Value of computed tomography radiomics combined with inflammation indices in predicting the efficacy of immunotherapy in patients with locally advanced and metastatic non-small cell lung cancer
Highlight box
Key findings
• Computed tomography radiomics combined with inflammation indices has a significant predictive value for whether durable clinical benefit (DCB) can be achieved in patients with locally advanced or metastatic non-small cell lung cancer (NSCLC) receiving immunotherapy patients.
What is known and what is new?
• Computed tomography radiomics can predict the benefit of immunotherapy. High systemic immune-inflammation index score was reported to be associated with a shorter progression-free survival in patients with NSCLC treated with immune checkpoint inhibitors.
• Radiomics score and peripheral blood inflammation indices are useful for creating a forecasting model which performed well in predicting whether patients with locally advanced or metastatic NSCLC can achieve DCB after receiving immunotherapy.
What is the implication, and what should change now?
• Further prospective studies about radiomics are required to validate the role radiomics plays in predicting the efficacy of immunotherapy in patients with NSCLC. It follows that we can create a more valuable forecasting model including radiomics and inflammation indices to help us decide whether patients should be treated by immunotherapy. To our best knowledge, it is the first such attempt.
Introduction
Lung cancer is the most common cancer and remains the leading cause of cancer-related death worldwide (1). It is an insidious malignancy that usually does not produce symptoms in its early stages. As a result, 40–57% of patients with lung cancer have already developed distant metastases at the time of diagnosis, leading to a 5-year survival rate of less than 10% (2,3). Non-small cell lung cancer (NSCLC) is the most prevalent subtype of lung cancer, accounting for approximately 85% of all cases (4). immune checkpoint inhibitors (ICIs) can activate T-cell-mediated antitumor immune response and thus exert an antitumor effect (5). Among them, antibodies targeting programmed cell death protein-1 (PD-1) or its ligand programmed death ligand-1 (PD-L1) have been approved for the treatment of a variety of advanced cancers, changing the landscape of treatment for patients with lung cancer. Several studies have shown that ICI-based immunotherapy is superior to chemotherapy and can dramatically improve the prognosis of patients with lung cancer. For example, the 5-year follow-up results of the KEYNOTE-042 trial revealed that pembrolizumab monotherapy was more efficacious than chemotherapy, had a lower incidence of adverse events, and could be used as a first-line treatment for patients with PD-L1-positive locally advanced or metastatic NSCLC (6). The 5-year follow-up results of the CA209-003 trial showed that patients with advanced NSCLC who had previously received other treatments had a 5-year overall survival (OS) rate of 16% after nivolumab treatment, with some patients achieving long-lasting responses (7).
Although immunotherapy is superior to the conventional chemotherapy, delayed response may occur during the treatment (8); however, this is only observed in 20% of patients. Meanwhile, a very rare but clinically significant subset of NSCLC patients exhibit a phenomenon known as hyperprogression has a significant impact on patient prognosis and influences the patients’ choice of treatment. Therefore, it is crucial to carefully select patients before beginning treatment. Although PD-L1 expression and tumor mutational burden (TMB) are widely recognized as good predictive biomarkers of immunotherapy response (9-14). Although TMB has not gained widespread clinical use due to its limited and controversial predictive value, PD-L1 have been applied in some clinical settings. there are still a number of factors impacting their clinical application. Firstly, there are differences in the determination methods and scoring criteria for PD-L1 expression and TMB levels. Secondly, intratumoral heterogeneity, immune microenvironment, and other factors can also affect PD-L1 expression level (15-19). Finally, although patients with PD-L1 expression or high TMB tend to respond better to immunotherapy, there are still cases where patients with no PD-L1 expression or low TMB still respond to ICI-based immunotherapy (20,21). This suggests that there is pressing need to identify noninvasive indicators that are more efficient in predicting early immunotherapy efficacy in patients with NSCLC. Computed tomography (CT) radiomics can extract radiomic features including shape, intensity, and texture features by depicting the phenotype of lung tumors and quantifying them. Algorithms can be used to analyze these quantified radiomics features. These features can further be used to construct models for, among other things, disease diagnosis and prognosis analysis. Imaging histology can assess the shape and heterogeneity of tumors by analyzing their shape features, intensity features, and texture features, most of which cannot be recognized by the human eye. The advantage of imaging histology is that it can provide a more comprehensive understanding of the entire tumor by reflecting not only the visible features of the tissue, but also the cellular and molecular properties of the tissue through non-invasive examination. Quantitative imaging histology features have been shown to provide rapid and accurate non-invasive biomarkers for lung cancer risk prediction, diagnosis, prognosis, treatment response monitoring and tumor biology (22). In patients with NSCLC, these models have demonstrated good performance in predicting lung cancer gene mutations (23,24) and PD-L1 expression (25), distinguishing between primary and metastatic lung tumors (26), and predicting the benefit of immunotherapy (27-29).
Inflammatory cells and mediators are important components of the tumor microenvironment (30) and are associated with the prognosis of various tumor types. Research has shown that a higher neutrophil-to-lymphocyte ratio (NLR) and platelet-to-lymphocyte ratio (PLR) are correlated with poor immunotherapy outcomes in patients with NSCLC (31-35), suggesting their potential use as prognostic markers. The systemic immune-inflammation index (SII), an inflammatory marker that combines NLR and platelet count was found to be an independent risk factor for solid cancers (36). Moreover, a high SII score was reported to be associated with a shorter progression-free survival (PFS) in patients with NSCLC treated with ICIs (37,38).
