A validated model to predict spread through air space in lung adenocarcinoma
Highlight box
Key findings
• The nomogram integrating shape, ground-glass opacity ratio and spicules achieved good predictive performance in predicting spread through air space (STAS) before surgery.
What is known and what is new?
• STAS is a new invasive pattern in lung adenocarcinoma and a risk factor for worse outcome in lung adenocarcinoma. Some computed tomography (CT) features were associated with STAS.
• In this study, the nomogram incorporating CT features was built and performed well in predicting STAS.
What is the implication, and what should change now?
• The nomogram incorporating CT features could be applied to facilitate individualized preoperative prediction of STAS. Additional studies should be designed with larger cohorts to confirm these findings.
Introduction
In recent years, the spread through air space (STAS) of tumours has received growing attention in the field of radiology (1). According to the 2015 World Health Organization (WHO) classification, STAS is a newly recognized pattern of invasion for lung adenocarcinomas. It consists of micropapillary clusters, solid nests, or single cells that extend beyond the edge of the tumour into air spaces surrounding the lung parenchyma (2). Previous studies have suggested that STAS is a significant risk factor related to overall survival (OS) and recurrence-free survival (RFS) of lung adenocarcinoma after limited resection (3,4). Kadota et al. (4) found that limited resection was significantly associated with a higher risk of recurrence than lobectomy in early-stage patients with STAS, suggesting that if these patients received lobectomy, the recurrence rate could be decreased. Therefore, the ability to discriminate STAS using preoperative radiological features is essential for prognosis prediction and formulating treatment strategies.
The nomogram has been accepted as a tool that can simplify statistical predictive models into a single numerical estimate for the probability of an event (5). In this study, we aimed to build a nomogram based on computed tomography (CT) features to predict STAS in lung adenocarcinoma for facilitating surgical pattern selection and to explore the survival outcomes and investigated the prognostic impact of STAS. We present this article in accordance with the TRIPOD reporting checklist (available at https://jtd.amegroups.com/article/view/10.21037/jtd-23-1871/rc).
Methods
The study was conducted in accordance with the Declaration of Helsinki (as revised in 2013). This retrospective study was approved by the Ethics Committee of Tongji Medical College of Huazhong University of Science and Technology (approval No. S377), and individual consent for this retrospective analysis was waived.
Patients and inclusion criteria
A total of 1,887 consecutive patients evaluated by the Multidisciplinary Thoracic Oncology Group at the Union Hospital of Tongji Medical College from December 2015 to March 2021 were retrospectively collected. The inclusion criteria were as follows: (I) primary lung adenocarcinoma pathologically confirmed by surgical resection; (II) available pathology reports (including the assessment of STAS, predominant pathological subtype, perineural, pleural, and lymphatic invasion); (III) available clinical data, including age, sex, smoking history, type of lung resection; and (IV) tumours classified as T1 or T2 stage based on the 8th edition of the tumour-node-metastasis (TNM) staging system. The exclusion criteria were as follows: (I) previous lung surgery (n=69); (II) previous chemoradiotherapy (n=132); (III) metastatic lung adenocarcinoma (n=48); (IV) specific variants of adenocarcinoma (n=89); (V) thin-section CT was not performed before treatment (n=60); and (VI) tumours classified as T3 or T4 stage (n=164). After exclusion, 1,325 patients (83 STAS-positive patients and 1,242 STAS-negative patients) remained. The lower proportion of STAS-positive patients of the group would lead to an imbalance of the model. According to the study of Kim (6), we used a matching method and selected an approximately 1:2 ratio (STAS-positive-to-STAS-negative) by matching demographic variables, including age, sex, and smoking status. The 1:2 ratio matching was selected for it yielding the most balanced data set. After 1:2 matching, the study included 83 STAS-positive patients and 161 STAS-negative patients for further analysis. Finally, the patients were randomly divided into testing and training sets at a ratio of 2:8 (Figure 1). Clinical demographic characteristics, including age, sex, smoking history, and type of lung resection (lobectomy, segmentectomy and wedge resection), were analysed as variables. Patients were followed up until January 28, 2024 via telephone. Based on the consensus agreement of Punt et al. (7), OS referred to the time between surgery and death from any cause. RFS referred to the time between surgery and recurrence/metastasis or death from any cause.
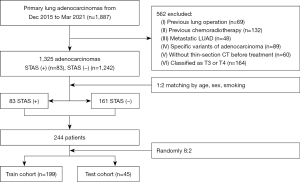
Histologic evaluation
Three pathologists examined all surgical specimens to assess the presence of STAS. The definition of STAS was in accordance with that of the WHO (2). All tumours were staged following the 8th edition of the TNM classification (8). The presence of perineural, pleural and lymphatic invasion was routinely evaluated for each tumour. Anaplastic lymphoma kinase (ALK) rearrangement and epidermal growth factor receptor (EGFR) status were evaluated in selected tumours depending on clinical needs.
CT image acquisition
All patients underwent unenhanced CT scans within 1 week prior to surgery. CT examinations were performed using multislice spiral CT systems (SOMATOM Definition AS +, Siemens Healthineers, Erlangen, Germany). CT examinations were performed ranging from the chest inlet to the inferior level of the costophrenic angle. The CT parameters were as follows: detector collimation width, 64 mm × 0.6 mm and 128 mm × 0.6 mm; tube voltage, 120 kV. The tube current was regulated by an automatic exposure control system (CARE Dose 4D). CT images were obtained at a slice thickness of 1.5 mm, and an interval of 1.5 mm was reconstructed. Then, the reconstructed image was transmitted to the workstation and picture archiving and communication system (PACS) for multiplanar reconstruction (MPR) postprocessing. The lung window level was −600 Hounsfield units (HU), and the width was 1,200 HU; the mediastinal window level was 50 HU, and the width was 1,200 HU.
CT image interpretation
All imaging data were evaluated independently by two experienced radiologists (H.S., a thoracic radiologist with 35 years of experience, and Y.Z., a radiology fellow with 4 years of experience in interpreting CT images). Both radiologists analysed CT images without access to clinical and histologic findings but were aware of the presence and sites of the tumours. Radiologic factors, including tumour location (peripheral or central), maximum tumour diameter on the lung window, ground-glass opacity (GGO) ratio, tumour density [pure GGO (pGGO), mixed GGO (mGGO) and solid], satellite lesions, homogeneity, shape (round or oval and irregular), margin, pleural indentation, spiculation, air bronchogram, vascular convergence, vacuole sign, lymphadenopathy, and cavitation, were assessed using both axial CT images and MPR images. Any disagreements between the radiologists were resolved by discussion.
