value. For continuous variables that were not in accordance with normal distribution, the missing values were imputed using the median value. After radiotherapy, follow-up evaluations were performed every 3 months in years 1–2, every 6 months in years 3–5, and once a year thereafter. The follow-up period ended in December 2022. Overall survival (OS) was calculated from the start of radiotherapy to any-cause death.
Construction of the radiomics model
The radiomics flowchart of this study was summarized in Figure 1. All patients underwent standard chest contrast-enhanced CT scanning before radiotherapy with a Brilliance Big Bore scanner (Philips, Amsterdam, the Netherlands) under the following acquisition parameters: 120-kV tube voltage, 300-mA tube current, 5-mm slice thickness, 512×512 pixels in-plane resolution, and standard B (body) reconstruction kernels. The tumor was then delineated as the region of interest (ROI) by one radiologist with 5 years of experience in imaging diagnosis via ITK-SNAP software (www.itksnap.org). Another two radiologists with 10 years of experience corrected the ROIs through consensus.
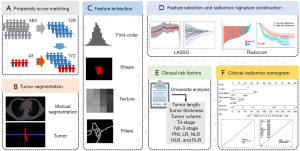
Z-score normalization, which can reduce the variability between images from different patients, was applied to the images of each patient. The 1,223 radiomics features were extracted with Pyradiomics (version 2.2.0) (28). To reduce any bias or overfitting caused by the presence of too many features, feature selection was conducted via the least absolute shrinkage and selection operator (LASSO) (29,30). The 1,223 radiomic features extracted with Pyradiomics included 107 original features and 1,116 texture features. The original features consisted of 18 first-order statistics features, 14 shape-based features, and 75 texture features [24 gray-level co-occurrence texture matrix (GLCM) features, 14 gray-level dependence matrix (GLDM) features, 16 gray-level run-length texture matrix (GLRLM) features, 16 gray-level size zone matrix (GLSZM) features, and 5 neighborhood gray-tone difference matrix (NGTDM) features]. The 1,116 texture features consisted of 372 Laplacian of Gaussian features (sigma: 2, 3, 4, 5) and 744 wavelet features. The specific hyperparameters are listed in Table S1. The detailed mathematical definitions of these radiomic features complied with the image biomarker standardization initiative (IBSI) guidelines and can be found within the Pyradiomics documentation (pyradiomics.readthedocs.io) (28).
In the parameter tuning phase of the LASSO algorithm, the parameter λ, which controls the strength of regularization, was chosen using 5-fold cross-validation repeated 100 times via the area under the receiver operating characteristic (ROC) curve (AUC) maximum criteria to extract the effective and predictive features. Most of the coefficients of the features were reduced to zero, and the remaining nonzero coefficients were selected by LASSO. A radiomics signature was developed as a radiomics score (Radscore) calculated as the linear combination of the selected features that were weighted by their respective coefficients (31). In the analysis of the association of Radscore with OS, patients were classified into a low-risk group and a high-risk group according to the median Radscore.
Statistical analysis
Data were expressed as mean ± standard deviation or as a number (percentage). To reduce the intergroup differences, the EF and non-EF groups were matched according to age at diagnosis and gender at a ratio of 1:3 using propensity score matching (PSM).
Multivariable analysis identified the risk factors for EF (independent variable). The variables with a P value <0.1 on univariate logistic regression entered into multivariate stepwise logistic regression. The best cutoff values of the potential associated factors for predicting EF risk were determined using the ROC curves. The clinical and clinical-radiomics prediction model were established based on the results of multivariate logistic regression, and the nomograms for the prediction of radiotherapy-related EF probability were generated. The discrimination performance of the clinical, radiomics, and clinical-radiomics models was assessed according to the AUC, accuracy, sensitivity, specificity, positive predictive value, and negative predictive value based on 100 repetitions of 5-fold cross-validation. Calibration curve and Brier score were adopted to evaluate the nomograms. The Brier score is a measure of the calibration of probabilistic prediction on a set of probabilistic predictions, which range from 0 to 1. A lower Brier score indicates a higher accuracy of the nomogram. Decision curve analysis was conducted to estimate the clinical usefulness of the models by quantifying the net benefits at different threshold probabilities. The Kaplan-Meier method was used to plot survival curves, and the log-rank test was used to compare survival between groups. Statistical analysis was performed using SPSS 26.0 (IBM Corp., Armonk, NY, USA) and R version 3.3.3 (The Foundation for Statistical Computing, Vienna, Austria; www.R-project.org).
Results
Patient characteristics
A total of 483 patients with ESCC who received IMRT or VMAT were included in this study. According to age at EC diagnosis and gender, 43 patients with EF and 129 patients without EF were matched at a ratio of 1:3. Among the 172 patients, 124 (72.1%) were males and 48 (27.9%) were females. The median age was 66 years (range, 45–81 years) in the EF group and 67 years (range, 36–91 years) in the non-EF group. The proportion of T4 stage patients in the two groups was 51.2% and 18.6%, respectively; the proportion of N2–3 stage patients in the two groups was 41.9% and 20.9%, respectively. The incidence of ulcerative tumors in the EF and non-EF groups was 2.3% and 7.0%, respectively. Nutrition evaluation showed that 11.6% of EF patients and 10.9% of non-EF patients had a BMI <18.5 kg/m2. The proportion of patients with PNI ≥45 in the EF and non-EF groups was 60.5% and 87.6%, respectively. The proportion of patients receiving induction chemotherapy in the two groups was 67.4% and 81.4%, respectively. Moreover, 90.7% of the EF patients and 85.3% of the non-EF patients received concurrent chemoradiotherapy. The proportions of cervical/upper, middle, and lower thoracic ESCC were 11.6%, 53.5%, and 34.9% in the EF group, while in the non-EF group, these proportions were 19.4%, 56.6%, and 24.0%. Concerning other treatment, 9 patients received IMRT, and 163 patients received VMAT. Additionally, 47 patients received radiotherapy with a dose equal to or greater than 60 Gy, 120 patients received radiotherapy with a dose between 50 and 60 Gy, and 5 patients received radiotherapy with a dose less than 50 Gy. In the EF and non-EF groups, 88.4% and 96.1% of the patients had a serum albumin level >35 g/L, respectively. Finally, 16 patients (9.3%) had grade IV esophageal stenosis, with 7 patients from the EF group and 9 patients from the non-EF group (Table 1).
