Automatic detection of obstructive sleep apnea based on speech or snoring sounds: a narrative review
Introduction
Obstructive sleep apnea (OSA) is a common chronic disorder, with estimates indicating a prevalence ranging from 22% in men and 17% in women in the general population (1) to as high as 70% in specific groups, such as patients undergoing bariatric surgery (2). OSA is characterized by repetitive instances of the upper airway collapsing during sleep, specifically at the pharyngeal level (3). The main complaint of patients with OSA is excessive daytime sleepiness, which can lead to deterioration in quality of life, impaired work performance, and accidents (4). Moreover, OSA is increasingly linked to cognitive deficits (5,6), risk of dementia (7,8), and other major neurologic and psychiatric disorders (9-12). OSA is a risk factor for both chronic conditions, such as cardiovascular diseases and hypertension, as well as acute conditions, such as stroke, myocardial infarction, congestive heart failure, and in extreme cases, sudden death (13-15).
Polysomnography (PSG) is the accepted gold standard for diagnosing OSA (16). Following the administration of this test, the apnea hypopnea index (AHI) is calculated by determining the average number of apnea and hypopnea occurrences (complete and partial cessation of breathing, respectively) per hour of sleep. A diagnosis of OSA is typically made when the patient has an AHI ≥5 events/hour accompanied with symptoms of excessive daytime sleepiness and/or cardiovascular morbidity (17). However, this diagnostic procedure requires an overnight stay of the patient at the sleep unit within a hospital, where their breathing patterns, heart rhythm, and limb movements can be monitored. Although this approach is available as ambulatory, it can be costly, time-consuming, and potentially uncomfortable for the patient. For these reasons, a large number of OSA cases remain undiagnosed (18). Consequently, there is a strong need to explore faster and less costly alternatives for the recognition of OSA.
The human speech signal can be easily and quickly obtained and contains abundant information on individual characteristics. Previous studies have identified characteristic differences of speech in patients with OSA that differ from those of healthy individuals (19-22). In addition, snoring is the most direct and earliest symptom of OSA, providing valuable insights into the patient’s condition, including the severity of the disorder and the site of obstruction within the upper airway (23). Therefore, speech and snoring sounds are expected to be good candidates for the evaluation of OSA. In recent years, with the rapid development of machine learning in audio analysis, investigators have attempted to use the abnormal changes of speech information or snoring sounds to develop an approach for the automatic detection of OSA. However, the prediction value of speech and snoring sounds in patients with OSA remains unclear.
In this review, we offer a comprehensive summary of the current research progress on the automatic detection of OSA using snoring sounds or speech signals. In addition, we discuss the key challenges that need to be overcome in future research of this novel approach. We present this article in accordance with the Narrative Review reporting checklist (available at https://jtd.amegroups.com/article/view/10.21037/jtd-24-310/rc).
Methods
A literature search was performed using the PubMed, IEEE Xplore, and Web of Science databases for literature published between 1989 and 2022. The search strategy is presented in Table 1. The following keyword search strategy was used: (“obstructive sleep apnea syndrome” OR “OSAS” OR “OSA”) AND (“speech” OR “voice” OR “snore sound”) AND (“screen” OR “prediction”) AND “deep learning” OR “machine learning” OR “artificial intelligence”). All English-language papers including original articles, reviews, and editorials related to studies in humans were included. Each article was screened for relevance through a reading of the titles and abstracts. Relevant information was extracted independently by two reviewers (S.C., M.X.).
Table 1
Items | Specification |
---|---|
Date of search | Nov 1, 2022 to Dec 1, 2022 |
Databases and other sources searched | PubMed, IEEE Xplore, and Web of Science |
Search terms used | Obstructive sleep apnea syndrome, OSAS, OSA, speech, voice, snore sound, screen, prediction, deep learning, machine learning, artificial intelligence |
Timeframe | 1989–2022 |
Inclusion criteria | English-language papers including original articles, reviews, and editorials related to studies in humans |
Selection process | Each article was screened for relevance by reading the titles and abstracts. Relevant information was independently extracted by two reviewers (S.C., M.X.) |
Speech and OSA
The generation of speech
The human speech is a complex signal of sound produced by vocal fold vibration. It is not only a primary source for communication between individuals, but also contains various characteristic information of the human body, including biological information (e.g., age, body size) and paralinguistic information (e.g., emotional state) (24).
Speech formation is an exceedingly complex physiological process. Generally speaking, under the control of the nervous system, air exhaled from the lungs vibrates through the vocal organs and resonates through the cavity organs, such as the pharynx, from which speech is generated.
The abnormal speech of patients with OSA
Previous study has indicated that individuals with OSA exhibit abnormalities in their speech compared to those unaffected by this condition (19). These abnormalities can be attributed to alterations in the upper airway anatomy, such as craniofacial abnormalities, dental occlusion, increased distance between the hyoid bone and the mandibular plane, relaxed soft tissues, enlarged tongue base, and other related factors, and the impact of persistent snoring during sleep.
The cues for the abnormal speech of OSA were firstly reported by Fox et al. (25). In this study, they found that abnormal resonance, articulation, or phonation was present in 74% of participants with OSA. In a study by Fiz et al. (26), in which the vocalizations in 18 men with OSA and 10 normal men were analyzed, significant differences were observed in the maximum frequency of harmonics of the /i/ and /e/ vowels between the two groups. Later, Robb et al. (27) reported that OSA speakers exhibited lower formant values and wider formant bandwidths compared to the non-OSA speakers, and they attributed this alteration to the longer vocal tract length and excessive vocal tract tissue compliance in patients with OSA. A recent study (28) explored the relationship between voice quality and OSA severity; according to its voice analysis results, there were significant differences between OSA and non-OSA individuals in the fundamental frequency, jitter percentage, shimmer percentage, harmonic-noise ratio (HNR), and maximum phonation times as the severity of OSA increased.
Another study employed spectrographic analysis to determine the correlations among AHI and acoustic features, indicating that AHI correlates poorly with formant frequencies and bandwidths extracted from sustained vowels (20). It was thus proposed that the effects of clinical variables should be considered in any research on speech and OSA.