CT features of lung adenocarcinoma
A central tumour was described as being located in the segmental or more proximal bronchi, and a peripheral tumour was described as residing in the subsegmental bronchi or more distal airway. The GGO ratio was measured as (maximum diameter of the GGO component/maximum diameter of the lesion), where the maximum diameter of the lesion included both the GGO and solid components. pGGO tumour referred to a lung tumour without a solid component, mGGO tumour referred to a lung tumour with both GGO and a solid component, and solid tumour referred to a tumour showing only consolidation without GGO on thin-section CT. Satellite lesions were defined as small nodules surrounding the primary tumour. Homogeneity was defined as a difference in CT values in the tumour of less than 20 HU. A round or oval tumour was circle shaped, and any shape that was not round or oval was defined as irregular. The margin was indicated as well-defined or ill-defined. Pleural indentation was defined as a linear attenuation extending to the pleural surface from the tumour. Spiculation was evaluated in the lung window, and indicated as different degrees of spinous or burr-like protrusions at the tumour margin. Air bronchogram presented with a cut-off air structure within the tumour. Vascular convergence was related to convergence of vessels around the tumour. The vacuole sign was defined as a small round or oval hole with air attenuation visible in the tumour. Lymphadenopathy was defined as lymph nodes with a short-axis diameter of more than 1 cm. Cavitation was characterized as a gas-filled space in the tumour with a thick wall.
Statistical analysis
Statistical analysis was conducted with R version 4.1.0 (R Foundation for Statistical Computing, Vienna, Austria) and STATA version 15.0 for Windows (StataCorp, College Station, TX, USA). The training data set was used to develop the prediction model in the final logistic regression. Continuous variables were expressed as median [interquartile range (IQR)] and categorical variables were expressed as frequency (percentage). The bivariate analysis was conducted with the Mann-Whitney U test for continuous variables and Fisher’s exact χ2 test for categorical variables. Forward stepwise selection was applied to select variables for inclusion in the final regression model, with Akaike’s information criterion (AIC) as the stopping rule (9,10). We formulated the nomogram using the rms package in R, version 4.1.0 (11). The components of the final model were independent of each other. The model performance was validated by discrimination and calibration using the testing data set. The discrimination ability of the model was determined by the area under the receiver operating characteristic (ROC) curve. The optimal cut-off was selected to maximize the Youden index, which is the difference between the sensitivity and the false-positive rate. The calibration curve was assessed using the Hosmer-Lemeshow goodness-of-fit test and the validation sample. By quantifying the net benefits at different threshold probabilities in the testing data set, we conducted a decision curve analysis (DCA) to evaluate the clinical value of the nomogram (12).
For survival analysis, the Kaplan-Meier survival analysis was conducted to estimate survival time. The log-rank test was used to compare groups. The independent prognostic effect of STAS was assessed by univariate and multivariate analyses using the Cox proportional hazard regression model. In all analyses, P<0.05 was considered statistically significant.
Results
Correlation of STAS with clinicopathologic features
Data from 244 patients (83 STAS-positive and 161 STAS-negative patients) were analysed. The clinicopathological characteristics are listed in Table 1, and a representative case is shown in Figure 2. There were 127 men and 117 women with an average age of 58 years (range, 35–83 years) in the cohort, and 141 (75.4%) had never smoked.
Table 1
Factors | Total patients (n=244) | STAS (−) (n=161) | STAS (+) (n=83) | P value |
---|---|---|---|---|
Gender | 0.95 | |||
Female | 117 (48.0) | 77 (47.8) | 40 (48.2) | |
Male | 127 (52.0) | 84 (52.2) | 43 (51.8) | |
Age (years) | 58 [52, 66] | 57 [51, 65] | 62.0 [53, 69] | 0.10 |
Smoke | n=187 | n=116 | n=71 | 0.11 |
Never | 141 (75.4) | 83 (71.6) | 58 (81.7) | |
Former or current | 46 (24.6) | 33 (28.4) | 13 (18.3) | |
Surgical resections | <0.01* | |||
Wedge resection | 28 (11.5) | 15 (9.3) | 13 (15.7) | |
Segmentectomy | 43 (17.6) | 37 (23.0) | 6 (7.2) | |
Lobectomy | 173 (70.9) | 109 (67.7) | 64 (77.1) | |
Location | 0.04 | |||
Central | 7 (2.9) | 2 (1.2) | 5 (6.0) | |
Peripheral | 237 (97.1) | 159 (98.8) | 78 (94.0) | |
Diameter (mm) | 20 [14.25, 29] | 19.0 [12, 27] | 23.0 [18, 31] | <0.01* |
GGO ratio† | 0 [0, 0.71] | 0.4 [0, 0.8] | 0.0 [0, 0] | <0.01* |
Density | <0.01* | |||
pGGO | 35 (14.3) | 33 (20.5) | 2 (2.4) | |
mGGO | 64 (26.2) | 57 (35.4) | 7 (8.4) | |
Solid | 145 (59.4) | 71 (44.1) | 74 (89.2) | |
Satellite lesions | <0.01* | |||
Absence | 233 (95.5) | 160 (99.4) | 73 (88.0) | |
Presence | 11 (4.5) | 1 (0.6) | 10 (12.0) | |
Homogeneity | 0.55 | |||
Absence | 31 (12.7) | 19 (11.8) | 12 (14.5) | |
Presence | 213 (87.3) | 142 (88.2) | 71 (85.5) | |
Shape | <0.01* | |||
Round or oval | 216 (88.5) | 156 (96.9) | 60 (72.3) | |
Irregular | 28 (11.5) | 5 (3.1) | 23 (27.7) | |
Margin | 0.30 | |||
Well-defined | 114 (46.7) | 79 (49.1) | 35 (42.2) | |
Ill-defined | 130 (53.3) | 82 (50.9) | 48 (57.8) | |
Pleural indentation | <0.01* | |||
Absence | 81 (33.2) | 65 (40.4) | 16 (19.3) | |
Presence | 163 (66.8) | 96 (59.6) | 67 (80.7) | |
Spiculation | <0.01* | |||
