Relationship of various COVID-19 antibody titer with individual characteristics and prediction of future epidemic trend in Xiamen City, China
Highlight box
Key findings
• A study of 1,191 public health workers unveiled age as a critical factor influencing immunoglobulin G (IgG), immunoglobulin M, and neutralizing antibody (NAb) titers, while past pulmonary conditions and smoking affected specific antibody levels. Notably, IgG decline mirrored epidemic trends, suggesting a potential correlation.
What is known and what is new?
• Factors, such as age, gender, smoking, severity of symptoms and so on, potentially affect antibody level.
• This manuscript uniquely ties IgG decay to epidemic trends, shedding light on its implications for coronavirus disease 2019 dynamics.
What is the implication, and what should change now?
• Understanding age-linked antibody variations and their connection to infection trends calls for tailored strategies against reinfection. Further exploration of influential factors and targeted protective measures are imperative in shaping effective long-term defenses.
Introduction
The global impact of coronavirus disease 2019 (COVID-19), caused by the severe acute respiratory syndrome coronavirus 2 (SARS-CoV-2), has been profound, and efforts to mitigate its effects have been ongoing for over 3 years (1). As of 13 August 2023, over 769 million confirmed cases and over 6.9 million deaths have been reported globally (1). Since December 2022, most countries have been adapting their emergency responses, and attention has turned to understanding the duration of the immune response to infection (2). As vaccination remains a cornerstone for controlling the COVID-19 infection, antibodies obtained from infection also play a role in warding off reinfection (3).
The humoral immune responses, whether triggered by COVID-19 infection or vaccination, are crucial to fight a second or third infection in the current massive reinfection circumstances (4). Humoral immunity is characterized by the production of antibodies by B cells as a response to antigens. Researchers concluded that after stimulating the immune reaction to COVID-19, the first antibody to appear is immunoglobulin M (IgM), succeeded by immunoglobulin A (IgA) and, notably, immunoglobulin G (IgG). However, the duration of these antibody types varies (5). IgM, for example, typically persists for only 20–30 days, while IgG, detectable approximately 10–14 days post-infection and peaking around day 25, provides longer-lasting immunity. Serum IgA levels rapidly decline, with seropositivity diminishing after 2 months (4), although neutralizing IgA can remain detectable in saliva for an extended period (49–73 days post-symptoms) (6). Importantly, a study suggested that IgA is a significant isotype in nearly neutralizing serum activity, whereas IgG is a key component of serum neutralizing antibodies (NAbs) following SARS-CoV-2 infection (7).
NAb titers after mild SARS-CoV-2 infection were reported to be comparable to those induced by first-generation COVID-19 vaccines (8), and second-vaccine doses further enhance cross-neutralizing activity against variants of concern. The persistence of protective immune responses is linked to the time since the initial infection and the robustness of the peak antibody response (2,3,8,9).
With SARS-CoV-2 persisting in the human population for over 3 years, reports of reinfection cases have become increasingly prevalent. Studies about the relationship between antibody duration (arising from infection or vaccination) and reinfection risk have been conducted. A study that followed 12,541 healthcare workers at Oxford University Hospital revealed that the incidence of SARS-CoV-2 was inversely proportional to baseline titers of antibodies (10). Another study about the reinfection of SARS-CoV-2 implicated that herd immunity from infection is unlikely to be sufficient to eliminate the virus if reinfections are common (11). However, it is unneglectable that there is no evidence confirming that current intramuscular vaccines can reduce shedding in the event of reinfection. What’s more, as SARS-CoV-2 continues to mutate, the immune escape of variants should also be taken into consideration when exploring antibody dynamics (12).
During the COVID-19 pandemic, researchers have come up with various methods to simulate and predict various epidemic scenarios. Transmission dynamics models have played a crucial role in this context (13-15). In light of the current situation, where mass virus testing has been postponed among the general population, constructing models that accurately reflect the real-world situation has become essential.
In this study, we examined the antibody titers of 1,191 public health workers from the Xiamen Center for Disease Control. Our objectives were to investigate potential relationships between individual characteristics and antibody titers, predict future outbreaks by optimizing existing COVID-19 transmission dynamics models, and explore the interplay between epidemic peaks and the decay of specific antibody types within the population. We present this article in accordance with the TRIPOD reporting checklist (available at https://jtd.amegroups.com/article/view/10.21037/jtd-23-1516/rc).
Methods
Study design
This study aims to investigate antibody titer dynamics among Xiamen healthcare workers through three key stages. First, we collected data via questionnaires distributed among healthcare staff in the Xiamen Healthcare System. This yielded 1,191 eligible participants, selected based on SARS-CoV-2 infection or vaccination status post-December 2022. Subsequently, serum antibody testing occurred at monthly intervals from February to May to track titer variations. Finally, we employed a high-dimensional transmission dynamics model to predict future epidemic trends, examining correlations between antibody decay and epidemic peaks. The study was conducted in accordance with the Declaration of Helsinki (as revised in 2013). The study was approved by the ethics committee of the Xiamen Center for Disease Control [XJK/LLSC(2022)004] and participants signed the agreement consent before filling out the questionnaire.