Undoubtedly, speech carries a large amount of characteristic information concerning the anatomical structures of the upper airway (29-31). Consequently, it may serve as a valuable tool for diagnosing OSA or, at the very least, for identifying individuals who are susceptible to the condition.
Automatic detection of OSA based on speech
According to the above-described contributions to this subject, studies have been carried out on the automatic assessment of OSA based on abnormal speech signals.
Table 2 provides summary of the studies that have employed speech data and artificial intelligence algorithms to facilitate the automatic detection of OSA.
Table 2
First author/year | Population characteristics | Speech materials | Audio features | Classification model | Classification performance |
---|---|---|---|---|---|
Fernández Pozo/2009 (32) | 80 male participants (AHI <10: 40 males, mean age 42.2 years, mean BMI 26.2 kg/m2; AHI >30: 40 males, mean age 49.5 years, mean BMI 32.8 kg/m2) | Four Spanish sentences: (I) Francia, Suiza y Hungr´ıa ya hicieron causa común. (II) Julián no vio la manga roja que ellos buscan, enning ún almacén. (III) Juan no puso la taza rota que tanto le gusta en el aljibe. (IV) Miguel y Manu llamará n entre ocho y nueve y media | The third and the second formant; HNR; dysperiodicity | GMM | Accuracy: 81%; sensitivity: 77.5%; specificity: 85% |
Goldshtein/2011 (33) | 93 participants (AHI ≤5: 14 females, mean age 47.2 years, mean BMI 28.4 kg/m2; AHI >5: 19 females, mean age 58.7 years, mean BMI 33.4 kg/m2; AHI ≤5: 12 males, mean age 45.6 years, mean BMI 27.7 kg/m2; AHI >5: 48 males, mean age 56.6 years, mean BMI 31.2 kg/m2) | Five vowels, /a/, /e/, /i/, /o/ and /u/ and the two nasal phonemes, /n/ and /m/ | 100 features; extracted from each frame, including spectral features such as formants, bandwidth and HNR; LPC; cepstral features; MFCC and its first and second derivatives; a physiologic feature: vocal tract length; prosodic features, such as pitch, jitter, shimmer, and energy | GMM | Males: specificity, 83%; sensitivity, 79%; females: specificity, 86; sensitivity, 84% |
Benavides/2012 (34) | 80 participants (AHI <10: 40 males; AHI >30: 40 males; age and BMI were not reported) | Four Spanish sentences | Not mentioned | HMMs | Accuracy: 85% |
Solé-Casals/2014 (35) |
127 controls (AHI ≤5: 48 males and 79 females, mean age 29.68 years, mean BMI 29.68 kg/m2); 121 patients with severe OSA (AHI ≥30: 101 males and 20 females, mean age 54.04 years, mean BMI, 32.56 kg/m2) | Five vowels: /a/, /e/, /i/, /o/, and /u/; one Spanish sentence: De golpe nos quedamos a oscuras |
A total of 253 features extracted which may be grouped as follows: formant and pitch-based features; time domain analysis; voice harshness and turbulence analysis; linear prediction analysis; dynamical systems analysis; long-term average spectrum-based features | BC, KNN, SVM, neural networks, Adaboost | The best strategy in terms of accuracy (average 82.04%), sensitivity (average 81.74%), and specificity (average 82.40%) was the BC with featured selected via genetic algorithms |
Espinoza-Cuadros/2016 (36) | 426 participants (AHI <10: 125 males; AHI ≥10: 301 males; mean age 48.8 years, mean BMI 29.8 kg/m2) | Five vowels /a/, /e/, /i/, /o/, and /u/; four Spanish sentences | Supervectors, ivectors | SVR | For supervectors: accuracy, 68%; specificity, 18%; sensitivity: 89%; AUC, 0.58. For i-vectors: accuracy, 71%; specificity, 20%; sensitivity, 92%; AUC, 0.64 |
Ding/2021 (37) | 151 participants (AHI ≤30: 76 males, mean age 39.7 years, mean BMI 26.1 kg/m2; AHI >30: 75 males, mean age 39.2 years, mean BMI 28.8 kg/m2) | Chinese vowels /a/, /o/, /e/, /i/, /u/, and /ü/; and nasals /en/ and /eng/ | LPCC | SVM | With the thresholds of AHI =30 and AHI =10, the accuracies were both 78.8%, the sensitivities were 77.3% and 79.1%, and the specificities were 80.3% and 78.0%, respectively |
Ding/2022 (38) | 158 participants (AHI <10: 41 males, mean age 39.1 years, mean BMI 26.1 kg/m2; AHI ≥10: 117 males, mean age 39.3 years, mean BMI 28.1 kg/m2; AHI <30: 78 males, mean age 39.2 years, mean BMI 26.3 kg/m2; AHI ≥30: 80 males, mean age 39.3 years, mean BMI 28.9 kg/m2) | All Chinese syllables | LPC | Decision tree | With the thresholds of AHI =10 and AHI =30, the AUCs were 0.83 and 0.87, the sensitivities were 81.8% and 78.1%, and the specificities were 81.3% and 82.6%, respectively |
OSA, obstructive sleep apnea; AHI, apnea hypopnea index; BMI, body mass index; GMM, Gaussian mixture model; HNR, harmonic-noise ratio; LPC, linear predictive coding; MFCC, mel-frequency cepstral coefficients; HMM, hidden Markov models; BC, Bayesian classifiers; KNN, k-nearest neighbour; SVM, support vector machines; SVR, support vector regression; AUC, area under the curve.
In 2009, Fernández Pozo et al. (32) presented pioneering research on the automatic diagnosis of severe OSA using a Gaussian mixture model (GMM) with continuous speech and achieved an 81% correct classification rate for male participants. A speech corpus containing four sentences in Spanish based on physiological OSA features was designed, which was then used in subsequent studies. In a study conducted by Goldshtein et al. (33) in 2011, a GMM-based system was developed to analyze speech recordings of 93 participants. The recordings included five vowels (/a/, /e/, /i/, /o/, /u/) and two nasal phonemes (/n/, /m/). The system achieved a specificity of 83% and a sensitivity of 79% for male patients with OSA, while for female patients, it achieved a specificity of 86% and a sensitivity of 84%. In another study by Benavides et al. (34), speech samples from control (AHI <10) and OSA (AHI >30) individuals and text-dependent hidden Markov models (HMMs) were employed to train a binary machine learning classifier for severe OSA detection, achieving an 85% correct classification rate among 80 male participants.