Immunotherapy has not been widely used to treat lung cancer, which limits the data on follow-up time and the availability of radiomics studies in predicting its durable clinical benefit (DCB). In this study, we retrospectively collected the data of patients receiving immunotherapy with anti-PD-1/PD-L1 monoclonal antibodies, established a radiomics model by extracting and screening for the CT radiomic features of these patients, and calculated the radiomic scores. In addition, by analyzing the patients’ clinical data and peripheral blood characteristics, we selected indices with predictive value to establish and validate a comprehensive forecasting model (CFM) to predict whether patients with NSCLC could obtain DCB after receiving immunotherapy. We present this article in accordance with the TRIPOD reporting checklist (available at https://jtd.amegroups.com/article/view/10.21037/jtd-24-526/rc).
Methods
Participants
The baseline data and CT images of patients with pathologically confirmed stage IIIa/IVb NSCLC treated with anti-PD-1/PD-L1 monoclonal antibodies at the First Affiliated Hospital of Soochow University from December 2019 to January 2023 were retrospectively collected. The patients were followed up until June 2023. The requirement for signed informed consent was waived since the data analyzed were anonymous and did not involve patient privacy. This study fully complied with the Declaration of Helsinki (2013 version) and was approved by the Ethics Committee of the First Affiliated Hospital of Soochow University (approval No. 2023540).
The inclusion criteria were as follows: (I) patients with stage IIIa/IVb NSCLC diagnosed according to the eighth edition of the Tumor Node Metastasis (TNM) staging system; (II) patients receiving anti-PD-1/PD-L1 monotherapy or immunotherapy in combination with conventional chemotherapy; (III) availability of CT images with a slice thickness of 1.25 mm obtained within 2 months prior to the initiation of immunotherapy; and (IV) routine blood tests and testing for serum tumor markers completed within 1 week prior to the treatment with anti-PD-1/PD-L1 monoclonal antibodies. The exclusion criteria were as follows: (I) inability to detect and segment the primary tumor on chest CT image or poor image quality; (II) other treatments in addition to immunotherapy or chemotherapy; (III) presence of autoimmune diseases; and (IV) detection of pneumonia or infections at other sites unrelated to the tumor. In total, 82 patients were enrolled in this study. According to the study design for prediction modeling, 57 (69.51%) of these patients were randomized as the training set, and the remaining 25 (30.49%) patients as the validation set.
Data collection
The baseline data collected included age at diagnosis, gender, smoking history, Eastern Cooperative Oncology Group (ECOG) performance status (PS) score, histological types of NSCLC, PD-L1 expression level, tumor stage, pretreatment CT data, results of routine blood tests, serum tumor markers, treatment regimens, and results of routine blood tests after two cycles of immunotherapy. Inflammation indices including SII (SII = platelet count × neutrophil count/lymphocyte count), NLR (NLR = neutrophil count/lymphocyte count), and PLR (PLR = platelet count/lymphocyte count) were calculated.
Response evaluation and study endpoints
Responses to treatment were assessed based on the [Response Evaluation Criteria in Solid Tumors (RECIST) v. 1.1] and were classified as progressive disease (PD), stable disease (SD), partial response (PR), and complete response (CR) based on the clinical data and imaging findings in the electronic medical records. The primary endpoint of this study was PFS, defined as the time from initiating immunotherapy to disease progression, death from any cause, or final follow-up visit. Patients were divided into two groups based on whether their PFS exceeded 12 months, with a PFS greater than 12 months considered to indicate DCB. The secondary endpoint was OS, defined as the time from initiation of immunotherapy to death from any cause or last follow-up visit.
Image acquisition and extraction of radiomics features
CT images obtained within 2 months using Toshiba, Philips, or Siemens CT scanners prior to treatment were retrieved from a picture archiving and communication system (PACS). The default scanner settings encompassed a tube voltage of 120 kV and a tube current ranging from 110 to 240 mA. Following the activation of the real-time dynamic dose mapper, the key parameters were set as follows: collimation at 0.6 mm × 128 mm, rotation time of 0.25 seconds, pitch factor of 0.9, and a slice thickness of 5 mm. The patient was positioned supine, with their upper limbs raised naturally. The head was advanced initially, and a standard chest scan was performed at the culmination of a deep inspiration. The scanning area extended from the thoracic inlet to a point 5 cm below the costophrenic angle. All CT images were evaluated using both the lung window (with a window width of 1,500 Hounsfield units (HU) and a window level of –500 HU) and the mediastinal window (window width of 400 HU and window level of 45 HU). The reconstructed slice thickness was set at 1.25 mm. An open-source radiomics software (3D Slicer) v. 5.4.0 was used to delineate the region of interest (ROI) and extract the radiomic features. The chest CT image data were imported into 3D Slicer, and a lung window was applied (window width, 1,500 HU; window level, –500 HU). The primary tumor was selected for target delineation, and the largest tumor was delineated if the primary tumor was not identified. After delineation, a three-dimensional (3D) ROI image of the lung cancer was generated. The radiomics features were extracted by using the SlicerRadiomics, which is an extension for 3D Slicer. Texture features were calculated using a bin width of 25 HU. The voxel size after resampling was 1 mm × 1 mm × 1 mm, and wavelet-based features were extracted. A total of 851 radiomics features were extracted from the 3D ROI images of lung cancer, including first-order statistics, shape features, texture features, and higher-order statistics. The texture features included gray-level co-occurrence matrix (GLCM), gray-level dependence matrix (GLDM), gray-level run-length matrix (GLRLM), gray-level size-zone matrix (GLSZM), and neighboring gray-tone difference matrix (NGTDM). Through wavelet filtering, high- (H) or low (L)-pass filters were applied to decompose the images in each of the three dimensions, which yielded eight decompositions including HHH, LLL, HHL, HLL, LHH, LHL, LLH, HLH. Higher-order statistics were acquired by wavelet transform on images.