Data collection
This study collected data in two stages. Initially, questionnaires were distributed among healthcare workers in Xiamen City, gathering individual information, health history, and COVID-19 vaccination records. For those previously infected with SARS-CoV-2, additional infection details were gathered. Based on prior research indicating that NAbs for COVID-19 might persist for a maximum of 73 days (6), questionnaires that were incomplete or lacking essential infection-related information were excluded from the study, and 1,191 valid samples remained. Subsequently, a monthly serum antibody test tracked antibody decay from February to May.
Explore the epidemiological characteristics as well as correlation of individual properties and antibody titer for COVID-19
This study investigated the correlation between individual baseline properties (age, height, weight, and previous health conditions) and COVID-19 antibody titers. Multivariate regression models were established to analyze the relationship, with regression coefficients (β), 95% confidence intervals (CIs), and standardized regression coefficients (β) calculated. Before establishing the regression model, we carried out variable distribution description and Spearman’s correlation analysis, and eliminated variables with strong correlation (r>0.7). The optimal model was selected using backward elimination, Bayesian information criterion (BIC), and Akaike information criterion (AIC).
The formula of the model is as follows:
A group-based trajectory model (GBTM) was applied to analyze the longitudinal serum antibody data (16). The GBTM model identified potential clusters with similar antibody trajectory patterns. The study hypothesized that the population’s serum could be divided into up to five categories, namely, gradual growth type, gradual decline type, unchanged type, growth and then decline type, and decline and then growth type, reflecting different patterns of change in antibody levels. The highest order of the model was set to 3, i.e., the number of potential subgroups in the population was 1–5 groups, 0–3 orders, with the order reflecting the degree of rapidity or slowness of the trend of change. BIC and AIC were used to assess the model’s fit, with an emphasis on minimizing the absolute value of the index.
χ2 analysis and ANOVA were used to compare demographic differences between different serologic change patterns. Multiple linear and logistic regression analyses were conducted to further explore demographic differences. Statistical significance was set at P<0.05.
Prediction and simulation of the current COVID-19 epidemic by constructing a high-dimensional transmission dynamics model
According to the natural history and vaccination situation for COVID-19 in Xiamen City, we have constructed a high-dimensional transmission dynamics model. This is a well-organized ordinary differential equation (ODE) model in tensor form, which includes factors of age, vaccine, regional contact, stages in disease progress, and critical events such as population growth, and migration. In this model, we grouped the total population N into susceptible, V; exposed, E; asymptomatic infection, A; pre-symptomatic infection, F; symptomatic infection, I; removed/recovered that will not be reinfected, Rp; recovered and will possibly be reinfected, R; therefore, this high-dimensional transmission dynamics model could also be named as VEAFIRPRV model (Figure 1).
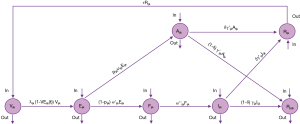
The model settings are detailed in Appendix 1.
All the values of parameters in the model are presented in Table 1.
Table 1
Parameters | Definition | Unit | Value | Source |
---|---|---|---|---|
p | Proportion of asymptomatic infections | 1 | 0.08 | Reference (17-21) |
ω | Inverse of the average latent period | Day-1 | 1/3 | |
ω' | Inverse of the average incubation period from exposed (e) to pre-symptomatic infection (F) | Day-1 | 1/2 | |
ω'' | Inverse of the average incubation period from pre-symptomatic infection (F) to infections (I) | Day-1 | 1/3 | |
γ | Recovery or removal rate for symptomatic infections | 1 | 1/6.8 | |
γ' | Recovery or removal rate for asymptomatic infections | 1 | 1/6.8 | |
τ | Inverse of the average duration of losing immunity | Day-1 | 1/180 | |
δ | Proportion of those become susceptible again after recovery | 1 | 0.7 |
COVID-19, coronavirus disease 2019.
The functions for the high-dimensional transmission dynamics model (VEAFIRPRV model) are as follows:
After building the model, we applied it in the prediction and simulation for the future COVID-19 epidemic. We used the incidence rate and infection rate as the indexes for the scale of the COVID-19 epidemic in Xiamen City and carried out interventions that meet the current policies.
