Screening and identification of hub genes for ischemic cardiomyopathy and construction and validation of a clinical prognosis model using bioinformatics analysis
Highlight box
Key findings
• Ischemic cardiomyopathy (ICM) is closely related to the changes of extracellular matrix and oxidoreductase activity.
What is known and what is new?
• Long-term myocardial ischemia and hypoxia may lead to myocardial cell necrosis, scar formation, and hyperplasia of myocardial fiber tissue, which results in myocardial fibrosis and ICM.
• Bioinformatics methods effectively analyzed the differentially expressed genes of ICM and successfully constructed a regulatory network of ICM susceptibility genes.
What is the implication, and what should change now?
• The genes with significant differences and hub genes obtained by this screening may provide new targets for early ICM diagnosis and treatment.
Introduction
Ischemic cardiomyopathy (ICM) is driven by an interaction of genetic and environmental factors, and encompasses a diverse clinical spectrum including systolic and diastolic dysfunction, congestive heart failure, arrhythmia, and valvular disease, which are caused by adverse proliferation of myocardial fibers after long-term myocardial ischemia or myocardial infarction (1). With the prolongation of life span and the rapid improvement of medical and device-based therapies for ICM, the incidence of ICM has gradually increased, becoming one of the most common causes of heart failure worldwide (2).
Long non-coding RNA (lncRNA) is a class of non-coding RNA with a length greater than 200 nucleotides, which is an important component of all non-coding transcripts. LncRNAs are involved in many important physiologic, as well as pathologic, processes of the body. Recent studies (3-5) have shown that lncRNAs play an important role in cardiovascular diseases, including ICM. Moreover, studies (6-8) have found that lncRNAs can act as competing endogenous RNAs (ceRNAs) and regulate the expression of messenger RNA (mRNA) by competing with mRNA for microRNAs (miRNAs).
Bioinformatics is an emerging interdisciplinary field that studies a variety of omics data, including transcriptomics, proteomics, and other biological data. The research methods mainly include the collection (collection and screening), processing (editing, sorting, management, and display), utilization (calculation and simulation), and analysis of biological data (9,10). Experience has shown that bioinformatics technology has great application value in the screening of disease biomarkers, which is of great significance for the diagnosis, treatment, and prognosis of diseases, and can provide a more comprehensive and profound understanding of diseases (11-14). A recent study showed that differentially expressed genes (DEGs) were significantly enriched in metabolic pathways, oxidative phosphorylation, and extracellular matrix (ECM) receptor interactions, and were closely related to fibrosis, collagen catabolic process and inflammatory response function (15). Interleukin-6 (IL-6) and Ras homologous gene family member A (RHOA) may play an important role in the development of ICM, IL-6 and RHOA, however, the value of the IL-6 and RHOA in ICM was poorly studied, further studies are needed to identify new biomarkers in myocardial ischemia.
To explore potential targets for ICM treatment, this study aims to analyze the genes related to ICM pathogenesis using samples screened from the Gene Expression Omnibus (GEO) database and constructing a regulatory network related to differentially expressed lncRNAs using the bioinformatics methods. We present this article in accordance with the TRIPOD reporting checklist (available at https://jtd.amegroups.com/article/view/10.21037/jtd-23-1722/rc).
Methods
Data source
Data were obtained from the National Center for Biotechnology Information (NCBI) (https://www.ncbi.nlm.nih.gov/) in the GEO database, using R language (R4.1.2; R Foundation for Statistical Computing, Vienna, Austria) The dataset GSE26887 was downloaded directly from the GEO database and contained samples obtained from left ventricular biopsy tissue, based on the GPL6244 detection platform, and Affymetrix Gene Chips Human Gene 1. Gene expression was detected by 0 ST microarray. RNA expression profiles of genes from five healthy controls, seven patients with diabetes mellitus (DM) were excluded, and finally 12 patients without DM were included. This study included five healthy samples as the control group (GSM662158–GSM662162) and 12 ICM patients as the experimental group (GSM662179, GSM662181). The study was conducted in accordance with the Declaration of Helsinki (as revised in 2013).
