Construction and validation of a risk prediction model for postoperative delirium in patients with off‑pump coronary artery bypass grafting
Highlight box
Key findings
• Postoperative delirium (POD) is associated with age, tissue oxygen saturation, mean arterial pressure, carotid artery stenosis, the anterior-posterior diameter of the aortic sinus, interventricular septal thickness, left ventricular ejection fraction, and mini-mental state examination scores in patients who undergo off‑pump coronary artery bypass grafting (OPCABG). The predictive model constructed based on these variables had high predictive performance and could be helpful in the early identification of patients who are at risk of POD.
What is known and what is new?
• Compared to on-pump coronary artery bypass grafting, OPCABG has the advantage of reduced trauma, but there is still a risk of neurological complications, such as POD. POD is associated with a poor prognosis and can lead to increased mortality, a prolonged hospital stay, an increased risk of re-admission within six months, decreased cognitive function, and memory decline.
• This study established a clinical prediction model, which is helpful for early identification of patients with increased risk for post-OPCABG delirium.
What is the implication, and what should change now?
• The early identification of patients with an increased risk of POD and appropriate interventions could reduce the incidence of POD and related adverse events.
Introduction
Along with the improvement of living standards among people, the incidence of coronary heart disease has increased year by year, as has the number of patients undergoing off-pump coronary artery bypass grafting (OPCABG) (1,2). Compared with on-pump coronary artery bypass grafting, OPCABG, a technique in which the anastomoses are performed on the beating heart, can reduce myocardial injury by preserving native coronary blood flow (3,4). However, it has a risk of neurological complications, including postoperative delirium (POD) (5,6).
POD is an acute psychotic disorder characterized by reversible changes in the central nervous system caused by an underlying systemic disorder (7). Its main manifestations include decreased consciousness, impaired thinking, and impaired attention (8). In the Guideline for Postoperative Delirium published by the European Society of Anaesthesiology in 2017, the observation time of POD was adjusted from the anesthesia recovery period to a timepoint of 120 hours after surgery (9). With advances in surgical techniques, the incidence of POD in cardiac surgery has decreased over time. However, at a rate of 26−52%, the incidence of POD remains high (10,11).
POD is associated with a poor prognosis and can lead to increased mortality, a prolonged hospital stay, an increased risk of rehospitalization within 6 months, cognitive decline, and memory decline, and it can also have adverse effects on patients’ physiological function and quality of life (12-14). The pathogenesis of POD is not completely clear. The core approach in POD management is prevention. Reducing POD has important clinical and social value (15). Delirium is usually reversible, and early identification and intervention in patients at high risk of POD can reduce the incidence of POD and complications, shorten hospital stays, improve patient outcomes, and reduce healthcare costs (16-18). To date, few studies have been conducted on the risk of POD in OPCABG patients, and no standardized prediction model has been established. This study sought to construct a POD prediction model for OPCABG patients that can assist medical staff to identify high-risk patients as early as possible and actively and effectively prevent POD. We present this article in accordance with the TRIPOD reporting checklist (available at https://jtd.amegroups.com/article/view/10.21037/jtd-24-578/rc).
Methods
Subjects
The clinical data of patients who underwent OPCABG at the Tianjin University Chest Hospital from June 2021 to March 2023 were screened. In total, 1,258 patients were randomly divided into the training set (944 cases, 75%) and test set (314 cases, 25%). Patients were excluded from the study if any important clinical data were lacking.
Methodology
Study design
This study is a sub-analysis of the Tianjin Science and Technology Program project—Early warning method and application of perioperative adverse events in off-pump coronary artery bypass surgery based on artificial intelligence data analysis (ChiCTR2100045079). The study was conducted in accordance with the Declaration of Helsinki (as revised in 2013) and was approved by the Ethics Committee of Tianjin University Chest Hospital (No. 2020YS-022-01). All the patients’ legal representatives signed the written informed consent form.
General clinical information
The general clinical information of the patients were collected, including their age, gender, smoking history, alcohol history, body mass index, education level, working type, history of type 2 diabetes mellitus, history of hypertension, history of myocardial infarction, history of arrhythmia, neurological history, and cardiac output, stroke volume, systemic vascular resistance one day before surgery, mean arterial pressure (MAP), heart rate, tissue oxygen saturation, Montreal Cognitive Assessment score, Mini-Mental State Examination (MMSE) score, metabolic equivalent, and European Cardiovascular Surgery Risk Factor score.
