Association between anion gap and the 30-day mortality of patients with ventilator-associated pneumonia: a study of the MIMIC-III database
Highlight box
Key findings
• The 30-day mortality in ventilator-associated pneumonia (VAP) patients increased along with the elevated serum anion gap (AG). The AG-related multivariate model (including AG, age, duration of mechanical ventilation, and vasopressors use) had a potential predictive value on 30-day mortality in VAP. In addition, the relationship of AG with 30-day mortality was found in male, bacterial infection, and fungal infection subgroups.
What is known and what is new?
• AG had a potential positive association with short-time mortality in patients with VAP, indicating serum AG should be paid close attention in the intensive care units patients who diagnosed as VAP in clinical practice.
• The AG-related multivariate model had a relatively superior predictive performance on 30-day mortality in VAP patients to the single AG value.
What are the implications, and what should change now?
• Focusing on serum AG level in VAP patients and keeping it within an appropriate range may be important for those who with a high risk of short-time mortality. Further studies could explore a more convenient and low-cost predictive model basing on the AG-related multivariate model to reduce the mortality in patients with VAP.
Introduction
Ventilator-associated pneumonia (VAP) is an important nosocomial infection of intensive care units (ICUs) in patients with mechanical ventilation, and generally occurs >48 hours after endotracheal intubation (1,2). VAP is often characterized by fever, purulent sputum, leukocytosis, and decreased oxygenation, and its treatment depends on many factors such as age and the responsible pathogens (3). The occurrence of VAP often prolongs ventilatory support, and the stays in the ICU, and facilitates nosocomial transmission, which could further increase economic burden and the risk of mortality (4). Therefore, it is meaningful to investigate the influencing factors or predictive indexes on the risk of short-term mortality in VAP patients.
In clinical practices, the mechanical ventilation may induce respiratory acid-base disturbances, because the plasma pH depends on adequate ventilation to expel CO2 (5). The serum anion gap (AG) is caused by the proportional decrease in anion excretion and/or an excessive production of organic acid anions that can potentially be applied in the diagnosis of various metabolic acidosis (6). Previous studies have indicated the AG is associated with the risk of mortality in patients with aortic aneurysm (7), coronary artery disease (CAD) (8), sepsis (9), acute myocardial infarction (AMI), and chronic kidney disease (CKD) (10). In addition, recent research has reported the association of AG with the mortality of patients with coronavirus disease 2019 (COVID-19), an epidemic respiratory disease, for whom the mechanical ventilation was commonly used as an adjuvant therapy (11).
Given the poor prognoses of VAP in the ICU patients, researchers keep seeking a simple and reliable prognostic marker (12). However, no epidemiological study has explored the association of AG with the prognoses of patients with VAP. Herein, the current study aimed to investigate the association of AG with the 30-day mortality of patients with VAP. Also, we constructed and assessed an AG-related multivariate model to predict the risk of 30-day mortality in VAP patients; and besides, we compared its predictive value with some common scoring systems, including the Sequential Organ Failure Assessment (SOFA) score, the confusion, urea, respiratory rate (RR), blood pressure, and age (≥65 years old) (CURB-65) score, and the blood urea nitrogen (BUN), altered mental status, pulse, and age (>65 years old) (BAP-65) score. We hope our findings could provide some references for exploring prognostic biomarkers, and help reduce the disease burdens of patients with VAP. We present this article in accordance with the STROBE reporting checklist (available at https://jtd.amegroups.com/article/view/10.21037/jtd-23-1735/rc).
Methods
Study design and participants
This investigation was a single-center retrospective cohort study. The study was conducted in accordance with the Declaration of Helsinki (as revised in 2013). We extracted data of patients with VAP from the Medical Information Mart for Intensive Care III (MIMIC-III) database. The MIMIC-III database was jointly published by the Computational Physiology Laboratory of Massachusetts Institute of Technology (MIT), Beth Israel Deaconess Medical Center (BIDMC), and Philips Medical, which collected and sorted out the information on clinical diagnosis and treatment of more than 40,000 real patients who are predominantly white people that lived in the ICU of the BIDMC in 2001–2012 (13). More details are publicly available on the MIMIC-III website: https://physionet.org/content/mimiciii/1.4/ (14).
Data of 547 adult patients with VAP were initially included. The exclusion criteria were (I) patients received mechanical ventilation for less than 48 hours; (II) patients stayed in the ICU less than 48 hours; and (III) information on serum AG or survival missed. Finally, 477 were eligible for further analyses. Since the database was publicly available, no ethical approval from our institutional review board was required.
Diagnosis of VAP
VAP was diagnosed on the basis of the International Classification of Diseases code, the 9th version (ICD-9) with the code of 99731 (VAP). In addition, to ensure we have included all cases of VAP, we searched for VAP-related keywords in the ICD-9, including VAP and ventilator-associated pneumonia (15).
Study outcome and follow-up duration
The outcome of this study was 30-day mortality. In the MIMIC-IV database, the in-hospital information of patients was recorded by the hospital department, and that the out-of-hospital information was recorded by the Social Security Bureau. Hence, the information on mortality could be obtained from the patients’ personal medical records. In addition, the follow-up started at the time of first ICU admission, and ended when patients discharged/died or 30 days after the ICU admission.
Potential covariates
We selected the potential covariates from the database: (I) population characteristics including age, gender, and ethnicity; (II) vital signs including body mass index (BMI; kg/m2), heart rate (HR), systolic blood pressure (SBP), diastolic blood pressure (DBP), RR, and temperature; (III) laboratory inspection examination between 12 hours before mechanical ventilation and 24 hours after mechanical ventilation, including white blood cell (WBC), platelet (PLT), hemoglobin (HB), red blood cell distribution width (RDW), creatinine (Cr), international normalized ratio (INR), prothrombin time (PT), potassium (K), BUN, glucose, lactate, pH, oxygen saturation index [OSI; calculated through the formula: OSI = pulse oximetry saturation (SpO2)/fraction of inspired oxygen ratio (FiO2)], and partial pressure of carbon dioxide (PCO2); (IV) pathogen detection including number of pathogens, types of infection (bacterial and fungal), and responsible bacteria [pseudomonas aeruginosa, Methicillin-resistant Staphylococcus aureus (MRSA), and Staphylococcus aureus]; (V) complications including effusion, emphysema, atelectasis, pneumothorax, lung abscess, and the elixhauser comorbidity index (ECI); (VI) treatments including duration of mechanical ventilation, vasopressors use, and antibiotic therapy; and (VII) disease severity scoring systems including CURB-65, Glasgow Coma Scale (GCS), SOFA, and BAP-65.