Table 1
Characteristics | Before PSM (n=483), n (%) | After PSM (n=172), n (%) | |||||
---|---|---|---|---|---|---|---|
EF (n=43) | Non-EF (n=440) | P value | EF (n=43) | Non-EF (n=129) | P value | ||
Gender | 0.509 | >0.99 | |||||
Male | 31 (72.1) | 337 (76.6) | 31 (72.1) | 93 (72.1) | |||
Female | 12 (27.9) | 103 (23.4) | 12 (27.9) | 36 (27.9) | |||
Age (years) | 0.595 | 0.453 | |||||
≤60 | 11 (25.6) | 97 (22.0) | 11 (25.6) | 26 (20.2) | |||
>60 | 32 (74.4) | 343 (78.0) | 32 (74.4) | 103 (79.8) | |||
ECOG PS | 0.311 | 0.929 | |||||
≤1 | 24 (55.8) | 210 (47.7) | 24 (55.8) | 73 (56.6) | |||
>1 | 19 (44.2) | 230 (52.3) | 19 (44.2) | 56 (43.4) | |||
Smoking | 0.597 | >0.99 | |||||
No | 19 (44.2) | 213 (48.4) | 19 (44.2) | 57 (44.2) | |||
Yes | 24 (55.8) | 227 (51.6) | 24 (55.8) | 72 (55.8) | |||
Alcohol abuse | 0.067 | 0.148 | |||||
No | 34 (79.1) | 287 (65.2) | 34 (79.1) | 87 (67.4) | |||
Yes | 9 (20.9) | 153 (34.8) | 9 (20.9) | 42 (32.6) | |||
Diabetes | 0.729 | 0.414 | |||||
No | 40 (93.0) | 415 (94.3) | 40 (93.0) | 124 (96.1) | |||
Yes | 3 (7.0) | 25 (5.7) | 3 (7.0) | 5 (3.9) | |||
Hypertension | 0.910 | 0.665 | |||||
No | 35 (81.4) | 355 (80.7) | 35 (81.4) | 101 (78.3) | |||
Yes | 8 (18.6) | 85 (19.3) | 8 (18.6) | 28 (21.7) | |||
BMI (kg/m2) | 0.956 | 0.963 | |||||
<18.5 | 5 (11.6) | 52 (11.8) | 5 (11.6) | 14 (10.9) | |||
18.5–23.9 | 28 (65.1) | 269 (61.1) | 28 (65.1) | 85 (65.9) | |||
24–27.9 | 9 (20.9) | 106 (24.1) | 9 (20.9) | 25 (19.4) | |||
≥28 | 1 (2.3) | 13 (3.0) | 1 (2.3) | 5 (3.9) | |||
Tumor axial maximum thickness (cm) | 0.019 | 0.005 | |||||
≤2.5 | 5 (11.6) | 124 (28.2) | 5 (11.6) | 44 (34.1) | |||
>2.5 | 38 (88.4) | 316 (71.8) | 38 (88.4) | 85 (65.9) | |||
Tumor volume (cm3) | <0.001 | <0.001 | |||||
≤59.6 | 20 (46.5) | 318 (72.3) | 20 (46.5) | 103 (79.8) | |||
>59.6 | 23 (53.5) | 122 (27.7) | 23 (53.5) | 26 (20.2) | |||
T stage | 0.014 | <0.001 | |||||
T1–3 | 21 (48.8) | 297 (67.5) | 21 (48.8) | 105 (81.4) | |||
T4 | 22 (51.2) | 143 (32.5) | 22 (51.2) | 24 (18.6) | |||
N stage | 0.030 | 0.007 | |||||
N0–1 | 25 (58.1) | 324 (73.6) | 25 (58.1) | 102 (79.1) | |||
N2–3 | 18 (41.9) | 116 (26.4) | 18 (41.9) | 27 (20.9) | |||
Introduction chemotherapy | 0.382 | 0.056 | |||||
No | 29 (67.4) | 324 (73.6) | 29 (67.4) | 105 (81.4) | |||
Yes | 14 (32.6) | 116 (26.4) | 14 (32.6) | 24 (18.6) | |||
Concurrent chemoradiotherapy | 0.311 | 0.365 | |||||
No | 4 (9.3) | 66 (15.0) | 4 (9.3) | 19 (14.7) | |||
Yes | 39 (90.7) | 374 (85.0) | 39 (90.7) | 110 (85.3) | |||
Radiation fields | 0.988 | 0.927 | |||||
IFI | 15 (34.9) | 153 (34.8) | 15 (34.9) | 46 (35.7) | |||
ENI | 28 (65.1) | 287 (65.2) | 28 (65.1) | 83 (64.3) | |||
Total dose (Gy) | <0.001 | 0.158 | |||||
<50 | 5 (11.6) | 5 (1.1) | 5 (11.6) | 5 (3.9) | |||
≥50 to <60 | 26 (60.5) | 304 (69.1) | 26 (60.5) | 89 (69.0) | |||
≥60 | 12 (27.9) | 131 (29.8) | 12 (27.9) | 35 (27.1) | |||
Average single dose (Gy) | 0.004 | 0.008 | |||||
<2 | 5 (11.6) | 44 (10.0) | 5 (11.6) | 11 (8.5) | |||