The age, gender, smoking, hypertension, and body mass index (BMI) of the two groups were similar, and no significant differences were found (Table 1).
Table 1
Variables | Healthy group (n=5) | ICM group (n=12) | t value | P value |
---|---|---|---|---|
Age (years) | 61.00±5.52 | 60.75±4.99 | 0.09 | 0.93 |
Gender | 0.14 | 0.71 | ||
Male | 2 (40.00) | 6 (50.00) | ||
Female | 3 (60.00) | 6 (50.00) | ||
Smoking | 2 (40.00) | 4 (33.33) | 0.07 | 0.79 |
Hypertension | 3 (60.00) | 8 (66.67) | 0.07 | 0.79 |
BMI (kg/m2) | 24.85±2.55 | 25.54±2.09 | 0.58 | 0.57 |
Data are presented as mean ± SD or n (%). ICM, ischemic cardiomyopathy; BMI, body mass index; SD, standard deviation.
Data search and alignment
The GEO query package based on R language (R4.1.2) was used to obtain the GSE26887 data set through the hugene10sttranscriptcluster.db corresponding to the GPL6244 platform. The package annotated the gene name of the data set gene chip probe, obtained the gene name and gene expression value, removed the data not corresponding to the gene name and the data whereby 1 gene name corresponded to multiple probes (i.e., it only retains the gene name corresponding to the maximum expression probe), and removed the data set of seven ICM patients with DM.
Acquisition, visualization, and analysis of DEGs
Based on the R language limma package (Smyth, 2005), the above data were analyzed for differential expression, and DEGs, log fold change (FC), t value, P value, and adjusted (adj.) P value were obtained. Due to the large base of DEGs, small FC of some DEGs, and lack of a statistically significant difference, the screening conditions were set to |logFC|>1 and adj. P<0.01 for further screening of the DEGs. Based on the R language ggplot2 package (Smyth, 2005), the pheatmap package was used to visualize the volcano map and heat map, respectively.
Gene Ontology (GO) and Kyoto Encyclopedia of Genes and Genomes (KEGG) enrichment analysis
GO enrichment analysis was used to analyze genes according to their biological process (BP), cell component (CC), and molecular function (MF). Gene annotation was carried out on the surface, whereas KEGG enrichment analysis was used to identify DEGs enrichment and perform analysis in the aspect of pathway, so as to determine the pathways of disease-related genes. The R language cluster Profiler package and org.Hs.eg.db was used to conduct GO and KEGG enrichment analysis of DEGs, and the ggplot2 package was used for visualization of the results.
Construction of protein-protein interaction (PPI) network corresponding to DEGs
A PPI network was created to establish functional proteins for DEGs based on existing data. The associated network was realized through the Search Tool for the Retrieval of Interacting Genes/Proteins (STRING) database (https://cn.string-db.org/), and the PPI network was constructed through the Cytoscape 3.7.1 software (https://cytoscape.org/download.html) to filter the hub genes with high correlation.
Preliminary verification of the application value of the hub genes
Using the R language ROC package (Smyth, 2005), and taking the degree value in the Cytoscape 3.7.1 software as the screening standard, the receiver operating characteristic (ROC) curve was made for some high correlation hub genes to preliminarily verify their value as disease markers.
Risk model construction and validation
The least absolute shrinkage and selection operator (LASSO) proportional risk regression model was constructed using the GLMNET package to obtain the related genetic risk coefficients, and patients were divided into a high-risk group and a low-risk group. Survival and SurvMiner packages were used to compare survival differences between the high- and low-risk groups, and time ROC packages were used to obtain the time-dependent ROC curve. Heat maps of patients’ risk values were obtained using the pheatmap package in R. The survival package was used for univariate and multifactorial independent prognostic analysis.
Quantitative real-time polymerase chain reaction method was used to detect the levels of key genes
Extracted RNA was absorbed after zero calibration with enzyme-free water, the absorbance value at wavelength 260 and 280 nm was determined, and the average value was taken after two consecutive measurements. According to the above method, Sqstm1, Zfp36, MAPK14, Stat3, IL-6, and RHOA screened out by bioinformatics method were detected as hub genes.