The calculations for scoring systems including CURB-65, BAP-65, and SOFA were as follow. In brief, the BAP-65 consists of four components, and each component is assigned 1 point, with a score ranging from 0 to 4. The parameter accounts for 1 point each when BUN >25 mg/dL (urea >53.5 mg/dL), altered mental status, pulse rate >109 beats per minute (bpm), and age >65 years old (16). Similarly, the CURB-65 contains five parameters, and each parameter is allocated 1 point, including confusion (defined as a Mental Test Score of 8 or less, or new disorientation in person, place, or time), blood urea >7 mmol/L, RR ≥30 per minute, blood pressure (SBP <90 mmHg or DBP ≤60 mmHg), and age >65 years old, which results in a six-point score ranging from 0 to 5 (17). Also, the SOFA score consists of six components including partial pressure of oxygen (PaO2)/FiO2 (P/F) ratio (mmHg), PLT ×103 (mm3), bilirubin (µmol/L), hypotension, GCS, and Cr (mg/dL) or urine output (mL/day) (18), and each component is allocated a score ranging from 0 to 4. In particular, among these parameters, due to data on the variable “PaO2” were all missing in the database, we calculated the OSI as it is a noninvasive surrogate for P/F ratio.
Statistical analysis
Mean ± standard deviation (SD) was used to describe the normal distributed data, and t-test was utilized to compare the difference between the two groups. Median (25% quantile, 75% quantile) [M (Q1, Q3)] were employed to describe the skewed distributed data, and Wilcoxon rank sum test was used for comparations. Frequency and composition ratio [n (%)] was utilized to describe the distribution of categorical data, and chi-square test (χ2) was employed for comparations.
In order to construct the AG-related multivariate predictive model on 30-day mortality of VAP patients, we divided data of participants into a training set and a testing set in a 7:3 ratio via completely random sampling method. Then, in the training set, we firstly compared the characteristics of patients between the survival group and 30-day mortality group through univariate Cox regression analysis. The variables with significant difference between these two groups were included in further screening for multivariate model’s components, where stepwise regression analysis was utilized. Finally, the multivariate predictive model included AG, age, duration of mechanical ventilation, and vasopressors use. Also, in both training set and testing set, we compared the predictive performance of multivariate model on 30-day mortality of VAP patients with other scoring systems (including CURB-65, BAP-65, and SOFA) and AG. Decision curves were drawn to assess the predictive value of different predictive models. Besides, the associations of AG with 30-day mortality in subgroups of gender, age, and pathogen were explored.
Evaluation indexes were hazard ratios (HRs), C-indexes, and 95% confidence intervals (CIs). The bilateral P<0.05 was considered as significant association. Descriptive statistics, inter-group comparison, and regression analyses were completed by SAS 9.4 (SAS Institute., Cary, NC, USA), whereas Cox modeling and C-index calculation were completed by R (R version 4.0.3). Variables including missing data were shown in Table S1. When a variable had a proportion of missing data more than 20%, it was deleted, and otherwise it was interpolated using multiple imputation method.
Results
Characteristics of participants
Figure 1 is the flowchart of participants screening. Initially, 547 patients with VAP from the MIMIC-III database were included. Patients who received mechanical ventilation <48 hours or stayed in the ICU for less than 48 hours (n=68), or did not have the information on serum AG or survival (n=2) were excluded. Finally, 477 patients were eligible for this study.
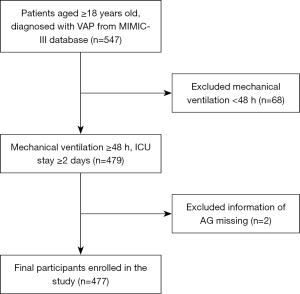
Comparing VAP patients between the training set (n=333) and testing set (n=144), their characteristics had no significant difference, indicating that the partitioning method was relatively robust (Table 1). Table 2 shows characteristics of patients between the survival group and mortality group in the training set. A total of 70 patients died within 30 days, with the mean follow-up time of 13.13 days. The average age of patients in the survival group and 30-day mortality group was 59.76 years old and 70.20 years old, respectively. The median CURB-65 score (3.00 vs. 2.00) and serum AG (16.66 vs. 14.95) in the 30-day mortality group were both significantly higher than those in the survival group. In addition, BUN, lactate, ECI, duration of mechanical ventilation, and vasopressors use were all significantly different between these two groups (all P<0.05).
