Development and validation of a nomogram superior to CHADS2 and CHA2DS2-VASc models for predicting left atrial appendage dense spontaneous echo contrast/left atrial appendage thrombus
Highlight box
Key findings
• We constructed a nomogram to accurately predict left atrial appendage (LAA) dense spontaneous echo contrast (SEC) or LAA thrombus (LAAT).
What is known and what is new?
• 90% of atrial fibrillation (AF) related strokes were caused by the detachment of LAAT. Female gender, left ventricular ejection fraction (LVEF), left atrial ejection fraction (LAEF) can be used to predict LAA dense SEC or LAAT.
• Left atrial reservoir strain rate (LASRS) also has an important value in predicting LAA dense SEC or LAAT. The nomogram, which included indicators of female gender, D-dimer level, LVEF, LAEF, and LASRS, had a higher clinical value in predicting LAA SEC/LAAT than traditional prediction models.
What is the implication, and what should change now?
• We can accurately identify patients at high risk of stroke with AF through non-invasive examination means, thereby reducing the pain caused by transesophageal cardiac ultrasound. We need a larger sample size and external validation population to further verify the application value of the model.
Introduction
Atrial fibrillation (AF) is clinically one of the most frequently occurring arrhythmias, and due to the aging population of the planet, is increasing in incidence annually on a global scale. It has been reported that by 2020, the total number of patients with AF worldwide may be as high as 50 million (1,2). In patients with AF, orderly atrial contractions are transited into rapid and disordered AF, and in addition to that of palpitations, the incidences of stroke and heart failure in patients with AF are also markedly higher (3). In particular, AF-associated stroke has garnered widespread attention owing to its high fatality and disability rates (4).
It has been reported that patients with AF have a 4- to 5-fold increased risk of stroke (5). Therefore, the prevention of stroke is a key concern in AF treatment (6). At present, the major preventive measure against AF-associated stroke is anticoagulant therapy, yet the application of anticoagulant drugs can also cause an increase in bleeding events (7). Therefore, it is particularly crucial to identify those patients with AF at high risk of stroke and offer targeted anticoagulation therapy for these patients. In current guideline recommendations and clinical practice, the CHA2DS2-VASc (congestive heart failure or left ventricular dysfunction hypertension, age ≥75 years, diabetes, stroke, vascular disease, age 65–74 years, sex) model is the main stroke prediction model (6). In the CHA2DS2-VASc model, population characteristics and medical history serve as the principal variables, making it amenable to clinical application. However, many studies have also shown the limited predictive value of this model (8,9). Detachment of left atrial appendage thrombus (LAAT) is known to be the cause of stroke occurring in patients with AF, while thrombosis is not only associated with systemic factors but also highly relevant to the local hemodynamics of the heart (10). These left atrial function variables are not included in the CHA2DS2-VASc predictive model, which may partly account for its low predictive performance.
Patients with AF exhibit local hemodynamic changes in the left atrium, manifesting as a slower local blood flow velocity and blood stasis. As an attachment structure of the left atrium, the left atrial appendage (LAA) is often more prone to blood stasis and thrombosis, which also underlies the high risk of stroke in patients with AF (11). Currently, patients with AF and LAA dense spontaneous echo contrast (SEC) and LAAT are often regarded as a high-risk population for stroke, and these factors adopted in the relevant guidelines (6). It is recommended that patients without sufficient anticoagulation therapy, such as those in need of emergency restoration of sinus rhythm, should undergo transesophageal echocardiography (TEE) and other examinations to exclude LAAT (12).
With the advancement of echocardiography (ECG), ultrasound speckle tracking technology has been extensively applied in clinical practise, displaying high application value in cardiac function assessment (13). A related study has shown that extensive left atrial strain rate can be used for left atrial function assessment and that left atrial strain can be used to predict SEC and LAAT in clinical practise (14). However, the study is limited to examining the relationship between ECG variables and LAA dense SEC or LAAT, with other clinical data rarely being included.
In this study, we selected patients with nonvalvular atrial fibrillation (NVAF) as the participants, with those with LAA dense SEC or LAAT being considered the high-risk population for AF stroke. Based on medical history data, clinical baseline data, and ECG variables, among other factors. We constructed a clinical prediction model for LAA dense SEC/LAAT. This prediction model, encompassing both structural variables of the left atrium and variables reflecting left atrial function, which are relatively clinically accessible, was expected to identify those patients with AF at high risk of stroke. We present this article in accordance with the TRIPOD reporting checklist (available at https://jtd.amegroups.com/article/view/10.21037/jtd-24-288/rc).