Statistical analysis
Data entry and organization related to this study were performed in Excel 2019. Continuous quantitative variables were described by median ± interquartile range (IQR), and categorical qualitative variables by percentages. Statistical analysis was performed by SPSS version 22.0, and differences were statistically significant at P<0.05. For a cross-sectional study, a multivariant regression model was constructed via Python’s statsmodels package. Then longitudinal data was analyzed by applying the GBTM model on traj package of Stats 17.0. High-dimensional transmission model was processed on Matlab R2023a, differential equations were solved using the fourth-order Runge Kutta method, and model convergence was based on the least root mean square (LRMS), further using the coefficient of determination (R2) to determine the goodness of fit. Graphs were plotted using CorelDRAW 2020.
Results
Crowd distribution for the samples
On February 10th, 2023, we initiated data collection by distributing questionnaires to healthcare workers in Xiamen City, including staff from hospitals, Centers for Disease Control and Prevention (CDCs), and primary healthcare organizations. Since it is difficult to collect both infection and serum antibody information from the general population or implement random sampling in the targeted healthcare workers, we have implemented convenience sampling. These questionnaires aimed to collect baseline information about the participants, which included details about their health conditions, COVID-19 vaccination status, and information regarding their previous SARS-CoV-2 infections, such as symptoms and infection dates. Subsequently, serum antibody tests were conducted on the participants. A total of 1,344 questionnaires were collected along with their corresponding serum antibody results. To establish clear inclusion and exclusion criteria for our study, we considered that serum antibodies could persist for a maximum of 73 days (6). We included individuals who had developed antibodies within 73 days before serum testing. For those who were infected by SARS-CoV-2, we considered the day of symptom onset as the antibody-emerging date, while for those who were not infected, we used the last vaccination date as the antibody-emerging date. As a result, 1,191 participants met our inclusion criteria and were included in the study.
Of the 1,191 participants included in the study, 1,056 individuals were confirmed to be infected with COVID-19 (infections), and 135 individuals were not infected (no infections), as indicated in Table 2. Statistical analysis revealed that there were no significant differences in terms of gender (χ2=0.09, P=0.92) and age groups (χ2=9.639, P=0.08) between infected and non-infected individuals. However, there is statistical significance in the difference of the addresses (χ2=30.053, P<0.05) and vaccination status between infected and non-infected groups (χ2=376.706, P<0.05). Notably, the distributions of antibody titers for IgG, IgM, and NAb were found to be similar, as depicted in Figure 2.
Table 2
Categories | Non-infected | Infected | Tests |
---|---|---|---|
Total, n | 135 | 1,056 | |
Gender, n | χ2=0.09, P=0.92 | ||
Male | 39 | 301 | |
Female | 96 | 755 | |
Age (years), n | χ2=9.639, P=0.08 | ||
<20 | 0 | 3 | |
21–30 | 48 | 263 | |
31–40 | 50 | 462 | |
41–50 | 23 | 233 | |
51–60 | 14 | 87 | |
>61 | 0 | 6 | |
Address, n | χ2=30.053, P<0.05 | ||
Jinjiang City | 0 | 1 | |
Haicang | 8 | 79 | |
Huli | 21 | 118 | |
Jimei | 32 | 140 | |
Siming | 36 | 517 | |
Tongan | 22 | 105 | |
Xiangan | 16 | 95 | |
Longhai | 0 | 1 | |
Vaccination, n | χ2=376.706, P<0.05 | ||
Fundamental immune | 1 | 61 | |
Booster 1 | 7 | 676 | |
Booster 2 | 87 | 304 | |
Booster 3 | 40 | 7 |
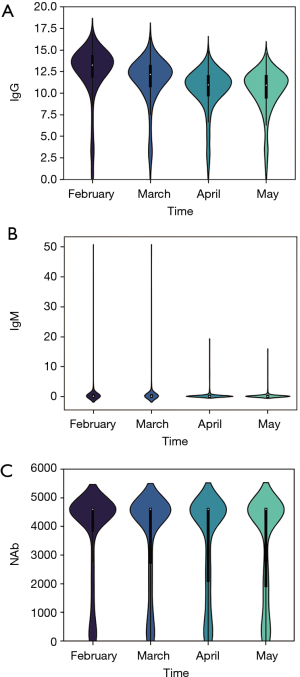
Correlation of individual properties and antibody titer for COVID-19
The distribution of individual properties and the results of Spearman correlation analysis are presented in Figures 3,4. Notably, none of the individual properties exhibited strong correlations with each other (r<0.7), and regression coefficients (β), and 95% CIs for IgG, IgM, and NAb with individual baseline properties are provided in Figures S1-S3, then the standardized regression coefficients (β) for these different antibodies are presented in Figures S4-S6. Consequently, all individual properties were included in the multilinear regression (MLR) model.