Table 1
Variables | Total (n=477) | Training set (n=333) | Testing set (n=144) | Statistics | P |
---|---|---|---|---|---|
Age (years) | 61.79±16.94 | 61.95±16.45 | 61.42±18.07 | t=0.320 | 0.75 |
Gender | χ2=0.055 | 0.81 | |||
Female | 186 (38.99) | 131 (39.34) | 55 (38.19) | ||
Male | 291 (61.01) | 202 (60.66) | 89 (61.81) | ||
Ethnicity | χ2=0.865 | 0.65 | |||
White | 316 (66.25) | 225 (67.57) | 91 (63.19) | ||
Black | 45 (9.43) | 30 (9.01) | 15 (10.42) | ||
Other/unknown | 116 (24.32) | 78 (23.42) | 38 (26.39) | ||
BMI (kg/m2) | 27.62 (23.70, 33.01) | 27.61 (23.46, 33.21) | 27.95 (24.44, 32.45) | Z=0.604 | 0.55 |
Heart rate (bpm) | 89.94±21.60 | 88.75±22.20 | 92.69±19.94 | t=−1.830 | 0.07 |
SBP (mmHg) | 127.42±28.66 | 127.33±29.76 | 127.64±26.04 | t=−0.110 | 0.91 |
DBP (mmHg) | 69.03±19.33 | 69.40±18.92 | 68.19±20.30 | t=0.630 | 0.53 |
RR (bpm) | 19.89±6.52 | 19.78±6.46 | 20.15±6.66 | t=−0.570 | 0.57 |
Temperature (℃) | 36.60±1.09 | 36.60±1.15 | 36.60±0.96 | t=−0.030 | 0.98 |
WBC (k/μL) | 12.00 (8.80, 16.80) | 11.70 (8.80, 16.20) | 12.50 (8.70, 17.70) | Z=1.289 | 0.20 |
PLT (k/μL) | 218.00 (159.00, 280.00) | 220.00 (160.00, 279.00) | 214.00 (156.00, 288.00) | Z=0.126 | 0.90 |
HB (g/dL) | 11.39±2.46 | 11.45±2.47 | 11.24±2.45 | t=0.840 | 0.40 |
RDW (%) | 15.10±1.99 | 15.15±2.03 | 15.00±1.90 | t=0.760 | 0.45 |
Cr (mg/dL) | 1.00 (0.80, 1.60) | 1.00 (0.80, 1.50) | 1.10 (0.80, 1.70) | Z=0.684 | 0.49 |
INR | 1.20 (1.10, 1.50) | 1.20 (1.10, 1.50) | 1.20 (1.10, 1.50) | Z=0.093 | 0.93 |
PT (seconds) | 14.40 (13.00, 17.00) | 14.40 (13.00, 17.00) | 14.50 (13.10, 16.70) | Z=−0.177 | 0.86 |
K (mEq/L) | 4.23±0.83 | 4.17±0.79 | 4.36±0.90 | t=−2.290 | 0.12 |
BUN (mg/dL) | 21.00 (14.00, 34.00) | 20.00 (14.00, 34.00) | 21.50 (14.00, 36.00) | Z=0.821 | 0.41 |
Glucose (mg/dL) | 140.00 (113.00, 182.00) | 143.00 (113.00, 184.00) | 138.00 (111.00, 167.50) | Z=−1.087 | 0.28 |
Lactate (mmol/L) | 1.70 (1.10, 2.90) | 1.70 (1.10, 2.90) | 1.70 (1.10, 2.90) | Z=−0.017 | 0.99 |
pH | 7.34±0.12 | 7.34±0.12 | 7.34±0.12 | t=0.270 | 0.79 |
OSI | 1.24 (0.99, 1.98) | 1.07 (0.99, 1.98) | 1.43 (1.00, 2.00) | Z=0.961 | 0.34 |
PCO2 | 43.00 (37.00, 51.00) | 43.00 (37.00, 51.00) | 43.00 (37.00, 50.00) | Z=0.370 | 0.71 |
Pathogens | 3.00 (2.00, 4.00) | 3.00 (2.00, 4.00) | 3.00 (1.50, 4.00) | Z=−0.393 | 0.69 |
Bacterial infection | χ2=0.018 | 0.89 | |||
No | 68 (14.26) | 47 (14.11) | 21 (14.58) | ||
Yes | 409 (85.74) | 286 (85.89) | 123 (85.42) | ||
Pseudomonas aeruginosa | χ2=0.563 | 0.45 | |||
No | 459 (96.23) | 319 (95.80) | 140 (97.22) | ||
Yes | 18 (3.77) | 14 (4.20) | 4 (2.78) | ||
MRSA | χ2=0.000 | 0.99 | |||
No | 444 (93.08) | 310 (93.09) | 134 (93.06) | ||
Yes | 33 (6.92) | 23 (6.91) | 10 (6.94) | ||
Staphylococcus aureus | χ2=1.173 | 0.28 | |||
No | 441 (92.45) | 305 (91.59) | 136 (94.44) | ||
Yes | 36 (7.55) | 28 (8.41) | 8 (5.56) | ||
Fungal infection | χ2=0.507 | 0.48 | |||
No | 230 (48.22) | 157 (47.15) | 73 (50.69) | ||
Yes | 247 (51.78) | 176 (52.85) | 71 (49.31) | ||
Effusion | – | 0.32 | |||
No | 473 (99.16) | 329 (98.80) | 144 (100.00) | ||
Yes | 4 (0.84) | 4 (1.20) | 0 (0.00) | ||
Emphysema | – | 0.67 | |||
No | 471 (98.74) | 328 (98.50) | 143 (99.31) | ||
Yes | 6 (1.26) | 5 (1.50) | 1 (0.69) | ||
ECI | 15.00 (8.00, 23.00) | 15.00 (8.00, 23.00) | 16.00 (8.50, 23.50) | Z=0.562 | 0.57 |
Duration of mechanical ventilation (hours) | 262.00 (166.53, 412.25) | 265.22 (172.87, 437.20) | 256.38 (150.41, 372.15) | Z=−1.173 | 0.24 |
Vasopressors use | χ2=2.084 | 0.15 | |||
No | 251 (52.62) | 168 (50.45) | 83 (57.64) | ||
Yes | 226 (47.38) | 165 (49.55) | 61 (42.36) | ||
Antibiotic therapy | χ2=0.920 | 0.34 | |||
No | 180 (37.74) | 121 (36.34) | 59 (40.97) | ||
Yes | 297 (62.26) | 212 (63.66) | 85 (59.03) | ||
CURB-65 score | 2.00 (1.00, 3.00) | 2.00 (1.00, 3.00) | 2.00 (1.00, 3.00) | Z=0.472 | 0.64 |
BAP-65 score | 3.49±0.64 | 3.48±0.64 | 3.53±0.63 | t=−0.790 | 0.43 |
GCS | 12.51±3.84 | 12.44±3.97 | 12.69±3.53 | t=−0.650 | 0.52 |
SOFA score | 7.00 (5.00, 10.00) | 7.00 (5.00, 10.00) | 6.50 (5.00, 10.00) | Z=−1.151 | 0.25 |
AG | 15.00 (12.00, 17.00) | 15.00 (12.00, 17.00) | 14.00 (12.00, 17.00) | Z=−0.148 | 0.88 |
Survival time (days) | 26.70±7.19 | 26.71±7.21 | 26.69±7.18 | t=0.020 | 0.98 |
Outcomes | χ2=0.002 | 0.96 | |||
Survival | 377 (79.04) | 263 (78.98) | 114 (79.17) | ||
30-day mortality | 100 (20.96) | 70 (21.02) | 30 (20.83) |
Data are presented as mean ± SD, n (%), or M (Q1, Q3). T, t-test; Z, rank sum test; χ2, chi-square test. VAP, ventilator-associated pneumonia; BMI, body mass index; bpm, beats per minute; SBP, systolic blood pressure; DBP, diastolic blood pressure; RR, respiratory rate; WBC, white blood cell; PLT, platelet; HB, hemoglobin; RDW, red blood cell distribution width; Cr, creatinine; INR, international normalized ratio; PT, prothrombin time; K, potassium; BUN, blood urea nitrogen; OSI, oxygen saturation index; PCO2, partial pressure of carbon dioxide; MRSA, Methicillin-resistant Staphylococcus aureus; ECI, elixhauser comorbidity index; CURB-65, confusion, urea, respiratory rate, blood pressure, and age (≥65 years old); BAP-65, blood urea nitrogen, altered mental status, pulse, and age (>65 years old); GCS, Glasgow Coma Scale; SOFA, Sequential Organ Failure Assessment; AG, anion gap; SD, standard difference; M, median; Q1, 25% quantile; Q3, 75% quantile.