Due to the impact of different body positions on the vocal tract in patients with OSA, individuals exhibit different speech features. Solé-Casals et al. (35) analyzed both recordings of five vowels and a sentence from two distinct positions, upright or seated and supine or stretched. They presented experimental findings regarding various feature selection and reduction schemes [statistical ranking, genetic algorithms, principal component analysis (PCA), linear discriminant analysis (LDA)] and compared various classifiers [Bayesian classifiers, k-nearest neighbor (KNN), support vector machines (SVM), neural networks, adaptive boosting (Adaboost)]. The classifier performed optimally when a well-suited genetic algorithm was used for feature selection, leading to the best results. In contrast to the promising outcomes documented in prior studies, Espinoza-Cuadros et al. (36) reported contradictory results when implementing the proposed methods on a sizable clinical database. In their study, the speaker recognition technology, including supervectors and i-vectors, was used for the first time to estimate AHI, yielding an accuracy of 68% and 71%, respectively. In this case, the variation in results can primarily be attributed to the sample size and participant composition. Unlike previous studies that used a limited sample size, the one by Espinoza-Cuadros et al. (36) included a database comprising 125 controls (AHI <10) and 118 patients with OSA (AHI >30). Another significant aspect contributing to the decline in performance is that the controls in previous studies were asymptomatic individuals. Meanwhile, in Espinoza-Cuadros et al.’s study (36), all participants were referred to a sleep disorders unit, indicating that they were suspected to have OSA; that is, most of them were heavy snorers. Furthermore, the imbalance in confounding factors (age, height, weight, sex) between the control group and the OSA group in earlier research might have produced overoptimistic results.
Considering the language-dependent differences in speech, Ding et al. (37) conducted the first study with Chinese participants. They classified the participants with thresholds of AHI =30 and AHI =10 events/hour by using linear prediction cepstral coefficients (LPCC) and SVM, which both obtained an accuracy of 78%. Recently, the researchers expanded on their investigation of the classification performance of Chinese syllables for OSA by using LPC and the decision tree model: specific Chinese syllables, such as [leng] and [jue], consonant pronunciations such as [zh] and [f], and vowel pronunciations such as [ing] and [ai], were found to be particularly effective for OSA classification (38). This study highlights the efficacy of using Chinese pronunciation as a reliable feature for predicting OSA and provides a comprehensive reference for the selection of an OSA corpus.
In general, speech appears to be a useful and an easily accessible predictor for OSA, which can optimize traditional OSA screening and diagnosis. Nevertheless, it should be noted that the studies by Ding et al. (37,38) mainly focused on male patients, and thus it would be interesting to further clarify whether this method is suitable for testing the female population. Further research using different acoustic features, approaches, languages, or recording positions are expected to bring new insights into this field.
Snoring sounds and OSA
The generation of snoring sounds
Similar to speech, snoring is also generated in the vocal tract (39). However, there are also several dissimilarities between snoring and speech. The vibration and resulting sound of snoring are primarily attributable to the oscillations of the soft palate, pharyngeal walls, epiglottis, and tongue rather than to the vocal cords (40). Snoring occurs in sleep, during which the muscle tone decreases and the soft tissue collapses, resulting in the narrowing and increased resistance of the pharyngeal airway (41). This triggers tissue vibrations and turbulent flows as the air passes through, which in turn causes a breathing noise (23). Moreover, the driving pressure is directed interiorly, as snoring is predominantly linked to the act of inspiration.
The acoustic difference of snoring sound between simple snorers and patients with OSA
Snoring serves as a significant clinical indicator of OSA. In cases where there are no apneas or hypopneas events during sleep and where the individual does not experience daytime symptoms, the respiratory noise is classified as simple snoring (42). However, if the snoring is accompanied by an AHI of ≥5 per hour and excessive daytime sleepiness, it is identified as OSA (3). The first study regarding the acoustic differences between these snoring phenotypes was conducted by Perez-Padilla et al. (43), who conducted the initial research on acoustic distinctions. Their study involved the analysis of snoring sounds emitted by 10 individuals identified as non-apneic heavy snorers and 9 patients diagnosed with OSA. The results showed that the initial snore following an apnea episode predominantly consists of broad-spectrum white noise, with a relatively higher power at higher frequencies. Perez-Padilla et al. (43) thus proposed utilizing the power ratio, specifically the ratio of power above 800 Hz to power below 800 Hz (PR800), as a means to differentiating individuals with OSA from those exhibiting simple snoring.
Fiz et al. (44) studied 10 patients with OSA and 7 simple snorers. The spectral analysis conducted on the snoring sounds revealed a significant decrease in the peak frequency of snoring among patients diagnosed with OSA. In addition, a significant negative correlation was found between AHI and peak and mean frequencies of the snoring power spectrum. Sola-Soler et al. (45) reported that the formant frequency of OSA snorers was significantly different from of simple snorers. Ng et al. (46) conducted a study employing the Linear Predictive Coding (LPC) technique, which revealed that snores during apnea events exhibited higher formant frequencies compared to non-apneic snores, particularly the first formant frequency. In another experiment, fast Fourier transform (FFT) analysis was applied and indicated that individuals with primary snoring exhibited a peak intensity range of 100–300 Hz, whereas those with OSA displayed a peak intensity above 1,000 Hz (47).
In addition to research focusing on the spectral parameters of snoring sounds, various other sound analysis techniques have also demonstrated discernible distinctions between individuals with apnea-related snoring and those with simple snoring (48,49). However, there is also a contrary opinion, in Alshaer’s (50) study, the snore index (calculated as the number of snores per hour of sleep) was found to have a weak relationship with AHI, indicating that the presence and frequency of snoring on its own is probably of limited usefulness in screening for the possibility of either OSA .
Overall, these findings suggest that alterations in the morphology of the upper airways manifest in the acoustic characteristics of snore signals, which can be used to reflect the existence and severity of OSA.