Methods
Study participants
We enrolled patients with NVAF who underwent TEE examination at the First Affiliated Hospital of Soochow University between October 2019 and July 2022. The sample size was not calculated beforehand and this was a convenience sample. Depending on the presence or absence of dense SEC/LAAT in TEE imaging, patients were assigned into a dense SEC/LAAT group or a non-dense SEC/LAAT group. The inclusion criteria were (I) patients with NVAF and (II) those aged over 18 years. Meanwhile, the exclusion criteria were the following: (I) congenital heart disease; (II) hypertrophic cardiomyopathy; (III) myocardial amyloidosis; and (IV) unclear ECG data. The study was conducted in accordance with the Declaration of Helsinki (as revised in 2013). This study was granted by the Ethics Committee of the First Affiliated Hospital of Soochow University (ethics approval No. 2024-010). Individual consent for this retrospective analysis was waived.
Clinical data acquisition
Data on age, gender, body mass index (BMI), medical history including smoking, drinking, and the presence or absence of anticoagulant therapy were recorded.
Comorbidities included hypertension, stroke, transient ischemic attack, systemic embolism, diabetes, congestive heart failure (CHF), vascular diseases (coronary heart disease, old myocardial infarction, peripheral vascular stenosis of more than 50%, aortic plaque), and persistent AF. The diagnosis of the aforementioned diseases complied with the relevant guidelines (6,15-17). The data from laboratory tests were also collected and included platelet count, D-dimer level, serum creatinine, and hemoglobin. And whether patients received adequate anticoagulant therapy was also collected. All laboratory tests were conducted by taking peripheral venous blood in the morning on an empty stomach. Moreover, the CHADS2 and CHA2DS2-VASc scores were computed for all cases.
Transthoracic ECG
ECG was implemented for all participants with a Vivid E9 or Vivid E95 device (GE HealthCare, California, USA) and the assistance of a 2.5-MHz transducer. Left ventricular ejection fraction (LVEF) was assessed with Simpson’s biplane method. The Simpson’s biplane method was used to gain the maximum left atrial volume (LAVmax) and minimum left atrial volume (LAVmin) in apical two- and four-chamber views, and the maximum left atrial volume index (LAVimax) and minimum left atrial volume index (LAVimin) were exponentially standardized based on body surface area. Accordingly, the total left atrial ejection fraction (LAEF) was computed.
Two-dimensional speckle tracking ECG
The optimized apical four- and two-chamber views of the left atrium were subjected to offline analysis using EchoPAC software version 201 (GE HealthCare). With the left ventricular end-diastolic as the zero reference point for left atrial strain, this study measured the left atrial reservoir strain rate (LASRS) and left atrial strain rate during rapid ventricular filling (LASRE) in apical four- and two-chamber views during three cardiac cycles. Left atrial reservoir strain (LASR), LASRS, and LASRE measured in the apical four- and two-chamber views were averaged for analysis.
TEE examination
Fasting for at least 6 hours before TEE, ECG and TEE were performed at the same time. TEE examination was carried out for all study participants on GE Vivid E9 or GE Vivid E95 devices (GE HealthCare) with the assistance of a multiplane 6VT (3.0–8.0 MHz) probe. The condition of the LAA was assessed from multiple angles (at least 45°, 60°, 90°, and 135° views of the middle segment of the esophagus).
SEC/LAAT definition and classification
SEC was defined as follows: despite the gain adjustment, dynamic smoke-like echoes could still observed with a slowly swirling motion in the LAA and left atrium. In compliance with the classification criterion proposed by Fatkin et al., the severity of SEC was graded from 0 to 4 (18). Meanwhile, LAAT was defined as follows: sharply demarcated, mass-like substantial echo images detected in the LAA.
Statistical analysis
If the proportion of missing data was less than 5%, the data were imputed via multiple interpolation, and if the proportion of missing data was greater than 5%, the related data were deleted. Normally distributed continuous variables are reported as the mean ± standard deviation; otherwise, they are represented as the median with interquartile range (IQR). Categorical variables are expressed as frequencies (%). For normally distributed data, differences between the two groups were examined through an independent Student t-test; otherwise, the Mann-Whitney test was used. The binary variables were compared with the chi-squared test and Fisher exact test. Given the collinearity between variables and the small sample size of the dense SEC/LAAT group, we initially applied least absolute shrinkage and selection operator (LASSO) regression for variable screening and then conducted collinearity tests on the screened variables. Subsequently, stepwise regression was used to further screen the significant variables in the LASSO regression analysis. On the strength of the stepwise regression analysis results, a nomogram was generated with the assistance of the “rms” package in the R 4.1 version (http://www.r-project.org; The Foundation for Statical Computing). The nomogram proportionally converted each regression coefficient in multiple logistic regression to a scale of 0 to 100, and the impact of the variable with the highest coefficient β was scored as 100 points. The sum of these scores (total score) was generated and then converted into the probability of predicting dense LAAT/SEC. The calibration curves (500 bootstrap samples) were employed to reduce overfitting and substantiate the calibration of the model. Its clinical utility was additionally defined with decision curve analysis (DCA) to evaluate the performance differences between our nomogram and that of other models. The consistency statistic (C statistic) was computed to evaluate the predictive capability of the nomogram. Additionally, its discrimination ability was assessed through the receiver operating characteristic (ROC) curve analysis. The area under the ROC curve (AUC) was calculated. A comparison of the predictive performance between our model (nomogram) and that of other classification models was implemented. Integrated discrimination improvement (IDI) and net reclassification improvement (NRI) were adopted for evaluating the reclassification ability of the nomogram. The procedures for model construction are detailed in Figure 1.