Statistical analysis
R language version 4.0.3 was used for statistical analysis. Statistical significance was considered when P<0.05. Time ROC packages were used to obtain the time-dependent ROC curve and the value of the area under the ROC curve (AUC). When the AUC value was greater than 0.7, it indicated good predictive value. Heat maps of patients’ risk values were obtained using the pheatmap package in R. The survival package was used for univariate and multifactorial independent prognostic analysis. Calculation of AUC and its threshold values for a good classification model in the method section.
Results
DEG screening results
A total of 259 genes with significantly changed FC values were obtained through conditional screening, including up-regulated genes and down-regulated genes (control group and ICM group), using R language to visualize the results in a volcano (Figure 1). Using |logFC|>1.5 and adj. P<0.01 as the primary calculations, 52 DEGs were screened, including 25 up-regulated genes and 27 down-regulated genes, and R language was used to map the results in a thermochemical diagram. The up-regulated and down-regulated genes were then filtered to yield the top 10 DEGs (Figure 2).
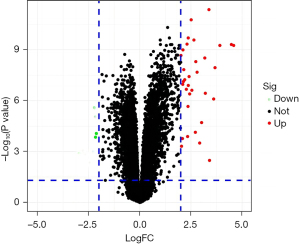
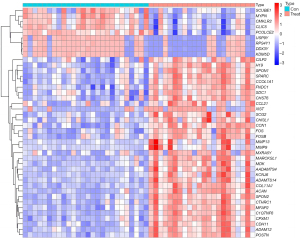
DEGs GO analysis results
GO enrichment analysis was conducted for up-regulated genes and down-regulated genes. The BPs of the up-regulated genes were mainly enriched in the regulation of inflammatory response, leukocyte migration, wound repair, intercellular adhesion regulation, response to endotoxin, response to bacteria-derived molecules, bone marrow leukocyte migration, leukocyte chemotaxis, positive regulation of inflammatory response, and monocyte chemotaxis. The BPs of the down-regulated genes were mainly enriched in the process of muscle system, muscle contraction, and the dynamic changes of cardiac conduction system. The CCs of up-regulated genes were mainly enriched in the ECM containing collagen fibers, cell focal adhesion, cell matrix connection, secretory cavity, cytoplasmic vesicle, vesicular cavity, outer side of plasma membrane, primary lysosome, and aniline blue granules (lymphocytes). The CCs of down-regulated genes were mainly concentrated in smooth muscle fibers. The MFs of up-regulated genes were mainly enriched in the binding of receptor for advanced glycation end products (RAGEs), whereas those of the down-regulated genes were mainly enriched in the activity of oxidoreductase, nicotinamide adenine dinucleotide phosphate (NADP) binding pathway, and structural components with compressive function in the ECM (Figure 3).
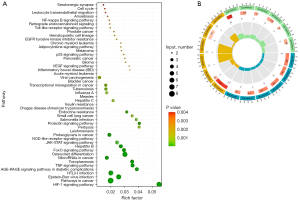
PPI network construction
The 259 DEGs were imported into STRING and the nodes were filtered from large to small according to the degree value. The nodes with larger degree values were more likely to be disease-related biomarkers. The first 16 nodes of DEGs were visualized network graphs generated by using the CytoHubba plug-in (Figure 4).
Verification of the application value of some hub genes
The first two hub genes (IL-6 and RHOA) with the largest degree value among the above up-regulated and down-regulated genes were selected and their expression values were combined in the gene chip to draw the ROC curve based on the pROC package of R language. The AUC values of IL-6 and RHOA were 0.956 and 0.995, respectively, which indicated their great value as potential disease-related biomarkers (Figure 5).
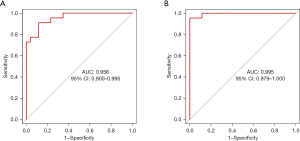
Construction of a risk prognosis model
LASSO regression analysis was used to build the risk model and the DEGs were screened out to build the risk model. The risk model calculation formula was as follows: risk value = NOX4 ×0.41 + NOX5 ×1.14 − GLS2 ×0.22 − MYb ×0.022 + TGFBR1 ×0.053 + DUSP1 ×0.092 − SLC1A4 ×0.12 − NF2 ×0.27 (Figure 6).