2 | 16 (37.2) | 75 (17.0) | 16 (37.2) | 21 (16.3) | |||
>2 | 22 (51.2) | 321 (73.0) | 22 (51.2) | 97 (75.2) | |||
Longitudinal length of tumor (cm) | <0.001 | <0.001 | |||||
≤8.4 | 39 (90.7) | 232 (52.7) | 39 (90.7) | 70 (54.3) | |||
>8.4 | 4 (9.3) | 208 (47.3) | 4 (9.3) | 59 (45.7) | |||
Tumor location | 0.342 | 0.274 | |||||
Cervical/upper | 5 (11.6) | 88 (20.0) | 5 (11.6) | 25 (19.4) | |||
Middle | 23 (53.5) | 230 (52.3) | 23 (53.5) | 73 (56.6) | |||
Lower | 15 (34.9) | 122 (27.7) | 15 (34.9) | 31 (24.0) | |||
Stenosis grade | 0.855 | 0.069 | |||||
I–III | 36 (83.7) | 373 (84.8) | 36 (83.7) | 120 (93.0) | |||
IV | 7 (16.3) | 67 (15.2) | 7 (16.3) | 9 (7.0) | |||
Ulcerative type | 0.018 | 0.454 | |||||
No | 42 (97.7) | 371 (84.3) | 42 (97.7) | 120 (93.0) | |||
Yes | 1 (2.3) | 69 (15.7) | 1 (2.3) | 9 (7.0) | |||
Leukocyte count (×10E9/L) | 0.180 | 0.068 | |||||
≤4.0 | 8 (18.6) | 51 (11.6) | 8 (18.6) | 11 (8.5) | |||
>4.0 | 35 (81.4) | 389 (88.4) | 35 (81.4) | 118 (91.5) | |||
Neutrophil count (×10E9/L) | 0.409 | 0.522 | |||||
≤1.8 | 2 (4.7) | 41 (9.3) | 2 (4.7) | 12 (9.3) | |||
>1.8 | 41 (95.3) | 399 (90.7) | 41 (95.3) | 117 (90.7) | |||
Lymphocyte count (×10E9/L) | 0.390 | 0.133 | |||||
≤1.1 | 15 (34.9) | 126 (28.6) | 15 (34.9) | 30 (23.3) | |||
>1.1 | 28 (65.1) | 314 (71.4) | 28 (65.1) | 99 (76.7) | |||
Hemoglobin level (g/L) | 0.539 | 0.611 | |||||
≤115 | 7 (16.3) | 57 (13.0) | 7 (16.3) | 17 (13.2) | |||
>115 | 36 (83.7) | 383 (87.0) | 36 (83.7) | 112 (86.8) | |||
Platelet count (×10E9/L) | 0.119 | 0.226 | |||||
≤125 | 3 (7.0) | 70 (15.9) | 3 (7.0) | 18 (14.0) | |||
>125 | 40 (93.0) | 370 (84.1) | 40 (93.0) | 111 (86.0) | |||
PNI | <0.001 | <0.001 | |||||
<45 | 17 (39.5) | 69 (15.7) | 17 (39.5) | 16 (12.4) | |||
≥45 | 26 (60.5) | 371 (84.3) | 26 (60.5) | 113 (87.6) | |||
LR | <0.001 | <0.001 | |||||
≤0.234 | 14 (32.6) | 311 (70.7) | 14 (32.6) | 93 (72.1) | |||
>0.234 | 29 (67.4) | 129 (29.3) | 29 (67.4) | 36 (27.9) | |||
NLR | <0.001 | <0.001 | |||||
≤2.500 | 7 (16.3) | 202 (45.9) | 7 (16.3) | 64 (49.6) | |||
>2.500 | 36 (83.7) | 238 (54.1) | 36 (83.7) | 65 (50.4) | |||
HLR | 0.009 | 0.008 | |||||
≤77.045 | 4 (9.3) | 121 (27.5) | 4 (9.3) | 38 (29.5) | |||
>77.045 | 39 (90.7) | 319 (72.5) | 39 (90.7) | 91 (70.5) | |||
PLR | <0.001 | 0.001 | |||||
≤133.685 | 8 (18.6) | 217 (49.3) | 8 (18.6) | 60 (46.5) | |||
>133.685 | 35 (81.4) | 223 (50.7) | 35 (81.4) | 69 (53.5) | |||
Serum albumin level (g/L) | 0.197 | 0.132 | |||||
≤35 | 5 (11.6) | 24 (5.5) | 5 (11.6) | 5 (3.9) | |||
>35 | 38 (88.4) | 416 (94.5) | 38 (88.4) | 124 (96.1) |
P value was obtained using the chi-square or Fischer’s Exact test. PSM, propensity score matching; EF, esophageal fistula; ECOG, Eastern Cooperative Oncology Group; PS, performance status; BMI, body mass index; IFI, involved-field irradiation; ENI, elective nodal prophylactic irradiation; PNI, prognostic nutrition index; LR, lymphocyte rate; NLR, neutrophil to lymphocyte ratio; HLR, hemoglobin to lymphocyte ratio; PLR, platelet to lymphocyte ratio.