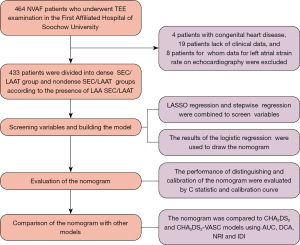
Results
Baseline characteristics of the dense SEC/LAAT and non-dense SEC/LAAT groups
In the study, 464 patients with NVAF were enrolled. After eliminating 4 cases of congenital heart disease, 19 cases without complete clinical data, and 8 patients for whom left atrial strain data on ECG images could not be obtained, we enrolled 433 patients. These patients were assigned into the dense SEC/LAAT group or non-dense SEC/LAAT group according to the presence or absence of LAA dense SEC/LAAT. There were 116 cases in the dense SEC/LAAT group, accounting for 26.8% of the enrolled patients. Among them, 55 patients were female and the median age was 67.0 (IQR, 62.0–72.0) years old. Representative TEE images and a left atrial strain rate diagram for patients in the non-dense SEC/LAAT group are depicted in Figure 2A,2B, respectively, while those for patients in the dense SEC/LAAT group are presented in Figure 2C,2D, respectively.
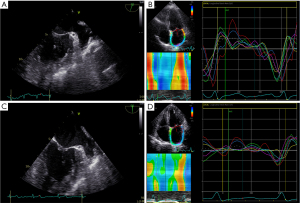
Table 1 displays the data on medical history, laboratory test results, and ECG variables of patients in both groups. Compared with the non-dense SEC/LAAT group, the dense SEC/LAAT group tended to have a higher proportion of older patients, females, and patients with persistent AF, diabetes, or CHF. Higher CHADS2 and CHA2DS2-VASc scores were found in the dense SEC/LAAT group as compared to the non-dense SEC/LAAT group. Laboratory test results showed a higher D-dimer level in the dense SEC/LAAT group versus the non-dense SEC/LAAT group. According to the on the ECG images, higher values of right axis deviation (RAD), right arterial pressure (RAP), left ventricular diastolic dysfunction (LVDD), peak mitral valve flow velocity during early ventricular diastole (E), ratio of E to peak mitral ring motion velocity during early ventricular diastole (E/e'), left axis deviation (LAD), LAVmax, LAVImax, and LAVI but lower values of LVEF, E-wave deceleration time (EDT), LAEF, LASRS, and LASRE were noted in the dense SEC/LAAT group relative to the nondense SEC/LAAT group.
Table 1
Variable | Non-dense SEC/LAAT (n=317) | Dense SEC/LAAT (n=116) | P value |
---|---|---|---|
Demographics | |||
Age (years), median [IQR] | 65.0 [58.0–69.0] | 67.0 [62.0–72.0] | 0.001 |
Female gender, n (%) | 101 (31.9) | 55 (47.4) | 0.003 |
BMI (kg/m2), median [IQR] | 24.8 [23.0–27.3] | 24.4 [23.1–26.6] | 0.12 |
Medical history, n (%) | |||
Smoking | 49 (15.5) | 27 (23.3) | 0.06 |
Drinking | 52 (16.4) | 20 17.2) | 0.88 |
Persistent AF | 156 (49.2) | 109 (94.0) | <0.01 |
Anticoagulants | 158 (49.8) | 67 (57.8) | 0.14 |
Comorbidity, n (%) | |||
HTN | 178 (56.2) | 77 (66.4) | 0.06 |
DM | 38 (12.0) | 26 (22.4) | 0.009 |
CHF | 17 (5.4) | 25 (21.6) | <0.01 |
TIA/stroke/embolism | 27 (8.5) | 11 (9.5) | 0.75 |
Vascular disease | 23 (7.3) | 10 (8.6) | 0.64 |
Model, median [IQR] | |||
CHADS2 | 1 [0–1] | 1 [1–2] | <0.01 |
CHA2DS2-VASc | 2 [1–3] | 2 [2–4] | <0.01 |
Laboratory tests | |||
Hemoglobin (g/L), mean ± SD | 139.5±16.7 | 140.5±15.5 | 0.57 |
Cr (μmmol/L), median [IQR] | 68.1 [58.4–78.4] | 69.0 [59.0–82.7] | 0.72 |
PLT (109/L), median [IQR] | 177 [144–214] | 169 [137–201] | 0.10 |
D-dimer (μg/mL), median [IQR] | 0.22 [0.14–0.32] | 0.28 [0.22–0.62] | <0.01 |
Echocardiography | |||
RAD (mm), median [IQR] | 39.0 [35.0–44.0] | 43.0 [38.0–48.0] | <0.01 |