Automatic detection of OSA based on snoring sounds
Based on the above-mentioned studies, it is reasonable to believe that snoring sounds contain a rich information regarding OSA status.
Table 3 presents an overview of the studies that have utilized snoring sounds in conjunction with machine learning techniques for the purpose of automating OSA detection.
Table 3
First author/year | Population characteristics | Audio features | Classification model | Classification performance |
---|---|---|---|---|
Solà-Soler/2007 (51) | 25 participants (AHI <10: 12 males and 5 females, mean age 46 years, mean BMI 27.1 kg/m2; AHI ≥10: 13 males and 6 females, mean age 51 years, mean BMI 32.3 kg/m2) | The time parameters characterized by the period of the sound vibrations or pitch; The frequency parameters calculated via power spectral density | LR | The AUCs were between 0.913 and 0.950, the sensitivities were between 82.3% and 94.1%, and the specificities were between 73.7% and 89.5% |
Karunajeewa/2011 (52) | 41 participants (AHI <10: 5 males and 8 females, mean age 45 years, mean BMI 33.5 kg/m2; AHI ≥10: 23 males and 5 females, mean age 54 years, mean BMI 34.5 kg/m2) | Pitch and total airway response | LR | AUC: 0.96; sensitivity: 89.3%; specificity: 92.3% |
Cavusoglu/2007 (53) | 30 participants (AHI <10: 16 males and 2 females, mean age 46.92 years, mean BMI 27.66 kg/m2; AHI ≥10: 12 males, mean age 53.26 years, mean BMI 32.76 kg/m2) | Subband energy distributions | LR | Accuracy: 90.2% |
Mesquita/2012 (54) | 34 participants (AHI <30: 7 females and 9 males, mean age 50 years, mean BMI 26.32 kg/m2; AHI ≥30: 1 female and 17 males, mean age 52 years, mean BMI 30.43 kg/m2) | The time interval between regular snores in short segments | BC | With the thresholds of AHI =5 and AHI =30, the accuracies were 88.2% and 94.1%, respectively |
Jiang/2021 (55) | 12 participants (AHI <5: 4 males; AHI >5: 2 females and 6 males). Age and BMI were not reported | 10 acoustic features, including MFCC, 800 Hz power ratio, spectral entropy, and LPC | LR, SVM, Gaussian Bayesian, KNN, and ANN | For the top 6 features, the overall accuracies were between 91.67% and 100% |
Kim/2018 (56) | 120 participants were classified into four groups, with 30 patients in each group: AHI <5, mean age 44.1 years, mean BMI 23.0 kg/m2; 5≤ AHI <15, mean age 54.8 years, mean BMI 24.4 kg/m2; 15≤ AHI <30, mean age 53.9 years, mean BMI 26.9 kg/m2; AHI ≥30, mean age 50.3 years, mean BMI, 27.3 kg/m2 | 132 audio features, including MFCC, spectral flux, and zero crossing rate | Simple logistics, SVM, and DNN | Simple logistics showed the best performance: the accuracy was 92.5% under the AHI thresholds of 15 and 30 |
Shen/2020 (57) | 32 participants (16 healthy individuals and 16 patients with OSA). Age and BMI were not reported | MFCC, LPCC, and LPMFCC | CNN and LSTM | The MFCC feature extraction method combined with the LSTM model had the highest accuracy rate of 87% |
Jiang/2020 (58) | 15 participants (AHI <5: 1 female and 3 males, mean age 37.5 years, mean BMI 25.6 kg/m2; AHI ≥5: 5 females, 6 males, mean age 45.3 years, mean BMI, 27.4 kg/m2) | The time-domain waveform, spectrum, spectrogram, mel spectrogram, and constant Q transform spectrogram | CNNs-DNNs, CNNs-LSTMs-DNNs, and DNNs | The mel-spectrogram can better reflect the differences between OSA patients and simple snorers, with an AUC of 0.977 and 0.978, respectively |
OSA, obstructive sleep apnea; AHI, apnea hypopnea index; BMI, body mass index; LR, logistic regression; AUC, area under the curve; BC, Bayesian classifiers; MFCC, mel-frequency cepstral coefficients; LPC, linear predictive coefficients; SVM, support vector machines; KNN, k-nearest neighbour; ANN, artificial neural network; DNN, deep neural network; LPCC, linear prediction cepstral coefficients; LPMFCC, linear prediction MFCC; CNN, convolutional neural network; LSTM, long short-term memory; CNNs, convolutional neural networks; LSTMs, long short-term memory networks.
Solà-Soler et al. (51) proposed a logistic regression model that utilizes various time and frequency parameters extracted from snoring signals, along with their variability, to classify patients with OSA and simple snorers. With the thresholds of AHI =10 events/hour, the classification of patients yielded a sensitivity exceeding 93% and a specificity ranging from 73% to 88%. Similarly, in a study conducted by Karunajeewa et al. (52), pitch and total airway response (TAR) extracted from snore sounds were exploited in the diagnosis of OSA with a logistic regression model, yielding a sensitivity of 89.3% and a specificity of 92.3%. Cavusoglu et al. (53) conducted an analysis of snoring sounds obtained from 18 individuals with simple snoring and 12 patients diagnosed with OSA. PCA was implemented to transform the feature vectors from snoring episodes in a two-dimensional space, and robust linear regression was applied to classify snores and non-snores. An accuracy of 90.2% was obtained when the dataset included both simple and OSA snores. Mesquita et al. (54) found that there was shorter time interval between regular snores in patients with severe OSA. Thus, they imported features derived from the time interval between snores to a Bayesian classifier for the identification of patients with OSA, achieving classification accuracies of 88.2% and 94.1% for AHI severity cutoff severities of 5 and 30 hour−1, respectively.
To mitigate potential issues arising from high-dimensional features that could induce overfitting during model training, Jiang et al. (55) employed a feature selection algorithm that leveraged random forest. This algorithm was used to identify the top 6 significant features from a total of 10 traditional acoustic features that were extracted. Subsequently, five distinct machine learning models were employed to assess the effectiveness of the selected feature subset. The findings highlighted that the logistic regression model, in conjunction with the top six features, delivered enhanced accuracy for evaluating OSA while also exhibiting lower computational complexity.
