Differentiating inflammatory and malignant pulmonary lesions on 3T lung MRI with radiomics of apparent diffusion coefficient maps and T2w derived radiomic feature maps
Highlight box
Key findings
• Radiomic features derived from 3 Tesla (3T) lung magnetic resonance imaging (MRI) allow noninvasive differentiation of inflammatory and malignant lung lesions with outstanding diagnostic performance.
What is known and what is new?
• Image-based differentiation of pneumonia and lung neoplasms can be challenging.
• Radiomics extracted from apparent diffusion coefficient (ADC) maps and T2 feature maps show significant differences and can help distinguish inflammatory and malignant lung lesions.
What is the implication, and what should change now?
• 3T lung MRI radiomics from ADC maps and T2 feature maps are straightforward to extract and could serve as a decision-making tool in clinical care.
Introduction
Since its emergence in the early 1980s, high-resolution computed tomography (HRCT) has become the method of choice for the diagnostic work-up of pulmonary parenchymal abnormalities (1,2). Free from the potentially harmful effects of radiation, magnetic resonance imaging (MRI) of the lungs emerged as a preferred modality over computed tomography (CT) in pediatric imaging and pregnant women (3,4). Limitations of pulmonary MRI include motion artifacts, a small signal-to-noise ratio due to low proton density of the lung, and poor detection of ground-glass opacities (3,5,6). Though still underused, MRI offers advantages for the diagnosis of various conditions affecting the pulmonary parenchyma by providing information on both soft tissue morphology and functional properties (4,7): MRI is feasible and valuable in immunocompromised patients with pneumonia and suspected fungal infection (5,8), free-breathing ultrashort echo time sequences have been reported to be highly sensitive in detecting pulmonary nodules (9-12) and promising results have been reported for diffusion-weighted imaging (DWI) including prediction of lung cancer invasiveness, determination of tumor type, and discrimination of malignant and benign nodules (13-17). For example, Koo et al. investigated multiparametric contrast-enhanced lung MRI, including T1-, T2-, and DWI, and identified decisive parameters for characterization of pulmonary nodules and prediction of malignancy (18). For DWI, different diffusion-sensitizing gradients are available to create apparent diffusion coefficient (ADC) maps, which can help identify malignancy by quantifying the diffusion-related modification of signal intensity (19-21).
Another approach to the quantitative assessment of the intrinsic properties of biological tissues is what is known as radiomics (22,23). For the last 15 years, radiomic features invisible to the human eye have been extracted from radiological images attempting to identify imaging biomarkers in their patterns or develop models combining such features for prediction of tumor entities, survival, and other biological endpoints (23-29). For example, Wang et al. suggested a radiomics model to predict the epidermal growth factor receptor status in non-small cell lung cancer using features derived from T2- and diffusion-weighted MR images (30). Another group recently conducted a retrospective analysis of 71 lung cancer lesions to develop a radiomics model based on features derived from T2-weighted MR images to distinguish lung adenocarcinoma from squamous cell carcinoma with solid components >8 mm (31). Despite the growing number of studies, radiomic feature analysis has not yet entered clinical practice, primarily due to poor reproducibility (32). Parametric feature maps have been proposed as one option to improve reproducibility and to simplify the use of radiomics (33,34). With this technique, a stack of parametric maps, one for each feature, is calculated from the original image dataset using a PyRadiomics-based, pretested software tool developed by our group. The software decomposes the images into small volumes of interest (VOIs) with a fixed size and calculates feature quantities. The quantities are stored in a map (and reflected by brightness) with the same spatial information as in the original image (33,34). So far, the parametric map approach has been applied in phantom and liver MRI studies, but not in lung MRI (33,35).
We therefore conducted a study to investigate whether radiomics from ADC maps and T2w derived parametric feature maps generated from lung MRI datasets can help distinguish inflammatory from malignant pulmonary lesions. We present this article in accordance with the STARD reporting checklist (available at https://jtd.amegroups.com/article/view/10.21037/jtd-23-1456/rc).
Methods
Study population
The study was conducted in accordance with the Declaration of Helsinki (as revised in 2013). The study was approved by the Institutional Review Board of Charité – Universitätsmedizin Berlin (No. EA4/017/14) and informed consent was taken from all the patients. Patients with and without underlying oncological disease were enrolled randomly if they had an unclear pulmonary lesion (defined as a localized parenchymal abnormality) in a clinically indicated chest CT scan. The intended sample size was 50 patients to achieve an area under the curve (AUC) of at least 0.75 with an alpha of 0.05 and a power of 0.85. Patients with suspected pulmonary metastasis on the basis of the CT appearance and/or clinical history were not enrolled. All patients were treated at our hospital and received a clinically indicated CT scan of the chest before the MRI. Study participants underwent pulmonary MRI within a median of 2–3 days after initial CT. One patient was examined by initial MRI because of his young age and since the findings were suspicious, a CT scan followed to plan a transbronchial lung biopsy. This study used histopathology as the reference standard since this method most precisely characterizes the underlying lung pathology into inflammatory and malignant. The diagnosis of malignant lesions was based on pathological examination of tissue obtained by invasive biopsy, while a diagnosis of inflammatory lesions was based on microbiological pathogen detection from BAL (except for one case, in which the pathogen was detected in tracheobronchial secretions). Clinical information was available to the assessors of the reference standard. Biopsy documentation was used to identify the target lesion. When BAL was performed to confirm the diagnosis, the most extensive and best visible lesion was chosen as the target lesion. Exclusion criteria were (I) marked breathing artifacts in lung MRI, (II) no reliable delineation of the lesion on the ADC map, (III) no biopsy or BAL performed, (IV) unclear histopathological specimen, and (V) sarcoidosis. Although sarcoidosis can have an infectious cause, it was excluded because the clinical presentation and treatment differ from infectious pneumonia. A subset of the study population was included in earlier studies (8,36-38). Figure 1 shows the flow diagram of the study population, and patient characteristics are compiled in Table 1.