Clinical outcomes
The median follow-up time was 24.0 months (range, 1.3–104.9 months). At the end of the follow-up, 5 patients with EF and 64 patients without EF were still alive. The 1-, 2-, and 3-year OS rates were 51.2%, 23.0%, and 14.4% in the EF group and 80.6%, 64.7%, and 54.7% in the non-EF group, respectively. The median OS of all 172 patients included in the analysis was 27.8 months (range, 1.3–104.9 months), and the median OS of the EF group (13.1 months, range, 1.3–65.3 months) was shorter than that of the non-EF group (49.4 months, range, 4.0–104.9 months) (P<0.001; Figure 2A). EF occurred during radiotherapy in 17 of 43 patients (39.5%) and occurred after radiotherapy in 26 (60.5%) patients, while the median time to development of EF after completion of radiotherapy was 9 months. The median OS of patients with EF during radiotherapy (8.1 months) was significantly shorter than that of patients with EF after radiotherapy (17.6 months) (P=0.041; Figure 2B).
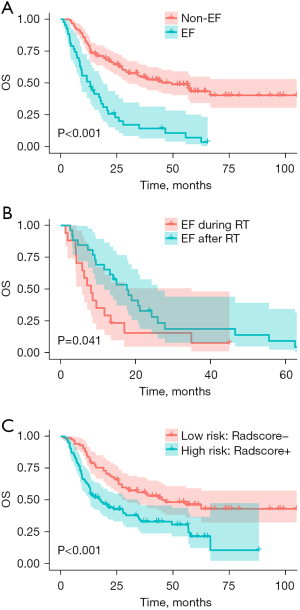
Performance of the clinical nomogram
In the univariate analysis of the clinical variables, the longitudinal length of tumor, tumor axial maximum thickness, tumor volume, T4 stage, N2–3 stage, PNI, LR, NLR, HLR, and PLR were identified as potential factors associated with EF. The multivariate stepwise logistic regression analysis showed that the longitudinal length of tumor (≤8.4 cm), tumor volume (>59.6 cm3), T4 stage, grade IV stenosis, and LR (≤0.234) were independent risk factors (Table 2), which were thus included in the clinical model. The performance of the clinical model based on 100 repetitions of 5-fold cross-validation is shown in Table 3. The AUC and accuracy were 0.849 and 85.45%, respectively. The clinical nomogram based on the clinical model is shown in Figure S3A. The calibration curve in Figure S3B indicates good conformity between the predicted and actual probability for EF. The Brier score of the clinical nomogram was 0.106 (Figure S3C).
Table 2
Characteristics | Univariate analysis | Multivariate analysis | |||||
---|---|---|---|---|---|---|---|
OR | 95% CI | P | OR | 95% CI | P | ||
Gender | |||||||
Male | 1.00 | Reference | |||||
Female | 1.00 | 0.463–2.159 | >0.99 | ||||
Age (years) | |||||||
≤60 | 1.00 | Reference | |||||
>60 | 0.734 | 0.327–1.649 | 0.454 | ||||
ECOG PS | |||||||
≤1 | 1.00 | Reference | |||||
>1 | 1.032 | 0.515–2.068 | 0.929 | ||||
Smoking | |||||||
No | 1.00 | Reference | |||||
Yes | 1.000 | 0.499–2.004 | >0.99 | ||||
Alcohol abuse | |||||||
No | 1.00 | Reference | |||||
Yes | 0.548 | 0.241–1.247 | 0.152 | ||||
Diabetes | |||||||
No | 1.00 | Reference | |||||
Yes | 1.860 | 0.426–8.131 | 0.410 | ||||
Hypertension | |||||||
No | 1.00 | Reference | |||||
Yes | 0.824 | 0.344–1.977 | 0.665 | ||||
BMI (kg/m2) | |||||||
<18.5 | 1.00 | Reference | |||||
18.5–23.9 | 0.922 | 0.305–2.790 | 0.886 | ||||
24–27.9 | 1.008 | 0.282–3.604 | 0.990 | ||||
≥28 | 0.560 | 0.052–6.036 | 0.633 | ||||
Tumor axial maximum thickness (cm) | |||||||
≤2.5 | 1.00 | Reference | |||||
>2.5 | 3.934 | 1.446–10.704 | 0.007 | ||||
Tumor volume (cm3) | |||||||
≤59.6 | 1.00 | Reference | |||||
>59.6 | 4.556 | 2.179–9.526 | <0.001 | 8.212 | 2.830–23.826 | <0.001 | |
T stage | |||||||
T1–3 | 1.00 | Reference | |||||
T4 | 4.583 | 2.177–9.649 | <0.001 | 4.387 | 1.664–11.569 | 0.003 | |
N stage | |||||||
N0–1 | 1.00 | Reference | |||||
N2–3 | 2.720 | 1.298–5.699 | 0.008 | ||||
Initial Introduction chemotherapy | |||||||
No | 1.00 | Reference | |||||
Yes | 2.112 | 0.971–4.593 | 0.059 | ||||
Concurrent chemoradiotherapy | |||||||
No | 1.00 | Reference | |||||
Yes | 1.684 | 0.539–5.257 | 0.370 | ||||
Radiation field | |||||||
IFI | 1.00 | Reference | |||||
ENI | 1.035 | 0.502–2.132 | 0.927 | ||||
Total dose (Gy) | |||||||
<50 | 1.00 | Reference | |||||
≥50 to <60 | 0.292 | 0.078–1.087 | 0.067 | ||||
≥60 | 0.343 | 0.084–1.394 | 0.135 | ||||
Average single dose (Gy) | |||||||
<2 | 1.00 | Reference | |||||
2 | 1.676 | 0.484–5.799 | 0.415 | ||||
>2 | 0.499 | 0.157–1.582 | 0.238 | ||||
Longitudinal length of tumor (cm) | |||||||
≤8.4 | 1.00 | Reference | |||||
>8.4 | 0.122 | 0.041–0.360 | <0.001 | 0.049 | 0.012–0.202 | <0.001 | |
Tumor location | |||||||
Cervical/upper | 1.00 | Reference | |||||
Middle | 1.575 | 0.541–4.586 | 0.404 | ||||