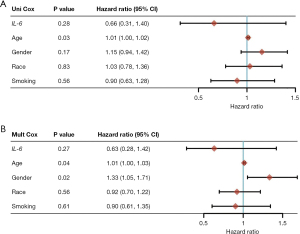
Quantitative real-time polymerase chain reaction analysis
The relative expression level of key genes in the ICM model and blank control groups screened by bioinformatics method is displayed in Figure 7. The expression levels of Sqstm1, Nos2, IL-6, RHOA, and Zfp36 genes in the ICM group are lower than those in the blank control group and the difference was statistically significant (P<0.05). RHOA and Stat3 were identified as the key genes controlling the occurrence and development of ICM (Figure 7).
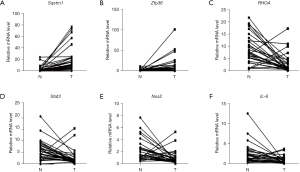
Discussion
ICM is a type of end-stage coronary atherosclerotic disease (16). Long-term imbalance of oxygen supply and demand leads to apoptosis and necrosis of myocardial cells, cardiac fibrosis, and scar tissue formation, leading to poor prognosis (17). Currently, because the necrosis of myocardial cells is an irreversible pathological process, effective treatment methods for ICM are unavailable. Although heart transplantation can replace the damaged organs, it cannot be widely carried out due to the limitations of donors and immune rejection. In the past few years, many studies (18,19) have focused on elucidating the molecular mechanisms of protein-encoding genes and miRNAs. Through scientific and technological advancement, lncRNAs (containing more than 200 nucleotides) have been discovered. This newly discovered RNA has been shown to play an important role in a wide range of BPs (20). From cancer to cardiovascular disease, lncRNA plays an important role in the occurrence and development of diseases. A study has shown that lncRNA can target and bind miRNA to play its role as ceRNA, that is, lncRNA can affect the expression of protein-coding genes by competitively binding miRNA, thus reducing the degradation of protein-coding genes (21).
There are differences in gene expression between patients with ICM and healthy persons. Using bioinformatics methods, we attempted to mine and analyze the genes and proteins of patients with ICM, and tried to find biomarkers related to ICM, so as to provide reference for early diagnosis, treatment, and prognosis of patients with ICM (22). Compared with the normal control group, the GO enrichment analysis of DEGs showed that the BP enrichment results of the up-regulated genes included changes in muscle contraction and cardiac conduction system, the CC enrichment results included smooth muscle fibers, and the MF enrichment results included compressive ECM components. CC enrichment results included ECM containing collagen fibers, cell-matrix junction, cell focal adhesion, and so on. KEGG enrichment analysis of down-regulated genes revealed that they were mainly concentrated in the ECM receptor interaction pathway. In ICM patients, the expression of genes controlling smooth muscle fibers, muscle contraction, and cardiac conduction was up-regulated, which is consistent with the pathophysiology of myocardial ischemia (23-25).
In addition, the ECM in patients with ICM is significantly different from that in normal people (26). A study of epicardial adipose tissue (EAT) in patients with ICM revealed that EAT involves severe ECM remodeling, whereas EAT and myocardium are not separated by fascia, and they have a common microcirculation with myocardium (27). The changes of ECM have important significance for the diagnosis and treatment of ICM. In addition, GO enrichment analysis in the genes up-regulated by ICM patients showed that MF was enriched in the combination of oxidoreductase activity and NADP, suggesting that the pathogenesis of ICM patients may be related to some oxidoreductase activities (28-30). The activity of xanthoreductase (XOR) is an independent risk factor for the occurrence of coronary artery spasm (CAS), and higher XOR activity is related to the severity of the spasm, xanthine oxidase, and xanthine dehydrogenase and most oxidoreductase all use nicotinamide adenine dinucleotide (NAD)+/NAD reduced form (NADH) as the electron transfer receptor and donor. It can be seen that some oxidoreductases in ICM patients may become potential biomarkers of the disease (31). IL-6 is not only a multi-effect immune regulatory cytokine, but is also secreted by many types of cells. IL-6 in vascular endothelial cells can increase the expression of platelet-derived growth factor (PDGF), which stimulates the proliferation and migration of vascular smooth of muscle cells and fibroblasts, acts as a chemotactic factor, and has a chemotactic effect on monocytes and neutrophils, inducing them to produce inflammatory factors (32). IL-6 can also increase the permeability of vascular endothelial cells and promote the occurrence of vasculitis and atherosclerosis (33). Endothelial cells can synthesize and release nitric oxide (NO) through L-arginine to regulate vascular resistance of coronary artery and adjacent vessels. IL-6 may activate the L-arginine-NO pathway after myocardial ischemia and infarction (34).