To enhance the classification performance in OSA detection, researchers have incorporated deep learning techniques. Kim et al. (56) conducted a study in which 132 dimensional features derived from various feature parameters were selected. These features were used to classify the severity of OSA via multiple machine learning techniques, including logistic regression, SVM, and deep neural networks (DNNs). The classifier that demonstrated the highest performance in terms of accuracy was simple logistic regression, achieving an accuracy rate of 92.5% when evaluated against the AHI thresholds of 15 and 30. As discussed by Kim et al. (56), the relative lower classification effect of deep learning may be due to the number of samples being insufficiently high, as only 120 participants were examined. This sample size has been deemed somewhat inadequate for the optimal learning and training of DNNs. Shen et al. (57) compared the classification differences for OSA between three-feature extraction methods [mel-frequency cepstral coefficients (MFCCs), LPCC, and linear prediction MFCC (LPMFCC)] and three neural networks [convolutional neural network (CNN), recurrent neural network (RNN), long short-term memory (LSTM)]. The experimental results showed that the optimal performance for OSA detection was achieved by using a combination of MFCC and LSTM, which yielded a classification accuracy of 0.87.
Spectral maps encompass a wealth of valuable data, including elements such as formant frequencies, spectral energy, fundamental frequency, and other relevant features. Jiang et al. (58) assessed the performance of different snoring sound maps (the time-domain waveform, spectrum, spectrogram, mel-spectrogram, and CQT-spectrogram) in distinguishing patients with OSA patients and simple snorers. CNN, LSTM, and DNNs were used to construct two types of classifiers [CNNs-DNNs and CNNs-long short-term memory networks (LSTMs)-DNNs]. Meanwhile, fewer network layers were used to prevent overfitting caused by the small sample size of the study. The findings indicated that the mel-spectrogram exhibited superior discriminatory power in distinguishing between patients with OSA and simple snorers, with an AUC of 0.977 and 0.978, respectively.
In summary, the snoring-based technique seems to be a promising tool for the detection of OSA. Snore sounds have the significant advantage of being conveniently acquired with low-cost, noncontact equipment (59,60). In addition, this method allows participants suspected of having OSA to circumvent the need for a comprehensive overnight polysomnographic or a respiratory study conducted in a hospital setting. Furthermore, this approach opens the possibility of using common devices equipped with microphones, such as smartphones, as a screening tool for identifying OSA outside of specialized facilities. However, it is important to acknowledge that snoring sounds exhibit considerable variation among individuals, and further studies with large target populations are needed to verify the proposed method before this approach can be introduced in everyday clinical practice.
Summary of the general process of OSA detection by speech/snoring sounds
Below is an overview of the typical process of utilizing speech or snoring sounds for OSA detection (Figure 1). Initially, snoring sounds are typically captured as part of PSG using a PSG-embedded microphone. Speech recordings can be categorized into two main types: short sentences and vowels/syllables. Pre-processing of the audio involves steps such as resampling, normalization, noise reduction, framing, and windowing the data. Various methods have been proposed in the literature for audio feature extraction, encompassing temporal, frequency, and cepstral domains (Table 4). Dimensionality reduction techniques like PCA and LDA may be employed to transform features and facilitate data visualization. Subsequently, after feature selection, machine learning or deep learning algorithms such as SVM, HMMs, and CNNs can be trained to automatically predict OSA. To ensure reliable performance estimates, cross-validation and out-of-bootstrap validation techniques are commonly utilized. Evaluation of models typically involves metrics such as accuracy, specificity, sensitivity, F-measure, and AUC.
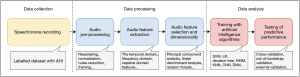
Table 4
Audio features | Definition |
---|---|
Time domain features (61) | Time domain features shows the signal variation with respect to time |
ZCR (61) | The ZCR of an audio frame is defined as the rate of change of sign of the signal during the frame. ZCR is the number of times signal crosses the zero level in one second interval |
STE (61) | STE is defined as average energy per frame. The STE is low for unvoiced segments and high for voiced segments |
Shimmer (62) | Difference of the peak amplitudes of consecutive fundamental frequency periods, which indicates irregularities in voice intensity |
Frequency domain features (61) | To analyze a signal in terms of frequency, the time-domain signal is converted into frequency domain signal by using Fourier transform or auto-regression analysis. Frequency domain analysis is a tool of utmost importance in audio signal processing |
Formant (61) | The spectral peaks (usually the first three) of the sound spectrum |
Fundamental frequency (61) | The fundamental frequency is the lowest frequency of a periodic waveform |
Bandwidth (61) | Spectral bandwidth is the second order statistical value the determines the low bandwidth sounds from the high frequency sounds |
HNR (62) | Ratio between fundamental frequency and noise components, which indirectly correlates with perceived aspiration. This may be due to reducing laryngeal muscle tension resulting in a more open, turbulent glottis |
Jitter (62) | Deviations in individual consecutive fundamental frequency period lengths, which indicates irregular closure and asymmetric vocal-fold vibrations |
Spectral slope (61) | It is the measure of slope of the amplitude of the signal and it is computed by linear regression. This feature is used in speech analysis and in identifying speaker from a speech signal |
PR800 (61) | The ratio of spectral energy above 800 Hz to that below 800 Hz |
Cepstral domain features (61) | A cepstrum is obtained by taking the inverse Fourier transform of the logarithm of the spectrum of the signal |
LPC (61) | Coefficients that best predict the values of the next time point of the audio signal using the values from the previous n time points, which is used to reconstruct filter properties |
LPCC (61) | LPC are too sensitive to numerical precision, hence it is desirable to transform the LPC to the cepstral domain. The resultant transformed coefficients are called as LPCCs |
MFCC (62) | The coefficients derived by computing a spectrum of the log-magnitude Mel-spectrum of the audio segment. The lower coefficients represent the vocal tract filter and the higher coefficients represent periodic vocal fold sources. MFCC is widely and successfully used in speech recognition |
OSA, obstructive sleep apnea; ZCR, zero crossing rate; STE, short time energy; HNR, harmonic-noise ratio; LPC, linear predictive coding; LPCC, linear prediction cepstral coefficients; MFCC, mel-frequency cepstral coefficients.