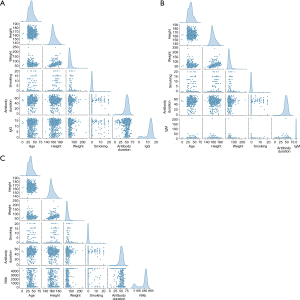
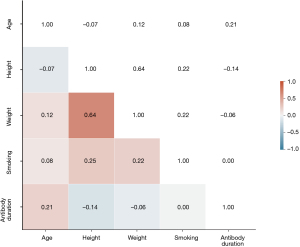
In the MLR model, we have included the following factors: age, height, weight, smoking status, and antibody duration. Model selection was based on the BIC and AIC scores. The model with the lowest score was deemed the optimized MLR model (Table 3). The analysis revealed that a history of pulmonary disease and hospitalization had a significant impact on IgG titer. The optimized MLR model for IgG was determined to be . However, none of the examined factors were found to be statistically significant for IgM titer, leading to the conclusion that was the mixed MLR model. For NAb titers, we found that sex, smoking, family history of pulmonary disease, and hospitalization significantly influenced the titer. The optimized MLR model for NAb was determined to be .
Table 3
Antibody | MLR model | AIC | BIC |
---|---|---|---|
IgG | 6,580.65 | 8,501.69 | |
6,578.65 | 8,494.61 | ||
6,576.66 | 8,487.75 | ||
6,576.62 | 8,592.98 | ||
6,574.67 | 8,586.66 | ||
6,572.91 | 8,582.96 | ||
6,571.19 | 8,579.77 | ||
6,564.77 | 8,616.74 | ||
6,561.42 | 8,611.84 | ||
6,560.03 | 8,613.38 | ||
6,559.26 | 8,623.92 | ||
6,559.28 | 8,645.66 | ||
6,557.90 | 8,647.51 | ||
IgM | 7,946.09 | 44,470.88 | |
7,935.07 | 44,471.87 | ||
7,933.07 | 44,464.98 | ||
7,925.57 | 44,540.34 | ||
7,923.60 | 44,534.36 | ||
7,921.63 | 44,528.56 | ||
7,917.96 | 44,529.20 | ||
7,916.00 | 44,523.82 | ||
7,914.04 | 44,518.57 | ||
7,912.11 | 44,514.47 | ||
7,910.25 | 44,513.71 | ||
7,908.85 | 44,533.38 | ||
7,907.30 | 44,546.42 | ||
7,905.92 | 7,905.92 | ||
7,905.23 | 7,905.23 | ||
NAb | 21,148.52 | 3,437,296,211.85 | |
21,146.52 | 3,437,296,701.40 | ||
21,144.52 | 3,437,299,018.61 | ||
21,142.52 | 3,437,308,928.06 | ||
21,138.11 | 3,453,456,677.99 | ||
21,136.13 | 3,453,534,733.00 | ||
21,134.36 | 3,454,199,593.04 | ||
21,133.60 | 3,457,789,104.03 | ||
21,134.56 | 3,466,392,372.71 | ||
21,127.39 | 3,480,471,771.79 | ||
21,128.19 | 3,488,662,304.51 | ||
21,127.58 | 3,498,633,038.26 |
Loca, location/address; smk, whether smoke; resd, respiratory diseases history; cd, chronical diseases; vac, vaccination condition; phsms, pharmaceutical or non-pharmaceutical protection condition of individuals; PI, whether infected by SARS-CoV-2; symp, severity of symptoms; hosp, whether admitted in hospitals; SI, time interval between onset of symptoms and testing for antibody titer; his, family history of respiratory or pulmonary diseases. AIC, Akaike information criterion; BIC, Bayesian information criterion; MLR, multilinear regression; IgG, immunoglobulin G; IgM, immunoglobulin M; NAb, neutralizing antibody; SARS-CoV-2, severe acute respiratory syndrome coronavirus 2.
For longitudinal analysis for the change of antibodies, optimized GBTM of IgG is an all-one-ordered four-group (1,1,1,1 group) model (Figure 5), average posterior probabilities for each group are 0.9735, 0.9665, 0.9894, and 08864, respectively. The results of the optimal model fitting showed that among the four categories, the first category group contained 52 individuals (4.3%) with low initial IgG levels, followed by a gradual increase in IgG levels, which was defined as a “gradual growth type”, and the other three groups contained 1,139 individuals (95.8%) with different initial IgG levels, followed by a gradual decrease in IgG levels, which was defined as a “gradual decline type”. The study determined that the optimal IgM model is a full first-order group (1 group), and the average posterior probability of each group is 1.0000. The optimal model fitting results show that the initial IgM levels of each group are different, and then show a gradual decline, which is defined as a “gradual decline type”. We determined that the optimal NAb model was also a full first-order group (1,1 group), and the average posterior probability of each group was 0.9790, and 0.9763, respectively. The results of fitting the optimal model showed that the initial NAb levels of each group varied, and then showed a gradual decline, which was defined as a “gradual decline type”. Detailed calculation results of GBTM are provided in appendix available at https://cdn.amegroups.cn/static/public/jtd-23-1516-1.xlsx.