Preoperative indicators
The preoperative laboratory examination results and ultrasound examination results of the patients were collected, including the pH, partial pressure of carbon dioxide, partial pressure of oxygen, K+, HCO3−, blood glucose, standard base excess (SBE), hemoglobin, platelet count, hematocrit in the blood routine examination, alanine aminotransferase, aspartate aminotransferase, albumin, urea nitrogen, creatinine, uric acid, creatine kinase, lactate dehydrogenase, hydroxybutyrate dehydrogenase, cardiac troponin T, C-reactive protein, B-type natriuretic peptide, carotid artery stenosis, atrioventricular cavity diameter, ventricular septal thickness, pulmonary artery systolic blood pressure, and left ventricular ejection fraction (LVEF).
Intraoperative indicators
In our hospital, the majority of coronary artery bypass grafting surgery were performed using the off-pump method. Only if intraoperative hemodynamic instability or serious arrhythmia occurred, the operation would switch to on-pump. The intraoperative anesthesia induction time, operation duration, number of coronary artery bypasses, minimum intraoperative hemoglobin concentration, and maximum intraoperative lactate level were collected for the patients.
Postoperative indicators
During the anesthesia recovery period and five days post-surgery, each patient was observed and monitored each morning and each afternoon. Under the Diagnostic and Statistical Manual of Mental Disorders, 5th edition developed by the American Psychiatric Association (12), the diagnostic criteria for POD are as follows: (I) acute alterations or fluctuations in mental status; (II) attention disorders; (III) disordered thinking; and (IV) a decreased level of consciousness. Patients were diagnosed with POD if their clinical presentation complied with (I) and (II), plus either (III) or (IV). POD was defined as a positive screening diagnosis at least once.
Statistical methods
The data analysis was performed using R Core Team [2023]. R: A language and environment for statistical computing. R Foundation for Statistical Computing, Vienna, Austria. URL https://www.R-project.org/. The normally distributed quantitative data are expressed as the mean ± standard deviation (), the independent samples t-test was used for comparisons between groups. The quantitative data with a skewed distribution are expressed as the median [interquartile range (IQR)], and the non-parametric test was used for comparisons between groups. The qualitative data are presented as number (percentage), and the chi-square test was used for comparisons between groups. The variables affecting the occurrence of POD were screened out by least absolute shrinkage and selection operator (LASSO) regression analysis. A prediction model and nomogram was constructed by the multivariate logistic regression analysis. The discrimination ability of the model was evaluated by using the receiver operating characteristic (ROC) curve. Prediction accuracy was assessed by the calibration curves. The clinical utility of the model was evaluated by a decision curve analysis (DCA). The effectiveness of the nomogram was verified by the test set.
Results
Cohort comparison
A total of 1,258 eligible patients were enrolled in the study. The enrolled patients were randomly divided into the training set (n=944) and the test set (n=314) at a ratio of 3:1. There was no significant difference in the clinical data between the training set and the test set (P>0.05). The incidence of delirium was 21.5% and 21.0% in the training and test sets, respectively (Table 1).
Table 1