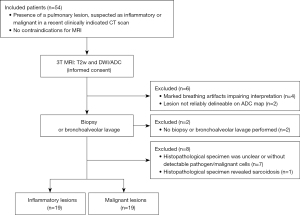
Table 1
Pulmonary lesion | Inflammatory | Malignant |
---|---|---|
Number of patients | 19 | 19 |
Median age [IQR] (years) | 59 [39–69] | 66 [54–76] |
Patient sex | ||
Female | 5 | 8 |
Male | 14 | 11 |
CT appearance of target lesion | ||
Solid | 5 | 9 |
Semisolid | 14 | 10 |
Ground-glass | 0 | 0 |
Biopsy | ||
CT-guided puncture | 1 (in addition to BAL and tracheobronchial secretions)* | 6 |
Wedge resection | – | 2 |
Transbronchial lung biopsy | – | 11 |
Pathogen sampling | ||
BAL | 19 | 1 (in addition to biopsy) |
Other | 1 patient: tracheobronchial secretions (in addition to BAL)* | – |
Pathogen | ||
Streptococcus pneumoniae | 1 | – |
Haemophilus influenzae | 2 | |
Aspergillus fumigatus | 8 | |
Aspergillus fumigatus/Varicella zoster virus | 1 | |
Candida albicans | 2 | |
Mycobacterium tuberculosis | 2 | |
Klebsiella oxytoca | 1 | |
Pseudomonas aeruginosa/Escherichia coli/ Staphylococcus aureus | 1* | |
Cryptogenic organizing pneumonia | 1 | |
Histopathological analysis of lung lesion | ||
Non-small cell lung cancer | – | 7 |
Non-Hodgkin lymphoma | 6 | |
Hodgkin lymphoma | 3 | |
Small-cell lung cancer | 1 | |
Germ cell tumor | 1 | |
Multiple myeloma | 1 | |
Primary/underlying disease | ||
AML | 6 | – |
Non-Hodgkin lymphoma | 5 | 6 |
ALL | 2 | – |
Aplastic anemia | 1 | – |
Hodgkin lymphoma | 1 | 3 |
Primary CNS lymphoma | 1 | – |
Gliosarcoma | 1 | – |
Squamous cell carcinoma head/neck | 1 | – |
Germ cell tumor | – | 1 |
Multiple myeloma | – | 1 |
Rectal cancer | – | 1*** |
Median interval between clinically indicated CT scan and study MRI (days) [IQR] | 2 [0.75–5.25] (1 patient underwent MRI prior to CT)** | 3 [1–6] |
Mean volume of interest size T2-weighted (mm3) | 13,252.79 | 17,606.45 |
Mean volume of interest size ADC (mm3) | 25,526.84 | 29,472.67 |
Mean diameter of target lesion**** (mm) [IQR] | 35.68 [22–52] | 56.05 [35–67] |
Mean number of lesions (≥3 mm diameter) per patient [IQR] | 8.94 [2–13.5]***** | 8.44 [1–12]***** |
*, one patient underwent a CT-guided biopsy that was negative for malignant cells. No pathogen was identified by microbiological analysis of the biopsy sample or the BAL. Analysis of tracheobronchial secretions revealed pathogens (P. aeruginosa, E. coli, S. aureus). **, one patient was not examined by CT before MRI. Due to the patient’s young age, MRI was performed first. Since MRI findings were suspicious, a clinically indicated CT scan followed afterwards. ***, biopsy of the pulmonary lesion revealed non-small cell lung cancer and not metastasis of rectal cancer. ****, on T2-weighted images. *****, one patient with multiple lesions. IQR, interquartile range; CT, computed tomography; BAL, bronchoalveolar lavage; AML, acute myeloid leukemia; ALL, acute lymphocytic leukemia; CNS, central nervous system; MRI, magnetic resonance imaging; ADC, apparent diffusion coefficient.
MRI examination and image acquisition
The MRI protocol included pulse sequences adapted from Biederer et al. and Attenberger et al. (5,39). No contrast medium was used. All MRI examinations were performed with the patient in supine position in the same 3 Tesla MRI scanner (Magnetom Skyra, Siemens Healthineers, Erlangen, Germany) using an 18-channel body phased-array coil positioned on the patient’s chest. The field of view (FOV) was adjusted to the patient’s size. Standard acquisition time was 53 seconds for the axial T2-weighted single-shot fast spin echo (FSE) sequences without fat saturation and 3 minutes and 8 seconds for the DWI sequences (b values of 50 and 800 mm2/s). A DWI sequence without motion correction designed by the vendor for thoracic imaging was used. T2w images were acquired in inspiration (multi-breath-hold, 2–3 acquisition steps, target breath hold time <20 s) and DWI/ADC during free breathing. ADC maps were automatically calculated by the scanner software. T2w images and ADC maps were retrieved from the picture archiving and communication system (PACS) in the DICOM format and pseudonymized. All MRI datasets used in the study were acquired from May 2014 to March 2021 using the same imaging protocol. Table 2 summarizes the scanning parameters of the two MRI sequences used in the study patients.
Table 2
Parameter | T2-weighted images | Diffusion-weighted images/apparent diffusion coefficient map |
---|---|---|
Target TR/TE (ms) | 500/28 | 6,800/56 |
Preset flip angle (degrees) | 121 | 90 |
In-plane phase-encoding direction | Columns (anterior to posterior) | Columns (anterior to posterior) |
Matrix | 320×208 | 134×100 |
Slice thickness (mm) | 5 | 5 |
Number of phase-encoding steps | 206 | 140 |
Breathing regimen | Multi-breath-hold (max. 20 s) | Free-breathing |
Scan time (s) | 53 | 188 |
Magnetic field strength | 3T | |
Patient position | Supine, head first |
MRI, magnetic resonance imaging; TR, repetition time; TE, echo time; T, Tesla.
Segmentation
Each target lesion was marked separately on the T2w images and ADC maps of each patient by placing three-dimensional VOIs using 3D slicer (3D Slicer, Version 4.10.0, www.slicer.org). The readers were required to delineate as much of the target lesion as possible while excluding larger airways and keeping a minimum inner distance of a few pixels from the edge to avoid voxels in the margin, where the lesion and adjacent tissue overlap. Each lesion was segmented by two radiologists: one was a board-certified radiologist with over 11 years of experience and the other a board-certified radiologist with 6 years of experience. If not otherwise stated, the reading of reader 1 was considered. The two readers had visual information on target lesion location but were blinded to the type of lesion. For lesion delineation in ADC maps, readers were allowed to view the corresponding T2w images.
Feature extraction from ADC maps
Features were conventionally extracted from VOIs in the ADC maps using PyRadiomics (Version 3.0) (40). Settings for extraction were adjusted as recommended by the PyRadiomics developers (see table available at https://cdn.amegroups.cn/static/public/jtd-23-1456-1.pdf). A total of 107 features were retrieved: 18 first-order features [energy, total energy, entropy, kurtosis, maximum, minimum, mean, median, interquartile range (IQR), skewness, range, mean absolute deviation (MAD), robust mean absolute deviation (RMAD), root mean squared (RMS), variance, uniformity, 10th percentile, and 90th percentile] and 75 second- and higher-order features [24 gray level co-occurrence matrix (GLCM) features, 14 gray level dependence matrix (GLDM) features, 16 gray level run-length matrix (GLRLM) features, 16 gray level size zone matrix (GLSZM) features, 5 neighboring gray tone difference matrix (NGTDM) features], and 14 shape features (40).