mPAP (mmHg), median [IQR] | 26 [22.0–30.0] | 29.0 [24.0–36.0] | <0.01 |
IVSth (mm), median [IQR] | 9.0 [9.0–10.0] | 9.0 [9.0–10.0] | 0.89 |
LVDD (mm), mean ± SD | 49.4±4.6 | 51.8±6.5 | <0.01 |
LVEF (%), median [IQR] | 61.0 [58.0–65.0] | 57.0 [43.0–61.0] | <0.01 |
E (cm/s), median [IQR] | 79.0 [67.0–99.0] | 95.0 [81.0,106.0] | <0.01 |
E/e', median [IQR] | 9.1 [7.4–11.4] | 11.7 [8.6–14.4] | <0.01 |
EDT (ms), median [IQR] | 188.0 [162.0–221.0] | 176.5 [140.0–189.0] | <0.01 |
LA, median [IQR] | |||
LAD (mm) | 43.0 [40.0–46.0] | 48.0 [45.0–52.0] | <0.01 |
LAEF (%) | 35.7 [21.6–51.9] | 14.5 [9.4–17.8] | <0.01 |
LAVmax (mm3) | 46.0 [29.0–67.0] | 89.0 [71.5–110.0] | <0.01 |
LAVImax (mL/m2) | 39.1 [31.8–51.4] | 59.7 [50.1–73.5] | <0.01 |
LAVImin (mL/m2) | 25.6 [16.6–38.2] | 51.8 [42.7–64.0] | <0.01 |
LASRS (%) | 0.94 [0.69–1.23] | 0.48 [0.37–0.65] | <0.01 |
LASRE (%) | 1.10 [0.85–1.46] | 0.76 [0.60–0.98] | <0.01 |
TTE, transthoracic echocardiogram; SEC/LAAT, spontaneous echo contrast/left atrial appendage thrombus; IQR, interquartile range; BMI, body mass index; AF, atrial fibrillation; HTN, hypertension; DM, diabetes mellitus; CHF, congestive heart failure; TIA, transient ischemic attack; PLT, platelet; RAD, right atrial diameter; mPAP, mean pulmonary artery pressure; IVSth, interventricular septum thickness; LVDD, left ventricle end-diastolic diameter; LVEF, left ventricular ejection fraction; E, peak mitral valve flow velocity during early ventricular diastole; e', peak mitral ring motion velocity during early ventricular diastole; E/e', ratio of E to e'; EDT, E-wave deceleration time; LAD, left atrial diameter; LAEF, left atrial ejection fraction; LAVmax, maximum left atrial volume; LAVImax, maximum left atrial volume index; LAVImin, minimum left atrial volume index; LASRS, left atrial reservoir strain rate; LASRE, left atrial strain rate during rapid ventricular filling.
Screening of variables for the predictive model
We conducted LASSO regression on 22 variables with statistical significance (Figure 3). The model had the minimum classification error at the level of λ=0.015 and the least number of variables at the level of λ=0.038 (female gender, D-dimer, CHF, LVEF, E/e', LAEF, LASVI, LASRS) (Table 2); these eight variables exhibited good predictive value for dense SEC/LAAT, with corresponding coefficients of 0.146, 0.187, 0.314, −0.025, 0.023, −0.050, 0.018, and –0.819, respectively.
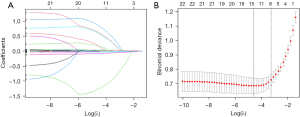
Table 2
Variable | LASSO regression | Stepwise regression analysis | |||
---|---|---|---|---|---|
Coefficient | OR | 95% CI | P | ||
Gender (female) | 0.146 | 2.811 | 1.445–5.469 | 0.002 | |
D-dimer (μg/mL) | 0.187 | 2.460 | 1.230–4.921 | 0.01 | |
CHF (%) | 0.314 | – | – | – | |
LVEF (%) | −0.025 | 0.961 | 0.927, 0.996 | 0.03 | |
E/e' | 0.023 | – | – | – | |
LAEF (%) | −0.050 | 0.950 | 0.932–0.967 | <0.01 | |
LAVImax (mL/m2) | 0.018 | – | – | – | |
LASRS (%) | −0.819 | 0.173 | 0.035–0.848 | 0.03 |
LASSO, least absolute shrinkage and selection operator; OR, odds ratio; CI, confidence interval; CHF, congestive heart failure; LVEF, left ventricular ejection fraction; E, peak mitral valve flow velocity during early ventricular diastole; e', peak mitral ring motion velocity during early ventricular diastole; E/e', ratio of E to e'; LAEF, left atrial ejection fraction; LAVImax, maximum left atrial volume index; LASRS, left atrial reservoir strain rate.