Absence | 143 (58.6) | 120 (74.5) | 23 (27.7) | |
Presence | 101 (41.4) | 41 (25.5) | 60 (72.3) | |
Air bronchogram | 0.73 | |||
Absence | 203 (83.2) | 133 (82.6) | 70 (84.3) | |
Presence | 41 (16.8) | 28 (17.4) | 13 (15.7) | |
Vascular convergence | <0.01* | |||
Absence | 129 (52.9) | 104 (64.6) | 25 (30.1) | |
Presence | 115 (47.1) | 57 (35.4) | 58 (69.9) | |
Vacuole sign | <0.01* | |||
Absence | 171 (70.1) | 103 (64.0) | 68 (81.9) | |
Presence | 73 (29.9) | 58 (36.0) | 15 (18.1) | |
Lymphadenopathy | 0.65 | |||
Absence | 196 (80.3) | 128 (79.5) | 68 (81.9) | |
Presence | 48 (19.7) | 33 (20.5) | 15 (18.1) | |
Cavity | 0.30 | |||
Absence | 235 (96.3) | 157 (97.5) | 78 (94.0) | |
Presence | 9 (3.7) | 4 (2.5) | 5 (6.0) | |
T stage‡ | 0.01 | |||
T1 | 156 (63.9) | 112 (69.6) | 44 (53.0) | |
T2 | 88 (36.1) | 49 (30.4) | 39 (47.0) | |
N stage‡ | <0.01* | |||
N0 | 185 (75.8) | 136 (84.5) | 49 (59.0) | |
N1 | 17 (7.0) | 10 (6.2) | 7 (8.4) | |
N2 | 37 (15.2) | 12 (7.5) | 25 (30.1) | |
N3 | 5 (2.0) | 3 (1.9) | 2 (2.4) | |
Histologic subtypes | <0.01* | |||
Lepidic predominant | 26 (10.7) | 22 (13.7) | 4 (4.8) | |
Acinar predominant | 105 (43.0) | 69 (42.9) | 36 (43.4) | |
Micropapillary predominant | 10 (4.1) | 1 (0.6) | 9 (10.8) | |
Papillary predominant | 81 (33.2) | 55 (34.2) | 26 (31.3) | |
Solid predominant | 20 (8.2) | 12 (7.5) | 8 (9.6) | |
Mucinous predominant | 2 (0.8) | 2 (1.2) | 0 (0.0) | |
Lymphatic invasion | <0.01* | |||
Absence | 205 (84.0) | 152 (94.4) | 53 (63.9) | |
Presence | 39 (16.0) | 9 (5.6) | 30 (36.1) | |
Perineural invasion | 0.69 | |||
Absence | 237 (97.1) | 157 (97.5) | 80 (96.4) | |
Presence | 7 (2.9) | 4 (2.5) | 3 (3.6) | |
Pleural invasion | <0.01* | |||
Absence | 188 (77.0) | 134 (83.2) | 54 (65.1) | |
Presence | 56 (23.0) | 27 (16.8) | 29 (34.9) | |
Surgical margin | <0.01* | |||
Absence | 237 (97.1) | 161 (100.0) | 76 (91.6) | |
Presence | 7 (2.9) | 0 (0.0) | 7 (8.4) | |
ALK rearrangement | n=115 | n=41 | n=74 | 0.21 |
Absence | 106 (92.2) | 40 (97.6) | 66 (89.2) | |
Presence | 9 (7.8) | 1 (2.4) | 8 (10.8) | |
EGFR | n=84 | n=42 | n=42 | 0.12 |
Absence | 35 (41.7) | 14 (33.3) | 21 (50.0) | |
Presence | 49 (58.3) | 28 (66.7) | 21 (50.0) | |
Death | n=219 | n=148 | n=71 | 0.01 |
No | 198 (90.4) | 140 (94.6) | 58 (81.7) | |
Yes | 21 (9.6) | 8 (5.4) | 13 (18.3) | |
Recurrence or distant metastases | n=219 | n=148 | n=71 | <0.01* |
No | 177 (80.8) | 130 (87.8) | 47 (66.2) | |
Yes | 42 (19.2) | 18 (12.2) | 24 (33.8) | |
OS (months) | 44 [40, 45] | 44 [43, 45] | 39 [36, 47] | <0.01* |
RFS (months) | 43 [36, 45] | 44 [42, 45] | 37 [27, 41] | <0.01* |
Data are presented as n (%) or median [IQR]. †, GGO ratio was measured as (maximum diameter of the GGO component/maximum diameter of the lesion); ‡, T and N staging was based on the IASLC 8th TNM Lung Cancer Staging System; *, P values <0.01. −, negative; +, positive. STAS, spread through air space; GGO, ground-glass opacity; pGGO, pure ground-glass opacity; mGGO, mixed ground-glass opacity; ALK, anaplastic lymphoma kinase; EGFR, epidermal growth factor receptor; OS, overall survival; RFS, recurrence-free survival; IQR, interquartile range; IASLC, International Association for the Study of Lung Cancer; TNM, tumour-node-metastasis.
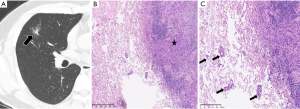
Lobectomy was performed in 70.9% (173 of 244) of patients; more STAS-positive patients (64 of 83, 77.1%) underwent lobectomy than STAS-negative patients (109 of 161, 67.7%) (P<0.01). A statistically significant relationship was observed between STAS and the histologic subtypes of lung adenocarcinoma; micropapillary or solid subtypes were more common among STAS-positive tumours (20.4% vs. 8.1%), while the lepidic subtype was more frequent among STAS-negative tumours (13.7% vs. 4.8%). Compared with patients with STAS-negative tumours, nodal involvement was more frequent in patients with STAS-positive tumours [41.0% (34 of 83) vs. 15.5% (25 of 161), P<0.01]. STAS was also related to a higher prevalence of lymphatic invasion (P<0.01), pleural invasion (P<0.01), and the T stage (P=0.01). No tumour metastasis was found in all patients from our study before surgery. The surgical margin was positive only for tumours with STAS [8.4% (7 of 83), P<0.01]. In addition, the ALK rearrangement analysis and EGFR mutation results were available for 115 and 84 patients, respectively, and there was no significant difference in ALK and EGFR status between STAS-positive and STAS-negative nodules (P=0.21, P=0.12, respectively).
Interobserver agreement in CT interpretation
The intraclass correlation coefficient for maximum tumour diameter was 0.972 (95% CI: 0.951–0.984), and that for the GGO ratio was 0.991 (95% CI: 0.984–0.995). Regarding other CT features, the concordance between the two observers was good, with k coefficients ranging between 0.650 and 0.921 (Table 2).
Table 2
CT features | N/Ntotal | Kappa (95% CI) | Kappa interpretation |
---|---|---|---|
Satellite lesions | 49/50 | 0.793 (0.663–0.877) | Almost perfect |
Intrapulmonary metastasis | 49/50 | 0.849 (0.750–0.911) | Almost perfect |
Homogeneity | 47/50 | 0.698 (0.523–0.817) | Substantial |
Shape | 49/50 | 0.903 (0.834–0.944) | Almost perfect |
Margin | 44/50 | 0.758 (0.610–0.855) | Almost perfect |
Pleural indentation | 47/50 | 0.880 (0.798–0.930) | Almost perfect |
Spiculation | 46/50 | 0.830 (0.719–0.899) | Almost perfect |
Vacuole sign | 48/50 | 0.921 (0.865–0.955) | Almost perfect |
Vascular convergence | 45/50 | 0.750 (0.598–0.850) | Almost perfect |
Lymphadenopathy | 48/50 | 0.854 (0.757–0.914) | Almost perfect |
Cavity | 48/50 | 0.650 (0.455–0.785) | Substantial |
CT, computed tomography; CI, confidence interval.