Calculation of parametric feature maps from T2w images and feature quantification
Parametric feature maps were computed from the T2w image stack of each patient using a PyRadiomics-based, pretested and published software tool developed by our group (33,34). The evaluation of the ADC and T2w images differs in that the ADC images are analyzed directly, whereas the T2w images are first converted into parametric maps. For the ADC images, the features are hence calculated directly from the respective VOI. In contrast, the T2w images are first converted into parametric maps, from which the values of the features within the VOI are retrieved in the next step. The parametric maps are generated by dissembling the original image into a grid of voxels (while voxel size can be modified in the software script to adjust the map’s resolution) and calculating feature quantity for each voxel. The feature quantity for each voxel, in turn, is reflected by brightness for each voxel, while spatial information is the same in the map as in the original image. Therefore, feature quantity can be directly retrieved from any location on the map. The rationale behind using the data differently is that ADC images are already calculated from different b-values and show functional information with low spatial resolution, whereas T2w images have greater anatomical detail and hence seem more suitable as a basis for parametric feature maps (34). Parametric feature maps should not be confused with T2 maps known, for instance, from cardiac MRI, since they do not contain any information on the T2 relaxation times of the examined tissues. After initial capacity analysis of the computing unit for spatial resolution of the feature maps, the voxel size was set to 5 mm (i.e., the software algorithm dissembled the original image into small VOIs of 5×5×5 mm, and the feature quantity was calculated from this small VOI and stored in the map in the same location as in the original image). Feature maps were calculated for the same 107 features as retrieved from the ADC maps. The same PyRadiomics configuration was used for the feature extraction from the ADC maps and the map generation from the T2w images (see table available at https://cdn.amegroups.cn/static/public/jtd-23-1456-1.pdf). VOIs were copied from each original T2w image stack to the corresponding 107 feature maps, and feature quantity was then directly extracted from each map using the mean. Figure 2 presents the processing of the T2w images and shows exemplary feature maps. The script we used is provided in the supplementary material (see table available at https://cdn.amegroups.cn/static/public/jtd-23-1456-2.pdf).
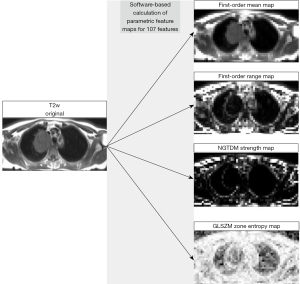
Statistical analysis
Statistical analysis was performed in R (version 4.2.1, R Foundation for Statistical Computing) (41). Due to the small study population, normal distribution was not assumed. Differences in radiomic feature quantities between the two groups (malignant versus inflammatory pulmonary lesions) were tested for statistical significance using the Mann-Whitney U (MWU)-test from the R base package. The diagnostic performance of the individual features was defined by receiver operating characteristic (ROC) curve analysis using the pROC package for R (42). The resulting AUCs were rated as follows: 70–80% acceptable, 80–90% excellent, and 90–100% outstanding performance (43). Interreader agreement was assessed by calculating intraclass correlation coefficients (ICCs) (ICC3 according to the Shrout and Fleiss convention) using the psych package for R (Version 2.2.5) (44,45). A P value <0.05 was generally considered to indicate statistical significance.
Results
Differentiation of inflammatory and malignant pulmonary lesions
ADC maps
The MWU test identified 58 features that differed significantly between inflammatory and malignant pulmonary lesions (P<0.05). Diagnostic performance ranged from 0.69 (GLCM maximum probability) to 0.94 (first-order root mean squared) in ROC analysis. Overall, AUCs of the decisive features were outstanding for 5 features, excellent for 27 features, acceptable for 25 features, and less than acceptable for one feature. Detailed results can be found in Table 3. Notably, features with outstanding diagnostic performance were first-order features, including simple mathematical features such as mean and median. AUCs for the outstanding features are shown in Figure 3. Exemplary boxplots of decisive features are shown in Figure 4. Figures 5,6 present examples of ADC maps. All results of the MWU test and ROC analysis for the ADC maps are provided in the supplementary material (see table available at https://cdn.amegroups.cn/static/public/jtd-23-1456-3.xlsx and https://cdn.amegroups.cn/static/public/jtd-23-1456-4.xlsx).
Table 3
Outstanding (AUC: 0.90–1.0) | Excellent (AUC: 0.80–0.90) | Acceptable (AUC: 0.70–0.80) | Below acceptable (AUC: <0.70) |
---|---|---|---|
First-order root mean squared (0.94) | First-order maximum (0.89) | GLRLM gray level variance (0.79) | GLCM maximum probability (0.69) |
First-order mean (0.93) | GLSZM size zone non uniformity normalized (0.89) | First-order variance (0.78) | |
First-order 90th percentile (0.93) | GLSZM small area emphasis (0.89) | GLDM gray level variance (0.78) | |
First-order median (0.93) | GLDM dependence non uniformity normalized (0.88) | GLSZM gray level variance (0.78) | |
First-order 10th percentile (0.92) | GLDM small dependence emphasis (0.88) | NGTDM contrast (0.78) | |
GLSZM zone percentage (0.88) | GLDM gray level non uniformity (0.78) | ||
GLDM idm (0.86) | GLRLM gray level non uniformity (0.78) | ||
GLRLM run length non uniformity normalized (0.88) | GLCM MCC (0.77) | ||
GLRLM short run emphasis (0.88) | GLSZM gray level non uniformity (0.76) | ||
GLRLM run variance (0.87) | GLCM difference variance (0.75) | ||
GLSZM large area emphasis (0.87) | GLCM cluster tendency (0.75) | ||
GLRLM long run emphasis (0.87) | First-order kurtosis (0.74) | ||
GLRLM run percentage (0.87) | GLCM cluster prominence (0.74) | ||
GLDM large dependence emphasis (0.86) | NGTDM busyness (0.73) | ||
GLCM inverse difference (0.84) | Shape surface volume ratio (0.73) | ||
GLSZM zone variance (0.86) | GLCM idmn (0.72) | ||
GLDM dependence variance (0.86) | GLCM idn (0.72) | ||
GLCM inverse variance (0.83) | GLCM joint entropy (0.72) | ||
NGTDM strength (0.83) | GLCM imc2 (0.72) | ||
First-order minimum (0.83) | NGTDM coarseness (0.72) | ||
First-order interquartile range (0.82) | GLCM joint energy (0.71) | ||
First-order robust mean absolute deviation (0.81) | Shape mesh volume (0.71) | ||
GLCM difference average (0.80) | Shape voxel volume (0.71) | ||
First-order mean absolute deviation (0.80) | GLRLM run length non uniformity (0.70) | ||
GLCM imc1 (0.80) | Shape minor axis length (0.70) | ||
GLCM sum squares (0.80) | |||
GLCM contrast (0.80) |
ADC, apparent diffusion coefficient; AUC, area under the curve; GLRLM, gray level run-length matrix; GLCM, gray level co-occurrence matrix; GLSZM, gray level size zone matrix; GLDM, gray level dependence matrix; NGTDM, neighboring gray tone difference matrix; MCC, maximal correlation coefficient.