Key challenges in future research
Audio characteristics can effectively reflect the majority of relevant characteristics present in the original audio signals, eliminating the need for using the entire signal. Therefore, feature extraction is a crucial step in the automatic OSA assessment process regardless of whether speech or snoring sounds are used. However, the extracted features used in this type of research vary from study to study, which makes interstudy comparison difficult. In addition, it should be noted that not all of the extracted features have been proven beneficial for detecting OSA in every participant, as some of them exhibited no significant differences across different patient groups. Consequently, it is essential to validate the utility of each feature in classifying OSA. The results of the classification task demonstrated that incorporating only the selected features offered notable advantages in terms of both performance and computational efficiency compared to utilizing statistical values of all audio features. Therefore, feature selection and dimension reduction may be a promising direction of future research in this field.
In this review, we mainly focused on speech- or snoring-based methods. We believe that in the future, other noninvasive features, including demographic characteristics (63), symptoms (64), and facial images (65), can be combined for better identification of OSA. However, it is important to note that more does not always mean better in the concatenation of features. In addition, any proposed models should be as simple as possible so as to facilitate their wider application.
It is worth noting that the criteria for OSA was based on both AHI and associated symptoms. Although easy to calculate, AHI can only obtain limited information from the rich PSG data (66). Therefore, the AHI may not fully reflect the severity of OSA, and there are OSA patients with low AHI but with as many OSA symptoms as in patients with high AHI (67,68). Thus, the different phenotypes of OSA should be taken into account in these new ways of diagnosing OSA.
Besides, it is crucial to recognize the common limitations that might have contributed to overly optimistic outcomes when employing machine learning techniques for diagnostic purposes. A limited size of training and validation dataset can lead to compromised performance of predictive models. External validation is still rare in the literature. When feature selection techniques are applied to a large number of variables with only limited training data available, there is a risk of overfitting. Therefore, it is crucial for future research to involve a significantly larger sample size of participants to validate the methodology before clinical implementation.
Last but not least, there is no standard protocol for voice analysis to identify OSA. How to select appropriate corpora, which audio features are more valuable, and which AI algorithms are more effective are all worthy of further in-depth research in the future.
Conclusions
Research on snoring or speech for OSA diagnosis has emerged as a recent research trend. We believe that this review can help to clarify the predictive value of snoring sounds and speech for OSA status and guide further research on the detection of OSA based on snoring sounds or speech. Although this innovative approach currently does not have the potential to completely replace the conventional diagnosis procedure of OSA involving PSG analysis and comprehensive clinical evaluation, it does offer significant improvements in OSA management and can contribute to conserving medical resources.
Acknowledgments
Funding: This study was supported by
Footnote
Reporting Checklist: The authors have completed the Narrative Review reporting checklist. Available at https://jtd.amegroups.com/article/view/10.21037/jtd-24-310/rc
Peer Review File: Available at https://jtd.amegroups.com/article/view/10.21037/jtd-24-310/prf
Conflicts of Interest: All authors have completed the ICMJE uniform disclosure form (available at https://jtd.amegroups.com/article/view/10.21037/jtd-24-310/coif). The authors have no conflicts of interest to declare.
Ethical Statement: The authors are accountable for all aspects of the work in ensuring that questions related to the accuracy or integrity of any part of the work are appropriately investigated and resolved.
Open Access Statement: This is an Open Access article distributed in accordance with the Creative Commons Attribution-NonCommercial-NoDerivs 4.0 International License (CC BY-NC-ND 4.0), which permits the non-commercial replication and distribution of the article with the strict proviso that no changes or edits are made and the original work is properly cited (including links to both the formal publication through the relevant DOI and the license). See: https://creativecommons.org/licenses/by-nc-nd/4.0/.
References
- Franklin KA, Lindberg E. Obstructive sleep apnea is a common disorder in the population-a review on the epidemiology of sleep apnea. J Thorac Dis 2015;7:1311-22. [Crossref] [PubMed]
- Frey WC, Pilcher J. Obstructive sleep-related breathing disorders in patients evaluated for bariatric surgery. Obes Surg 2003;13:676-83. [Crossref] [PubMed]
- Veasey SC, Rosen IM. Obstructive Sleep Apnea in Adults. N Engl J Med 2019;380:1442-9. [Crossref] [PubMed]
- Arita A, Sasanabe R, Hasegawa R, et al. Risk factors for automobile accidents caused by falling asleep while driving in obstructive sleep apnea syndrome. Sleep Breath 2015;19:1229-34. [Crossref] [PubMed]
- Bubu OM, Andrade AG, Umasabor-Bubu OQ, et al. Obstructive sleep apnea, cognition and Alzheimer's disease: A systematic review integrating three decades of multidisciplinary research. Sleep Med Rev 2020;50:101250. [Crossref] [PubMed]
- Bucks RS, Olaithe M, Rosenzweig I, et al. Reviewing the relationship between OSA and cognition: Where do we go from here? Respirology 2017;22:1253-61. [Crossref] [PubMed]
- Dunietz GL, Chervin RD, Burke JF, et al. Obstructive sleep apnea treatment and dementia risk in older adults. Sleep 2021;44:zsab076. [Crossref] [PubMed]
- Polsek D, Gildeh N, Cash D, et al. Obstructive sleep apnoea and Alzheimer's disease: In search of shared pathomechanisms. Neurosci Biobehav Rev 2018;86:142-9. [Crossref] [PubMed]
- Stubbs B, Vancampfort D, Veronese N, et al. The prevalence and predictors of obstructive sleep apnea in major depressive disorder, bipolar disorder and schizophrenia: A systematic review and meta-analysis. J Affect Disord 2016;197:259-67. [Crossref] [PubMed]
- Nobili L, Beniczky S, Eriksson SH, et al. Expert Opinion: Managing sleep disturbances in people with epilepsy. Epilepsy Behav 2021; Epub ahead of print. [Crossref]
- Rosenzweig I, Williams SC, Morrell MJ. CrossTalk opposing view: the intermittent hypoxia attending severe obstructive sleep apnoea does not lead to alterations in brain structure and function. J Physiol 2013;591:383-5; discussion 387,389.