To further simplify the model, the aforementioned eight variables were subjected to stepwise regression analysis. The stepwise regression data in Table 2 indicated that female gender, high D-dimer level, low LVEF, low LAEF, and low LASRS were independent markers for predicting SEC/LAAT (P<0.05), with odds ratios (OR) values and 95% confidence intervals of 2.811 (1.445–5.469), 2.46 (1.230–4.921), 0.961 (0.927–0.996), 0.950 (0.932–0.967), and 0.173 (0.035–0.848).
Construction of a nomogram model for predicting dense SEC/LAAT in patients with NVAF
Five variables selected through stepwise regression, including female gender, high D-dimer, low LVEF, low LAEF, and low LASRS, were adopted to construct a nomogram prediction model (Figure 4). The results revealed that female patients and patients with elevated D-dimer levels had a higher incidence of LAA dense SEC/LAAT, while patients with lower LVEF, LAEF, and LASRS had an increased incidence of dense SEC/LAAT.
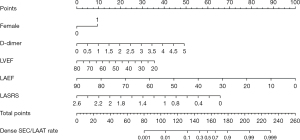
Validation of the predictive capability of the nomogram for dense SEC/LAAT in patient with NVAF
The statistical results indicated that the nomogram had a C-statistic of 0.921, which was suggestive of good discrimination capability. To further calibrate the nomogram, we plotted the calibration curve of this nomogram (Figure 5). Given the relatively small amount of data in this study, we conducted internal validation through 500 repetitions of bootstrapping. In the calibration curve results, the calibration curve of this nomogram was very close to 45°, indicating good calibration of the predictive model. Meanwhile, the bootstrap C-statistic value was 0.903, with a bias of 0.018.
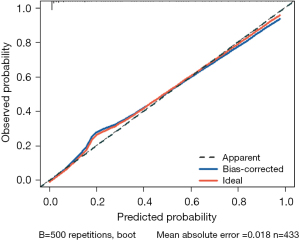
Comparison of the predictive performance between the nomogram model and traditional prediction models CHADS2 and CHA2DS2-VASc for dense SEC/LAAT in patients with NVAF
In an attempt to further compare our prediction model with traditional prediction models (CHADS2 and CHA2DS2-VASc) in their predictive performance for dense SEC/LAAT, we plotted the ROC curves of three prediction models (Figure 6A). Accordingly, the AUC value and 95% confidence interval of nomogram was the highest, equal to 0.921 (0.785–0.931), while that of CHADS2 and CHA2DS2-VASc was 0.624 (0.397–0.792) and 0.635 (0.483–0.750), respectively. Therefore, the nomogram better predicted dense SEC/LAAT. Furthermore, corresponding DCA curves were drawn (Figure 6B). A higher net benefit was detected for the nomogram under different probability thresholds. Additionally, we compared the performance of the three models (Table 3) by calculating the NRI and IDI values and 95% confidence intervals. The results indicated that the nomogram had an NRI of 0.449 (0.324–0.575) and an IDI of 0.461 (0.408–0.515) compared with the CHADS2 model (P<0.05), along with am NRI of 0.521 (0.411–0.632) and an IDI of 0.432 (0.400–0.504) in comparison with the CHA2DS2-VASc model (P<0.05). Hence, it was reasonable to conclude that the nomogram had superior performance compared to the traditional CHADS2 and CHA2DS2-VASc models in predicting LAA dense SEC/LAAT.
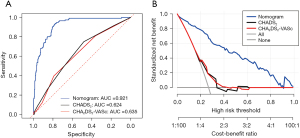
Table 3
Index | Values | 95% CI | P |
---|---|---|---|
NRI | |||
vs. CHADS2 | 0.449 | 0.324–0.575 | <0.05 |
vs. CHA2DS2-VASc | 0.521 | 0.411–0.632 | <0.05 |
IDI | |||
vs. CHADS2 | 0.461 | 0.408–0.515 | <0.05 |
vs. CHA2DS2-VASc | 0.432 | 0.400–0.504 | <0.05 |
NRI, net reclassification index; IDI, integrated discrimination improvement; CI, confidence interval.