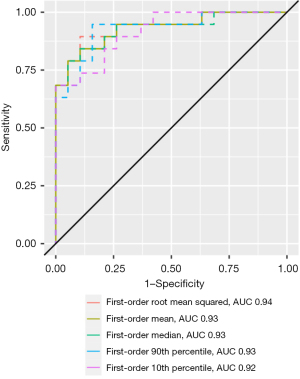
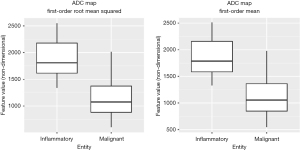
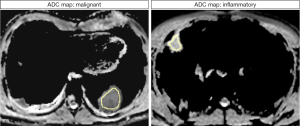
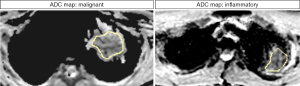
T2w derived feature maps
Sixty-seven of the T2w map-derived features significantly differed between inflammatory and malignant pulmonary lesions in the MWU test (P<0.05). Diagnostic performance ranged from acceptable to excellent, with excellent AUCs for 5 and acceptable AUCs for 62 features, as shown by the results compiled in Table 4. Except for first-order skewness, features with excellent performance are second- and higher-order features derived using complex mathematical equations. Still, the mathematics underlying skewness, the measure of the asymmetry of the distribution of values around the mean, is already very complex with X being a set of Np voxels included in the ROI (40):
Table 4
Outstanding (AUC: 0.90–1.0) | Excellent (AUC: 0.80–0.90) | Acceptable (AUC: 0.70–0.80) | Below acceptable (AUC: <0.70) |
---|---|---|---|
– | First-order skewness (0.86) | GLCM imc1 (0.79) | – |
GLDM gray level non uniformity (0.80) | GLSZM zone variance (0.79) | ||
GLSZM gray level non uniformity (0.80) | NGTDM busyness (0.79) | ||
GLCM MCC (0.80) | GLDM dependence non uniformity normalized (0.78) | ||
GLRLM gray level non uniformity (0.80) | First-order interquartile range (0.78) | ||
First-order mean absolute deviation (0.78) | |||
First-order robust mean absolute deviation (0.78) | |||
GLCM imc2 (0.78) | |||
GLRLM run variance (0.78) | |||
GLSZM size zone non uniformity normalized (0.78) | |||
GLCM inverse variance (0.78) | |||
GLDM large dependence high gray level emphasis (0.78) | |||
GLSZM large area high gray level emphasis (0.78) | |||
GLDM dependence variance (0.77) | |||
GLDM high gray level emphasis (0.77) | |||
GLDM small dependence high gray level emphasis (0.77) | |||
GLRLM long run emphasis (0.77) | |||
GLRLM run percentage (0.77) | |||
GLSZM large area emphasis (0.77) | |||
GLSZM small area emphasis (0.77) | |||
GLSZM zone percentage (0.77) | |||
NGTDM contrast (0.77) | |||
GLCM inverse difference (0.77) | |||
GLCM idm (0.77) | |||
GLCM joint average (0.77) | |||
GLCM sum average (0.77) | |||
GLDM large dependence emphasis (0.77) | |||
GLDM small dependence emphasis (0.77) | |||
GLRLM run length non uniformity normalized (0.77) | |||
GLRLM short run emphasis (0.77) | |||
GLRLM short run high gray level emphasis (0.77) | |||
GLCM autocorrelation (0.77) | |||
GLRLM high gray level run emphasis (0.77) | |||
GLRLM long run high gray level emphasis (0.77) | |||
GLSZM high gray level zone emphasis (0.77) | |||
GLCM cluster tendency (0.76) | |||
GLCM sum squares (0.76) | |||
NGTDM strength (0.76) | |||
First-order range (0.76) | |||
First-order variance (0.76) | |||
GLDM gray level variance (0.76) | |||
GLRLM gray level variance (0.76) | |||
GLSZM gray level variance (0.76) | |||
GLSZM small area high gray level emphasis (0.76) | |||
NGTDM complexity (0.76) | |||
GLCM difference average (0.76) | |||
GLSZM large area low gray level emphasis (0.75) | |||
GLCM contrast (0.75) | |||
GLCM difference variance (0.75) | |||
GLCM cluster shade (0.75) | |||
GLCM cluster prominence (0.74) | |||
First-order uniformity (0.74) | |||
GLRLM gray level non uniformity normalized (0.74) | |||
GLSZM gray level non uniformity normalized (0.74) | |||
GLDM large dependence low gray level emphasis (0.73) | |||
GLCM difference entropy (0.73) | |||
First-order entropy (0.73) | |||
GLRLM long run low gray level emphasis (0.72) | |||
GLSZM low gray level zone emphasis (0.71) | |||
GLDM low gray level emphasis (0.70) | |||
GLRLM short run low gray level emphasis (0.70) | |||
GLRLM low gray level run emphasis (0.70) |
T2w, T2-weighted; AUC, area under the curve; GLCM, gray level co-occurrence matrix; GLDM, gray level dependence matrix; GLSZM, gray level size zone matrix; GLRLM, gray level run-length matrix; NGTDM, neighboring gray tone difference matrix.
AUCs of the features with excellent diagnostic performance are shown in Figure 7. Figure 8 shows parametric feature maps of the T2w map-derived features with excellent AUCs. All results of the MWU test and ROC analysis for the T2w maps are provided in the supplementary material (see table available at https://cdn.amegroups.cn/static/public/jtd-23-1456-5.xlsx and https://cdn.amegroups.cn/static/public/jtd-23-1456-6.xlsx).
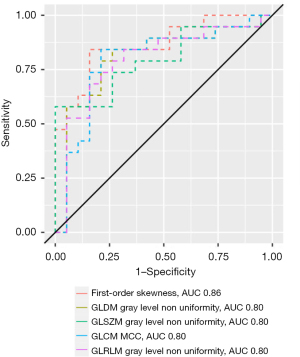
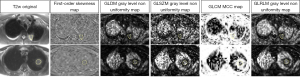
Interreader agreement
Interreader agreement was excellent for all features from ADC maps (all ICCs >0.9; P<0.001). ICCs for T2w map-derived features were excellent for all 93 features (all >0.9; P<0.001), excluding the 14 shape features, since the numerical values of these features were not usable due to the method used for calculating the maps (disassembly of the image into small voxels eliminating the properties of the shape features). Numerical values of the ICCs for ADC and T2w map-derived features are provided in the supplementary material (see table available at https://cdn.amegroups.cn/static/public/jtd-23-1456-7.xlsx and https://cdn.amegroups.cn/static/public/jtd-23-1456-8.xlsx).