Correlation of STAS with CT features
In total, 199 (68 STAS-positive and 131 STAS-negative) and 45 (15 STAS-positive and 30 STAS-negative) patients were divided into the training and testing sets, respectively. Data from the 199 patients in the training set were used to establish the nomogram predictive model, and data from the 45 patients in the testing set were used to evaluate its performance. Table 3 summarizes the CT characteristics of lung adenocarcinoma in the training data set. Lesions were found in the central (7/244, 2.9%) and peripheral lung (237/244, 97.1%). Central tumours were observed more frequently among STAS-positive tumours than among STAS-negative tumours (7.4% vs. 1.5%, P=0.04). The maximum diameter of STAS-positive lesions was larger than that of STAS-negative lesions (24 vs. 19 mm, P<0.01). STAS-negative tumours had a higher ratio of GGO components {0.3 [0, 0.81] vs. 0 [0, 0], P<0.01}. The tumour density was significantly different between STAS-positive and STAS-negative tumours (P<0.01). STAS-positive tumours mainly presented as pure solid lesions (61 of 68, 89.7%). Of note, only 45.8% (60 of 131) of STAS-negative tumours presented as pure solid lesions, and 20.6% (27 of 131) of tumours presented as pure ground-glass lesions. STAS was also related to satellite lesions (P<0.01), shape (P<0.01), pleural indentation (P<0.01), spiculation (P<0.01), vascular convergence (P<0.01), and vacuole signs (P=0.01). No difference was found among other CT features (P>0.05).
Table 3
Factors | Train cohort | Test cohort | |||||
---|---|---|---|---|---|---|---|
STAS (−) (n=131) | STAS (+) (n=68) | P value | STAS (−) (n=30) | STAS (+) (n=15) | P value | ||
Gender | 0.64 | 0.39 | |||||
Female | 61 (46.6) | 34 (50.0) | 16 (53.3) | 6 (40.0) | |||
Male | 70 (53.4) | 34 (50.0) | 14 (46.7) | 9 (60.0) | |||
Age (years) | 57 [51, 65] | 61.5 [52, 69.75] | 0.17 | 62 [51.5, 68] | 63 [54, 68] | 0.35 | |
Smoke | n=95 | n=58 | 0.06 | n=21 | n=13 | >0.99 | |
Never | 68 (71.6) | 49 (84.5) | 15 (71.4) | 9 (69.2) | |||
Former or current | 27 (28.4) | 9 (15.5) | 6 (28.6) | 4 (30.8) | |||
Surgical resections | 0.04 | 0.04 | |||||
Wedge resection | 12 (9.2) | 11 (16.2) | 3 (10.0) | 2 (13.3) | |||
Segmentectomy | 28 (21.4) | 6 (8.8) | 9 (30.0) | 0 (0.0) | |||
Lobectomy | 91 (69.5) | 51 (75.0) | 18 (60.0) | 13 (86.7) | |||
Location | 0.04 | >0.99 | |||||
Center | 2 (1.5) | 5 (7.4) | 0 (0.0) | 0 (0.0) | |||
Peripheral | 129 (98.5) | 63 (92.6) | 30 (100.0) | 15 (100.0) | |||
Diameter (mm) | 19 [12, 28] | 24 [17.25, 31.75] | <0.01* | 18.5 [14, 24.75] | 23 [20, 28] | 0.01 | |
GGO ratio† | 0.3 [0, 0.81] | 0 [0, 0] | <0.01* | 0.65 [0, 1] | 0 [0, 0.13] | <0.01* | |
Density | <0.01* | <0.01* | |||||
pGGO | 27 (20.6) | 2 (2.9) | 6 (20.0) | 0 (0.0) | |||
mGGO | 44 (33.6) | 5 (7.4) | 13 (43.3) | 2 (13.3) | |||
Solid | 60 (45.8) | 61 (89.7) | 11 (36.7) | 13 (86.7) | |||
Satellite lesions | <0.01* | 0.10 | |||||
Absence | 130 (99.2) | 60 (88.2) | 30 (100.0) | 13 (86.7) | |||
Presence | 1 (0.8) | 8 (11.8) | 0 (0.0) | 2 (13.3) | |||
Homogeneity | 0.71 | 0.67 | |||||
Absence | 15 (11.5) | 9 (13.2) | 4 (13.3) | 3 (20.0) | |||
Presence | 116 (88.5) | 59 (86.8) | 26 (86.7) | 12 (80.0) | |||
Shape | <0.01* | 0.15 | |||||
Round or oval | 128 (97.7) | 49 (72.1) | 28 (93.3) | 11 (73.3) | |||
Irregular | 3 (2.3) | 19 (27.9) | 2 (6.7) | 4 (26.7) | |||
Margin | 0.46 | 0.39 | |||||
Well-defined | 61 (46.6) | 28 (41.2) | 18 (60.0) | 7 (46.7) | |||
Ill-defined | 70 (53.4) | 40 (58.8) | 12 (40.0) | 8 (53.3) | |||
Pleural indentation | <0.01* | 0.02 | |||||
Absence | 51 (38.9) | 14 (20.6) | 14 (46.7) | 2 (13.3) | |||
Presence | 80 (61.1) | 54 (79.4) | 16 (53.3) | 13 (86.7) | |||
Spiculation | <0.01* | <0.01* | |||||
Absence | 96 (73.3) | 19 (27.9) | 24 (80.0) | 4 (26.7) | |||
Presence | 35 (26.7) | 49 (72.1) | 6 (20.0) | 11 (73.3) | |||
Air bronchogram | 0.96 | 0.72 | |||||
Absence | 112 (85.5) | 58 (85.3) | 21 (70.0) | 12 (80.0) | |||
Presence | 19 (14.5) | 10 (14.7) | 9 (30.0) | 3 (20.0) | |||
Vascular convergence | <0.01* | <0.01* | |||||
Absence | 83 (63.4) | 21 (30.9) | 21 (70.0) | 4 (26.7) | |||
Presence | 48 (36.6) | 47 (69.1) | 9 (30.0) | 11 (73.3) | |||
Vacuole sign | 0.01 | 0.28 | |||||
Absence | 83 (63.4) | 55 (80.9) | 20 (66.7) | 13 (86.7) | |||
Presence | 48 (36.6) | 13 (19.1) | 10 (33.3) | 2 (13.3) | |||
Lymphadenopathy | 0.38 | 0.69 | |||||
Absence | 103 (78.6) | 57 (83.8) | 25 (83.3) | 11 (73.3) | |||
Presence | 28 (21.4) | 11 (16.2) | 5 (16.7) | 4 (26.7) | |||