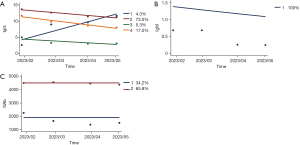
Simulation of the current COVID-19 epidemic by high-dimensional transmission dynamics model
As shown in Figure 6, in the absence of available data on the precise number of COVID-19 infections, we employed a high-dimensional transmission dynamics model that considers reinfection and the vaccination status of individuals to simulate the current epidemic in Xiamen City. Notably, after the initial peak observed in late December 2022 and January 2023, a second peak occurred in mid-May and early June in Xiamen City. Additionally, the model predicts a third peak in mid-October and early November. According to Figure 7, the analysis reveals variations in infection and incidence rates across different age groups. The age groups 30–39 and 40–49 years exhibit higher incidence and infection rates during both the second and third peaks, followed by age groups 50–59, 18–29, and 70–79 years. Conversely, the eldest age group (80+) and the youngest [0–2] tend to have lower infection and incidence rates. However, it is essential to note that, overall, the incidence and infection rates for all age groups follow a similar scale as observed in the mid-aged group.
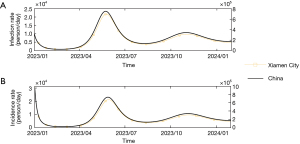
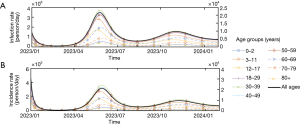
Discussion
The COVID-19 pandemic has sparked extensive research into prevention and symptom management (22). Notably, the quantity and longevity of antibodies produced during infection or vaccination are critical factors related to reinfection risk and symptom severity (23). The consensus holds that as antibody levels wane, individuals become more susceptible to reinfection, leading to discussions on the protective role of antibodies after natural infection or vaccination (24).
Crowd distribution for the samples
In this study, serum antibody titers of 1,191 public health workers in Xiamen City were tested over four consecutive monthly intervals. The study population exhibited no significant differences in antibody distribution due to a likely common immune response to both infection and vaccination (11). As the staff are all from the Xiamen Healthcare System, including doctors, CDCs workers, and primary healthcare organizations, it explains the insignificant difference in age groups and gender, but it also indicates that these indifferences could not be extrapolated to the general population.
However, for the differences in addresses, it is found that some staff live in cities near Xiamen and work in Xiamen CDC, therefore, there would be some differences in the regional distribution of the staff. However, due to the limitations of the sample, we cannot assume that there is a difference in infection among the population in different districts of the whole of Xiamen City. What’s more, before December 2022, it was recommended that people take one booster dose of the COVID-19 vaccine, yet after the new COVID-19 policy, there has been a new peak in people accepting vaccination (25). This questionnaire was sent out in February, therefore, there will be some differences in the vaccinated status between infected and non-infected groups.
Correlation of individual properties and antibody titer for COVID-19
Previously, there have been studies exploring the serological information for COVID-19 antibodies, which included immune process, seroconversion time, immune response as related to clinical presentation, and immune duration (3,5,6,10,26-29). Results of these studies have indicated that immune responses to SARS-CoV-2 are consistent with general viral infection patterns. However, some researchers suggest that immunity generated from natural infection appears to be short, which gives a hint that reinfection is inevitable (6,30-32). Should we find out individual properties that could make a difference in the antibody titer, it would be possible for us to select those who are at a high risk of short-term reinfection.
A MLR analysis was conducted to identify factors influencing IgG, IgM, and NAb titers. For IgM, only age demonstrated a significant impact, likely due to its role as the primary response and potential age-related variations in immune response (5); IgM plays a prominent role during the early age and only exists for 20–30 days. Since T-cell-derived antibody production decreases and B-lymphocyte generation decreases with age, antibody response against infectious agents and after vaccination may not be sufficient (17). In contrast, IgG, whose titers remained elevated and relatively stable for a longer period after induced, were significantly influenced by age, weight, height, history of pulmonary disease, and hospitalization. History of pulmonary diseases could be illustrated as soundness of the immune system, which indicates that those who have suffered from pulmonary diseases may have pulmonary immune system damage and result in lower antibody titers (18). Hospitalization could be explained as the severity of symptoms, which can be indicative of immune system health and symptom severity (19). The effect of smoking on antibody response has shown variable results with different viruses. For example, a very low antibody response was measured in smokers after the hepatitis B vaccine (20). NAb titers were impacted by sex, smoking, family history of pulmonary disease, and hospitalization. Smoking’s effect on NAb titers can be explained by NAb’s longer-lasting nature, indicating its significance in protection (8).