Discussion
The present study has shown that differentiating inflammatory from malignant lung lesions is possible using radiomic features from ADC maps and by retrieving feature quantities from T2w derived feature maps. While the diagnostic performance of ADC-derived features is superior to that from T2w derived maps (outstanding for ADC versus excellent for T2w), this is accomplished at the cost of a much longer acquisition time for DWI/ADC datasets. Yet, the powerful features extracted from ADC maps are basic and all represent brightness, what already allows their measurement using a standard image viewer in the routine clinical setting. Altogether, results are promising in that ADC maps and T2w derived feature maps can enhance decision making regarding further patient management and might also be helpful if biopsy is unsuccessful or refused (e.g., to start empirical antibiotic/antifungal treatment of inflammatory lesions).
While the ADC maps were directly calculated by the scanner software, we used our software tool to compute the feature maps after DICOM export of the T2w datasets (34). Our rationale behind the computation of T2 maps was (I) to compensate for differences in volume of interest sizes of target lesions, as shown in previous studies (33,35), and (II) to gain transparency of feature behavior and control of the data (while otherwise, calculation of feature quantities is more or less a black box) (33). Computation of parametric maps thus is an attempt to overcome the lack of reproducibility of radiomic features across different VOI sizes. Although technically feasible, we abstained from also calculating parametric maps of the ADC images, which already are calculated maps (20,21). Further processing them to obtain parametric maps would reduce transparency of results.
Differentiating inflammatory from malignant lung lesions continues to be a challenge in clinical routine, and invasive methods, like biopsy, are frequently needed. Prompt initiation of adequate treatment is crucial, particularly in immunocompromised patients (46). Therefore, a fast-track guide seems desirable. MRI is an emerging modality in lung imaging. Yet, motion artifacts caused by respiration and cardiac motion and a poor signal-to-noise ratio due to a low proton density of pulmonary tissue remain challenging (3). In line with this, we had to exclude four patients because of respiratory artifacts blurring the target lesion in ADC maps. Recent technical advances in MRI, such as non-Cartesian data sampling (e.g., PROPELLER imaging) and ultrashort TE sequences, have improved the quality of lung MRI, allowing evaluation of lung tissue and large airways (3). Radiomics analysis has the potential to further enhance the diagnostic performance of lung MRI (37).
Overcoming the ambiguity of lung nodules reflected by their overlapping imaging appearance has been a concern of many investigators (47). For example, Yang et al. developed a helpful novel statistical model combining radiomics and T2-based quantitative parameters derived from T2-fBLADE-TSE sequences to differentiate between malignant and benign pulmonary nodules based on their findings in 107 pulmonary nodules in 96 patients (48). Another study presented a radiomics model using contrast-enhanced axial T2-BLADE-TSE images to differentiate adenocarcinoma from squamous cell carcinoma with solid components >8 mm in 71 patients (31). In a study conducted to distinguish malignant from benign pulmonary nodules using multiparametric MR-derived radiomics in 68 patients, features even outperformed traditional imaging parameters such as ADC (49).
The fact that all ADC features with outstanding performance in our analysis also represent image brightness suggests that no true gain in information is obtained by radiomics analysis. Hence, as already outlined before, retrieving the mean value in a standard image viewer can already be helpful, since DWI has been shown to be valuable in differentiating benign and malignant pulmonary lesions in several studies (13,15,50). Nevertheless, there is no general consensus regarding a threshold below which ADC indicates malignancy. ADC values around 1,000×10−6 mm2/s are regarded as normal, and lower values indicate restricted diffusion, which may possibly correlate with the higher cellularity of cancer tissue (19,51). Durmaz et al., who reported promising results for ADC values in distinguishing benign from malignant cavitary lung lesions, found significantly lower ADC values for malignant compared with benign lung lesions with a wall thickness of ≥5 mm on an initial CT scan in a study population of 45 patients, which is consistent with our results (50).
In the present study, we calculated two-value ADC maps with b values 50 and 800 mm2/s. Wan et al. showed superior performance for ADC with diffusion coefficients of 0 and ≥500 compared to 0 and <500 in differentiating between benign and malignant solitary pulmonary lesions in a 3T MRI study on 89 patients comparing different two-value combinations from a total of 14 different b-values (15). In their study, they placed ROIs in solid lesion portions at the level of the largest transverse diameter whereas we segmented the entire lesion. Çakmak et al. conducted a 1.5T MRI study including 47 patients to discriminate between benign and malignant pulmonary lesions. They compared the diagnostic performance of the minimum ADC (0 and 600 mm2/s) and lesion-to-spinal cord signal intensity ratio (on DWI), showing superior diagnostic performance for ADC. Yet, in contrast to our study, they also did not segment the entire lesion to calculate the minimum ADC value but calculated the average of three ROIs per lesion (13).
Our study has some limitations. We investigated a rather small study population of 38 patients (19 with inflammatory and 19 with malignant pulmonary lesions). Further, the study population is rather heterogeneous since patients with and without a history of cancer were included. Patients were chosen carefully for inclusion (based on invasive diagnosis confirmation) to ensure the validity of our results, and inconclusive cases were excluded. Also, four patients had to be excluded due to breathing artifacts and two patients because the target lesion was not readily identified in the ADC map. A further limitation is that T2w images allowed more precise segmentation than ADC maps. Performing segmentation solely from ADC maps without other anatomical MR images would have been challenging. Overall, the stability of radiomics is known to be limited across different MR systems, field strengths, and vendors, impeding the introduction of MR radiomics into clinical care and restricting the generalizability of our results across centers and scanners (19,32,52).
Conclusions
In conclusion, 3T MRI allows noninvasive differentiation of inflammatory and malignant pulmonary lesions based on radiomic feature quantification with outstanding (ADC) and excellent (T2w derived feature maps) diagnostic performance. MRI could thus guide the further diagnostic workup and a timely initiation of the appropriate therapy.
Acknowledgments
The authors thank Bettina Herwig for language editing.
Funding: None.