Table 2
Variables | Total (n=333) | Survival (n=263) | 30-day mortality (n=70) | Statistics | P |
---|---|---|---|---|---|
Age (years) | 61.95±16.45 | 59.76±16.22 | 70.20±14.67 | t=−4.880 | <0.001 |
Gender | χ2=3.166 | 0.08 | |||
Female | 131 (39.34) | 97 (36.88) | 34 (48.57) | ||
Male | 202 (60.66) | 166 (63.12) | 36 (51.43) | ||
Ethnicity | χ2=4.461 | 0.11 | |||
White | 225 (67.57) | 183 (69.58) | 42 (60.00) | ||
Black | 30 (9.01) | 25 (9.51) | 5 (7.14) | ||
Other/unknown | 78 (23.42) | 55 (20.91) | 23 (32.86) | ||
BMI (kg/m2) | 27.61 (23.46, 33.21) | 28.02 (23.71, 33.78) | 25.54 (21.57, 32.65) | Z=−1.811 | 0.07 |
Heart rate (bpm) | 88.75±22.20 | 89.18±22.21 | 87.14±22.22 | t=0.680 | 0.50 |
SBP (mmHg) | 127.33±29.76 | 127.54±29.54 | 126.56±30.78 | t=0.240 | 0.81 |
DBP (mmHg) | 69.40±18.92 | 70.27±18.89 | 66.11±18.78 | t=1.640 | 0.10 |
RR (bpm) | 19.00 (15.00, 23.00) | 19.00 (15.00, 23.00) | 20.00 (15.00, 23.00) | Z=0.449 | 0.65 |
Temperature (℃) | 36.60±1.15 | 36.64±1.15 | 36.43±1.13 | t=1.320 | 0.19 |
WBC (k/μL) | 11.70 (8.80, 16.20) | 11.60 (8.70, 16.30) | 12.15 (8.80, 16.00) | Z=0.367 | 0.71 |
PLT (k/μL) | 220.00 (160.00, 279.00) | 219.00 (160.00, 274.00) | 223.00 (162.00, 285.00) | Z=0.139 | 0.89 |
HB (g/dL) | 11.45±2.47 | 11.49±2.51 | 11.31±2.29 | t=0.550 | 0.58 |
RDW (%) | 15.15±2.03 | 15.10±2.02 | 15.32±2.09 | t=−0.810 | 0.42 |
Cr (mg/dL) | 1.00 (0.80, 1.50) | 1.00 (0.70, 1.50) | 1.15 (0.80, 1.70) | Z=1.643 | 0.10 |
INR | 1.20 (1.10, 1.50) | 1.20 (1.10, 1.50) | 1.20 (1.10, 1.60) | Z=1.267 | 0.21 |
PT (seconds) | 14.40 (13.00, 17.00) | 14.30 (12.90, 17.00) | 14.55 (13.10, 17.10) | Z=0.855 | 0.39 |
K (mEq/L) | 4.17±0.79 | 4.16±0.80 | 4.21±0.79 | t=−0.490 | 0.63 |
BUN (mg/dL) | 20.00 (14.00, 34.00) | 19.00 (13.00, 32.00) | 24.50 (16.00, 39.00) | Z=2.329 | 0.02 |
Glucose (mg/dL) | 143.00 (113.00, 184.00) | 139.00 (112.00, 184.00) | 156.00 (121.00, 188.00) | Z=1.601 | 0.11 |
Lactate (mmol/L) | 1.70 (1.10, 2.90) | 1.60 (1.10, 2.60) | 2.40 (1.40, 4.00) | Z=3.185 | 0.001 |
pH | 7.34±0.12 | 7.34±0.12 | 7.36±0.11 | t=−1.120 | 0.27 |
OSI | 1.07 (0.99, 1.98) | 1.24 (0.99, 1.98) | 1.00 (0.98, 1.98) | Z=−1.164 | 0.24 |
PCO2 | 43.00 (37.00, 51.00) | 43.00 (37.00, 52.00) | 40.00 (35.00, 47.00) | Z=−2.517 | 0.01 |
Pathogens | 3.00 (2.00, 4.00) | 3.00 (2.00, 4.00) | 3.00 (2.00, 4.00) | Z=−0.422 | 0.67 |
Bacterial infection | χ2=0.187 | 0.67 | |||
No | 47 (14.11) | 36 (13.69) | 11 (15.71) | ||
Yes | 286 (85.89) | 227 (86.31) | 59 (84.29) | ||
Pseudomonas aeruginosa | – | 0.50 | |||
No | 319 (95.80) | 253 (96.20) | 66 (94.29) | ||
Yes | 14 (4.20) | 10 (3.80) | 4 (5.71) | ||
MRSA | – | 0.43 | |||
No | 310 (93.09) | 243 (92.40) | 67 (95.71) | ||
Yes | 23 (6.91) | 20 (7.60) | 3 (4.29) | ||
Staphylococcus aureus | χ2=2.278 | 0.13 | |||
No | 305 (91.59) | 244 (92.78) | 61 (87.14) | ||
Yes | 28 (8.41) | 19 (7.22) | 9 (12.86) | ||
Fungal infection | χ2=0.289 | 0.59 | |||
No | 157 (47.15) | 122 (46.39) | 35 (50.00) | ||
Yes | 176 (52.85) | 141 (53.61) | 35 (50.00) | ||
Effusion | – | 0.58 | |||
No | 329 (98.80) | 259 (98.48) | 70 (100.00) | ||
Yes | 4 (1.20) | 4 (1.52) | 0 (0.00) | ||
Emphysema | – | 0.59 | |||
No | 328 (98.50) | 258 (98.10) | 70 (100.00) | ||
Yes | 5 (1.50) | 5 (1.90) | 0 (0.00) | ||
ECI | 15.00 (8.00, 23.00) | 14.00 (7.00, 22.00) | 17.00 (10.00, 25.00) | Z=2.110 | 0.04 |
Duration of mechanical ventilation (hours) | 265.22 (172.87, 437.20) | 284.00 (182.00, 461.00) | 218.38 (138.00, 374.83) | Z=−2.686 | 0.007 |
Vasopressors use | χ2=7.699 | 0.006 | |||
No | 168 (50.45) | 143 (54.37) | 25 (35.71) | ||
Yes | 165 (49.55) | 120 (45.63) | 45 (64.29) | ||
Antibiotic therapy | χ2=3.370 | 0.07 | |||
No | 121 (36.34) | 89 (33.84) | 32 (45.71) | ||