Cavity | 0.30 | >0.99 | |||||
Absence | 127 (96.9) | 63 (92.6) | 30 (100.0) | 15 (100.0) | |||
Presence | 4 (3.1) | 5 (7.4) | 0 (0.0) | 0 (0.0) | |||
T stage‡ | 0.01 | 0.50 | |||||
T1 | 91 (69.5) | 35 (51.5) | 21 (70.0) | 9 (60.0) | |||
T2 | 40 (30.5) | 33 (48.5) | 9 (30.0) | 6 (40.0) | |||
N stage‡ | <0.01* | <0.01* | |||||
N0 | 109 (83.2) | 42 (61.8) | 27 (90.0) | 7 (46.7) | |||
N1 | 9 (6.9) | 5 (7.4) | 1 (3.3) | 2 (13.3) | |||
N2 | 10 (7.6) | 19 (27.9) | 2 (6.7) | 6 (40.0) | |||
N3 | 3 (2.3) | 2 (2.9) | – | – | |||
Histologic subtypes | 0.02 | 0.04 | |||||
Lepidic predominant | 15 (11.5) | 4 (5.9) | 7 (23.3) | 0 (0.0) | |||
Acinar predominant | 59 (45.0) | 30 (44.1) | 10 (33.3) | 6 (40.0) | |||
Micropapillary | 0 (0.0) | 5 (7.4) | 1 (3.3) | 4 (26.7) | |||
Papillary predominant | 44 (33.6) | 21 (30.9) | 11 (36.7) | 5 (33.3) | |||
Solid predominant | 11 (8.4) | 8 (11.8) | 1 (3.3) | 0 (0.0) | |||
Mucinous predominant | 2 (1.5) | 0 (0.0) | – | – | |||
Lymphatic invasion | <0.01* | <0.01* | |||||
Absence | 123 (93.9) | 45 (66.2) | 29 (96.7) | 8 (53.3) | |||
Presence | 8 (6.1) | 23 (33.8) | 1 (3.3) | 7 (46.7) | |||
Perineural invasion | 0.41 | >0.99 | |||||
Absence | 128 (97.7) | 65 (95.6) | 29 (96.7) | 15 (100.0) | |||
Presence | 3 (2.3) | 3 (4.4) | 1 (3.3) | 0 (0.0) | |||
Pleural invasion | <0.01* | 0.17 | |||||
Absence | 109 (83.2) | 45 (66.2) | 25 (83.3) | 9 (60.0) | |||
Presence | 22 (16.8) | 23 (33.8) | 5 (16.7) | 6 (40.0) | |||
Surgical margin | <0.01* | 0.10 | |||||
Absence | 131 (100.0) | 63 (92.6) | 30 (100.0) | 13 (86.7) | |||
Presence | 0 (0.0) | 5 (7.4) | 0 (0.0) | 2 (13.3) | |||
ALK rearrangement | n=35 | n=60 | 0.25 | n=6 | n=14 | >0.99 | |
Absence | 34 (97.1) | 53 (88.3) | 6 (100.0) | 13 (92.9) | |||
Presence | 1 (2.9) | 7 (11.7) | 0 (0.0) | 1 (7.1) | |||
EGFR | n=36 | n=36 | 0.15 | n=6 | n=6 | >0.99 | |
Absence | 13 (36.1) | 19 (52.8) | 1 (16.7) | 2 (33.3) | |||
Presence | 23 (63.9) | 17 (47.2) | 5 (83.3) | 4 (66.7) |
Data are presented as n (%) or median [IQR]. †, GGO ratio was measured as (maximum diameter of the GGO component/maximum diameter of the lesion); ‡, T and N staging was based on the IASLC 8th TNM Lung Cancer Staging System; *, P values <0.01. STAS, spread through air space; GGO, ground-glass opacity; pGGO, pure ground-glass opacity; mGGO, mix ground-glass opacity; ALK, anaplastic lymphoma kinase; EGFR, epidermal growth factor receptor; IQR, interquartile range; IASLC, International Association for the Study of Lung Cancer; TNM, tumour-node-metastasis.
Development and validation of the STAS prediction nomogram
After using the stepwise regression model for variable selection, the GGO ratio, shape and spicules were selected as the best subset of STAS predictors (Table 4), with typical signs shown in Figure 3. The nomogram containing these predictors is presented in Figure 4A. Tumours with irregular shape, spiculations and GGO ratio of 0 gets a “1” in the nomogram. The nomogram showed good discrimination, with areas under the curve (AUCs) of 0.875 [95% confidence interval (CI): 0.830–0.920] and 0.922 (95% CI: 0.853–0.990) in the training and testing data sets, respectively (Figure 4B). Meanwhile, the GGO ratio, spiculations and shape had sensitivities of 86.7%, 73.3%, and 26.7%, and specificities of 70%, 80%, and 93.3% in predicting STAS in the testing set, respectively (Figure 5). The nomogram combined three features outperformed all individual CT features. The optimal cut-off value of the GGO ratio was 0.43 with a sensitivity of 80.9% and a specificity of 62.6%.
Table 4
STAS | Coef. | St. err. | z-value | P value | Sig. |
---|---|---|---|---|---|
Intercept | −1.3582 | 0.2910 | −4.667 | <0.001 | *** |
GGO ratio | −1.4367 | 0.3935 | −3.651 | <0.001 | *** |
Spicule | 0.8558 | 0.2153 | 3.974 | <0.001 | *** |
Shape | 1.2544 | 0.2651 | 4.733 | <0.001 | *** |
***, P<0.001. STAS, spread through air space; coef., coefficient; st., standard; err., error; sig., significant; GGO, ground-glass opacity.
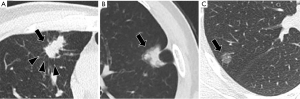
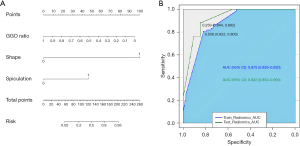
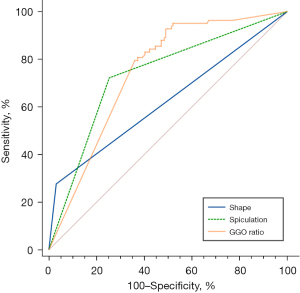
Calibration analysis and clinical use
In the validation cohort, the calibration curve showed high consistency between the predicted proportion of STAS and the actual proportion of STAS. The Hosmer-Lemeshow test showed a good fit, with a nonsignificant statistic (P=0.43), and the concordance index (C-index) of the nomogram for STAS prediction was 0.922 (Figure S1). The DCA for the nomogram is shown in Figure S2.