Lower | 2.419 | 0.773–7.573 | 0.129 | ||||
Stenosis grade | |||||||
I–III | 1.00 | Reference | |||||
IV | 2.593 | 0.902–7.450 | 0.077 | 4.124 | 1.071–15.872 | 0.039 | |
Ulcerative type | |||||||
No | 1.00 | Reference | |||||
Yes | 0.371 | 0.039–2.581 | 0.283 | ||||
Leukocyte count (×10E9/L) | |||||||
≤4.0 | 1.00 | Reference | 0.075 | ||||
>4.0 | 0.408 | 0.152–1.093 | |||||
Neutrophil count (×10E9/L) | |||||||
≤1.8 | 1.00 | Reference | 0.344 | ||||
>1.8 | 2.103 | 0.451–9.794 | |||||
Lymphocyte count (×10E9/L) | |||||||
≤1.1 | 1.00 | Reference | 0.136 | ||||
>1.1 | 0.566 | 0.268–1.196 | |||||
Hemoglobin level (g/L) | |||||||
≤115 | 1.00 | Reference | 0.612 | ||||
>115 | 0.781 | 0.300–2.033 | |||||
Platelet count (×10E9/L) | |||||||
≤125 | 1.00 | Reference | 0.236 | ||||
>125 | 2.162 | 0.604–7.734 | |||||
PNI | |||||||
<45 | 1.00 | Reference | <0.001 | ||||
≥45 | 0.217 | 0.097–0.484 | |||||
LR | |||||||
≤0.234 | 1.00 | Reference | |||||
>0.234 | 0.209 | 0.100–0.439 | <0.001 | 0.213 | 0.084–0.537 | 0.001 | |
NLR | |||||||
≤2.500 | 1.00 | Reference | |||||
>2.500 | 5.064 | 2.100–12.210 | <0.001 | ||||
HLR | |||||||
≤77.045 | 1.00 | Reference | |||||
>77.045 | 4.071 | 1.360–12.188 | 0.012 | ||||
PLR | |||||||
≤133.685 | 1.00 | Reference | |||||
>133.685 | 3.804 | 1.638–8.833 | 0.002 | ||||
Serum albumin level (g/L) | |||||||
≤35 | 1.00 | Reference | |||||
>35 | 0.306 | 0.084–1.115 | 0.073 |
OR, odds ratio; CI, confidence interval; ECOG, Eastern Cooperative Oncology Group; PS, performance status; BMI, body mass index; IFI, involved-field irradiation; ENI, elective nodal prophylactic irradiation; PNI, prognostic nutrition index; LR, lymphocyte rate; NLR, neutrophil to lymphocyte ratio; HLR, hemoglobin to lymphocyte ratio; PLR, platelet to lymphocyte ratio.
Table 3
Metric | Clinical model | Radiomics signature | Clinical-radiomics model |
---|---|---|---|
AUC | 0.849±0.008 | 0.794±0.006 | 0.896±0.009 |
Accuracy (%) | 85.45±1.30 | 81.40±0.77 | 87.72±0.88 |
PPV (%) | 77.12±4.06 | 74.51±2.82 | 77.84±2.21 |
NPV (%) | 87.49±0.86 | 82.44±0.57 | 90.66±0.71 |
Sensitivity (%) | 59.65±3.12 | 38.91±2.33 | 71.16±2.38 |
Specificity (%) | 94.05±1.36 | 95.56±0.58 | 93.23±0.82 |
AUC, area under the receiver operating characteristic curve; PPV, positive predictive value; NPV, negative predictive value.
Performance of the radiomics signature
The eight radiomics features with the best performance in predicting EF were selected by LASSO for calculating the radiomics signature (Radscore) (Table S2 and Figure S1). The formula for calculating the Radscore is as follows:
The distribution of the radiomics signature for each patient is shown in Figure S2. The performance of the radiomics signature based on 100 repetitions of 5-fold cross-validation is shown in Table 3. The AUC and accuracy were 0.794 and 81.40%, respectively. The median of Radscore (−1.245) was used to divide patients into high- (Radscore+) and low-risk (Radscore−) groups. The low-risk group had a significantly better median OS (46.6 months) than did the high-risk group (17.6 months) (P<0.001; Figure 2C).
Performance of the clinical-radiomics nomogram
Based on multivariate logistic regression analysis, a clinical-radiomics nomogram was constructed from the combination of clinical factors and the radiomics signature (Figure 3A). The performance of the clinical-radiomics model based on 100 repetitions of 5-fold cross-validation is shown in Table 3. The AUC and accuracy were 0.896 and 87.72%, respectively, and were higher than those of the clinical nomogram and radiomics signature. As shown in Figure 3B, the calibration plot showed good conformity between the predicted and actual probability for EF. The Brier score of the clinical-radiomics nomogram was 0.088, which was much closer to 0 than was the clinical nomogram (0.106), indicating better predictive ability (Figure 3C). The ROC curves are shown in Figure 4A. Finally, we performed a decision curve analysis to evaluate the clinical utility of the models, and their effective threshold ranged from approximately 10% to 75% (Figure 4B), indicating that using these models is more effective than is the “treat-all” or the “treat-none” strategy and that the clinical-radiomics nomogram was the most effective in predicting EF when the prediction probability was within this range.