Yes | 212 (63.66) | 174 (66.16) | 38 (54.29) | ||
CURB-65 score | 2.00 (1.00, 3.00) | 2.00 (1.00, 3.00) | 3.00 (2.00, 3.00) | Z=3.594 | <0.001 |
BAP-65 score | 3.48±0.64 | 3.46±0.66 | 3.56±0.56 | t=−1.170 | 0.24 |
GCS | 15.00 (10.00, 15.00) | 15.00 (11.00, 15.00) | 15.00 (8.00, 15.00) | Z=−1.077 | 0.28 |
SOFA score | 7.00 (5.00, 10.00) | 7.00 (5.00, 10.00) | 7.50 (6.00, 11.00) | Z=0.671 | 0.50 |
AG | 15.31±4.73 | 14.95±4.50 | 16.66±5.30 | t=−2.700 | 0.007 |
Follow-up time (days) | 30.00 (30.00, 30.00) | 30.00 (30.00, 30.00) | 13.13 (7.50, 20.61) | Z=−18.052 | <0.001 |
Data are presented as mean ± SD, n (%), or M (Q1, Q3). T, t-test; Z, rank sum test; χ2, chi-square test. VAP, ventilator-associated pneumonia; BMI, body mass index; bpm, beats per minute; SBP, systolic blood pressure; DBP, diastolic blood pressure; RR, respiratory rate; WBC, white blood cell; PLT, platelet; HB, hemoglobin; RDW, red blood cell distribution width; Cr, creatinine; INR, international normalized ratio; PT, prothrombin time; K, potassium; BUN, blood urea nitrogen; OSI, oxygen saturation index; PCO2, partial pressure of carbon dioxide; MRSA, Methicillin-resistant Staphylococcus aureus; ECI, elixhauser comorbidity index; CURB-65, confusion, urea, respiratory rate, blood pressure, and age (≥65 years old); BAP-65, blood urea nitrogen, altered mental status, pulse, and age (>65 years old); GCS, Glasgow Coma Scale; SOFA, Sequential Organ Failure Assessment; AG, anion gap; SD, standard difference; M, median; Q1, 25% quantile; Q3, 75% quantile.
Construction of multivariate predictive model on 30-day mortality in VAP patients
Table 3 shows the components of multivariate predictive model on 30-day mortality in VAP patients. To be specific, the significantly different variables between the survival group and 30-day mortality group were included in univariate Cox regression and stepwise regression analyses. The results showed that the risk of 30-day mortality increased 1.052 along with the AG elevated per unit (95% CI: 1.008–1.098), and increased 1.037 along with age elevated per unit (95% CI: 1.019–1.055). Whereas the 30-day mortality risk decreased 0.998 along with duration of mechanical ventilation elevated per unit (95% CI: 0.996–0.999). In addition, compared with patients who had not use vasopressors, those who had used seemed to have a higher risk of 30-day mortality (HR =1.795, 95% CI: 1.066–3.023).
Table 3
Components | HR (95% CI) | P |
---|---|---|
AG | 1.052 (1.008–1.098) | 0.02 |
Age | 1.037 (1.019–1.055) | <0.001 |
Duration of mechanical ventilation | 0.998 (0.996–0.999) | 0.002 |
Vasopressors | ||
No | Ref. | |
Yes | 1.795 (1.066–3.023) | 0.03 |
VAP, ventilator-associated pneumonia; HR, hazard ratio; CI, confidence interval; AG, anion gap; ref., reference.
Assessing the predictive performance of the multivariate model on 30-day mortality of VAP patients and comparing it with AG, CURB-65, BAP-65, and SOFA
We then assessed the predictive performance of the multivariate model on 30-day mortality in VAP patients in training set and testing set, respectively; and then we compared it with different scoring systems (Table 4). Compared with single AG value, only the multivariate model had a relatively superior predictive performance both in training set and testing set, with the C-index and 95% CI of 0.725 (0.670–0.780) and 0.717 (0.637–0.797), respectively. In addition, Figure 2 clearly shows that compared with the other scoring systems, the multivariate model had the highest benefit ratio when used to assess the risk of 30-day mortality in VAP patients, which indicated the multivariate model had a potential predictive value.