ROIs were jointly delineated by two investigators (H.S. and L.S.) to increase the reproducibility. Each investigator delineated the same tumor twice at different time points, and the consistencies of the extracted radiomic features were tested by using the intragroup correlation coefficient (ICC). After the intra- and intergroup ICCs were calculated, features with ICCs >0.8 at both time points were selected. Any disagreement was resolved through discussion between these two investigators.
Model establishment
Calculation of radiomics scores (Rad-scores)
For the radiomics features extracted in the training set, the least absolute shrinkage and selection operator (LASSO) algorithm was used to screen for radiomics features and obtain the feature values after 10-fold cross-validation, thus obtaining the Rad-score of each patient. The area under the receiver operating characteristic (ROC) curve (AUC) was used to evaluate the predictive performance of Rad-score in both the training set and validation set.
Construction and evaluation of the CFM
Univariate analyses with a t-test, chi-squared test, or Mann-Whitney test were performed to screen for variables, with significant differences in clinical baseline data and/or hematological characteristics. Multivariate binary logistic regression analyses were then applied to screen for the independent predictors of the population with DCB among patients treated with ICIs. Comprehensive model nomograms were generated by combining the independent predictors with Rad-score. The CFM was evaluated by using ROC curves, the reliability of the model was evaluated using calibration curves, and decision curve analysis (DCA) was used to assess the clinical utilities of the radiomics model and the CFM.
Statistical analysis
Statistical analyses were performed using R software v. 4.3.1 (The R Foundation for Statistical Computing, Vienna, Austria) and SPSS v. 27 (IBM Corp., Armonk, NY, USA). Descriptive statistics are presented for all the variables. Normality tests were conducted for continuous variables. Independent samples t-tests were used for normally distributed data, while Mann-Whitney tests were used for nonnormally distributed data. The analyses of categorical variables were based on the χ2 test. Binary logistic regression model was applied during multivariate analyses. A P value of less than 0.05 was considered statistically significant.
LASSO regression analysis was performed using the “glmne” package in the R to screen for the radiomics features and calculate the Rad-scores based on the assigned feature values. Multivariate logistic regression analysis was performed using the “rms” package in R to build the nomograms of the CFM. ROC curves were plotted using the “pROC” package in R to evaluate the predictive performance of the model. Model calibration was checked using the Hosmer-Lemeshow goodness-of-fit test, and internal validation was performed using the bootstrap technique to generate calibration curves. DCA curves were plotted using the “rmda” R package.
Results
Baseline characteristics of patients
A total of 295 patients with stage IIIa/IVb lung cancer were treated with anti-PD-1/PD-L1 monoclonal antibody at the First Affiliated Hospital of Soochow University period from December 2019 to January 2023. After patients with small-cell lung cancer, those receiving postoperative adjuvant therapy/radiotherapy/antiangiogenic therapy, those without available CT images, and/or those with missing baseline data were ruled out, 82 patients included into the final analysis. Of the 82 patients, 41 were treated with sintilimab, 18 with tislelizumab, 19 with pembrolizumab, and 4 with durvalumab. As of October 2023, 9 (10.98%) of the 82 enrolled patients achieved PR, 43 (52.44%) achieved SD, 30 (36.58%) experienced PD, and no patient reached CR. PFS exceeded 12 months in 48 patients (58.54%), with a maximum PFS of 44 months. Among these patients, 34 (59.65%) patients in the training set (n=57) had a PFS more than 12 months (Table 1). The independent samples t-test or Mann-Whitney test for continuous variables and the chi-squared test for categorical variables revealed that there were no significant differences in the demographic or clinical characteristics between the training and validation sets.