Footnote
Reporting Checklist: The authors have completed the STARD reporting checklist. Available at https://jtd.amegroups.com/article/view/10.21037/jtd-23-1456/rc
Data Sharing Statement: Available at https://jtd.amegroups.com/article/view/10.21037/jtd-23-1456/dss
Peer Review File: Available at https://jtd.amegroups.com/article/view/10.21037/jtd-23-1456/prf
Conflicts of Interest: All authors have completed the ICMJE uniform disclosure form (available at https://jtd.amegroups.com/article/view/10.21037/jtd-23-1456/coif). B.H. is Grant recipient for the Department of Radiology outside the presented study. B.H. is Grant recipient from Abbott, AbbVie, Ablative Solutions, Accovion, Achogen Inc., Actelion Pharmaceuticals, ADIR, Aesculap, Agios Pharmaceuticals, INC., AGO, AIF: Arbeitsgemeinschaft industrieller Forschungsvereinigungen, AIO: Arbeitsgemeinschaft internistische Onkologie, Aktionsbündnis Partnersicherheit e.V., Alexion Pharmaceuticals, Amgen, AO Foundation, Aravive, Arena Pharmaceuticals, ARMO Biosciences, Inc., Array Biopharma Inc., Art photonics GmbH Berlin, ASAS, Ascelia Pharma AB, Ascendis, ASR Advanced sleep research, Astrellas, AstraZeneca, August Research OOF, Sofia, BG, BARD, Basiliea, Bayer Healthcare, Bayer Schering Pharma, Bayer Vital, BBraun, BerGenBioASA, Berlin-Brandenburger Centrum für regenerative Therapie (BCRT), Berliner Krebsgesellschaft, Biontech Mainz, BioNTech SE, Biotronik, Bioven, BMBF, BMS, Boehring Ingelheimer, Boston Biomedical Inc., Boston Scientific Medizintechnik GmbH, BRACCO Group, Brahms GmbH, Brainsgate, Bistol-Myers Squibb, Calithera Biosciences UK, Cantargia AB, Medicon Village, Cascadian Therapeutics, Inc., Celgene, CELLACT Pharma, Celldex Therapeutics, Cellestia Biotech AG CH, CeloNova BioSciences, Charité research organization GmbH, Chiltern, CLOVIS ONCOLOGY, INC., Covance, CRO Charité, CTI Ulm, CUBIST, CureVac AG, Tübingen, Curis, Daiichi Sankyo, Dartmouth College, Hanover, NH, USA, DC Devices, Inc. USA, Delcath Systems, Dermira Inc., Deutsche Krebshilfe, Deutsche Rheuma Liga, DZ – Deutsche Diabetes Forschungsgesellschaft e.V., Deutsches Zentrum für Luft- und Raumfahrt e.V., DFG, Dr. Falk Pharma GmbH, DSM Nutritional Products AG, Dt. Gesellschaft für muskuloskelettale Radiologie, Dt. Stiftung für Herzforschung, Dynavax, Aisai Ltd., European Knowledge Centre, Mosquito Way, Hatfield, Eli Lilly and Company Ltd., EORTC, Episurf Medical, Epizyme, Inc., Essex Pharma, EU Programmes, European society of gastrointestinal and abdominal radiology, Euroscreen S.A., F20 Biotech GmbH, Ferring Pharmaceuticals A/S, Fibrex Medical Inc., Focused Ultrasound Surgery Foundation, Fraunhofer Gesellschaft, GALA Therapeutics, US, Galena Biopharma, Galmed Research and Development Ltd., Ganymed, GBG Forschungs GmbH, GE, Gentech. Inc., Genmab A/S, Genzyme Europe B.V., GETNE (Grupo Espanol de Tumores Neuroendocrinos), Gilead Sciences, Inc., Glaxo Smith Kline, Glycotype GmbH Berlin, Goethe Uni Frankfurt, Guerbet, Guidant Europe NV, Halozyme, Hans-Böckler-Stiftung, Hewlett Packard GmbH, Holaira Inc., Horizon Therapeutics Ireland, ICON (CRO), Idera Pharmaceuticals, Inc., Ignyta, Inc., Immunomedics Inc., Immunocore, Inari Medical Europe GmbH Basel, Incyte, INC Research, Innate Pharma, InSightec Ltd., Inspiremd, InVentiv Health Clinical UK Ltd., Inventivhealth, IO Biotech ApS Copenhagen, IOMEDICO, IONIS, IPSEN Pharma, IQVIA ISA Therapeutics, Isis Pharmaceuticals Inc., ITM Solucin GmbH, Jansen-Cilag GmbH, Kantar Health GmbH (CRO), Kartos Therapeutics, Inc., Karyopharm Therapeutics, Inc., Kendle/MorphoSys AG, Kite Pharma, Kli Fo Berlin Mitte, Kura Oncology, Labcorb, La Roche, Land Berlin, Lilly GmbH, Lion Biotechnology, Lombard Medical, Loxo Oncology, Inc., LSK BioPartners, USA, Lundbeck GmbH, LUX Biosciences, LYSARC, MacroGenics, MagForce, MedImmune Inc., MedImmune Limited, Medpace, Medpace Germany GmbH (CRO), MedPass (CRO), Medtronic, Medtraveo GmbH, Merck, Merrimack Pharmaceuticals Inc., MeVis Medical Solutions AG, Millenium Pharmaceuticals Inc., Miltenyi Biomedicine GmbH, Bergisch Gladbach, miRagen Boukider, Mologen, Monika Kutzner Stiftung, MophoSys AG, MSD Sharp, Nektar Therapeutics, NeoVacs SA, Netzwerkverbund Radiologie, Neurocrine Biosciences Inc., US, Newlink Genetics Corporation, Nexus Oncology, NIH, NOGGO Berlin, Nord-Ostddeutsche Gesellschaft e.V., Novartis, Novocure, Nuvisan, Ockham oncology, Odonate Therapeutics San Diego, OHIRC Kanada, Oppilan Pharma ldt., London, Orion Corporation Orion Pharma, OSE Immunotherapeutics, Parexel CRO Service, Pentixal Pharma GmbH Perceptive, Pfizer GmbH, PharmaCept GmbH, Pharma Mar, Pharmaceutical Reseach Associates GmbH (PRA), Pharmacyclics Inc., Philipps, Philogen s.p.a. Siena, Pliant therapeutics San Francisco, PIQUR Therapeutics Ltd., Pluristem, PneuRX.Inc., Portola Pharmaceuticals, PPD (CRO), PRaint, Precision GmbH, Premier-research, Priovant Therapeutics USA, Provectus Biopharmaceuticals, Inc., psi-cro, Pulmonx International Sarl, Quintiles GmbH, Radiobotics ApS, Regeneraon Pharmaceuticals Inc., Replimune, Respicardia, Rhythm Pharmaceuticals, Inc. Boston USA, Roche, Salix Pharmaceuticals Inc., Samsung, Sanofi, sanofis-aventis S.A., Sarepta Therapeutics, Cambridge, US, Saving Patient’s Lives Medical B.V., Schumacher GmbH, Seagen, Seattle Genetics, Servier (CRO), SGS Life Science Sercives (CRO), Shape Memorial Midical Inc., USA, Shire Human Genetic Therapies, Siemens, Silena Therapeutics, SIRTEX Medical Europe GmbH, SOTIO Biotech, Boston, Spectranetics GmbH, Spectrum Pharmaceuticals, Stiftung Charite / BIH, St. Jude Medical, Stiftung Wolfgang Schulze, Syneos Health UK, Ltd., Symphogen, Taiho Oncology, Inc., Taiho Pharmaceutical Co., Target Pharma Solutions Inc., TauRx Therapeutics Ltd., Terumo Medical Corporation, Tesaro, tetec-ag, TEVA, Theorem, Theradex, Theravance, Threshold Pharmaceuticals Inc., TNS Healthcare GmbH, Toshiba, UCB Pharma, Ulrich GmbH Ulm, Uni Jena, Uni München, Uni Tübingen, Vaccibody A.S., VDI/VDE, Vertex Pharmaceuticals Incorporated, Viridian Therapeutics, US, Virtualscopis LLC, Winicker-norimed, Wyeth Pharma, Xcovery Holding Company, Zukunftsfond Berlin (TSB) for the Department of Radiology. The other authors have no conflicts of interest to declare.