Limitations
The present study failed to study the function research of differential genes. Moreover, ingenuity pathway analysis was not conducted in the present study. Additionally, we were unable to further validate the expression levels and clinical value of relevant genes in in vivo and in vitro experiment. Finally, the sample sizes of healthy myocardial and ICM myocardial tissues were relatively small without patients with other heart diseases.
Conclusions
Through the application of bioinformatics methods and high-throughput data sets to analyze the DEGs of healthy and ischemic myocardial tissues, enrich and analyze the DEGs, build PPI networks to find hub genes, and perform ROC curves on some hub genes to verify their application value, the findings in this study suggest that the RHOA gene overexpression, changes in ECM, oxidoreductase activity, and the regulation of inflammatory response may be closely related to the pathogenesis of ICM. Likewise, the study revealed that RHOA and IL-6 are potential biomarkers of ICM. Further verification of their value in the diagnosis and treatment of ICM and observation of curative effect is required. This clinical prognosis model provided a potential pathway for the treatment of myocardial cells in ICM.
Acknowledgments
Funding: None.
Footnote
Reporting Checklist: The authors have completed the TRIPOD reporting checklist. Available at https://jtd.amegroups.com/article/view/10.21037/jtd-23-1722/rc
Peer Review File: Available at https://jtd.amegroups.com/article/view/10.21037/jtd-23-1722/prf
Conflicts of Interest: All authors have completed the ICMJE uniform disclosure form (available at https://jtd.amegroups.com/article/view/10.21037/jtd-23-1722/coif). C.G.M. serves as an unpaid editorial board member of Journal of Thoracic Disease from February 2023 to January 2025. C.G.M. received grants or contracts from GE Healthcare and Florida Heart and Research Foundation; and payments from GE Healthcare for educational symposiums and webinars. The other authors have no conflicts of interest to declare.
Ethical Statement: The authors are accountable for all aspects of the work in ensuring that questions related to the accuracy or integrity of any part of the work are appropriately investigated and resolved. The study was conducted in accordance with the Declaration of Helsinki (as revised in 2013).
Open Access Statement: This is an Open Access article distributed in accordance with the Creative Commons Attribution-NonCommercial-NoDerivs 4.0 International License (CC BY-NC-ND 4.0), which permits the non-commercial replication and distribution of the article with the strict proviso that no changes or edits are made and the original work is properly cited (including links to both the formal publication through the relevant DOI and the license). See: https://creativecommons.org/licenses/by-nc-nd/4.0/.