Ever since the pandemic, there have been a variety of studies that revealed the duration of different antibodies through typical textbook knowledge as well as experimental studies, cohort studies, etc. (5,21,29,33). Here in this study, we have collected and tested for serum antibodies of 1,191 healthcare workers four times and applied a GBTM model to explore potential development trajectories of antibodies. It was found in this study that only IgG presented a different trajectory, while IgM and NAb are the unchanged type. As IgM indicates the instant infections, remained unchanged since the timing for serological tests were all conducted in the middle of the month, when the infection was over. For NAb, it may be because they could last for more than 3 months, and due Labor Day holiday from May 1st to 5th, people started travelling and increased the possibility of contact, then there was a peak in the infection rate, which may be the reason why NAb titer was unchanged. However, it was found that there were 52 individuals had a low IgG titer at first then kept growing during the follow-up period, while other 1,139 individuals with different initial IgG titer kept decreasing. Then we made a further investigation on those 52 individuals and found out that there were no differences in their infection status at the first serum test or in their gender, age, and other properties. Therefore, we need a further exploration of the reason why some would show an increasing trend while others declined (34).
Simulation of the current COVID-19 epidemic by high-dimensional transmission dynamics model
Here we are under the circumstance that obtaining the current epidemic is unable to be acquired. We refined the previous SEIAR (Susceptible-Exposed-Infection-Asymptomate-Removed/Recovered) transmission dynamics model (35), we have introduced some real-world scenarios such as vaccination, pre-symptomatic infections, reinfection to the simple model and constructed VEAFIRPRV model. Then we simulated the future incidence rate and infection rate of COVID-19. We have simulated and predicted that there was a peak in the middle of May and early July here in Xiamen City, which may result from an increased contact rate among the population due to the Labor Day holiday and Dragonboat Festival. And it was consistent with the time when IgG titer decreased and NAb titer increased in the tested participants. Hence, we believe that the authorities should remind people to take precautions during holidays when there is a large movement of people, and the government should be prepared for the possible shortage of medical resources due to COVID-19 during holidays.
Then we predicted that starting from late September, there would be another peak in the mid-October to early November. This could be explained by the increase in contact due to school starting, National Holidays, and cold weather, leading to low ventilation rates in rooms. Therefore, there may be a further decrease in IgG and another increase in NAb. Another prediction and simulation of incidence rate and infection rate was for different age groups. The quality of the antibody response is influenced by age, as is the case for T-cell responses (17). Given the risk of severe COVID-19 in the elderly is higher, age-dependent immunological mechanisms are particularly important to elucidate, as these could be targeted to improve responses to natural infection and vaccination (29). During simulation, we also found that the highest infection and incidence rate lie in the age group of 30–39 years, where the old and the babies are the lowest, we believe this result was driven by the total population of Xiamen City and the number of people by age groups. The independent population (age 15–60 years) has more chance of contact with each other than the dependent population (age <15 and >60 years).
Limitation
The limitation of the study is that the participants did not reflect the general population, as the study was conducted in a small group of healthy volunteers and we applied convenience sampling. Therefore, we could not adequately examine the differences in antibody levels in the presence of various diseases that are more likely to be present in the population with the demographic data we examine. Another limitation of this study lies in the high-dimensional transmission dynamics model. In this model, the contact among the population is considered to be the major factor affecting the epidemic peak, mutation of the virus as well as the possible immune escape of variants are not taken into consideration, which indicates fact that the model is supposed to be refined to make a better prediction on the future epidemic trend.
Conclusions
While COVID-19 policies have evolved, the health challenges posed by the disease remain significant. New mutants continue to emerge, suggesting that reinfection is still a pressing problem. This study reveals key information about COVID-19 antibody dynamics in Xiamen healthcare workers. It highlights age-related impacts on IgM and diverse influences on IgG, and NAb titers, emphasizing the complexity of individual characteristics in antibody responses. However, the distinct trajectory in IgG levels among subsets warrants further investigation for potential factors driving these contrasting trends.
To build a more robust defense against reinfection and optimize self-protection strategies, we must delve deeper into understanding antibody dynamics and tailor more effective public health strategies as we navigate the ongoing challenges posed by COVID-19 epidemics.
Acknowledgments
Funding: This work was supported by
Footnote
Provenance and Peer Review: This article was commissioned by the Guest Editors (Jing Cheng, Tao Xu, Zifeng Yang, Wenda Guan) for the series “Current Status of Diagnosis and Forecast of COVID-19” published in Journal of Thoracic Disease. The article has undergone external peer review.