Table 1
Feature | Patients (n=82) | Training set (n=57) | Validation set (n=25) | P value |
---|---|---|---|---|
Age (years) | 67.04±9.56 | 66.61±8.94 | 68.00±11.00 | 0.549 |
Gender | 0.662 | |||
Male | 76 (92.68) | 52 (91.23) | 24 (96.00) | |
Female | 6 (7.32) | 5 (8.77) | 1 (4.00) | |
History of smoking | 0.770 | |||
Yes | 56 (68.29) | 35 (61.40) | 21 (84.00) | |
No | 26 (31.71) | 22 (38.60) | 4 (16.00) | |
Tumor location | 0.530 | |||
Central | 55 (67.07) | 37 (64.91) | 18 (72.00) | |
Peripheral | 27 (32.93) | 20 (35.09) | 7 (28.00) | |
Histological type | 0.915 | |||
Squamous cell carcinoma | 41 (50.00) | 28 (49.12) | 13 (52.00) | |
Adenocarcinoma | 38 (46.34) | 27 (47.37) | 11 (44.00) | |
Other | 3 (3.66) | 2 (3.51) | 1 (4.00) | |
PD-L1 expression | 0.930 | |||
<1% | 4 (4.88) | 6 (10.52) | 2 (8.00) | |
1–49% | 24 (29.27) | 15 (26.32) | 8 (32.00) | |
≥50% | 33 (40.24) | 21 (36.84) | 8 (32.00) | |
NA | 21 (25.61) | 15 (26.32) | 7 (28.00) | |
TNM stage | 0.566 | |||
Stage III | 40 (48.78) | 29 (50.88) | 11 (44.00) | |
Stage IV | 42 (51.22) | 28 (49.12) | 14 (56.00) | |
ECOG PS score | 0.424 | |||
≤1 | 74 (90.24) | 50 (87.72) | 24 (96.00) | |
≥2 | 8 (9.76) | 7 (12.28) | 1 (4.00) | |
Treatment regimen | 1.000 | |||
Single-agent immunotherapy | 12 (14.63) | 8 (14.04) | 3 (12.00) | |
Immunotherapy + chemotherapy | 70 (85.37) | 49 (85.96) | 22 (88.00) | |
Treatment line | 0.372 | |||
1 | 62 (75.61) | 41 (71.93) | 21 (84.00) | |
2 | 20 (24.39) | 16 (28.07) | 4 (16.00) | |
PFS, months | 13.37 (13.93) | 13.90 (14.45) | 12.80 (12.93) | 0.992 |
OS, months | 17.48 (11.78) | 17.13 (14.03) | 17.67 (8.90) | 0.426 |
The data are presented as the mean ± SD, median (interquartile range), or n (percentage). PD-L1, programmed death ligand-1; NA, not available; TNM, Tumor Node Metastasis; ECOG PS, Eastern Cooperative Oncology Group performance status; PFS, progression-free survival; OS, overall survival; SD, standard deviation.
Rad-scores and their predictive performance
Eight features with nonzero coefficients were finally obtained from 851 radiomic features in the training set via the LASSO method (Figure 1), and the formula for calculating the Rad-score was established based on the following features as follows:
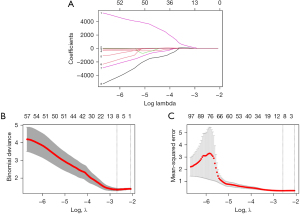
The distribution of the Rad-scores in the training set is shown in Figure 2A. The ROC curve was plotted based on the calculated Rad-scores (Figure 2B), which yielded an AUC of 0.858 [95% confidence interval (CI): 0.763–0.953] for the training set. The optimal threshold for the Rad-scores was 1.576, at which the sensitivity was 73.5% and the specificity was 91.3%. The Rad-scores in the validation set were also calculated using the same method (Figure 2C), and ROC curves were plotted accordingly (Figure 2D), which yielded an AUC of 0.812 (95% CI: 0.641–0.982). The optimal threshold for the Rad-scores in the validation set was 1.572, at which the sensitivity was 78.6% and the specificity was 81.8%. The calibration curve of the radiomics model is shown in Figure 3, which indicated that the radiomics model had good performance and reliability in predicting the efficacy of immunotherapy.
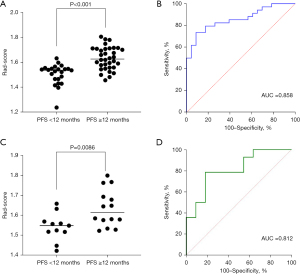
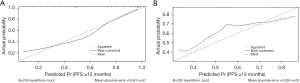
Baseline data and hematologic features
In the training set, no valid predictor, including age, gender, or smoking history, was identified in the univariate analyses of the baseline data (Table 2). Based on the peripheral blood cell profiles before immunotherapy and after two cycles of immunotherapy, we calculated the NLR, PLR, and SII, and their change in value before treatment and after two cycles of treatment. In the training set, the Mann-Whitney test showed that NLR (P=0.03) before immunotherapy and SII (P=0.006) and NLR (P=0.04) after two cycles of immunotherapy were valuable predictors, while serum tumor markers such as neuron-specific enolase (NSE), carcinoembryonic antigen (CEA), and cancer antigen 125 (CA-125) did not show predictive value. The ROC curves of the three screened indices were plotted and generated the following results: the AUC of NLR was 0.676 (95% CI: 0.536–0.817) before immunotherapy, with an optimal cutoff value of 3.26; the AUC of SII after two cycles of immunotherapy was 0.717 (95% CI: 0.581–0.851), with an optimal cutoff value of 500.88; and the AUC of NLR after two cycles of immunotherapy was 0.676 (95% CI: 0.536–0.817), with an optimal cut-off value of 2.08. The screened blood inflammation indices were dichotomized according to their respective cutoff values and subjected to multivariate binary logistic regression analysis, which showed that an SII score of <500.88 after two cycles of treatment was a protective factor for PFS >12 months [odds ratio (OR) 0.054; 95% CI: 0.008–0.365; P=0.003].