Table 4
Datasets | Training set (n=333) | Testing set (n=144) | |||
---|---|---|---|---|---|
C-index (95% CI) | P | C-index (95% CI) | P | ||
CURB-65 | 0.654 (0.570–0.738) | 0.13 | 0.623 (0.499–0.746) | 0.20 | |
BAP-65 | 0.577 (0.476–0.677) | 0.41 | 0.521 (0.357–0.684) | 0.38 | |
SOFA | 0.530 (0.460–0.601) | 0.09 | 0.588 (0.479–0.698) | 0.28 | |
AG | 0.591 (0.519–0.663) | Ref. | 0.550 (0.447–0.663) | Ref. | |
Multivariate model† | 0.725 (0.670–0.780) | <0.001 | 0.717(0.637–0.797) | 0.003 |
†, multivariate model consisted of AG, age, vasopressors use, and duration of mechanical ventilation. AG, anion gap; CURB-65, confusion, urea, respiratory rate, blood pressure, and age (≥65 years old); BAP-65, blood urea nitrogen, altered mental status, pulse, and age (>65 years old); SOFA, Sequential Organ Failure Assessment; VAP, ventilator-associated pneumonia; CI, confidence interval; ref., reference.
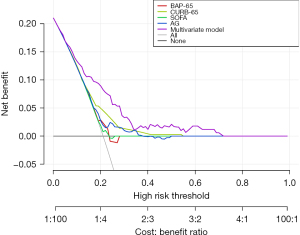
Relationship of AG with 30-day mortality in subgroups of gender, age, and infection status
Moreover, we investigated the association of AG with 30-day mortality in different subgroups (Table 5). Interestingly, the positive relationship between AG and 30-day mortality was only found in male patients (HR =1.088, 95% CI: 1.029–1.150). Although no significant associations were observed in age subgroup, whatever pathogens the patients infected, the risk of 30-day mortality was significantly increased (bacterial infection: HR =1.059, 95% CI: 1.011–1.109; fungal infection: HR =1.057, 95% CI: 1.002–1.115).
Table 5
Subgroups | HR (95% CI) | P |
---|---|---|
Gender | ||
Female | 1.015 (0.942–1.093) | 0.70 |
Male | 1.088 (1.029–1.150) | 0.003 |
Age | ||
<65 years old | 0.944 (0.851–1.048) | 0.28 |
≥65 years old | 1.009 (0.944–1.079) | 0.80 |
Pathogens | ||
Bacterial infection | 1.059 (1.011–1.109) | 0.02 |
Fungal infection | 1.057 (1.002–1.115) | 0.04 |
AG, anion gap; HR, hazard ratio; CI, confidence interval.
Discussion
The current study investigated the relationship of AG with 30-day mortality in patients with VAP, and constructed and assessed an AG-related multivariate model for predicting the 30-day mortality of VAP. The results showed that AG had a potential positive association with 30-day mortality in VAP patients, and this association was also observed in male, bacterial infection, and fungal infection subgroups. In addition, the AG-related multivariate model showed a relatively superior predictive performance to the single AG value on 30-day mortality in VAP.
VAP is a major complication in patients received mechanical ventilation, which often prolongs the stays in the ICU, and increases economic burdens as well as mortality risk of patients. The 30-day mortality of VAP patients was 21.02% in our study. A growing number of clinical studies have identified that blood biomarkers may reveal additional information about the prognosis of patients with pneumonia. AG as a strong influencer for mortality prediction model (19) may be a good choice to assess the prognosis of critically ill patients (20). Nevertheless, there still have been no research investigating the relationship of AG with short-term mortality in VAP patients so far. In a recent study, a higher level of AG was significantly correlated to an increased risk of all-cause mortality and short-term prognosis of cerebral infarction patients (21). Bezuidenhout et al. (22) found that pH levels in arterial blood were significantly linked to survival possibility of COVID-19 intensive care patients. On the basis of these previous studies, we speculated that AG may be involved in the prognosis of patients with VAP. In the current study, we found that the risk of 30-day mortality in VAP patients was significantly increased along with the elevated serum AG, which relatively supplemented the literature blank. However, the causal relationship between AG and the short-term mortality of VAP needs further clarification.
The specific mechanism that AG had a potential positive relationship with 30-day mortality of VAP is still unclear. In fact, AG in patients with VAP consists of a wide array of anionic substances. Anions such as indoxyl sulfate, indole-3 acetic acid, p-cresyl sulfate, and trimethylamine N-oxide are known to induce endothelial inflammation and oxidative stress (23), arteriosclerosis (24), and fibrosis (25). The poorer health condition was linked to a higher risk of the mortality in the ICU patients. Also, VAP patients with a higher AG level may have hypovolaemia or dehydration on the ICU admission, which might be caused either by severe infection or the presence of underlying complications such as renal impairment. Hence, when sodium (Na) and K are stable, and anionic acid products increase, in order to maintain the balance of anion and cation, the organism transfers the intracellular chlorine (Cl), which reduces the sum of bicarbonate radical (HCO3−) and Cl in plasma, thereby increasing the ratio of AG increases (26). In turn, the increased serum AG level may indicate a sicker condition in the VAP patients, who had a high possibility of short-term mortality.
The relationship of AG with 30-day mortality was also observed in male, bacterial infection, and fungal infection subgroups. According to previous evidences, male gender and broad-spectrum antimicrobial therapy are acknowledged risk factors for the development of VAP (27,28). Different to our finding, López-de-Andrés et al. (29) compared the incidence and in-hospital outcomes of VAP according to gender, and suggested that the in-hospital mortality rate for VAP was approximately 35% in patients of both genders. The possible explanation for this difference could be the ethnic difference between López-de-Andrés’s study and ours, because they used the Spanish National Hospital Discharge Database whereas our data were from patients in the United States. In our study, there were 60.66% male patients, 85.89% patients had bacterial infection, and 52.85% had fungal infection. Critically ill patients often exhibit loss of commensal microbiota, and overgrowth of potential pathogens, which thus highly perturbed microbial communities (30). Our research indicated that the imbalance of intestinal microbiome may result in the disturbance of acid-base balance in VAP patients, thereby affecting their prognoses. Herein, attentions should be paid to monitoring the serum AG in VAP patients, especially in male gender, whatever the infection status in the ICU.