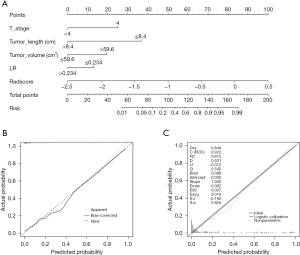
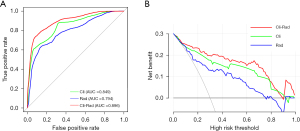
Discussion
EF is one of the serious adverse events that can occur during or after radiotherapy in patients with EC. Direct radiation therapy might not the optimal treatment choice for patients with locally advanced ESCC who had higher risk of developing EF. For these patients, treatment options included initial systemic chemotherapy or immunotherapy to reduce tumor volume before undergoing curative chemoradiotherapy or placement of a nasojejunal feeding tube to facilitate radiation therapy.
Risk prediction models of EF in EC have been established in several studies (9,27), but incorporated clinical parameters alone. Previous studies has shown the potential of CT radiomics in predicting the risk of EF and the prognosis of patients with EC. Xu et al. (26) developed a deep learning model to integrate CT imaging features and clinical factors for predicting EF caused by the tumor itself and treatment in patients with EC, which achieved a concordance index of 0.901 in the validation set. Zhu et al. (25) constructed nomograms incorporating independent clinical risk factors and a radiomics signature to predict the prognosis of malignant EF. However, in some studies (25,26) a prediction model for EF was not established as only some patients underwent radiotherapy while in others the complexity and poor interpretability of radiomics algorithms limited their worldwide application (9,27). To overcome these limitations, for the first time we developed a predictive model for EF in patients with ESCC underwent IMRT or VMAT using CT-based radiomics associated with clinical parameters.
Our results showed the longitudinal length of tumor, tumor volume, T4 stage, grade IV stenosis, and LR were significant prognostic factors for EF. The radiomics signature could separate patients into high- and low-risk groups in terms of different OS. Furthermore, the combination model of clinical and radiomics parameters showed a better prediction performance and accuracy with an AUC value of 0.896. The results also showed that the radiomics signature could provide reference value for predicting the OS of patients with EF.
Patients who developed EF during radiotherapy had worse survival than did those who developed EF after radiotherapy. This might be related to the deterioration of nutritional status caused by fistula during radiotherapy or tumor progression during radiotherapy. Several studies have investigated the potential factors associated with radiotherapy-related EF, including tumor- and patient-related factors (4,9,10,13,27). Pao et al. reported that baseline T4 and esophageal stenosis may also be risk factors for EF in patients with ESCC treated with definitive concurrent chemoradiotherapy of IMRT (11). Similarly, our study found T4 to be highly predictive of EF in patients who received radiotherapy. It is likely that the T4 stage represents a tumor that directly invades surrounding normal tissues and organs, with the repair of normal tissues being unable to compensate for the shrinkage of the tumor during radiotherapy, which results in the occurrence of EF. Our results suggested that tumor volume, the longitudinal length of tumor, and LR also play important roles in the formation of EF. These are similar to the risk factors for developing EF investigated in patients with conventional radiotherapy and three-dimensional conformal radiotherapy (32). The correlation between modern radiotherapy techniques and the occurrence of EF needs to be further validated.
There is no consensus regarding the classification and definition of esophageal stenosis. Kawakami et al. (7) and Pao et al. (11) consider esophageal stenosis to be present when the endoscope cannot pass through the lesion, and Hu et al. (14) describe a means to determining the grade of esophageal stenosis involving measuring the internal diameter at the local stricture segment on a barium esophagram. However, these methods are either complicated in manipulation or have not been reproduced and validated. Gui et al. (27) classified the quantification of esophageal stenosis into four grades and found that not all esophageal stenosis events were associated with EF formation. Only grade IV esophageal stenosis was a high-risk factor for EF formation (27), which is consistent with our findings. Taniyama et al. (8) and Hu et al. (14) reported the axis of a tumor thickness on CT images to be associated with the formation of EF. Our study also showed that in the univariate analysis, the tumor axial thickness was associated with the formation of EF. However, the longitudinal length of tumor and tumor volume might be more predictive factors for EF than tumor thickness. Larger tumor size indicates greater local tumor burden, which may cause more severe local normal tissue damage. Previous studies suggest that that ulcer type is related to the formation of EF (10,13,14,16). However, in our study, the results of ulcer type were not significant, which may be related to the fact that our ulcer determination was only based on barium meal examination and the proportion of ulcerative tumor patients was too low, resulting in data bias.
Other studies have reported that high PLR is associated with the occurrence of EF (27,33). Our univariate analysis results indicated that PLR was related to the formation of EF, but PLR did not show significance in the multivariate analyses analysis. The value of PLR in predicting EF formation and the determination of threshold still needs to be validated with additional data. Wang et al. (13) found absolute lymphocyte count to be associated with the occurrence of EF. However, in our results, absolute lymphocyte count was not associated with EF, and EF was more likely to occur when the LR was lower than 0.234. A decrease in LR may represent a decrease in the patient’s immune function and a decline in the patient’s normal tissue repair function, thus resulting in a higher incidence of EF. Xue et al. (34) reported that PNI was an independent prognostic factor for EC, and the survival of patients with EC with low PNI was worse; however, in our study, PNI demonstrated no clear value in predicting EF. Some studies have shown that the continuous improvement of nutritional status during treatment is significantly related to a low incidence of EF (32,35). Due to a lack of historical data, the changes of nutritional status and indicators during radiotherapy for EF were not analyzed in this study. The nutritional score and nutritional improvement of patients with EC may have a consistent relationship with the formation of EF, but further research is still needed to verify this association.