Reporting Checklist: The authors have completed the TRIPOD reporting checklist. Available at https://jtd.amegroups.com/article/view/10.21037/jtd-23-1516/rc
Data Sharing Statement: Available at https://jtd.amegroups.com/article/view/10.21037/jtd-23-1516/dss
Peer Review File: Available at https://jtd.amegroups.com/article/view/10.21037/jtd-23-1516/prf
Conflicts of Interest: All authors have completed the ICMJE uniform disclosure form (available at https://jtd.amegroups.com/article/view/10.21037/jtd-23-1516/coif). The series “Current Status of Diagnosis and Forecast of COVID-19” was commissioned by the editorial office without any funding or sponsorship. The authors have no other conflicts of interest to declare.
Ethical Statement: The authors are accountable for all aspects of the work in ensuring that questions related to the accuracy or integrity of any part of the work are appropriately investigated and resolved. The study was conducted in accordance with the Declaration of Helsinki (as revised in 2013). The study was approved by the ethics committee of the Xiamen Center for Disease Control [XJK/LLSC(2022)004] and participants signed the agreement consent before filling out the questionnaire.
Open Access Statement: This is an Open Access article distributed in accordance with the Creative Commons Attribution-NonCommercial-NoDerivs 4.0 International License (CC BY-NC-ND 4.0), which permits the non-commercial replication and distribution of the article with the strict proviso that no changes or edits are made and the original work is properly cited (including links to both the formal publication through the relevant DOI and the license). See: https://creativecommons.org/licenses/by-nc-nd/4.0/.
References
- World Health Organization. Strategy and planning. Available online: https://www.who.int/emergencies/diseases/novel-coronavirus-2019/strategies-and-plans
- Cohen JI, Burbelo PD. Reinfection With SARS-CoV-2: Implications for Vaccines. Clin Infect Dis 2021;73:e4223-8. [Crossref] [PubMed]
- Castro Dopico X, Ols S, Loré K, et al. Immunity to SARS-CoV-2 induced by infection or vaccination. J Intern Med 2022;291:32-50. [Crossref] [PubMed]
- Cruz AT, Zeichner SL. Duration of Effective Antibody Levels After COVID-19. Pediatrics 2021;148:e2021052589. [Crossref] [PubMed]
- Fearon DT, Locksley RM. The instructive role of innate immunity in the acquired immune response. Science 1996;272:50-3. [Crossref] [PubMed]
- Sneller MC, Liang CJ, Marques AR, et al. A Longitudinal Study of COVID-19 Sequelae and Immunity: Baseline Findings. Ann Intern Med 2022;175:969-79. [Crossref] [PubMed]
- Jiang JC, Zhang Y. Serological antibody testing in the COVID-19 pandemic: their molecular basis and applications. Biochem Soc Trans 2020;48:2851-63. [Crossref] [PubMed]
- Evans JP, Zeng C, Carlin C, et al. Neutralizing antibody responses elicited by SARS-CoV-2 mRNA vaccination wane over time and are boosted by breakthrough infection. Sci Transl Med 2022;14:eabn8057. [Crossref] [PubMed]
- De Giorgi V, West KA, Henning AN, et al. Naturally Acquired SARS-CoV-2 Immunity Persists for Up to 11 Months Following Infection. J Infect Dis 2021;224:1294-304. [Crossref] [PubMed]
- Padoan A, Dall'Olmo L, Rocca FD, et al. Antibody response to first and second dose of BNT162b2 in a cohort of characterized healthcare workers. Clin Chim Acta 2021;519:60-3. [Crossref] [PubMed]
- Nordström P, Ballin M, Nordström A. Risk of SARS-CoV-2 reinfection and COVID-19 hospitalisation in individuals with natural and hybrid immunity: a retrospective, total population cohort study in Sweden. Lancet Infect Dis 2022;22:781-90. [Crossref] [PubMed]
- Hachmann NP, Miller J, Collier AY, et al. Neutralization escape by SARS-CoV-2 Omicron subvariants BA. 2.12. 1, BA. 4, and BA. 5. N Engl J Med 2022;387:86-8. [Crossref] [PubMed]
- Brand SPC, Ojal J, Aziza R, et al. COVID-19 transmission dynamics underlying epidemic waves in Kenya. Science 2021;374:989-94. [Crossref] [PubMed]
- Cai J, Deng X, Yang J, et al. Modeling transmission of SARS-CoV-2 Omicron in China. Nat Med 2022;28:1468-75. [Crossref] [PubMed]
- Donnat C, Bunbury F, Kreindler J, et al. Predicting COVID-19 Transmission to Inform the Management of Mass Events: Model-Based Approach. JMIR Public Health Surveill 2021;7:e30648. [Crossref] [PubMed]