In addition, we assessed the predictive performance of the AG-related multivariate model on 30-day mortality in VAP, and we compared it as well as other scoring systems with well as the single AG value. In our study, the multivariate model consisted variables that were significantly associated with 30-day mortality in VAP, including AG, age, duration of mechanical ventilation, and vasopressors use. It had a relatively superior predictive performance to the single AG, indicating that the comprehensive consideration of multiple indicators may be able to predict the short-term death outcome of VAP patients better in the clinical practice. Also, compared with the single AG value, other common scoring systems, such as CURB-65, BAP-65, and SOFA score, did not show significant differences in the predictive value. The SOFA score, CURB-65, and BAP-65 have been reported that they could be applied to assess the severity of pneumonia and predict mortality outcomes (31,32). A study on hospital mortality of patients with higher AG found that with the elevation of AG level, SOFA score increased significantly (33). Previous literature showed that high SOFA scores, inappropriate antibiotic therapy, diabetes mellitus, tracheostomy, CURB-65 score ≥3, high C-reactive protein levels, high Cr levels, VAP-related septic shock, and chronic respiratory disease are independent risk factors of the mortality in VAP (34). However, Oliva et al. (35) considered the CURB-65 scores or extended CURB-65 scores alone may not be fully suitable for COVID-19. Similarly, it reminded that the existing scoring system may not be particularly sensitive to the short-term mortality of VAP, and an index of conveniently and reliably predicting the VAP mortality, it studied on, has only just begun to uncover. Therefore, since AG had a potential association with 30-day mortality in VAP patients, and the AG multivariate model had a relatively good predictive performance, this study may provide some references for further exploration on predictors of short-term VAP mortality, which may further help clinicians to early identify patients with a high mortality risk in the ICU.
To the best of our knowledge, it was the first time to investigate the relationship of AG with the 30-day mortality of VAP, and to construct a multivariate model for predicting the prognosis of VAP. However, there were still some limitations in this current study. As a single-center retrospective study, it could not conclude a causal relationship between AG and the 30-day mortality of VAP patients. Also, due to the retrospective nature, the selection bias was unavoidable. Serum AG levels were influenced by circulating protein levels, but unfortunately, the plasma albumin data was missing in database. Similarly, data on PaO2 were missing in the database, thereby limiting the calculation of the P/F ratio. However, we used the OSI (a commonly used noninvasive surrogate for P/F ratio in clinical practice) to reflect the baseline respiratory status as well as the calculation of the SOFA score. Besides, we only extracted the information on AG levels at the start of mechanical ventilation, but it changes during the mechanical ventilation, which may also affect patients’ outcomes. Therefore, further prospective cohort studies are needed to investigate the causal associations of AG and AG changes during the ICU stay (with plasma albumin correction) with the short-term mortality in VAP patients.
Conclusions
AG was linked to the 30-day mortality in patients with VAP. Future studies could explore convenient and low-cost indexes of predicting VAP short-term mortality on the basis of the AG-related multivariate model.
Acknowledgments
Funding: This work was supported by
Footnote
Reporting Checklist: The authors have completed the STROBE reporting checklist. Available at https://jtd.amegroups.com/article/view/10.21037/jtd-23-1735/rc
Peer Review File: Available at https://jtd.amegroups.com/article/view/10.21037/jtd-23-1735/prf
Conflicts of Interest: Both authors have completed the ICMJE uniform disclosure form (available at https://jtd.amegroups.com/article/view/10.21037/jtd-23-1735/coif). The authors have no conflicts of interest to declare.
Ethical Statement: The authors are accountable for all aspects of the work in ensuring that questions related to the accuracy or integrity of any part of the work are appropriately investigated and resolved. The study was conducted in accordance with the Declaration of Helsinki (as revised in 2013). No ethical approval of our institutional review board was required since this survey data were publicly available.
Open Access Statement: This is an Open Access article distributed in accordance with the Creative Commons Attribution-NonCommercial-NoDerivs 4.0 International License (CC BY-NC-ND 4.0), which permits the non-commercial replication and distribution of the article with the strict proviso that no changes or edits are made and the original work is properly cited (including links to both the formal publication through the relevant DOI and the license). See: https://creativecommons.org/licenses/by-nc-nd/4.0/.
References
- Bassetti M, Mularoni A, Giacobbe DR, et al. New Antibiotics for Hospital-Acquired Pneumonia and Ventilator-Associated Pneumonia. Semin Respir Crit Care Med 2022;43:280-94. [Crossref] [PubMed]
- Plata-Menchaca EP, Ferrer R. Current treatment of nosocomial pneumonia and ventilator-associated pneumonia. Rev Esp Quimioter 2022;35:25-9. [Crossref] [PubMed]
- Sosa-Hernández O, Matías-Téllez B, Estrada-Hernández A, et al. Incidence and costs of ventilator-associated pneumonia in the adult intensive care unit of a tertiary referral hospital in Mexico. Am J Infect Control 2019;47:e21-5. [Crossref] [PubMed]
- Ramírez-Estrada S, Lagunes L, Peña-López Y, et al. Assessing predictive accuracy for outcomes of ventilator-associated events in an international cohort: the EUVAE study. Intensive Care Med 2018;44:1212-20. [Crossref] [PubMed]
- Quade BN, Parker MD, Occhipinti R. The therapeutic importance of acid-base balance. Biochem Pharmacol 2021;183:114278. [Crossref] [PubMed]