Survival analysis
In this study, the median follow-up time in patients was 44 months; the lost follow-up rate was 10.2% (25/244). Of all patients, 19.2% experienced recurrence or distant metastases, and 9.6% had died by the end of the follow-up period. STAS-positive patients had significantly worse OS and RFS than STAS-negative patients (both P<0.01) (Table 1). Figure 6 illustrated the OS and RFS based on the presence of STAS. OS and RFS at 5-year for STAS-positive patients was 63.1% and 59.5%, respectively. In the univariate analysis, age, adjuvant therapy, diameter, density (mGGO and solid), satellite lesions, spiculation, N stage (N0), histologic subtypes (micropapillary), STAS, lymphatic invasion and surgical margin were identified as significant predictors of both RFS and OS (all P<0.05) (Table 5). Segmentectomy, homogeneity, margin, pleural indentation, N stage (N2), histologic subtypes (solid), perineural invasion, and pleural invasion were also significantly related to RFS. GGO ratio was significantly associated with OS. Additional multivariate models were applied to identify independent prognostic factors (Table 6). Age [hazard ratio (HR), 1.1; 95% CI: 1.035–1.169; P=0.002], diameter (HR, 1.06; 95% CI: 1.04–1.11; P=0.03) and surgical margin (HR, 32.8; 95% CI: 6.8–158.3; P<0.001) remained significant predictors for OS. Adjuvant therapy (HR, 7.345; 95% CI: 2.52–21.41; P<0.001), N stage (N2) (HR, 0.239; 95% CI: 0.069–0.828; P=0.02) and surgical margin (HR, 15.6; 95% CI: 5.9–41.1; P<0.001) were found to be independent risk factors for RFS.
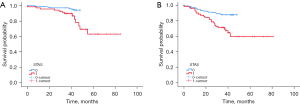
Table 5
Variables | RFS | OS | |||
---|---|---|---|---|---|
HR (95% CI) | P value | HR (95% CI) | P value | ||
Age | 1.05 (1.01, 1.08) | 0.01 | 1.13 (1.07, 1.20) | <0.001 | |
Surgical resections, segmentectomy (vs. others) | 4.944 (1.194, 20.48) | 0.02 | 4.896 (0.655, 36.603) | 0.12 | |
Adjuvant therapy | 17.4 (7.33, 41.65) | <0.001 | 4.54 (1.82, 11.35) | 0.001 | |
Diameter | 1.08 (1.06, 1.11) | 0.001 | 1.06 (1.02, 1.10) | 0.001 | |
GGO ratio | 0.96 (0.76, 1.21) | 0.71 | 1.16 (1.02, 1.31) | 0.02 | |
Density | |||||
mGGO (vs. others) | 36.15 (2.21, 592.07) | 0.01 | 8.12 (1.09, 60.62) | 0.04 | |
Solid (vs. others) | 0.029 (0.004, 0.214) | <0.001 | 0.132 (0.03, 0.568) | 0.007 | |
Satellite lesions | 5.18 (2.18, 12.31) | 0.001 | 3.53 (1.01, 12.33) | 0.04 | |
Homogeneity | 0.43 (0.21, 0.87) | 0.01 | 0.472 (0.173, 1.291) | 0.14 | |
Margin | 2.5 (1.26, 4.98) | 0.009 | 2.02 (0.78, 5.22) | 0.14 | |
Pleural indentation | 25.7 (3.5, 187.2) | 0.001 | 43.38 (1.01, 1871.5) | 0.05 | |
Spiculation | 3.7 (1.9, 7.2) | <0.001 | 3.96 (1.52, 10.33) | 0.005 | |
N stage | |||||
N0 (vs. others) | 5.69 (3.05, 10.61) | <0.001 | 2.696 (1.13, 6.427) | 0.02 | |
N2 (vs. others) | 0.181 (0.097, 0.337) | <0.001 | 0.389 (0.151, 1.004) | 0.05 | |
Histologic subtypes | |||||
Micropapillary (vs. others) | 0.354 (0.126, 0.994) | 0.04 | 0.153 (0.051, 0.463) | 0.001 | |
Solid (vs. others) | 0.252 (0.120, 0.528) | <0.001 | 0.352 (0.118, 1.049) | 0.06 | |
STAS | 3.3 (1.8, 6.1) | <0.001 | 4.21 (1.69, 10.51) | 0.002 | |
Lymphatic invasion | 3.82 (2.0, 7.3) | <0.001 | 3.042 (1.171, 7.901) | 0.02 | |
Perineural invasion | 5.0 (1.8, 14.0) | 0.01 | 2.334 (0.31, 17.56) | 0.41 | |
Pleural invasion | 2.7 (1.5, 5.0) | 0.001 | 1.11 (0.403, 3.054) | 0.84 | |
Surgical margin | 15.6 (5.9, 41.1) | <0.001 | 43.24 (14.17, 132.0) | <0.001 |
RFS, recurrence-free survival; OS, overall survival; HR, hazard ratio; CI, confidence interval; GGO, ground-glass opacity; mGGO, mix ground-glass opacity; STAS, spread through air space.
Table 6
Variables | RFS | OS | |||
---|---|---|---|---|---|
HR (95% CI) | P value | HR (95% CI) | P value | ||
Age | 1.034 (0.99, 1.08) | 0.14 | 1.1 (1.035, 1.169) | 0.002 | |
Adjuvant therapy | 7.345 (2.52, 21.41) | <0.001 | 1.654 (0.485, 5.642) | 0.42 | |
Diameter | 1.038 (0.998, 1.08) | 0.06 | 1.06 (1.04, 1.11) | 0.03 | |
N2 (vs. other) | 0.239 (0.069, 0.828) | 0.02 | 0.763 (0.258, 2.258) | 0.62 | |
STAS | 0.521 (0.196, 1.385) | 0.19 | 0.515 (0.12, 2.206) | 0.37 | |
Surgical margin | 15.6 (5.9, 41.1) | <0.001 | 32.8 (6.8, 158.3) | <0.001 |
RFS, recurrence-free survival; OS, overall survival; HR, hazard ratio; CI, confidence interval; STAS, spread through air space.
Discussion
In our study, the incidence of STAS in our hospital was approximately 6.3% between December 2015 and March 2021, which was lower than that in previous reports, which ranged from 15% to 50% (13). This result may have occurred because we excluded stage T3 and T4 patients. In brief, a nomogram for STAS prediction was developed by integrating the predictors of shape, GGO ratio and spicules. Using these factors, our nomogram had optimal discrimination and well-fitted calibration curves, with high AUCs in both the training and testing data sets.
STAS is typically associated with the more aggressive histological subtypes and limited resection is significantly associated with a higher risk of recurrence than lobectomy (4,14). Thus, preoperative detection of STAS could help select an appropriate surgery type. However, it is not limited to aggressive histological subtypes. In this study, the main histological subtypes of STAS-positive adenocarcinomas were acinar and papillary. Meanwhile, the decision to perform a lobectomy takes into account multiple factors, such as the patient’s health condition, tumour size, location, surgical margin and so on.