References
- Chery G, Kamp N, Kosinski AS, et al. Prognostic value of myocardial fibrosis on cardiac magnetic resonance imaging in patients with ischemic cardiomyopathy: A systematic review. Am Heart J 2020;229:52-60. [Crossref] [PubMed]
- Jin Y, Qiu CG, Zheng QS, et al. Correlating blood levels of 8-hydroxydeoxyguanosine to hOGG1 genotypes and the incidence of ischemic cardiomyopathy. Kaohsiung J Med Sci 2016;32:241-7. [Crossref] [PubMed]
- Jin Q, Gong Q, Le X, et al. Bioinformatics and Experimental Analyses Reveal Immune-Related LncRNA-mRNA Pair AC011483.1-CCR7 as a Biomarker and Therapeutic Target for Ischemic Cardiomyopathy. Int J Mol Sci 2022;23:11994. [Crossref] [PubMed]
- Wang K, Lin Y, Shen H, et al. LncRNA TUG1 Exacerbates Myocardial Fibrosis in Diabetic Cardiomyopathy by Modulating the microRNA-145a-5p/Cfl2 Axis. J Cardiovasc Pharmacol 2023;81:192-202. [Crossref] [PubMed]
- Xu Y, Cao L, Ji S, et al. LncRNA ANRIL-mediated miR-181b-5p/S1PR1 axis is involved in the progression of uremic cardiomyopathy through activating T cells. Sci Rep 2022;12:18027. [Crossref] [PubMed]
- Zou Y, Zheng WB, Elsheikha HM, et al. Modulation of long noncoding RNA (lncRNA) and messenger RNA (mRNA) expression in the liver of Beagle dogs by Toxocara canis infection. Parasit Vectors 2023;16:114. [Crossref] [PubMed]
- Zhu HR, Yu XN, Zhang GC, et al. Comprehensive analysis of long non‑coding RNA‑messenger RNA‑microRNA co‑expression network identifies cell cycle‑related lncRNA in hepatocellular carcinoma. Int J Mol Med 2019;44:1844-54. [Crossref] [PubMed]
- Lu P, Yang J, Li M, et al. A desert lncRNA HIDEN regulates human endoderm differentiation via interacting with IMP1 and stabilizing FZD5 mRNA. Genome Biol 2023;24:92. [Crossref] [PubMed]
- Zhang X, Qin X, Yu T, et al. Chromatin regulators-related lncRNA signature predicting the prognosis of kidney renal clear cell carcinoma and its relationship with immune microenvironment: A study based on bioinformatics and experimental validation. Front Genet 2022;13:974726. [Crossref] [PubMed]
- Shu Q, Liu X, Yang J, et al. The clinical prognostic value of lncRNA FOXP4-AS1 in cancer patients: A meta-analysis and bioinformatics analysis based on TCGA datasets. Medicine (Baltimore) 2022;101:e31439. [Crossref] [PubMed]
- Xue S, Guo H, Wei S, et al. Identification of a combined lncRNA prognostic signature and knockdown of lncRNA MANCR to inhibit progression of clear cell renal cell carcinoma by bioinformatics analysis. Transl Androl Urol 2022;11:1304-17. [Crossref] [PubMed]
- Chen Y, Si H, Bao B, et al. Integrated analysis of intestinal microbiota and host gene expression in colorectal cancer patients. J Med Microbiol 2022; [Crossref] [PubMed]
- Tang S, Liao K, Shi Y, et al. Bioinformatics analysis of potential Key lncRNA-miRNA-mRNA molecules as prognostic markers and important ceRNA axes in gastric cancer. Am J Cancer Res 2022;12:2397-418. [PubMed]
- Wang J, Wang B, Zhou B, et al. A novel immune-related lncRNA pair signature for prognostic prediction and immune response evaluation in gastric cancer: a bioinformatics and biological validation study. Cancer Cell Int 2022;22:69. [Crossref] [PubMed]
- Zhang N, Yang C, Liu YJ, et al. Analysis of susceptibility genes and myocardial infarction risk correlation of ischemic cardiomyopathy based on bioinformatics. J Thorac Dis 2022;14:3445-53. [Crossref] [PubMed]
- Hoffman M, Palioura D, Kyriazis ID, et al. Cardiomyocyte Krüppel-Like Factor 5 Promotes De Novo Ceramide Biosynthesis and Contributes to Eccentric Remodeling in Ischemic Cardiomyopathy. Circulation 2021;143:1139-56. [Crossref] [PubMed]