Table 2
Feature | PFS <12 months (n=23) | PFS ≥12 months (n=34) | P value |
---|---|---|---|
Age, years | 66.09±9.07 | 66.97±8.97 | 0.718 |
Gender | >0.99 | ||
Male | 21 (91.30) | 31 (91.18) | |
Female | 2 (8.70) | 3 (8.82) | |
History of smoking | 0.239 | ||
Yes | 12 (52.17) | 23 (67.65) | |
No | 11 (47.83) | 11 (32.35) | |
Tumor location | 0.968 | ||
Central | 15 (65.22) | 22 (64.71) | |
Peripheral | 8 (34.78) | 12 (35.29) | |
Histological type | 0.093 | ||
Squamous cell carcinoma | 13 (56.52) | 15 (44.12) | |
Adenocarcinoma | 8 (34.78) | 19 (55.88) | |
Other | 2 (8.70) | 0 | |
PD-L1 expression | 0.744 | ||
<1% | 2 (8.70) | 4 (11.76) | |
1–49% | 8 (34.78) | 7 (20.59) | |
≥50% | 8 (34.78) | 13 (38.24) | |
NA | 5 (21.74) | 10 (29.41) | |
TNM stage | 0.955 | ||
Stage III | 12 (52.17) | 18 (52.94) | |
Stage IV | 11 (47.83) | 16 (47.06) | |
ECOG PS score | 0.106 | ||
≤1 | 18 (78.26) | 32 (94.12) | |
≥2 | 5 (21.74) | 2 (5.88) | |
Treatment regimen | >0.99 | ||
Single-agent immunotherapy | 3 (13.04) | 5 (14.71) | |
Immunotherapy + chemotherapy | 20 (86.96) | 29 (85.29) | |
Treatment line | 0.126 | ||
1 | 14 (60.87) | 27 (79.41) | |
2 | 9 (39.13) | 7 (20.59) |
The data are presented as the mean ± SD or n (percentage). PD-L1, programmed death ligand-1; NA, not available; TNM, Tumor Node Metastasis; ECOG PS, Eastern Cooperative Oncology Group performance status.
Establishment and evaluation of CFM
The Rad-scores and SII scores after two cycles of immunotherapy were included in the logistic regression analysis; accordingly, the CFM was established and the nomograms were plotted (Figure 4). The eigenvalues of the features in the CFM are shown in Figure 5A. The CFM scores were calculated, and the ROC curve was plotted based on these eigenvalues (Figure 5B). In the training set, the CFM had an AUC of 0.930 (95% CI: 0.866–0.993), a Youden index score of 0.765, a sensitivity of 76.5%, and a specificity of 95.7%. In the validation set, the CFM had an AUC of 0.922 (95% CI: 0.794–1.000) and a Youden index score of 0.818, with the diagnostic sensitivity and specificity being 100.0% and 91.8%, respectively.
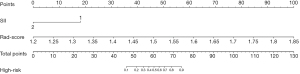
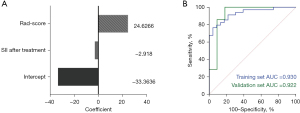
The calibration curves of the CFM in the training and validation sets are shown in Figure 6A,6B, which indicate that the CFM had good reliability and was superior to a single radiomics model. The DCA curves (Figure 6C,6D) suggested that both the radiomics model and the CFM had clinical applications in both the training and validation sets.
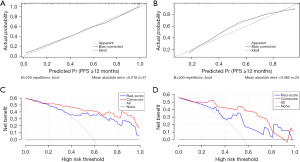
Comparison between radiomics model and CFM
The AUC of the radiomics model in the training group was 0.858 (95% CI: 0.763–0.953), while in the validation group, it was 0.812 (95% CI: 0.641–0.982). The AUC of the comprehensive prediction model in the training group was 0.930 (95% CI: 0.866–0.993), and in the validation group, it was 0.922 (95% CI: 0.794–1.000). Regardless of whether it was in the training or validation group, the comprehensive prediction model seemed to outperform the radiomics model based on the AUC values. However, after calculating the differences between the two models using Delong’s test, the differences between the radiomics model and the comprehensive model were not statistically significant in both the training and validation groups (P values were 0.077 and 0.111, respectively).
Interpretation of the calibration curves revealed that compared to the radiomics model, the calibration curve of the comprehensive model exhibited a higher consistency with the ideal diagonal line, indicating better calibration and higher reliability of the model. In the DCA curve, the net benefit of the comprehensive model was also higher.
Discussion
The radiomics model established in this study had a certain predictive value for whether DCB can be achieved in patients with stage IIIa/IVb NSCLC receiving immunotherapy; furthermore, the CFM, which includes both radiomic features and peripheral blood inflammation indices, had higher reliability than the radiomics model, as shown in both the training set and the validation set.
Immunotherapy remarkably prolongs the PFS and improves the prognosis of patients with NSCLC. However, the delayed immune response and pseudoprogression during immunotherapy may impact the choice of treatment plan. The model developed in our present study can serve as a decision support tool for prognosis prediction and treatment planning, as it can aid in identifying patients who may achieve DCB following immunotherapy and prevent premature discontinuation of immunotherapy or delay in changing an ineffective immunotherapy protocol.
Twenty-one (25.6%) patients in this study were not tested for PD-L1 expression levels, possibly due to economic circumstances or insufficient specimen volume, which might have been one of the reasons why PD-L1 was not included in the CFM. Similar problems may exist in real-world clinical practice. In addition, local PD-L1 expression may not accurately reflect its expression in the whole tumor due to tumor heterogeneity. Furthermore, PD-L1 is continuously expressed, and its expression level can be affected by external factors (39) such as radiochemotherapy. Initial diagnostic biopsy cannot reliably predict the long-term outcomes of immunotherapy (40). CT radiomics has been extensively studied as an economical, convenient, noninvasive, and repeatable tool. Its potential in developing predictive models to aid clinical decision-making has also been investigated.