In terms of pathological factors, we observed that advanced histologic subtypes and pleural and lymphatic invasion were related to STAS, suggesting a highly aggressive feature; this was also confirmed in previous studies (15,16). In particular, our study found that solid and micropapillary subtypes were more common among STAS-positive adenocarcinomas, while a lepidic predominance was more common among STAS-negative adenocarcinomas, similar to other reports (17,18). Notably, the presence of solid or micropapillary components and vascular and pleural invasion have been considered to be high-risk factors contributing to tumour recurrence (19-21). These results indicated that STAS is an invasive mechanism and may lead to tumour recurrence and poor prognosis. Most STAS-positive patients in our study had higher T stages and N stages, suggesting that STAS was correlated with advanced tumour stage. According to previous studies (4,18), STAS is strongly associated with ALK rearrangement but negatively associated with EGFR mutation. A possible explanation could be that STAS occurs frequently in tumours with solid or micropapillary components, which are significantly associated with ALK positivity and wild-type EGFR (18,22,23). Although no statistical significance for ALK rearrangement or wild-type EGFR was observed in our cohort, increased ALK rearrangement and wild-type EGFR could be found in STAS-positive patients. Besides, the lack of statistically significant associations may be related to the small sample size, as well as to the low number of people undergoing ALK (47.1%, 115/244) and EGFR testing (34.4%, 84/244).
Regarding CT images, STAS-positive adenocarcinoma was independently associated with the manifestation of a lower GGO/tumour ratio, in line with previous reports (6,24). The reason might be that the pathological results of this study showed that STAS positivity is associated with solid or micropapillary subtypes because adenocarcinoma with solid or micropapillary predominant subtypes rarely present with GGO on CT. These results indicate that a solid component is a risk factor for STAS. The presence of spiculation and irregular shape also demonstrated strong discriminative power in our nomogram. These signs may be caused by infiltrative tumour growth, indicating the invasion of STAS (25). Interestingly, there was no significant relationship between satellite lesions and STAS. This is consistent with the findings of Kim et al. (6), who revealed that STAS was a microscopic phenomenon, while satellite lesions were defined as macroscopic tumours spreading through the airways on CT. Another possible explanation is that the incidence of satellite lesions is low.
Our present nomogram showed excellent predictive value in both the training set (AUC, 0.875) and testing set (AUC, 0.922). Qi et al. found that the AUC was greater in a model that used the consolidation tumour ratio than in a model that used the long diameter of the entire lesion to predict STAS (AUC, 0.76 vs. 0.64) (26). A model by Zhang et al. (27), which included a combination of an air bronchogram, the maximum tumour diameter, maximum solid component diameter and consolidation/tumour ratio performed well, with an AUC of 0.726. The predictive effect of our nomogram model, including the shape, GGO ratio and spicules, showed better results than those of the above two studies. Possible explanations for the above differences could include the inclusion of CT features and the study designs. Moreover, the nomogram is a good clinical prediction model with high accuracy.
Studies (3,28) have reported that the presence of STAS is closely correlated with clinical outcome. In the study, patients with STAS had significantly worse RFS and OS than those without STAS, and the presence of STAS was related to the recurrence and distant metastases. However, in multivariate models, STAS was not an independent prognostic factor for both RFS and OS. This may be related to different inclusion criteria. Masai et al. (3) included patients with early-stage lung cancer who underwent limited resection, while Shimomura et al. (28) included patients with completely resected stage I lung adenocarcinoma. In this study, we did not restrict factors such as patient treatment methods. Thus, the prognostic impact of STAS on different populations is controversial and should be further explored in future studies.
So far, several studies (29-31) have utilized CT-based radiomics and machine learning to predict STAS in lung adenocarcinoma. The predictive models achieved AUCs of 0.75–0.84, which was lower than the results of this study. Although radiomics can extract high-throughput quantitative features from medical images which are invisible to the human, the stability and high efficiency of the radiomics model relies on a large amount of image data. Moreover, the interpretability of radiomics is poor, making it challenging to explain the relationship between these features and STAS, thus restricting the application of radiomics in clinical practice. Nevertheless, future research and technological advancements will help overcome these limitations and further promote the application of radiomics in clinical practice.
This study had some limitations. First, this analysis was based on a small sample from a single institution; thus, it is necessary to validate the results in large multicenter populations so that they are more generalizable. Second, this retrospective study excluded patients with tumours classified as T3 or T4 stage because they should undergo lobectomy regardless of STAS status, and this might have introduced selection bias. Third, as this was a retrospective study, patients were routinely given unenhanced scans preoperatively in our hospital. Thus, the degree of tumour enhancement cannot be observed.
Conclusions
In summary, STAS is a risk factor for poor outcome in lung adenocarcinoma and lobectomy is usually selected for it in clinical practice. Preoperative prediction of STAS could help select the appropriate surgical approach and improve prognosis. Thus, we developed and validated a nomogram based on radiographic variables that can be applied to facilitate the individualized prediction of STAS before surgery. In the future, additional studies should be designed with larger cohorts to confirm these findings.
Acknowledgments
Funding: None.
Footnote
Reporting Checklist: The authors have completed the TRIPOD reporting checklist. Available at https://jtd.amegroups.com/article/view/10.21037/jtd-23-1871/rc
Data Sharing Statement: Available at https://jtd.amegroups.com/article/view/10.21037/jtd-23-1871/dss
Peer Review File: Available at https://jtd.amegroups.com/article/view/10.21037/jtd-23-1871/prf
Conflicts of Interest: All authors have completed the ICMJE uniform disclosure form (available at https://jtd.amegroups.com/article/view/10.21037/jtd-23-1871/coif). C.D. is an employee of Bayer Healthcare. The other authors have no conflicts of interest to declare.
Ethical Statement: The authors are accountable for all aspects of the work in ensuring that questions related to the accuracy or integrity of any part of the work are appropriately investigated and resolved. The study was conducted in accordance with the Declaration of Helsinki (as revised in 2013). This retrospective study was approved by the Ethics Committee of Tongji Medical College of Huazhong University of Science and Technology (approval No. S377), and individual consent for this retrospective analysis was waived.
Open Access Statement: This is an Open Access article distributed in accordance with the Creative Commons Attribution-NonCommercial-NoDerivs 4.0 International License (CC BY-NC-ND 4.0), which permits the non-commercial replication and distribution of the article with the strict proviso that no changes or edits are made and the original work is properly cited (including links to both the formal publication through the relevant DOI and the license). See: https://creativecommons.org/licenses/by-nc-nd/4.0/.