- Hegeman RRMJJ, Swaans MJ, Van Kuijk JP, et al. Midterm Outcome of Hybrid Transcatheter and Minimally Invasive Left Ventricular Reconstruction for the Treatment of Ischemic Heart Failure. Struct Heart 2022;6:100081. [Crossref] [PubMed]
- Dang H, Ye Y, Zhao X, et al. Identification of candidate genes in ischemic cardiomyopathy by gene expression omnibus database. BMC Cardiovasc Disord 2020;20:320. [Crossref] [PubMed]
- Haas J, Frese KS, Sedaghat-Hamedani F, et al. Energy Metabolites as Biomarkers in Ischemic and Dilated Cardiomyopathy. Int J Mol Sci 2021;22:1999. [Crossref] [PubMed]
- Akhtar M, Elliott PM. Risk Stratification for Sudden Cardiac Death in Non-Ischaemic Dilated Cardiomyopathy. Curr Cardiol Rep 2019;21:155. [Crossref] [PubMed]
- Lu Y, An L, Taylor MRG, et al. Nrf2 signaling in heart failure: expression of Nrf2, Keap1, antioxidant, and detoxification genes in dilated or ischemic cardiomyopathy. Physiol Genomics 2022;54:115-27. [Crossref] [PubMed]
- Iravani Saadi M, Salami J, Abdi H, et al. Expression of interleukin 1, interleukin 27, and TNF α genes in patients with ischemic cardiomyopathy versus idiopathic dilated cardiomyopathy: A case-control study. Health Sci Rep 2022;5:e701. [Crossref] [PubMed]
- Wu L, Zheng Y, Ruan X, et al. Long-chain noncoding ribonucleic acids affect the survival and prognosis of patients with esophageal adenocarcinoma through the autophagy pathway: construction of a prognostic model. Anticancer Drugs 2022;33:e590-603. [Crossref] [PubMed]
- Chen C, Tian J, He Z, et al. Identified Three Interferon Induced Proteins as Novel Biomarkers of Human Ischemic Cardiomyopathy. Int J Mol Sci 2021;22:13116. [Crossref] [PubMed]
- Wang J, Xie S, Cheng Y, et al. Identification of potential biomarkers of inflammation-related genes for ischemic cardiomyopathy. Front Cardiovasc Med 2022;9:972274. [Crossref] [PubMed]
- Alimadadi A, Aryal S, Manandhar I, et al. Identification of Upstream Transcriptional Regulators of Ischemic Cardiomyopathy Using Cardiac RNA-Seq Meta-Analysis. Int J Mol Sci 2020;21:3472. [Crossref] [PubMed]
- Ye Y, Jin Q, Gong Q, et al. Bioinformatics and Experimental Analyses Reveal NFIC as an Upstream Transcriptional Regulator for Ischemic Cardiomyopathy. Genes (Basel) 2022;13:1051. [Crossref] [PubMed]
- Sawhney V, Brouilette S, Campbell N, et al. Association of genetic variation in telomere-related SNP and telomerase with ventricular arrhythmias in ischemic cardiomyopathy. Pacing Clin Electrophysiol 2018;41:261-6. [Crossref] [PubMed]
- Chen JH, Wang LL, Tao L, et al. Identification of MYH6 as the potential gene for human ischaemic cardiomyopathy. J Cell Mol Med 2021;25:10736-46. [Crossref] [PubMed]
- Li Y, Jiang Q, Ding Z, et al. Identification of a Common Different Gene Expression Signature in Ischemic Cardiomyopathy. Genes (Basel) 2018;9:56. [Crossref] [PubMed]
- Wang J, Wang F, Zhu J, et al. Transcriptome Profiling Reveals PHLDA1 as a Novel Molecular Marker for Ischemic Cardiomyopathy. J Mol Neurosci 2018;65:102-9. [Crossref] [PubMed]
- Wu L, Zhong Y, Yu X, et al. Selective poly adenylation predicts the efficacy of immunotherapy in patients with lung adenocarcinoma by multiple omics research. Anticancer Drugs 2022;33:943-59. [Crossref] [PubMed]
- Zou Y, Wang Y, Rong Z, et al. A susceptibility biomarker identification strategy based on significantly differentially expressed ceRNA triplets for ischemic cardiomyopathy. Biosci Rep 2020;40:BSR20191731. [Crossref] [PubMed]
- Yi X, Jiang DS, Feng G, et al. An altered left ventricle protein profile in human ischemic cardiomyopathy revealed in comparative quantitative proteomics. Kardiol Pol 2019;77:951-9. [Crossref] [PubMed]