In the study, all patients were divided into three groups based on stage IIIa, stage IIIb–IIIc, and stage IVa–IVb. The analysis found that the differences among the three groups were no significant difference (P=0.15). Regarding the failure of TNM staging to demonstrate differences between the two groups of PFS <12 months and PFS ≥12 months, possible reasons include: (I) insufficient sample size and insufficient follow-up time; (II) immunotherapy has improved the prognosis of patients, resulting in PFS longer than 12 months for some stage IV NSCLC patients; (III) the existence of selection bias.
With the advancements in radiomics, various models with good performance have been built using machine learning and deep learning. For example, the PD-L1 expression prediction model constructed by Wang et al. (25) using deep learning combined with radiomics showed good performance in assessing OS when combined with clinical features. Deep learning integrates the processes of both feature extraction and model evaluation and can automatically conduct repeated learning and training, thus consuming considerably less manpower. However, it is associated with the risk of overfitting and poor model interpretability, and an optimized evaluation system is needed to improve its clinical applications. Moreover, its efficacy is limited to really big samples which are not typical for such studies. In our study, we used the LASSO method to compress the regression coefficients of the screened radiomics features by generating a penalty function, which helped to prevent overfitting. In addition, we combined the radiomics features with the screened blood inflammation indices to create a CFM, which showed good prediction performance.
Despite these promising findings, our study was limited by the small sample size, a lack of external validation, missing data on PD-L1 expression, and inconsistent immunotherapy protocols due to its retrospective design, which may affect the application and generalization of our model. Multicenter, large-sample, prospective, randomized controlled trials are warranted to optimize our model. In addition, we only collected CT images and blood test results before immunotherapy and only delineated the tumor foci. In our future studies, we will collect CT images and blood test results before and after immunotherapy and delineate both tumors and peritumor areas so as to extract a larger number of radiomics features, identify differential radiomic characteristics, and determine the potential correlation between the changes in the blood test results and the efficacy of immunotherapy. By doing so, we can discover additional factors related to the prognosis of immunotherapy for patients with NSCLC and develop prediction models with improved performance and greater clinical applicability. On the other hand, the authors in (41) have observed that radiomic features with high predictive potential perform much worse when combined with increased feature numbers than when used isolated. It suggests the importance of selective algorithm sensitive to feature interactions. It may be also useful to take advantage of information from multiple lesions to improve predictive ability of radiomic-based prediction model [as suggested in the study by Wilk et al. (42)].
Conclusions
The radiomics model and the CFM performed well in predicting whether patients with locally advanced or metastatic NSCLC can achieve DCB after receiving immunotherapy. Radiomics assessment is essential in the follow-up of patients with cancer, and the efficacy of antitumor therapy is not only reflected in the change of tumor size. More specifically, radiomics can help to detect the early change of internal texture features of the tumors, thus evaluating the efficacy of immunotherapy and informing the adjustment of treatment plans, which is critical for longer survival. However, further prospective studies are required to validate this radiomics technology.
There is still a long way to go before it can be widely used in the clinic as TNM staging, PD-L1 expression and other indicators, and we need to pay attention to the standardization of model construction and the rigor of patient inclusion and data analysis, avoiding the influence of confounding factors on the model, and at the same time, we can combine imaging genomics with the indicators commonly used in the clinic, and even the genomics and pathomics that are developing rapidly, to find the predictive indicators that can really solve the clinical problems. predictive indicators that can really solve the clinical problems. Clinicians and researchers can also make efforts to integrate clinical information, biological and imaging data into databases to facilitate the construction of multicenter and large-sample imaging genomics models, in order to promote imaging genomics to help doctors and patients solve problems more quickly.
Acknowledgments
Funding: None.
Footnote
Reporting Checklist: The authors have completed the TRIPOD reporting checklist. Available at https://jtd.amegroups.com/article/view/10.21037/jtd-24-526/rc
Data Sharing Statement: Available at https://jtd.amegroups.com/article/view/10.21037/jtd-24-526/dss
Peer Review File: Available at https://jtd.amegroups.com/article/view/10.21037/jtd-24-526/prf
Conflicts of Interest: All authors have completed the ICMJE uniform disclosure form (available at https://jtd.amegroups.com/article/view/10.21037/jtd-24-526/coif). A.S. receives grant from the National Science Centre (NCN), Poland (grant No. 2020/37/B/ST6/01959). The other authors have no conflicts of interest to declare.
Ethical Statement: The authors are accountable for all aspects of the work in ensuring that questions related to the accuracy or integrity of any part of the work are appropriately investigated and resolved. This study fully complied with the Declaration of Helsinki (2013 version) and was approved by the Ethics Committee of the First Affiliated Hospital of Soochow University (approval No. 2023540). The requirement for signed informed consent was waived since the data analyzed were anonymous and did not involve patient privacy.
Open Access Statement: This is an Open Access article distributed in accordance with the Creative Commons Attribution-NonCommercial-NoDerivs 4.0 International License (CC BY-NC-ND 4.0), which permits the non-commercial replication and distribution of the article with the strict proviso that no changes or edits are made and the original work is properly cited (including links to both the formal publication through the relevant DOI and the license). See: https://creativecommons.org/licenses/by-nc-nd/4.0/.