Ethical Statement: The authors are accountable for all aspects of the work in ensuring that questions related to the accuracy or integrity of any part of the work are appropriately investigated and resolved. The study was conducted in accordance with the Declaration of Helsinki (as revised in 2013). The study was approved by the Institutional Review Board of Charité – Universitätsmedizin Berlin (No. EA4/017/14) and informed consent was taken from all the patients.
Open Access Statement: This is an Open Access article distributed in accordance with the Creative Commons Attribution-NonCommercial-NoDerivs 4.0 International License (CC BY-NC-ND 4.0), which permits the non-commercial replication and distribution of the article with the strict proviso that no changes or edits are made and the original work is properly cited (including links to both the formal publication through the relevant DOI and the license). See: https://creativecommons.org/licenses/by-nc-nd/4.0/.
References
- Kazerooni EA. High-resolution CT of the lungs. AJR Am J Roentgenol 2001;177:501-19. [Crossref] [PubMed]
- Nathan SD, Pastre J, Ksovreli I, et al. HRCT evaluation of patients with interstitial lung disease: comparison of the 2018 and 2011 diagnostic guidelines. Ther Adv Respir Dis 2020;14:1753466620968496. [Crossref] [PubMed]
- Liszewski MC, Ciet P, Winant AJ, et al. Magnetic Resonance Imaging of Pediatric Lungs and Airways: New Paradigm for Practical Daily Clinical Use. J Thorac Imaging 2024;39:57-66. [Crossref] [PubMed]
- Eibel R, Herzog P, Dietrich O, et al. Pulmonary abnormalities in immunocompromised patients: comparative detection with parallel acquisition MR imaging and thin-section helical CT. Radiology 2006;241:880-91. [Crossref] [PubMed]
- Attenberger UI, Morelli JN, Henzler T, et al. 3 Tesla proton MRI for the diagnosis of pneumonia/lung infiltrates in neutropenic patients with acute myeloid leukemia: initial results in comparison to HRCT. Eur J Radiol 2014;83:e61-6. [Crossref] [PubMed]
- Azour L, Condos R, Keerthivasan MB, et al. Low-field 0.55 T MRI for assessment of pulmonary groundglass and fibrosis-like opacities: Inter-reader and inter-modality concordance. Eur J Radiol 2022;156:110515. [Crossref] [PubMed]
- Ohno Y, Koyama H, Yoshikawa T, et al. Pulmonary high-resolution ultrashort TE MR imaging: Comparison with thin-section standard- and low-dose computed tomography for the assessment of pulmonary parenchyma diseases. J Magn Reson Imaging 2016;43:512-32. [Crossref] [PubMed]
- Nagel SN, Wyschkon S, Schwartz S, et al. Can magnetic resonance imaging be an alternative to computed tomography in immunocompromised patients with suspected fungal infections? Feasibility of a speed optimized examination protocol at 3 Tesla. Eur J Radiol 2016;85:857-63. [Crossref] [PubMed]
- Burris NS, Johnson KM, Larson PE, et al. Detection of Small Pulmonary Nodules with Ultrashort Echo Time Sequences in Oncology Patients by Using a PET/MR System. Radiology 2016;278:239-46. [Crossref] [PubMed]
- Wang F, Lin X, Lin C, et al. Ability of three-dimensional 3-Tesla ultrashort echo time magnetic resonance imaging to display the morphological characteristics of pulmonary nodules: a sensitivity analysis. Quant Imaging Med Surg 2023;13:1792-801. [Crossref] [PubMed]
- Wielpütz MO, Lee HY, Koyama H, et al. Morphologic Characterization of Pulmonary Nodules With Ultrashort TE MRI at 3T. AJR Am J Roentgenol 2018;210:1216-25. [Crossref] [PubMed]
- Yang S, Shan F, Shi Y, et al. Sensitivity and specificity of magnetic resonance imaging in routine diagnosis of pulmonary lesions: a comparison with computed tomography. J Thorac Dis 2022;14:3762-72. [Crossref] [PubMed]
- Çakmak V, Ufuk F, Karabulut N. Diffusion-weighted MRI of pulmonary lesions: Comparison of apparent diffusion coefficient and lesion-to-spinal cord signal intensity ratio in lesion characterization. J Magn Reson Imaging 2017;45:845-54. [Crossref] [PubMed]
- Kanauchi N, Oizumi H, Honma T, et al. Role of diffusion-weighted magnetic resonance imaging for predicting of tumor invasiveness for clinical stage IA non-small cell lung cancer. Eur J Cardiothorac Surg 2009;35:706-10; discussion 710-1. [Crossref] [PubMed]
- Wan Q, Deng YS, Lei Q, et al. Differentiating between malignant and benign solid solitary pulmonary lesions: are intravoxel incoherent motion and diffusion kurtosis imaging superior to conventional diffusion-weighted imaging? Eur Radiol 2019;29:1607-15. [Crossref] [PubMed]
- Liu J, Xia X, Zou Q, et al. Diagnostic performance of diffusion-weighted imaging versus 18F-FDG PET/CT in differentiating pulmonary lesions: an updated meta-analysis of comparative studies. BMC Med Imaging 2023;23:37. [Crossref] [PubMed]
- Li G, Huang R, Zhu M, et al. Native T1-mapping and diffusion-weighted imaging (DWI) can be used to identify lung cancer pathological types and their correlation with Ki-67 expression. J Thorac Dis 2022;14:443-54. [Crossref] [PubMed]
- Koo CW, Lu A, Takahashi EA, et al. Can MRI contribute to pulmonary nodule analysis? J Magn Reson Imaging 2019;49:e256-64. [Crossref] [PubMed]
- Peerlings J, Woodruff HC, Winfield JM, et al. Stability of radiomics features in apparent diffusion coefficient maps from a multi-centre test-retest trial. Sci Rep 2019;9:4800. [Crossref] [PubMed]
- Padhani AR, Liu G, Koh DM, et al. Diffusion-weighted magnetic resonance imaging as a cancer biomarker: consensus and recommendations. Neoplasia 2009;11:102-25. [Crossref] [PubMed]
- Koh DM, Collins DJ. Diffusion-weighted MRI in the body: applications and challenges in oncology. AJR Am J Roentgenol 2007;188:1622-35. [Crossref] [PubMed]
- Fritz B, Yi PH, Kijowski R, et al. Radiomics and Deep Learning for Disease Detection in Musculoskeletal Radiology: An Overview of Novel MRI- and CT-Based Approaches. Invest Radiol 2023;58:3-13. [Crossref] [PubMed]