- Rosenzweig I, Glasser M, Polsek D, et al. Sleep apnoea and the brain: a complex relationship. Lancet Respir Med 2015;3:404-14. [Crossref] [PubMed]
- Javaheri S, Barbe F, Campos-Rodriguez F, et al. Sleep Apnea: Types, Mechanisms, and Clinical Cardiovascular Consequences. J Am Coll Cardiol 2017;69:841-58. [Crossref] [PubMed]
- Tasali E, Ip MS. Obstructive sleep apnea and metabolic syndrome: alterations in glucose metabolism and inflammation. Proc Am Thorac Soc 2008;5:207-17. [Crossref] [PubMed]
- Otto-Yáñez M, Torres-Castro R, Nieto-Pino J, et al. Obstructive sleep apnea-hypopnea and stroke. Medicina (B Aires) 2018;78:427-35.
- Lee JJ, Sundar KM. Evaluation and Management of Adults with Obstructive Sleep Apnea Syndrome. Lung 2021;199:87-101. [Crossref] [PubMed]
- Ruehland WR, Rochford PD, O'Donoghue FJ, et al. The new AASM criteria for scoring hypopneas: impact on the apnea hypopnea index. Sleep 2009;32:150-7. [Crossref] [PubMed]
- Benjafield AV, Ayas NT, Eastwood PR, et al. Estimation of the global prevalence and burden of obstructive sleep apnoea: a literature-based analysis. Lancet Respir Med 2019;7:687-98. [Crossref] [PubMed]
- Davidson TM, Sedgh J, Tran D, et al. The anatomic basis for the acquisition of speech and obstructive sleep apnea: evidence from cephalometric analysis supports The Great Leap Forward hypothesis. Sleep Med 2005;6:497-505. [Crossref] [PubMed]
- Montero Benavides A, Blanco Murillo JL, Fernández Pozo R, et al. Formant Frequencies and Bandwidths in Relation to Clinical Variables in an Obstructive Sleep Apnea Population. J Voice 2016;30:21-9. [Crossref] [PubMed]
- Tyan M, Espinoza-Cuadros F, Fernández Pozo R, et al. Obstructive Sleep Apnea in Women: Study of Speech and Craniofacial Characteristics. JMIR Mhealth Uhealth 2017;5:e169. [Crossref] [PubMed]
- Cernomaz AT, Boişteanu D, Vasiluţă R, et al. Obstructive sleep apnea patients voice analysis. Rev Med Chir Soc Med Nat Iasi 2010;114:707-10.
- Janott C, Schuller B, Heiser C. Acoustic information in snoring noises. HNO 2017;65:107-16. [Crossref] [PubMed]
- Zhang Z. Mechanics of human voice production and control. J Acoust Soc Am 2016;140:2614. [Crossref] [PubMed]
- Fox AW, Monoson PK, Morgan CD. Speech dysfunction of obstructive sleep apnea. A discriminant analysis of its descriptors. Chest 1989;96:589-95. [Crossref] [PubMed]
- Fiz JA, Morera J, Abad J, et al. Acoustic analysis of vowel emission in obstructive sleep apnea. Chest 1993;104:1093-6. [Crossref] [PubMed]
- Robb MP, Yates J, Morgan EJ. Vocal tract resonance characteristics of adults with obstructive sleep apnea. Acta Otolaryngol 1997;117:760-3. [Crossref] [PubMed]
- Yaslıkaya S, Geçkil AA, Birişik Z. Is There a Relationship between Voice Quality and Obstructive Sleep Apnea Severity and Cumulative Percentage of Time Spent at Saturations below Ninety Percent: Voice Analysis in Obstructive Sleep Apnea Patients. Medicina (Kaunas) 2022;58:1336. [Crossref] [PubMed]
- Sataloff RT, Heman-Ackah YD, Hawkshaw MJ. Clinical anatomy and physiology of the voice. Otolaryngol Clin North Am 2007;40:909-29. v. [Crossref] [PubMed]
- Macari AT, Karam IA, Tabri D, et al. Formants frequency and dispersion in relation to the length and projection of the upper and lower jaws. J Voice 2015;29:83-90. [Crossref] [PubMed]
- Macari AT, Karam IA, Tabri D, et al. Correlation between the length and sagittal projection of the upper and lower jaw and the fundamental frequency. J Voice 2014;28:291-6. [Crossref] [PubMed]
- Fernández Pozo R, Blanco Murillo JL, Hernández Gómez L, et al. Assessment of Severe Apnoea through Voice Analysis, Automatic Speech, and Speaker Recognition Techniques. EURASIP Journal on Advances in Signal Processing 2009;2009:982531.
- Goldshtein E, Tarasiuk A, Zigel Y. Automatic detection of obstructive sleep apnea using speech signals. IEEE Trans Biomed Eng 2011;58:1373-82. [Crossref] [PubMed]
- Benavides AM, Blanco JL, Fernández A, et al. Using HMM to Detect Speakers with Severe Obstructive Sleep Apnoea Syndrome. In: Torre Toledano D, Giménez AO, Teixeira A, et al. editors. Advances in Speech and Language Technologies for Iberian Languages. Communications in Computer and Information Science, vol 328. Berlin, Heidelberg: Springer, Berlin, Heidelberg, 2012.
- Solé-Casals J, Munteanu C, Martín OC, et al. Detection of severe obstructive sleep apnea through voice analysis. Applied Soft Computing 2014;23:346-54.
- Espinoza-Cuadros F, Fernández-Pozo R, Toledano DT, et al. Reviewing the connection between speech and obstructive sleep apnea. Biomed Eng Online 2016;15:20. [Crossref] [PubMed]
- Ding Y, Wang J, Gao J, et al. Severity evaluation of obstructive sleep apnea based on speech features. Sleep Breath 2021;25:787-95. [Crossref] [PubMed]
- Ding Y, Sun Y, Li Y, et al. Selection of OSA-specific pronunciations and assessment of disease severity assisted by machine learning. J Clin Sleep Med 2022;18:2663-72. [Crossref] [PubMed]
- Ghoneim MM. Mechanism of snoring. JAMA 1981;245:1729.