- Memoli V, Ekanmian G, Lunghi C, et al. What methods are used to study the association between medication adherence trajectories, estimated with the group-based trajectory modeling (GBTM) method, and health-related outcomes?-a protocol for a systematic review. Syst Rev 2022;11:102. [Crossref] [PubMed]
- Grifoni A, Weiskopf D, Ramirez SI, et al. Targets of T Cell Responses to SARS-CoV-2 Coronavirus in Humans with COVID-19 Disease and Unexposed Individuals. Cell 2020;181:1489-1501.e15. [Crossref] [PubMed]
- Swartz MD, DeSantis SM, Yaseen A, et al. Antibody Duration After Infection From SARS-CoV-2 in the Texas Coronavirus Antibody Response Survey. J Infect Dis 2023;227:193-201. [Crossref] [PubMed]
- Smits VAJ, Hernández-Carralero E, Paz-Cabrera MC, et al. The Nucleocapsid protein triggers the main humoral immune response in COVID-19 patients. Biochem Biophys Res Commun 2021;543:45-9. [Crossref] [PubMed]
- Huang YP, Gauthey L, Michel M, et al. The relationship between influenza vaccine-induced specific antibody responses and vaccine-induced nonspecific autoantibody responses in healthy older women. J Gerontol 1992;47:M50-5. [Crossref] [PubMed]
- Piccoli L, Ferrari P, Piumatti G, et al. Risk assessment and seroprevalence of SARS-CoV-2 infection in healthcare workers of COVID-19 and non-COVID-19 hospitals in Southern Switzerland. Lancet Reg Health Eur 2021;1:100013. [Crossref] [PubMed]
- Iwasaki A, Yang Y. The potential danger of suboptimal antibody responses in COVID-19. Nat Rev Immunol 2020;20:339-41. [Crossref] [PubMed]
- Newell KL, Clemmer DC, Cox JB, et al. Switched and unswitched memory B cells detected during SARS-CoV-2 convalescence correlate with limited symptom duration. PLoS One 2021;16:e0244855. [Crossref] [PubMed]
- Zhang Y, Zeng G, Pan H, et al. Safety, tolerability, and immunogenicity of an inactivated SARS-CoV-2 vaccine in healthy adults aged 18-59 years: a randomised, double-blind, placebo-controlled, phase 1/2 clinical trial. Lancet Infect Dis 2021;21:181-92. [Crossref] [PubMed]
- Nair S, Chen X. Biology of severe acute respiratory syndrome coronavirus 2 (SARS-CoV-2) and the humoral immunoresponse: a systematic review of evidence to support global policy-level actions and research. Glob Health J 2022;6:38-43. [Crossref] [PubMed]
- Baumgarth N, Nikolich-Žugich J, Lee FE, et al. Antibody Responses to SARS-CoV-2: Let's Stick to Known Knowns. J Immunol 2020;205:2342-50. [Crossref] [PubMed]
- Dan JM, Mateus J, Kato Y, et al. Immunological memory to SARS-CoV-2 assessed for up to 8 months after infection. Science 2021;371:eabf4063. [Crossref] [PubMed]
- Lee WS, Wheatley AK, Kent SJ, et al. Antibody-dependent enhancement and SARS-CoV-2 vaccines and therapies. Nat Microbiol 2020;5:1185-91. [Crossref] [PubMed]
- Xie J, Ding C, Li J, et al. Characteristics of patients with coronavirus disease (COVID-19) confirmed using an IgM-IgG antibody test. J Med Virol 2020;92:2004-10. [Crossref] [PubMed]
- Kusunoki H, Ohkusa M, Iida R, et al. Longitudinal Changes in IgG-Type SARS-CoV-2 Antibody Titers after COVID-19 Vaccination and a Prominent Increase in Antibody Titers When Infected after Vaccination. Vaccines (Basel) 2023;11:860. [Crossref] [PubMed]
- Chen J, Liu X, Zhang X, et al. Decline in neutralising antibody responses, but sustained T-cell immunity, in COVID-19 patients at 7 months post-infection. Clin Transl Immunology 2021;10:e1319. [Crossref] [PubMed]
- Iyer AS, Jones FK, Nodoushani A, et al. Persistence and decay of human antibody responses to the receptor binding domain of SARS-CoV-2 spike protein in COVID-19 patients. Sci Immunol 2020;5:eabe0367. [Crossref] [PubMed]
- Uysal EB, Gümüş S, Bektöre B, et al. Evaluation of antibody response after COVID-19 vaccination of healthcare workers. J Med Virol 2022;94:1060-6. [Crossref] [PubMed]
- Altawalah H. Antibody Responses to Natural SARS-CoV-2 Infection or after COVID-19 Vaccination. Vaccines (Basel) 2021;9:910. [Crossref] [PubMed]
- Niu Y, Rui J, Wang Q, et al. Containing the Transmission of COVID-19: A Modeling Study in 160 Countries. Front Med (Lausanne) 2021;8:701836. [Crossref] [PubMed]