Characteristics | Training set (n=944) | Validation set (n=314) | t/χ2 | P value |
---|---|---|---|---|
Age (years) | 66.77±7.05 | 66.90±6.68 | –0.280 | 0.78 |
Male | 681 (72.1) | 232 (73.9) | 0.361 | 0.56 |
Drink (yes) | 247 (26.2) | 85 (27.1) | 0.099 | 0.76 |
BMI (kg/m2) | 25.52±3.21 | 25.36±3.35 | 0.724 | 0.46 |
Education (secondary school and below) | 611 (64.7) | 203 (64.6) | 0.001 | >0.99 |
Work type (manual labor) | 652 (69.1) | 206 (65.6) | 1.303 | 0.25 |
Diabetes history | 1.947 | 0.37 | ||
No | 544 (57.6) | 195 (62.1) | ||
Yes, good control | 47 (5.0) | 14 (4.5) | ||
Yes, bad control | 353 (37.4) | 105 (33.4) | ||
Hypertension history | 0.973 | 0.61 | ||
No | 291 (30.8) | 105 (33.4) | ||
Yes, good control | 303 (32.1) | 93 (29.6) | ||
Yes, bad control | 350 (37.1) | 116 (36.9) | ||
MI history (yes) | 243 (25.7) | 83 (26.4) | 0.059 | 0.80 |
Arrhythmia history (yes) | 85 (9.0) | 27 (8.6) | 0.048 | 0.82 |
Neurological history (yes) | 228 (24.2) | 68 (21.7) | 0.816 | 0.36 |
CO (L/min) | 4.57±1.52 | 4.47±1.43 | 1.064 | 0.28 |
SV (mL) | 64.67 (53.67, 79.25) | 64.00 (53.59, 79.00) | –0.358 | 0.72 |
SVR (dynes·s/cm5) | 1,538.67 (1,244.33, 1,866.34) | 1,538.34 (1,259.59, 1,903.50) | –0.720 | 0.47 |
MAP (mmHg) | 90.00±12.16 | 90.03±13.22 | –0.037 | 0.97 |
HR (beat/min) | 67.93±9.46 | 67.07±9.24 | 1.400 | 0.16 |
Tissue oxygen (%) | 74.04±4.90 | 73.97±4.53 | 0.224 | 0.82 |
MoCA score | 23.00 (19.00, 26.00) | 23.00 (19.00, 26.00) | –0.872 | 0.38 |
MMSE score | 26.00 (23.00, 28.00) | 26.00 (23.00, 28.00) | –1.528 | 0.12 |
MET score | 4.50 (4.50, 4.50) | 4.50 (4.38, 4.50) | –0.196 | 0.84 |
EuroScore | 3.469 | 0.17 | ||
0.80% | 280 (29.7) | 104 (33.1) | ||
3.00% | 499 (52.9) | 147 (46.8) | ||
11.20% | 165 (17.5) | 63 (20.1) | ||
pH | 7.40±0.20 | 7.41±0.03 | –0.759 | 0.44 |
pCO2 (mmHg) | 37.96±3.81 | 37.85±4.53 | 0.406 | 0.68 |
pO2 (mmHg) | 95.54±23.34 | 95.6±23.88 | –0.041 | 0.96 |
Serum potassium (mmol/L) | 3.74±1.08 | 3.69±0.30 | 0.789 | 0.43 |
HCO3− (mmol/L) | 23.67±6.84 | 23.44±1.88 | 0.594 | 0.55 |
Glu (mmol/L) | 9.59±3.89 | 9.30±3.98 | 1.145 | 0.25 |
Standard base excess (mmol/L) | –0.73±2.09 | –0.73±2.29 | –0.025 | 0.98 |
Hb (g/L) | 133.71±20.71 | 134.51±19.01 | –0.602 | 0.54 |
PLT (109/L) | 211.82±57.82 | 215.52±56.84 | –0.985 | 0.32 |
Hct (%) | 40.34±9.49 | 40.30±4.48 | 0.059 | 0.95 |
ALT (U/L) | 18.20 (13.10, 26.68) | 18.80 (13.18, 28.15) | –0.936 | 0.34 |
AST (U/L) | 18.10 (14.80, 24.00) | 17.80 (14.40, 24.13) | –0.490 | 0.62 |
ALB (g/L) | 41.15±3.77 | 41.21±3.41 | –0.231 | 0.81 |
BUN (mmol/L) | 5.60 (4.60, 6.80) | 5.50 (4.50, 6.73) | –0.850 | 0.39 |
Cr (μmol/L) | 79.41±20.7 | 80.26±18.52 | –0.648 | 0.51 |
UA (μmol/L) | 323.69±88.49 | 327.02±85.66 | –0.581 | 0.56 |
CK (U/L) | 67.00 (49.00, 91.75) | 66.50 (47.00, 95.25) | –0.117 | 0.90 |
LDH (U/L) | 193.13±48.03 | 199.29±48.36 | –1.966 | 0.05 |
HBDH (U/L) | 148.49±39.88 | 152.00±39.71 | –1.351 | 0.17 |
cTnT (μg/L) | 0.07±0.26 | 0.10±0.43 | –1.230 | 0.21 |
CRP (mg/L) | 2.07 (0.77, 5.39) | 1.76 (0.74, 5.05) | –0.663 | 0.50 |
BNP (pg/mL) | 39.95 (14.71, 130.62) | 38.93 (15.40, 118.70) | –0.049 | 0.96 |
Bilateral carotid artery stenosis | 351 (37.2) | 121 (38.5) | 0.184 | 0.68 |
Aortic sinus post-diameter (mm) | 34.00±3.36 | 34.10±3.58 | –0.448 | 0.65 |
Left atriumo post-diameter (mm) | 37.99±4.23 | 38.42±4.63 | –1.526 | 0.12 |
Left ventricle end diastolic diameter (mm) | 51.99±5.02 | 52.23±5.61 | –0.718 | 0.47 |
Interventricular septal thickness (mm) | 10.38±1.79 | 10.39±1.93 | –0.007 | 0.99 |
Right ventricle post-diameter (mm) | 17.09±2.70 | 16.99±1.61 | 0.571 | 0.56 |
Right ventricle basa diameters (mm) | 32.96±3.38 | 32.94±3.87 | 0.079 | 0.93 |