References
- Bray F, Laversanne M, Sung H, et al. Global cancer statistics 2022: GLOBOCAN estimates of incidence and mortality worldwide for 36 cancers in 185 countries. CA Cancer J Clin 2024;74:229-63. [Crossref] [PubMed]
- Goldstraw P, Chansky K, Crowley J, et al. The IASLC Lung Cancer Staging Project: Proposals for Revision of the TNM Stage Groupings in the Forthcoming (Eighth) Edition of the TNM Classification for Lung Cancer. J Thorac Oncol 2016;11:39-51. [Crossref] [PubMed]
- Bade BC, dela Cruz CS. Lung Cancer 2020: Epidemiology, Etiology, and Prevention. Clinics in Chest Medicine 2020;41:1-24. [Crossref] [PubMed]
- Molina JR, Yang P, Cassivi SD, et al. Non-small cell lung cancer: epidemiology, risk factors, treatment, and survivorship. Mayo Clin Proc 2008;83:584-94. [Crossref] [PubMed]
- Li B, Chan HL, Chen P. Immune Checkpoint Inhibitors: Basics and Challenges. Curr Med Chem 2019;26:3009-25. [Crossref] [PubMed]
- de Castro G Jr, Kudaba I, Wu YL, et al. Five-Year Outcomes With Pembrolizumab Versus Chemotherapy as First-Line Therapy in Patients With Non-Small-Cell Lung Cancer and Programmed Death Ligand-1 Tumor Proportion Score ≥ 1% in the KEYNOTE-042 Study. J Clin Oncol 2023;41:1986-91. [Crossref] [PubMed]
- Gettinger S, Horn L, Jackman D, et al. Five-Year Follow-Up of Nivolumab in Previously Treated Advanced Non-Small-Cell Lung Cancer: Results From the CA209-003 Study. J Clin Oncol 2018;36:1675-84. [Crossref] [PubMed]
- Suresh K, Naidoo J, Lin CT, et al. Immune Checkpoint Immunotherapy for Non-Small Cell Lung Cancer: Benefits and Pulmonary Toxicities. Chest 2018;154:1416-23. [Crossref] [PubMed]
- Mhandu P, McGonigle N. Lung cancer. Surgery (Oxford) 2023;41:142-7.
- Yarchoan M, Hopkins A, Jaffee EM. Tumor Mutational Burden and Response Rate to PD-1 Inhibition. N Engl J Med 2017;377:2500-1. [Crossref] [PubMed]
- Garon EB, Rizvi NA, Hui R, et al. Pembrolizumab for the treatment of non-small-cell lung cancer. N Engl J Med 2015;372:2018-28. [Crossref] [PubMed]
- Gandara DR, Paul SM, Kowanetz M, et al. Blood-based tumor mutational burden as a predictor of clinical benefit in non-small-cell lung cancer patients treated with atezolizumab. Nat Med 2018;24:1441-8. [Crossref] [PubMed]
- Negrao MV, Skoulidis F, Montesion M, et al. Oncogene-specific differences in tumor mutational burden, PD-L1 expression, and outcomes from immunotherapy in non-small cell lung cancer. J Immunother Cancer 2021;9:e002891. [Crossref] [PubMed]
- So WV, Dejardin D, Rossmann E, et al. Predictive biomarkers for PD-1/PD-L1 checkpoint inhibitor response in NSCLC: an analysis of clinical trial and real-world data. J Immunother Cancer 2023;11:e006464. [Crossref] [PubMed]
- Scognamiglio G, De Chiara A, Di Bonito M, et al. Variability in Immunohistochemical Detection of Programmed Death Ligand 1 (PD-L1) in Cancer Tissue Types. Int J Mol Sci 2016;17:790. [Crossref] [PubMed]
- Roulleaux Dugage M, Albarrán-Artahona V, Laguna JC, et al. Biomarkers of response to immunotherapy in early stage non-small cell lung cancer. Eur J Cancer 2023;184:179-96. [Crossref] [PubMed]
- Havel JJ, Chowell D, Chan TA. The evolving landscape of biomarkers for checkpoint inhibitor immunotherapy. Nat Rev Cancer 2019;19:133-50. [Crossref] [PubMed]
- Ilie M, Long-Mira E, Bence C, et al. Comparative study of the PD-L1 status between surgically resected specimens and matched biopsies of NSCLC patients reveal major discordances: a potential issue for anti-PD-L1 therapeutic strategies. Ann Oncol 2016;27:147-53. [Crossref] [PubMed]
- Bianco A, Perrotta F, Barra G, et al. Prognostic Factors and Biomarkers of Responses to Immune Checkpoint Inhibitors in Lung Cancer. Int J Mol Sci 2019;20:4931. [Crossref] [PubMed]
- Rizvi NA, Hellmann MD, Brahmer JR, et al. Nivolumab in Combination With Platinum-Based Doublet Chemotherapy for First-Line Treatment of Advanced Non-Small-Cell Lung Cancer. J Clin Oncol 2016;34:2969-79. [Crossref] [PubMed]
- Ricciuti B, Wang X, Alessi JV, et al. Association of High Tumor Mutation Burden in Non-Small Cell Lung Cancers With Increased Immune Infiltration and Improved Clinical Outcomes of PD-L1 Blockade Across PD-L1 Expression Levels. JAMA Oncol 2022;8:1160-8. [Crossref] [PubMed]
- Pan F, Feng L, Liu B, et al. Application of radiomics in diagnosis and treatment of lung cancer. Front Pharmacol 2023;14:1295511. [Crossref] [PubMed]
- Tran KA, Kondrashova O, Bradley A, et al. Deep learning in cancer diagnosis, prognosis and treatment selection. Genome Med 2021;13:152. [Crossref] [PubMed]