Discussion
This study demonstrated the significant value of left atrial structural and functional variables in predicting dense SEC/LAAT. Two variables, LAEF—reflecting left atrial function—and LASVI—reflecting left atrial structure—exhibited the highest application value. Additionally, we integrated comprehensive and clinically accessible variables including female gender, D-dimer level, LVEF, LAEF, and LASRS to construct a nomogram model for predicting dense SEC/LAAT. This prediction model had higher discrimination and application value in predicting dense SEC/LAAT than did the traditional CHADS2 and CHA2DS2-VASc models.
The CHADS2 score is a predictive model that was proposed for identifying populations at high risk of stroke in patients with AF (19). Owing to its limited discrimination ability, the CHA2DS2-VASc score was proposed in 2010 to improve upon the CHADS2 score (20). Nevertheless, clinical study based on large sample sizes have shown a relatively limited value of CHA2DS2-VASc score in identifying high-risk stroke populations, with a C-statistic less than 0.7, which is only 0.004 higher than that of the CHADS2 score (21). The findings of our study indicated that the CHADS2 and CHA2DS2-VASc scores were not independent predictors of LAA dense SEC/LAAT, while only female gender was an independent predictor in these two scoring systems.
Due to the limited application value of previous prediction models, a recent study has used the presence of LAA dense SEC/LAAT as an alternative indicator for those at high risk of stroke to construct prediction models for patients with AF. Cetin et al. developed a clinical prediction model using 433 patients with NVAF as the studying population and found that ECG variables, particularly LAD, LVEF, and pulmonary artery systolic pressure, were independent predictors of LAA SEC/LAAT (22). In addition to this indicator, our study also enrolled indicators that could better reflect left atrial structure and left atrial function. Similar to the study of Cetin et al. (22), we found that LVEF was an independent predictor of LAA SEC/LAAT, but mean pulmonary artery pressure and LAD, which reflects left atrial size, were not. A study (23) has substantiated that LAV and LAVI, which better reflect left atrial structure, have limited predictive value for LAA SEC/LAAT. In this study, we additionally incorporated variables of LAEF and LASRS, which could reflect left atrial function, and our results suggested that these indicators were independent predictors for LAA SEC/LAAT, with LAEF having the highest value.
D-dimer level has always been an important laboratory indicator for predicting embolic events, showing high clinical value. However, few studies have examined the use of D-dimer level in predicting LAA SEC/LAAT, and the scant findings are inconsistent (24,25). In our study, D-dimer level was found to be an independent predictor for LAA dense SEC/LAAT, which might be related to the fact that the event investigated in our study was dense SEC rather than SEC.
The nomogram has emerged as a common prediction method in the field of medical research in recent years due to is ability to convert the contribution of each variable into a score and then calculate the probability of events, making it more intuitive (26). Liu et al. (27) also integrated clinical data and ECG data to construct a nomogram model for predicting left atrial spontaneous echo contrast (LASEC) and found that age and nonparoxysmal AF to be two independent predictors for LAAT/SEC. In our study, although the patients were older in the LAAT/SEC group, age was not an independent predictor for LAA SEC/LAAT after adjustment for confounding factors. Although multiple studies have reported (27,28) that nonparoxysmal AF has high predictive value in predicting LAA SEC/LAAT, our findings did not indicate nonparoxysmal AF to be a predictor of LAA SEC/LAAT, which may be linked to the inclusion in our study of LAEF, an indicator reflecting left atrial function. During an AF attack, left atrial hemodynamic changes can be observed in the patients with lower LAEF values. Thus, nonparoxysmal patients with AF may have reduced left atrial hemodynamics, leading to the onset of LAA SEC/LAAT. This study had similar findings to the study of Liu et al. (29). Furthermore, a higher C-statistic indicated the better discrimination potential of our nomogram.
Ultimately, we evaluated and compared the performance of our nomogram model with the CHADS2 and CHA2DS2-VASc models in predicting LAA SEC/LAAT. The results showed that the CHADS2 and CHA2DS2-VASc models had limited value in predicting LAA SEC/LAAT, with the nomogram demonstrating greater predictive ability. Some limitations to this study should be noted. First, our single-center, retrospective design lacked external validation, possibly limiting the performance of the model. Second, the sample size in this study was relatively small, only involving 433 cases, and the patient ECG data did not include left atrial strain data, only left atrial strain rates. Finally, TEE is an invasive procedure, so some frail patients, such as those with severe stroke who could not cooperate with TEE, were not included.
Conclusions
The nomogram, which included indicators of female gender, D-dimer level, LVEF, LAEF, and LASRS, had high clinical value in predicting LAA SEC/LAAT. This may make it possible to predict LAASEC/LAAT through non-invasive examination, accurately identify high-risk stroke populations in AF patients, and then implement personalized anticoagulation. Moreover, this can also significantly alleviate the pain of patients with AF who should receive TEE to confirm the presence of LAA SEC/LAAT for urgent restoration of sinus rhythm.