- Kraut JA, Nagami GT. The serum anion gap in the evaluation of acid-base disorders: what are its limitations and can its effectiveness be improved? Clin J Am Soc Nephrol 2013;8:2018-24. [Crossref] [PubMed]
- Chen Q, Chen Q, Li L, et al. Serum anion gap on admission predicts intensive care unit mortality in patients with aortic aneurysm. Exp Ther Med 2018;16:1766-77. [Crossref] [PubMed]
- Yang SW, Zhou YJ, Zhao YX, et al. The serum anion gap is associated with disease severity and all-cause mortality in coronary artery disease. J Geriatr Cardiol 2017;14:392-400. [PubMed]
- Mohr NM, Vakkalanka JP, Faine BA, et al. Serum anion gap predicts lactate poorly, but may be used to identify sepsis patients at risk for death: A cohort study. J Crit Care 2018;44:223-8. [Crossref] [PubMed]
- Abramowitz MK, Hostetter TH, Melamed ML. The serum anion gap is altered in early kidney disease and associates with mortality. Kidney Int 2012;82:701-9. [Crossref] [PubMed]
- Papadopoulos VP, Koutroulos MV, Zikoudi DG, et al. Diabetes-related acute metabolic emergencies in COVID-19 patients: a systematic review and meta-analysis. Diabetol Int 2021;12:445-59. [Crossref] [PubMed]
- Hellyer TP, McAuley DF, Walsh TS, et al. Biomarker-guided antibiotic stewardship in suspected ventilator-associated pneumonia (VAPrapid2): a randomised controlled trial and process evaluation. Lancet Respir Med 2020;8:182-91. [Crossref] [PubMed]
- Zhao Y, Zhou H, Tan W, et al. Prolonged dexmedetomidine infusion in critically ill adult patients: a retrospective analysis of a large clinical database Multiparameter Intelligent Monitoring in Intensive Care III. Ann Transl Med 2018;6:304. [Crossref] [PubMed]
- Johnson AE, Pollard TJ, Shen L, et al. MIMIC-III, a freely accessible critical care database. Sci Data 2016;3:160035. [Crossref] [PubMed]
- Liu Q, Yang J, Zhang J, et al. Description of Clinical Characteristics of VAP Patients in MIMIC Database. Front Pharmacol 2019;10:62. [Crossref] [PubMed]
- Allena N, Khanal S, Jog A, et al. Decoding the Chronic Obstructive Pulmonary Disease (COPD) Puzzle: Investigating the Significance of Exacerbation Scores in Triage Decision-Making. Cureus 2023;15:e41975. [Crossref] [PubMed]
- Aujesky D, Auble TE, Yealy DM, et al. Prospective comparison of three validated prediction rules for prognosis in community-acquired pneumonia. Am J Med 2005;118:384-92. [Crossref] [PubMed]
- Moreno R, Rhodes A, Piquilloud L, et al. The Sequential Organ Failure Assessment (SOFA) Score: has the time come for an update? Crit Care 2023;27:15. [Crossref] [PubMed]
- Godeau D, Petit A, Richard I, et al. Return-to-work, disabilities and occupational health in the age of COVID-19. Scand J Work Environ Health 2021;47:408-9. [Crossref] [PubMed]
- Zheng CM, Liu WC, Zheng JQ, et al. Metabolic acidosis and strong ion gap in critically ill patients with acute kidney injury. Biomed Res Int 2014;2014:819528. [Crossref] [PubMed]
- Liu X, Feng Y, Zhu X, et al. Serum anion gap at admission predicts all-cause mortality in critically ill patients with cerebral infarction: evidence from the MIMIC-III database. Biomarkers 2020;25:725-32. [Crossref] [PubMed]
- Bezuidenhout MC, Wiese OJ, Moodley D, et al. Correlating arterial blood gas, acid-base and blood pressure abnormalities with outcomes in COVID-19 intensive care patients. Ann Clin Biochem 2021;58:95-101. [Crossref] [PubMed]
- Dou L, Sallée M, Cerini C, et al. The cardiovascular effect of the uremic solute indole-3 acetic acid. J Am Soc Nephrol 2015;26:876-87. [Crossref] [PubMed]
- Nakano T, Katsuki S, Chen M, et al. Uremic Toxin Indoxyl Sulfate Promotes Proinflammatory Macrophage Activation Via the Interplay of OATP2B1 and Dll4-Notch Signaling. Circulation 2019;139:78-96. [Crossref] [PubMed]
- Li Z, Wu Z, Yan J, et al. Gut microbe-derived metabolite trimethylamine N-oxide induces cardiac hypertrophy and fibrosis. Lab Invest 2019;99:346-57. [Crossref] [PubMed]
- Shen J, Li DL, Yang ZS, et al. Anion gap predicts the long-term neurological and cognitive outcomes of spontaneous intracerebral hemorrhage. Eur Rev Med Pharmacol Sci 2022;26:3230-6. [PubMed]
- Keneally RJ, Peterson TJ, Benjamin JR, et al. Making Ventilator Associated Pneumonia Rate a Meaningful Quality Marker. J Intensive Care Med 2021;36:1354-60. [Crossref] [PubMed]
- Johnstone J, Meade M, Lauzier F, et al. Effect of Probiotics on Incident Ventilator-Associated Pneumonia in Critically Ill Patients: A Randomized Clinical Trial. JAMA 2021;326:1024-33. [Crossref] [PubMed]
- López-de-Andrés A, Albaladejo-Vicente R, de Miguel-Diez J, et al. Gender differences in incidence and in-hospital outcomes of community-acquired, ventilator-associated and nonventilator hospital-acquired pneumonia in Spain. Int J Clin Pract 2021;75:e13762. [Crossref] [PubMed]
- Lamarche D, Johnstone J, Zytaruk N, et al. Microbial dysbiosis and mortality during mechanical ventilation: a prospective observational study. Respir Res 2018;19:245. [Crossref] [PubMed]
- Akhter S, Warraich UA, Ghazal S, et al. Assessment and comparison of APACHE II (Acute Physiology and Chronic Health Evaluation), SOFA (Sequential Organ Failure Assessment) score and CURB 65 (Confusion; Urea; Respiratory Rate; Blood Pressure), for prediction of inpatient mortality in Acute Exacerbation of Chronic Obstructive Pulmonary Disease. J Pak Med Assoc 2019;69:211-5. [PubMed]
- Önal U, Aydın Güçlü Ö, Akalın H, et al. Prognostic factors for COVID-19 patients. J Infect Dev Ctries 2022;16:409-17. [Crossref] [PubMed]
- Sun T, Cai C, Shen H, et al. Anion Gap Was Associated with Inhospital Mortality and Adverse Clinical Outcomes of Coronary Care Unit Patients. Biomed Res Int 2020;2020:4598462. [Crossref] [PubMed]
- Özvatan T, Akalın H, Sınırtaş M, et al. Nosocomial Acinetobacter pneumonia: Treatment and prognostic factors in 356 cases. Respirology 2016;21:363-9. [Crossref] [PubMed]
- Oliva A, Borrazzo C, Mascellino MT, et al. CURB-65 plus hypoalbuminemia: a new score system for prediction of the in-hospital mortality risk in patients with SARS-CoV-2 pneumonia. Infez Med 2021;29:408-15. [Crossref] [PubMed]