This study has several limitations. First, its retrospective, single-center design, and small sample size might have introduced a degree of selection bias. Second, the clinical-radiomics nomogram model was not verified by external data, and further validation in a multicenter study is needed to determine its clinical applicability. Third, despite the slight performance improvement brought by incorporating radiomics into the clinical model, the clinical-radiomics model did not offer a significant advantage over the clinical model. This may be due to our insufficient exploration and analysis of imaging information. In the future, we will utilize other artificial intelligence technologies to delve deeper into mining imaging information, with the hope of achieving higher performance improvement.
Conclusions
In this study, we developed a clinical-radiomics model for predicting EF in ESCC. The nomogram may be valuable for identifying patients who are high-risk for the development of an EF and would then benefit from individualized treatment that omits radiotherapy.
Acknowledgments
We are grateful to all the participants in this study.
Funding: This study was supported by
Footnote
Reporting Checklist: The authors have completed the TRIPOD reporting checklist. Available at https://jtd.amegroups.com/article/view/10.21037/jtd-24-191/rc
Data Sharing Statement: Available at https://jtd.amegroups.com/article/view/10.21037/jtd-24-191/dss
Peer Review File: Available at https://jtd.amegroups.com/article/view/10.21037/jtd-24-191/prf
Conflicts of Interest: All authors have completed the ICMJE uniform disclosure form (available at https://jtd.amegroups.com/article/view/10.21037/jtd-24-191/coif). The authors have no conflicts of interest to declare.
Ethical Statement: The authors are accountable for all aspects of the work in ensuring that questions related to the accuracy or integrity of any part of the work are appropriately investigated and resolved. The study was conducted in accordance with the Declaration of Helsinki (as revised in 2013), and it was approved by the ethics committee of Xijing Hospital (No. KT20172035-1). Informed consent was waived due to the retrospective nature of the study.
Open Access Statement: This is an Open Access article distributed in accordance with the Creative Commons Attribution-NonCommercial-NoDerivs 4.0 International License (CC BY-NC-ND 4.0), which permits the non-commercial replication and distribution of the article with the strict proviso that no changes or edits are made and the original work is properly cited (including links to both the formal publication through the relevant DOI and the license). See: https://creativecommons.org/licenses/by-nc-nd/4.0/.
References
- Zheng RS, Zhang SW, Sun KX, et al. Cancer statistics in China, 2016. Zhonghua Zhong Liu Za Zhi 2023;45:212-20. [Crossref] [PubMed]
- He Y, Li D, Shan B, et al. Incidence and mortality of esophagus cancer in China, 2008-2012. Chin J Cancer Res 2019;31:426-34. [Crossref] [PubMed]
- Ng J, Lee P. The Role of Radiotherapy in Localized Esophageal and Gastric Cancer. Hematol Oncol Clin North Am 2017;31:453-68. [Crossref] [PubMed]
- Shinoda M, Ando N, Kato K, et al. Randomized study of low-dose versus standard-dose chemoradiotherapy for unresectable esophageal squamous cell carcinoma (JCOG0303). Cancer Sci 2015;106:407-12. [Crossref] [PubMed]
- Hihara J, Hamai Y, Emi M, et al. Role of definitive chemoradiotherapy using docetaxel and 5-fluorouracil in patients with unresectable locally advanced esophageal squamous cell carcinoma: a phase II study. Dis Esophagus 2016;29:1115-20. [Crossref] [PubMed]
- Sun X, Han S, Gu F, et al. A Retrospective Comparison of Taxane and Fluorouracil-based Chemoradiotherapy in Patients with Inoperable Esophageal Squamous Cell Carcinoma. J Cancer 2016;7:1066-73. [Crossref] [PubMed]
- Kawakami T, Tsushima T, Omae K, et al. Risk factors for esophageal fistula in thoracic esophageal squamous cell carcinoma invading adjacent organs treated with definitive chemoradiotherapy: a monocentric case-control study. BMC Cancer 2018;18:573. [Crossref] [PubMed]
- Taniyama TK, Tsuda T, Miyakawa K, et al. Analysis of fistula formation of T4 esophageal cancer patients treated by chemoradiotherapy. Esophagus 2020;17:67-73. [Crossref] [PubMed]
- Xu Y, Wang L, He B, et al. Development and validation of a risk prediction model for radiotherapy-related esophageal fistula in esophageal cancer. Radiat Oncol 2019;14:181. [Crossref] [PubMed]
- Chen B, Deng M, Yang C, et al. High incidence of esophageal fistula on patients with clinical T4b esophageal squamous cell carcinoma who received chemoradiotherapy: A retrospective analysis. Radiother Oncol 2021;158:191-9. [Crossref] [PubMed]
- Pao TH, Chen YY, Chang WL, et al. Esophageal fistula after definitive concurrent chemotherapy and intensity modulated radiotherapy for esophageal squamous cell carcinoma. PLoS One 2021;16:e0251811. [Crossref] [PubMed]