Acknowledgments
Funding: None.
Footnote
Reporting Checklist: The authors have completed the TRIPOD reporting checklist. Available at https://jtd.amegroups.com/article/view/10.21037/jtd-24-288/rc
Data Sharing Statement: Available at https://jtd.amegroups.com/article/view/10.21037/jtd-24-288/dss
Peer Review File: Available at https://jtd.amegroups.com/article/view/10.21037/jtd-24-288/prf
Conflicts of Interest: All authors have completed the ICMJE uniform disclosure form (available at https://jtd.amegroups.com/article/view/10.21037/jtd-24-288/coif). The authors have no conflicts of interest to declare.
Ethical Statement: The authors are accountable for all aspects of the work in ensuring that questions related to the accuracy or integrity of any part of the work are appropriately investigated and resolved. The study was conducted in accordance with the Declaration of Helsinki (as revised in 2013). This study was granted by the Ethics Committee of the First Affiliated Hospital of Soochow University (ethics approval No. 2024-010). Individual consent for this retrospective analysis was waived.
Open Access Statement: This is an Open Access article distributed in accordance with the Creative Commons Attribution-NonCommercial-NoDerivs 4.0 International License (CC BY-NC-ND 4.0), which permits the non-commercial replication and distribution of the article with the strict proviso that no changes or edits are made and the original work is properly cited (including links to both the formal publication through the relevant DOI and the license). See: https://creativecommons.org/licenses/by-nc-nd/4.0/.
References
- Tsao CW, Aday AW, Almarzooq ZI, et al. Heart Disease and Stroke Statistics-2023 Update: A Report From the American Heart Association. Circulation 2023;147:e93-e621. [Crossref] [PubMed]
- Chugh SS, Havmoeller R, Narayanan K, et al. Worldwide epidemiology of atrial fibrillation: a Global Burden of Disease 2010 Study. Circulation 2014;129:837-47. [Crossref] [PubMed]
- Odutayo A, Wong CX, Hsiao AJ, et al. Atrial fibrillation and risks of cardiovascular disease, renal disease, and death: systematic review and meta-analysis. BMJ 2016;354:i4482. [Crossref] [PubMed]
- Emdin CA, Wong CX, Hsiao AJ, et al. Atrial fibrillation as risk factor for cardiovascular disease and death in women compared with men: systematic review and meta-analysis of cohort studies. BMJ 2016;532:h7013. [Crossref] [PubMed]
- Benjamin EJ, Blaha MJ, Chiuve SE, et al. Heart Disease and Stroke Statistics-2017 Update: A Report From the American Heart Association. Circulation 2017;135:e146-603. [Crossref] [PubMed]
- Joglar JA, Chung MK, Armbruster AL, et al. 2023 ACC/AHA/ACCP/HRS Guideline for the Diagnosis and Management of Atrial Fibrillation: A Report of the American College of Cardiology/American Heart Association Joint Committee on Clinical Practice Guidelines. Circulation 2024;149:e1-e156. [Crossref] [PubMed]
- Silverio A, Di Maio M, Prota C, et al. Safety and efficacy of non-vitamin K antagonist oral anticoagulants in elderly patients with atrial fibrillation: systematic review and meta-analysis of 22 studies and 440 281 patients. Eur Heart J Cardiovasc Pharmacother 2021;7:f20-9. [Crossref] [PubMed]
- Zhu W, Fu L, Ding Y, et al. Meta-analysis of ATRIA versus CHA(2)DS(2)-VASc for predicting stroke and thromboembolism in patients with atrial fibrillation. Int J Cardiol 2017;227:436-42. [Crossref] [PubMed]
- Dalgaard F, Pieper K, Verheugt F, et al. GARFIELD-AF model for prediction of stroke and major bleeding in atrial fibrillation: a Danish nationwide validation study. BMJ Open 2019;9:e033283. [Crossref] [PubMed]
- Shah M, Mobaligh N, Niku A, et al. Predictors of left atrial appendage thrombus despite NOAC use in nonvalvular atrial fibrillation and flutter. Int J Cardiol 2020;317:86-90. [Crossref] [PubMed]
- Blackshear JL, Odell JA. Appendage obliteration to reduce stroke in cardiac surgical patients with atrial fibrillation. Ann Thorac Surg 1996;61:755-9. [Crossref] [PubMed]
- January CT, Wann LS, Calkins H, et al. 2019 AHA/ACC/HRS Focused Update of the 2014 AHA/ACC/HRS Guideline for the Management of Patients With Atrial Fibrillation: A Report of the American College of Cardiology/American Heart Association Task Force on Clinical Practice Guidelines and the Heart Rhythm Society in Collaboration With the Society of Thoracic Surgeons. Circulation 2019;140:e125-51. [Crossref] [PubMed]