Pulmonary artery systolic pressure (mmHg) | 30.40±2.45 | 30.54±2.50 | –0.878 | 0.38 |
LVEF (%) | 57.36±7.48 | 57.46±7.22 | –0.193 | 0.84 |
Anesthesia induction time (am) | 539 (57.1) | 177 (56.4) | 0.051 | 0.82 |
Surgical duration (min) | 191.00 (162.00, 224.75) | 190.00 (165.75, 224.00) | –0.042 | 0.96 |
Coronary arteries number | 3.00 (2.00, 3.00) | 3.00 (2.00, 3.00) | –1.013 | 0.31 |
Hb mini (g/L) | 11.30 (10.30, 12.40) | 11.50 (10.30, 12.70) | –1.155 | 0.24 |
Lactic acid max (mmol/L) | 1.10 (0.90, 1.40) | 1.10 (0.90, 1.50) | –1.231 | 0.21 |
Delirium | 203 (21.5) | 66 (21.0) | 0.033 | 0.85 |
Data are expressed as the , n (%), or the M (P25, P75). BMI, body mass index; CO, cardiac output; SV, stroke volume; SVR, systemic vascular resistance; MAP, mean arterial pressure; HR, heart rate; MoCA, Montreal Cognitive Assessment; MMSE, Mini-Mental State Examination; MET, metabolic equivalent; pCO2, partial pressure of carbon dioxide; pO2, partial pressure of oxygen; Glu, blood glucose; Hb, hemoglobin; PLT, platelet; Hct, hematocrit in the blood routine; ALT, alanine aminotransferase; AST, aspartate aminotransferase; ALB, albumin; BUN, urea nitrogen; Cr, creatinine; UA, uric acid; CK, creatine kinase; LDH, lactate dehydrogenase; HBDH, hydroxybutyrate dehydrogenase; cTnT, cardiac troponin T; CRP, C-reactive protein; BNP, B-type natriuretic peptide; LVEF, left ventricular ejection fraction; M (P25, P75), median (percentiles25, percentiles75).
Screening of variables affecting the occurrence of POD in OPCABG patients
A total of 59 variables were analyzed by a univariate regression analysis and 46 variables with statistically significant differences were identified. These 46 variables were included in the LASSO regression analysis, and the most significant variables included in the model were identified. In total, 15 variables were identified in the LASSO regression analysis (Figure 1). Next, 8 clinically significant variables (i.e., age, tissue oxygen saturation, MAP, carotid stenosis, the anteroposterior diameter of the aortic sinus, ventricular septum thickness, LVEF, and MMSE score) were further identified by the multivariate logistic regression analysis and included in the prediction model.
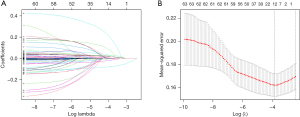
Construction of a prediction model for POD in patients with OPCABG
The results of the multivariate logistic regression analysis showed that age, tissue oxygen saturation, MAP, carotid artery stenosis, the anteroposterior diameter of the aortic sinus, ventricular septal thickness, LVEF, and MMSE score were predictors of POD in patients with OPACDG (P<0.05) (Table 2). Based on the results of the multivariate regression analysis, a nomogram model was constructed using R Core Team [2023] to predict the occurrence of POD in OPACDG patients (Figure 2).
Table 2
Variables | Estimate | Wald | OR (95% CI) | P value |
---|---|---|---|---|
Bilateral carotid artery stenosis | 0.419 | 6.160 | 1.520 (1.091−2.116) | 0.01 |
Age | 0.038 | 7.884 | 1.038 (1.012−1.067) | 0.005 |
Tissue oxygen | –0.050 | 8.694 | 0.951 (0.920−0.983) | 0.003 |
MAP | 0.016 | 5.192 | 1.016 (1.002−1.030) | 0.02 |
Aortic sinus post-diameter | 0.052 | 4.281 | 1.053 (1.003−1.107) | 0.03 |
Interventricular septal thickness | 0.099 | 4.581 | 1.104 (1.008−1.210) | 0.03 |
LVEF | –0.026 | 5.535 | 0.974 (0.954−0.996) | 0.01 |
MMSE | –0.102 | 29.538 | 0.903 (0.871−0.937) | <0.001 |
POD, postoperative delirium; OPCABG, off‑pump coronary artery bypass grafting; MAP, mean arterial pressure; LVEF, left ventricular ejection fraction; MMSE, Mini-Mental State Examination; OR, odds ratio; CI, confidence interval.