- Chen HY, Ma XM, Ye M, et al. Esophageal perforation during or after conformal radiotherapy for esophageal carcinoma. J Radiat Res 2014;55:940-7. [Crossref] [PubMed]
- Wang S, Zhang C, Wang Y, et al. Risk factors and prognosis for esophageal fistula in patients with esophageal squamous cell carcinoma during radiotherapy. Esophagus 2022;19:660-9. [Crossref] [PubMed]
- Hu B, Jia F, Zhou H, et al. Risk Factors Associated with Esophageal Fistula after Radiotherapy for Esophageal Squamous Cell Carcinoma. J Cancer 2020;11:3693-700. [Crossref] [PubMed]
- Tsushima T, Mizusawa J, Sudo K, et al. Risk Factors for Esophageal Fistula Associated With Chemoradiotherapy for Locally Advanced Unresectable Esophageal Cancer: A Supplementary Analysis of JCOG0303. Medicine (Baltimore) 2016;95:e3699. [Crossref] [PubMed]
- Zhu C, Wang S, You Y, et al. Risk Factors for Esophageal Fistula in Esophageal Cancer Patients Treated with Radiotherapy: A Systematic Review and Meta-Analysis. Oncol Res Treat 2020;43:34-41. [Crossref] [PubMed]
- Guan X, Liu C, Zhou T, et al. Survival and prognostic factors of patients with esophageal fistula in advanced esophageal squamous cell carcinoma. Biosci Rep 2020;40:BSR20193379. [Crossref] [PubMed]
- Bera K, Braman N, Gupta A, et al. Predicting cancer outcomes with radiomics and artificial intelligence in radiology. Nat Rev Clin Oncol 2022;19:132-46. [Crossref] [PubMed]
- Guiot J, Vaidyanathan A, Deprez L, et al. A review in radiomics: Making personalized medicine a reality via routine imaging. Med Res Rev 2022;42:426-40. [Crossref] [PubMed]
- Larue RTHM, Klaassen R, Jochems A, et al. Pre-treatment CT radiomics to predict 3-year overall survival following chemoradiotherapy of esophageal cancer. Acta Oncol 2018;57:1475-81. [Crossref] [PubMed]
- Gong J, Zhang W, Huang W, et al. CT-based radiomics nomogram may predict local recurrence-free survival in esophageal cancer patients receiving definitive chemoradiation or radiotherapy: A multicenter study. Radiother Oncol 2022;174:8-15. [Crossref] [PubMed]
- Zhu C, Mu F, Wang S, et al. Prediction of distant metastasis in esophageal cancer using a radiomics-clinical model. Eur J Med Res 2022;27:272. [Crossref] [PubMed]
- Wang J, Yu X, Zeng J, et al. Radiomics model for preoperative prediction of 3-year survival-based CT image biomarkers in esophageal cancer. Eur Arch Otorhinolaryngol 2022;279:5433-43. [Crossref] [PubMed]
- Cui Y, Li Z, Xiang M, et al. Machine learning models predict overall survival and progression free survival of non-surgical esophageal cancer patients with chemoradiotherapy based on CT image radiomics signatures. Radiat Oncol 2022;17:212. [Crossref] [PubMed]
- Zhu C, Ding J, Wang S, et al. Development and validation of a prognostic nomogram for malignant esophageal fistula based on radiomics and clinical factors. Thorac Cancer 2021;12:3110-20. [Crossref] [PubMed]
- Xu Y, Cui H, Dong T, et al. Integrating Clinical Data and Attentional CT Imaging Features for Esophageal Fistula Prediction in Esophageal Cancer. Front Oncol 2021;11:688706. [Crossref] [PubMed]
- Gui Z, Liu H, Shi W, et al. A Nomogram for Predicting the Risk of Radiotherapy-Related Esophageal Fistula in Esophageal Cancer Patients. Front Oncol 2021;11:785850. [Crossref] [PubMed]
- Zwanenburg A, Vallières M, Abdalah MA, et al. The Image Biomarker Standardization Initiative: Standardized Quantitative Radiomics for High-Throughput Image-based Phenotyping. Radiology 2020;295:328-38. [Crossref] [PubMed]
- Zhang B, Tian J, Dong D, et al. Radiomics Features of Multiparametric MRI as Novel Prognostic Factors in Advanced Nasopharyngeal Carcinoma. Clin Cancer Res 2017;23:4259-69. [Crossref] [PubMed]
- Katzman JL, Shaham U, Cloninger A, et al. DeepSurv: personalized treatment recommender system using a Cox proportional hazards deep neural network. BMC Med Res Methodol 2018;18:24. [Crossref] [PubMed]
- Liu Z, Zhang XY, Shi YJ, et al. Radiomics Analysis for Evaluation of Pathological Complete Response to Neoadjuvant Chemoradiotherapy in Locally Advanced Rectal Cancer. Clin Cancer Res 2017;23:7253-62. [Crossref] [PubMed]
- Watanabe S, Ogino I, Kunisaki C, et al. Relationship between nutritional status and esophageal fistula formation after radiotherapy for esophageal cancer. Cancer Radiother 2019;23:222-7. [Crossref] [PubMed]
- Han D, Zhang J, Zhao J, et al. Platelet-to-lymphocyte ratio is an independent predictor of chemoradiotherapy-related esophageal fistula in esophageal cancer patients. Ann Transl Med 2020;8:1163. [Crossref] [PubMed]
- Xue Y, Zhou X, Xue L, et al. The role of pretreatment prognostic nutritional index in esophageal cancer: A meta-analysis. J Cell Physiol 2019;234:19655-62. [Crossref] [PubMed]
- Ma L, Luo GY, Ren YF, et al. Concurrent chemoradiotherapy combined with enteral nutrition support: a radical treatment strategy for esophageal squamous cell carcinoma patients with malignant fistulae. Chin J Cancer 2017;36:8. [Crossref] [PubMed]