- Silva MR, Sampaio F, Braga J, et al. Left atrial strain evaluation to assess left ventricle diastolic dysfunction and heart failure with preserved ejection fraction: a guide to clinical practice: Left atrial strain and diastolic function. Int J Cardiovasc Imaging 2023;39:1083-96. [Crossref] [PubMed]
- Mostafa S, El-Rabbat K, Salah S, et al. Association of left atrial deformation indices with left atrial appendage thrombus in patients with non valvular atrial fibrillation. Indian Heart J 2020;72:265-71. [Crossref] [PubMed]
- Jones NR, McCormack T, Constanti M, et al. Diagnosis and management of hypertension in adults: NICE guideline update 2019. Br J Gen Pract 2020;70:90-1. [Crossref] [PubMed]
- Kleindorfer DO, Towfighi A, Chaturvedi S, et al. 2021 Guideline for the Prevention of Stroke in Patients With Stroke and Transient Ischemic Attack: A Guideline From the American Heart Association/American Stroke Association. Stroke 2021;52:e364-467. [Crossref] [PubMed]
- Buse JB, Wexler DJ, Tsapas A, et al. 2019 Update to: Management of Hyperglycemia in Type 2 Diabetes, 2018. A Consensus Report by the American Diabetes Association (ADA) and the European Association for the Study of Diabetes (EASD). Diabetes Care 2020;43:487-93. [Crossref] [PubMed]
- Fatkin D, Kelly RP, Feneley MP. Relations between left atrial appendage blood flow velocity, spontaneous echocardiographic contrast and thromboembolic risk in vivo. J Am Coll Cardiol 1994;23:961-9. [Crossref] [PubMed]
- Gage BF, Waterman AD, Shannon W, et al. Validation of clinical classification schemes for predicting stroke: results from the National Registry of Atrial Fibrillation. JAMA 2001;285:2864-70. [Crossref] [PubMed]
- Lip GY, Nieuwlaat R, Pisters R, et al. Refining clinical risk stratification for predicting stroke and thromboembolism in atrial fibrillation using a novel risk factor-based approach: the euro heart survey on atrial fibrillation. Chest 2010;137:263-72. [Crossref] [PubMed]
- Aspberg S, Chang Y, Atterman A, et al. Comparison of the ATRIA, CHADS2, and CHA2DS2-VASc stroke risk scores in predicting ischaemic stroke in a large Swedish cohort of patients with atrial fibrillation. Eur Heart J 2016;37:3203-10. [Crossref] [PubMed]
- Cetin EHO, Ozbay MB, Cetin MS, et al. A new risk model for the evaluation of the thromboembolic milieu in patients with atrial fibrillation: the PALSE score. Kardiol Pol 2020;78:732-40. [Crossref] [PubMed]
- Tan BYQ, Ho JSY, Sia CH, et al. Left Atrial Volume Index Predicts New-Onset Atrial Fibrillation and Stroke Recurrence in Patients with Embolic Stroke of Undetermined Source. Cerebrovasc Dis 2020;49:285-91. [Crossref] [PubMed]
- Lin C, Bao Y, Hua W, et al. Differences in D-dimer blood concentration in atrial fibrillation patients with left atrial thrombus or severe left atrial spontaneous echo contrast. J Interv Card Electrophysiol 2022;64:341-7. [Crossref] [PubMed]
- Sun S, Su B, Lin J, et al. A nomogram to predict left atrial appendage thrombus and spontaneous echo contrast in non-valvular atrial fibrillation patients. BMC Cardiovasc Disord 2022;22:311. [Crossref] [PubMed]
- Hess DR. A nomogram for use of non-invasive respiratory strategies in COVID-19. Lancet Digit Health 2021;3:e140-1. [Crossref] [PubMed]
- Liu K, Li Y, Wu K, et al. Retrospective Study of 1255 Non-Anticoagulated Patients with Nonvalvular Atrial Fibrillation to Determine the Risk of Ischemic Stroke Associated with Left Atrial Spontaneous Echo Contrast on Transesophageal Echocardiography. Med Sci Monit 2021;27:e934795. [Crossref] [PubMed]
- Kim HD, Cho DH, Kim MN, et al. Left Atrial Dysfunction, Fibrosis and the Risk of Thromboembolism in Patients With Paroxysmal and Persistent Atrial Fibrillation. Int J Heart Fail 2022;4:42-53. [Crossref] [PubMed]
- Liu XW, Tian X, Hu J, et al. Factors associated with left atrial appendage filling defects on early-phase cardiac computed tomography in patients with nonvalvular atrial fibrillation: a case-control study. Quant Imaging Med Surg 2023;13:720-34. [Crossref] [PubMed]