- Pevernagie D, Aarts RM, De Meyer M. The acoustics of snoring. Sleep Med Rev 2010;14:131-44. [Crossref] [PubMed]
- Parker RJ, Hardinge M, Jeffries C. Snoring. BMJ 2005;331:1063. [Crossref] [PubMed]
- Deary V, Ellis JG, Wilson JA, et al. Simple snoring: not quite so simple after all? Sleep Med Rev 2014;18:453-62. [Crossref] [PubMed]
- Perez-Padilla JR, Slawinski E, Difrancesco LM, et al. Characteristics of the snoring noise in patients with and without occlusive sleep apnea. Am Rev Respir Dis 1993;147:635-44. [Crossref] [PubMed]
- Fiz JA, Abad J, Jané R, et al. Acoustic analysis of snoring sound in patients with simple snoring and obstructive sleep apnoea. Eur Respir J 1996;9:2365-70. [Crossref] [PubMed]
- Sola-Soler J, Jane R, Fiz JA, et al. Spectral envelope analysis in snoring signals from simple snorers and patients with Obstructive Sleep Apnea. Proceedings of the 25th Annual International Conference of the IEEE Engineering in Medicine and Biology Society (IEEE Cat. No.03CH37439); 2003. 17-21 Sept. 2003.
- Ng AK, Koh TS, Baey E, et al. Could formant frequencies of snore signals be an alternative means for the diagnosis of obstructive sleep apnea? Sleep Med 2008;9:894-8. [Crossref] [PubMed]
- Herzog M, Schmidt A, Bremert T, et al. Analysed snoring sounds correlate to obstructive sleep disordered breathing. Eur Arch Otorhinolaryngol 2008;265:105-13. [Crossref] [PubMed]
- Hara H, Murakami N, Miyauchi Y, et al. Acoustic analysis of snoring sounds by a multidimensional voice program. Laryngoscope 2006;116:379-81. [Crossref] [PubMed]
- Sola-Soler J, Jane R, Fiz JA, et al. Variability of snore parameters in time and frequency domains in snoring subjects with and without Obstructive Sleep Apnea. Conf Proc IEEE Eng Med Biol Soc 2005;2005:2583-6. [Crossref] [PubMed]
- Alshaer H, Hummel R, Mendelson M, et al. Objective Relationship Between Sleep Apnea and Frequency of Snoring Assessed by Machine Learning. J Clin Sleep Med 2019;15:463-70. [Crossref] [PubMed]
- Solà-Soler J, Jané R, Fiz JA, et al. Automatic classification of subjects with and without sleep apnea through snoring analysis. Annu Int Conf IEEE Eng Med Biol Soc 2007;2007:6094-7. [Crossref] [PubMed]
- Karunajeewa AS, Abeyratne UR, Hukins C. Multi-feature snore sound analysis in obstructive sleep apnea-hypopnea syndrome. Physiol Meas 2011;32:83-97. [Crossref] [PubMed]
- Cavusoglu M, Kamasak M, Erogul O, et al. An efficient method for snore/nonsnore classification of sleep sounds. Physiol Meas 2007;28:841-53. [Crossref] [PubMed]
- Mesquita J, Solà-Soler J, Fiz JA, et al. All night analysis of time interval between snores in subjects with sleep apnea hypopnea syndrome. Med Biol Eng Comput 2012;50:373-81. [Crossref] [PubMed]
- Jiang Y, Peng J, Song L. An OSAHS evaluation method based on multi-features acoustic analysis of snoring sounds. Sleep Med 2021;84:317-23. [Crossref] [PubMed]
- Kim T, Kim JW, Lee K. Detection of sleep disordered breathing severity using acoustic biomarker and machine learning techniques. Biomed Eng Online 2018;17:16. [Crossref] [PubMed]
- Shen F, Cheng S, Li Z, et al. Detection of Snore from OSAHS Patients Based on Deep Learning. J Healthc Eng 2020;2020:8864863. [Crossref] [PubMed]
- Jiang Y, Peng J, Zhang X. Automatic snoring sounds detection from sleep sounds based on deep learning. Phys Eng Sci Med 2020;43:679-89. [Crossref] [PubMed]
- Abeyratne UR, Wakwella AS, Hukins C. Pitch jump probability measures for the analysis of snoring sounds in apnea. Physiol Meas 2005;26:779-98. [Crossref] [PubMed]
- Abeyratne UR, de Silva S, Hukins C, et al. Obstructive sleep apnea screening by integrating snore feature classes. Physiol Meas 2013;34:99-121. [Crossref] [PubMed]
- Sharma G, Umapathy K, Krishnan S. Trends in audio signal feature extraction methods. Applied Acoustics 2020;158:107020.
- Low DM, Bentley KH, Ghosh SS. Automated assessment of psychiatric disorders using speech: A systematic review. Laryngoscope Investig Otolaryngol 2020;5:96-116. [Crossref] [PubMed]
- Mencar C, Gallo C, Mantero M, et al. Application of machine learning to predict obstructive sleep apnea syndrome severity. Health Informatics J 2020;26:298-317. [Crossref] [PubMed]
- Ramesh J, Keeran N, Sagahyroon A, et al. Towards Validating the Effectiveness of Obstructive Sleep Apnea Classification from Electronic Health Records Using Machine Learning. Healthcare (Basel) 2021;9:1450. [Crossref] [PubMed]
- Espinoza-Cuadros F, Fernández-Pozo R, Toledano DT, et al. Speech Signal and Facial Image Processing for Obstructive Sleep Apnea Assessment. Comput Math Methods Med 2015;2015:489761. [Crossref] [PubMed]
- Butler MP, Emch JT, Rueschman M, et al. Apnea-Hypopnea Event Duration Predicts Mortality in Men and Women in the Sleep Heart Health Study. Am J Respir Crit Care Med 2019;199:903-12. [Crossref] [PubMed]
- Sagaspe P, Taillard J, Chaumet G, et al. Maintenance of wakefulness test as a predictor of driving performance in patients with untreated obstructive sleep apnea. Sleep 2007;30:327-30.
- Sasaki N, Nagai M, Mizuno H, et al. Associations Between Characteristics of Obstructive Sleep Apnea and Nocturnal Blood Pressure Surge. Hypertension 2018;72:1133-40. [Crossref] [PubMed]