- Pinto Dos Santos D. A meta-discussion on radiomics - Meta-research, bias, quality and other issues. Eur J Radiol 2023;166:111007. [Crossref] [PubMed]
- Haneberg AG, Pierre K, Winter-Reinhold E, et al. Introduction to Radiomics and Artificial Intelligence: A Primer for Radiologists. Semin Roentgenol 2023;58:152-7. [Crossref] [PubMed]
- Wong CW, Chaudhry A. Radiogenomics of lung cancer. J Thorac Dis 2020;12:5104-9. [Crossref] [PubMed]
- Wang Y, Chen H, Chen Y, et al. A semiautomated radiomics model based on multimodal dual-layer spectral CT for preoperative discrimination of the invasiveness of pulmonary ground-glass nodules. J Thorac Dis 2023;15:2505-16. [Crossref] [PubMed]
- Han X, Fan J, Zheng Y, et al. Value of radiomics in differentiating synchronous double primary lung adenocarcinomas from intrapulmonary metastasis. J Thorac Dis 2023;15:3685-98. [Crossref] [PubMed]
- Shi L, Yang M, Yao J, et al. Application of computed tomography-based radiomics combined with clinical factors in the diagnosis of malignant degree of lung adenocarcinoma. J Thorac Dis 2022;14:4435-48. [Crossref] [PubMed]
- Majumder S, Katz S, Kontos D, et al. State of the art: radiomics and radiomics-related artificial intelligence on the road to clinical translation. BJR Open 2024;6:tzad004. [Crossref] [PubMed]
- Wang Y, Wan Q, Xia X, et al. Value of radiomics model based on multi-parametric magnetic resonance imaging in predicting epidermal growth factor receptor mutation status in patients with lung adenocarcinoma. J Thorac Dis 2021;13:3497-508. [Crossref] [PubMed]
- Yang M, Shi L, Huang T, et al. Value of contrast-enhanced magnetic resonance imaging-T2WI-based radiomic features in distinguishing lung adenocarcinoma from lung squamous cell carcinoma with solid components >8 mm. J Thorac Dis 2023;15:635-48. [Crossref] [PubMed]
- Pinto Dos Santos D, Dietzel M, Baessler B. A decade of radiomics research: are images really data or just patterns in the noise? Eur Radiol 2021;31:1-4. [Crossref] [PubMed]
- Jensen LJ, Kim D, Elgeti T, et al. Enhancing the stability of CT radiomics across different volume of interest sizes using parametric feature maps: a phantom study. Eur Radiol Exp 2022;6:43. [Crossref] [PubMed]
- Kim D, Jensen LJ, Elgeti T, et al. Radiomics for Everyone: A New Tool Simplifies Creating Parametric Maps for the Visualization and Quantification of Radiomics Features. Tomography 2021;7:477-87. [Crossref] [PubMed]
- Jensen LJ, Kim D, Elgeti T, et al. The role of parametric feature maps to correct different volume of interest sizes: an in vivo liver MRI study. Eur Radiol Exp 2023;7:48. [Crossref] [PubMed]
- Kim D, Elgeti T, Penzkofer T, et al. Enhancing the differentiation of pulmonary lymphoma and fungal pneumonia in hematological patients using texture analysis in 3-T MRI. Eur Radiol 2021;31:695-705. [Crossref] [PubMed]
- Jensen LJ, Kim D, Elgeti T, et al. Differentiation of Pulmonary Lymphoma Manifestations and Nonlymphoma Infiltrates in Possible Invasive Fungal Disease Using Fast T1-weighted Magnetic Resonance Imaging at 3 T Comparison of Texture Analysis, Mapping, and Signal Intensity Quotients. J Thorac Imaging 2022;37:80-9. [Crossref] [PubMed]
- Nagel SN, Kim D, Penzkofer T, et al. Pulmonary MRI at 3T: Non-enhanced pulmonary magnetic resonance Imaging Characterization Quotients for differentiation of infectious and malignant lesions. Eur J Radiol 2017;89:33-9. [Crossref] [PubMed]
- Biederer J, Beer M, Hirsch W, et al. MRI of the lung (2/3). Why … when … how? Insights Imaging 2012;3:355-71. [Crossref] [PubMed]
- PyRadiomics community: Radiomic features (2016). Available online: https://pyradiomics.readthedocs.io/en/latest/features.html.
- R Development Core Team. R: A language and environment for statistical computing. Vienna, Austria: R Foundation for Statistical Computing; 2016.
- Robin X, Turck N, Hainard A, et al. pROC: an open-source package for R and S+ to analyze and compare ROC curves. BMC Bioinformatics 2011;12:77. [Crossref] [PubMed]
- Koo TK, Li MY. A Guideline of Selecting and Reporting Intraclass Correlation Coefficients for Reliability Research. J Chiropr Med 2016;15:155-63. [Crossref] [PubMed]
- Shrout PE, Fleiss JL. Intraclass correlations: uses in assessing rater reliability. Psychol Bull 1979;86:420-8. [Crossref] [PubMed]
- Revelle W. psych: Procedures for Psychological, Psychometric, and Personality Research. Northwestern University, Evanston, Illinois. R package version 2.2.9 (2022). Available online: https://CRAN.R-project.org/package=psych.
- Perea S, Patterson TF. Invasive Aspergillus infections in hematologic malignancy patients. Semin Respir Infect 2002;17:99-105. [Crossref] [PubMed]
- Fischer S, Spath N, Hamed M. Data-Driven Radiogenomic Approach for Deciphering Molecular Mechanisms Underlying Imaging Phenotypes in Lung Adenocarcinoma: A Pilot Study. Int J Mol Sci 2023;24:4947. [Crossref] [PubMed]
- Yang S, Wang Y, Shi Y, et al. Radiomics nomogram analysis of T2-fBLADE-TSE in pulmonary nodules evaluation. Magn Reson Imaging 2022;85:80-6. [Crossref] [PubMed]
- Koo CW, Kline TL, Yoon JH, et al. Magnetic resonance radiomic feature performance in pulmonary nodule classification and impact of segmentation variability on radiomics. Br J Radiol 2022;95:20220230. [Crossref] [PubMed]
- Durmaz F, Özgökçe M, Aydin Y, et al. The Efficiency of Diffusion-weighted Magnetic Resonance Imaging in the Differentiation of Malign and Benign Cavitary Lung Lesions. J Thorac Imaging 2023;38:154-8. [Crossref] [PubMed]
- deSouza NM, Winfield JM, Waterton JC, et al. Implementing diffusion-weighted MRI for body imaging in prospective multicentre trials: current considerations and future perspectives. Eur Radiol 2018;28:1118-31. [Crossref] [PubMed]
- Rai R, Holloway LC, Brink C, et al. Multicenter evaluation of MRI-based radiomic features: A phantom study. Med Phys 2020;47:3054-63. [Crossref] [PubMed]