- Le NQK, Kha QH, Nguyen VH, et al. Machine Learning-Based Radiomics Signatures for EGFR and KRAS Mutations Prediction in Non-Small-Cell Lung Cancer. Int J Mol Sci 2021;22:9254. [Crossref] [PubMed]
- Wang C, Ma J, Shao J, et al. Non-Invasive Measurement Using Deep Learning Algorithm Based on Multi-Source Features Fusion to Predict PD-L1 Expression and Survival in NSCLC. Front Immunol 2022;13:828560. [Crossref] [PubMed]
- Zhou Y, Ma XL, Zhang T, et al. Use of radiomics based on (18)F-FDG PET/CT and machine learning methods to aid clinical decision-making in the classification of solitary pulmonary lesions: an innovative approach. Eur J Nucl Med Mol Imaging 2021;48:2904-13. [Crossref] [PubMed]
- Mu W, Tunali I, Gray JE, et al. Radiomics of (18)F-FDG PET/CT images predicts clinical benefit of advanced NSCLC patients to checkpoint blockade immunotherapy. Eur J Nucl Med Mol Imaging 2020;47:1168-82. [Crossref] [PubMed]
- Trebeschi S, Drago SG, Birkbak NJ, et al. Predicting response to cancer immunotherapy using noninvasive radiomic biomarkers. Ann Oncol 2019;30:998-1004. [Crossref] [PubMed]
- Farina B, Guerra ADR, Bermejo-Peláez D, et al. Integration of longitudinal deep-radiomics and clinical data improves the prediction of durable benefits to anti-PD-1/PD-L1 immunotherapy in advanced NSCLC patients. J Transl Med 2023;21:174. [Crossref] [PubMed]
- Greten FR, Grivennikov SI. Inflammation and Cancer: Triggers, Mechanisms, and Consequences. Immunity 2019;51:27-41. [Crossref] [PubMed]
- Pavan A, Calvetti L, Dal Maso A, et al. Peripheral Blood Markers Identify Risk of Immune-Related Toxicity in Advanced Non-Small Cell Lung Cancer Treated with Immune-Checkpoint Inhibitors. Oncologist 2019;24:1128-36. [Crossref] [PubMed]
- Russo A, Russano M, Franchina T, et al. Neutrophil-to-Lymphocyte Ratio (NLR), Platelet-to-Lymphocyte Ratio (PLR), and Outcomes with Nivolumab in Pretreated Non-Small Cell Lung Cancer (NSCLC): A Large Retrospective Multicenter Study. Adv Ther 2020;37:1145-55. [Crossref] [PubMed]
- Takada K, Takamori S, Yoneshima Y, et al. Serum markers associated with treatment response and survival in non-small cell lung cancer patients treated with anti-PD-1 therapy. Lung Cancer 2020;145:18-26. [Crossref] [PubMed]
- Yang Y, Xu H, Yang G, et al. The value of blood biomarkers of progression and prognosis in ALK-positive patients with non-small cell lung cancer treated with crizotinib. Asia Pac J Clin Oncol 2020;16:63-9. [Crossref] [PubMed]
- Ksienski D, Wai ES, Alex D, et al. Prognostic significance of the neutrophil-to-lymphocyte ratio and platelet-to-lymphocyte ratio for advanced non-small cell lung cancer patients with high PD-L1 tumor expression receiving pembrolizumab. Transl Lung Cancer Res 2021;10:355-67. [Crossref] [PubMed]
- Fest J, Ruiter R, Mulder M, et al. The systemic immune-inflammation index is associated with an increased risk of incident cancer-A population-based cohort study. Int J Cancer 2020;146:692-8. [Crossref] [PubMed]
- Zheng F, Meng Q, Zhang L, et al. Prognostic roles of hematological indicators for the efficacy and prognosis of immune checkpoint inhibitors in patients with advanced tumors: a retrospective cohort study. World J Surg Oncol 2023;21:198. [Crossref] [PubMed]
- Fang Q, Yu J, Li W, et al. Prognostic value of inflammatory and nutritional indexes among advanced NSCLC patients receiving PD-1 inhibitor therapy. Clin Exp Pharmacol Physiol 2023;50:178-90. [Crossref] [PubMed]
- Fan Z, Wu C, Chen M, et al. The generation of PD-L1 and PD-L2 in cancer cells: From nuclear chromatin reorganization to extracellular presentation. Acta Pharm Sin B 2022;12:1041-53. [Crossref] [PubMed]
- Reck M, Remon J, Hellmann MD. First-Line Immunotherapy for Non-Small-Cell Lung Cancer. J Clin Oncol 2022;40:586-97. [Crossref] [PubMed]
- Wilk AM, Kozłowska E, Borys D, et al. Radiomic signature accurately predicts the risk of metastatic dissemination in late-stage non-small cell lung cancer. Transl Lung Cancer Res 2023;12:1372-83. [Crossref] [PubMed]
- Wilk AM, Kozlowska E, Borys D, et al. Improving the predictive ability of radiomics-based regression survival models through incorporating multiple regions of interest. In: Strumillo P, Klepaczko A, Strzelecki M, et al., editors. The Latest Developments and Challenges in Biomedical Engineering. Lecture Notes in Networks and Systems 746, Springer Nature, Switzerland, 2024. doi:
10.1007/978-3-031-38430-1_13 .