References
- de Margerie-Mellon C, VanderLaan PA, Bankier AA. CT Manifestations of Tumor Spread through Air Spaces in Lung Adenocarcinoma: Different Pathways toward Common Perspectives. Radiology 2019;290:271-72. [Crossref] [PubMed]
- Travis WD, Brambilla E, Nicholson AG, et al. The 2015 World Health Organization Classification of Lung Tumors: Impact of Genetic, Clinical and Radiologic Advances Since the 2004 Classification. J Thorac Oncol 2015;10:1243-60. [Crossref] [PubMed]
- Masai K, Sakurai H, Sukeda A, et al. Prognostic Impact of Margin Distance and Tumor Spread Through Air Spaces in Limited Resection for Primary Lung Cancer. J Thorac Oncol 2017;12:1788-97. [Crossref] [PubMed]
- Kadota K, Kushida Y, Kagawa S, et al. Limited Resection Is Associated With a Higher Risk of Locoregional Recurrence than Lobectomy in Stage I Lung Adenocarcinoma With Tumor Spread Through Air Spaces. Am J Surg Pathol 2019;43:1033-41. [Crossref] [PubMed]
- Iasonos A, Schrag D, Raj GV, et al. How to build and interpret a nomogram for cancer prognosis. J Clin Oncol 2008;26:1364-70. [Crossref] [PubMed]
- Kim SK, Kim TJ, Chung MJ, et al. Lung Adenocarcinoma: CT Features Associated with Spread through Air Spaces. Radiology 2018;289:831-40. [Crossref] [PubMed]
- Punt CJ, Buyse M, Köhne CH, et al. Endpoints in adjuvant treatment trials: a systematic review of the literature in colon cancer and proposed definitions for future trials. J Natl Cancer Inst 2007;99:998-1003. [Crossref] [PubMed]
- Eberhardt WE, Mitchell A, Crowley J, et al. The IASLC Lung Cancer Staging Project: Proposals for the Revision of the M Descriptors in the Forthcoming Eighth Edition of the TNM Classification of Lung Cancer. J Thorac Oncol 2015;10:1515-22.
- Akaike H. Information theory and an extension of the maximum likelihood principle. In: 2nd International Symposium on Information Theory. Budapest: Akadémiai Kiadó; 1973;267-81.
- Burnham KP, Anderson DR. Multimodel inference: understanding AIC and BIC in model selection. Sociological Methods & Research 2004;33:261-304. [Crossref]
- Harrell FE. rms: Regression modeling strategies. R Package version 4.1.0. Available online: https://cran.r-project.org/package=rms
- Vickers AJ, Cronin AM, Elkin EB, et al. Extensions to decision curve analysis, a novel method for evaluating diagnostic tests, prediction models and molecular markers. BMC Med Inform Decis Mak 2008;8:53. [Crossref] [PubMed]
- Linden PA. Commentary: Spread the news: Spread through air spaces matters. J Thorac Cardiovasc Surg 2022;163:285. [Crossref] [PubMed]
- Ren Y, Xie H, Dai C, et al. Prognostic Impact of Tumor Spread Through Air Spaces in Sublobar Resection for 1A Lung Adenocarcinoma Patients. Ann Surg Oncol 2019;26:1901-8. [Crossref] [PubMed]
- Han YB, Kim H, Mino-Kenudson M, et al. Tumor spread through air spaces (STAS): prognostic significance of grading in non-small cell lung cancer. Mod Pathol 2021;34:549-61. Erratum in: Mod Pathol 2021. [Crossref] [PubMed]
- Cao L, Jia M, Sun PL, et al. Histopathologic features from preoperative biopsies to predict spread through air spaces in early-stage lung adenocarcinoma: a retrospective study. BMC Cancer 2021;21:913. [Crossref] [PubMed]
- Chae M, Jeon JH, Chung JH, et al. Prognostic significance of tumor spread through air spaces in patients with stage IA part-solid lung adenocarcinoma after sublobar resection. Lung Cancer 2021;152:21-6. [Crossref] [PubMed]
- Lee JS, Kim EK, Kim M, et al. Genetic and clinicopathologic characteristics of lung adenocarcinoma with tumor spread through air spaces. Lung Cancer 2018;123:121-6. [Crossref] [PubMed]
- Kadota K, Villena-Vargas J, Yoshizawa A, et al. Prognostic significance of adenocarcinoma in situ, minimally invasive adenocarcinoma, and nonmucinous lepidic predominant invasive adenocarcinoma of the lung in patients with stage I disease. Am J Surg Pathol 2014;38:448-60. [Crossref] [PubMed]
- Ujiie H, Kadota K, Chaft JE, et al. Solid Predominant Histologic Subtype in Resected Stage I Lung Adenocarcinoma Is an Independent Predictor of Early, Extrathoracic, Multisite Recurrence and of Poor Postrecurrence Survival. J Clin Oncol 2015;33:2877-84. [Crossref] [PubMed]
- Hung JJ, Yeh YC, Jeng WJ, et al. Predictive value of the international association for the study of lung cancer/American Thoracic Society/European Respiratory Society classification of lung adenocarcinoma in tumor recurrence and patient survival. J Clin Oncol 2014;32:2357-64. [Crossref] [PubMed]
- Li P, Gao Q, Jiang X, et al. Comparison of Clinicopathological Features and Prognosis between ALK Rearrangements and EGFR Mutations in Surgically Resected Early-stage Lung Adenocarcinoma. J Cancer 2019;10:61-71. [Crossref] [PubMed]
- Yuan M, Zhang YD, Pu XH, et al. Comparison of a radiomic biomarker with volumetric analysis for decoding tumour phenotypes of lung adenocarcinoma with different disease-specific survival. Eur Radiol 2017;27:4857-65. [Crossref] [PubMed]
- Ledda RE, Milanese G, Gnetti L, et al. Spread through air spaces in lung adenocarcinoma: is radiology reliable yet? J Thorac Dis 2019;11:S256-61. [Crossref] [PubMed]
- Kim HY, Shim YM, Lee KS, et al. Persistent pulmonary nodular ground-glass opacity at thin-section CT: histopathologic comparisons. Radiology 2007;245:267-75. [Crossref] [PubMed]
- Qi L, Xue K, Cai Y, et al. Predictors of CT Morphologic Features to Identify Spread Through Air Spaces Preoperatively in Small-Sized Lung Adenocarcinoma. Front Oncol 2020;10:548430. [Crossref] [PubMed]
- Zhang Z, Liu Z, Feng H, et al. Predictive value of radiological features on spread through air space in stage cIA lung adenocarcinoma. J Thorac Dis 2020;12:6494-504. [Crossref] [PubMed]
- Shimomura M, Miyagawa-Hayashino A, Omatsu I, et al. Spread through air spaces is a powerful prognostic predictor in patients with completely resected pathological stage I lung adenocarcinoma. Lung Cancer 2022;174:165-71. [Crossref] [PubMed]
- Jiang C, Luo Y, Yuan J, et al. CT-based radiomics and machine learning to predict spread through air space in lung adenocarcinoma. Eur Radiol 2020;30:4050-7. [Crossref] [PubMed]
- Onozato Y, Nakajima T, Yokota H, et al. Radiomics is feasible for prediction of spread through air spaces in patients with nonsmall cell lung cancer. Sci Rep 2021;11:13526. [Crossref] [PubMed]
- Jin W, Shen L, Tian Y, et al. Improving the prediction of Spreading Through Air Spaces (STAS) in primary lung cancer with a dynamic dual-delta hybrid machine learning model: a multicenter cohort study. Biomark Res 2023;11:102. [Crossref] [PubMed]