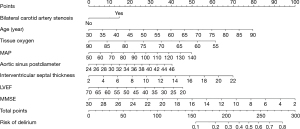
Evaluation and validation of the POD prediction model in patients with OPCABG
The ROC curve results demonstrated that the area under the curve (AUC) of the development model was 0.702 [95% confidence interval (CI): 0.662–0.743], and the discrimination performance of the model was verified in the validation model (AUC: 0.658; 95% CI: 0.585–0.730) (Figure 3).
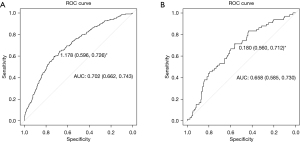
The calibration curves of the nomogram model were then plotted, and the accuracy of the nomogram model was evaluated by the Hosmer-Lemeshow goodness-of-fit test. The analysis showed that the predicted POD risk of OPCABG patients in the training and test sets was consistent with the actual POD risk (χ2=5.154, P=0.74) (Figure 4).
The DCA demonstrated that the model was clinically useful with the net benefit at a threshold probability of 0.1–0.35 (Figure 5).
Discussion
POD is a common complication of heart surgery (19), and coronary artery bypass grafting accounts for about 21.1% of all cardiac surgeries in China (20). OPCABG has a lower risk of post-operative delirium compared to other types of bypass grafting surgery (6,21). In this study, the incidence of POD in the OPCABG patients was 21.5%. This represents a decrease from the incidence of POD reported in previous studies on cardiac surgery of 26–52% (22,23). POD significantly increases the short- and long-term mortality rate of patients after surgery, and affects the physiological and social functions of patients (24,25). Rapid identification and effective prevention could have great significance in reducing the occurrence of POD; thus, a model based on the risk factors of POD in patients with OPCABG is necessitated. In this study, the retrospective analysis showed that age, tissue oxygen saturation, MAP, carotid artery stenosis, anteroposterior diameter of the aortic sinus, ventricular septal thickness, LVEF, and MMSE score were independent influencing factors for POD in OPCABG patients. The preliminary validation results indicated that the prediction model had certain clinical practical value.
Risk factors and precautionary measures for POD in patients with OPCABG
POD in OPCABG patients is influenced by a variety of factors. In this study, MMSE score and age were the top two independent risk factors for the occurrence of POD. This finding is consistent with the results of other domestic and foreign study (26). Patients with mild preoperative cognitive impairment are at an increased risk of POD. Impaired cognitive function is an independent predictor of POD (27-32). Cognitive function is assessed by the MMSE. The MMSE can be classified into the following five dimensions: orientation, memory, attention, recall, and language ability. Among these dimensions, individuals with reduced memory and recall scores have been shown to be at greater risk of developing delirium (33,34).
Aging is often associated with cerebral atrophy, decreased brain volume, other senile brain changes, and an increased risk of neurological diseases, such as stroke and cerebral blood supply insufficiency (24,28). Chronic inflammatory changes in cerebral blood vessels can also lead to brain dysfunction, reduced compensatory ability, metabolic disorders of neurotransmitters, and cerebral hypoperfusion, inducing POD (31,35). Ventricular septal thickening predominantly occurs in patients with hypertension. Persistently elevated blood pressure increases afterload, a large resistance to pumping blood from the left heart system, and thickening of the ventricular septum may occur, all of which indicate poorly controlled hypertension. Hypertension with complications is associated with an increased risk of POD (36).
Tissue oxygen saturation, a parameter influenced by the balance between tissue oxygen consumption and oxygen supply, can reflect tissue perfusion. When oxygen consumption is greater than oxygen supply, it will lead to tissue hypoperfusion, which could cause functional lesions and the subsequent POD (37,38). As anti-hypertension may be performed during OPCABG surgery, blood pressure fluctuations can occur during surgery in patients with relatively high preoperative blood pressure. It has been reported that blood pressure fluctuation during surgery is significantly correlated with POD (6).
In addition, reduced LVEF is an independent risk factor for POD. Cai et al. (39) found that the higher the LVEF, the lower the incidence of POD after OPCAB surgery. LVEF reflects left ventricular systolic function. Reduced LVEF, reduced left ventricular systolic function, reduced cardiac output, and less perfusion to each organ may contribute to cerebral ischemia-reperfusion injury. Carotid artery disease has been associated with POD after cardiac surgery (32), which is consistent with the results of this study. We found that the widening of the aortic sinus is also an independent risk factor for POD. The widening of the aortic sinus may lead to a decrease in cardiac output and a decrease in cerebral blood supply, which could lead to POD.
Several previously published studies had reported that preoperative and intraoperative interventions could attenuate the risk of POD. Preoperative measures mainly include education and psychological comfort (40), rehabilitation training, shortening preoperative fasting water duration, hearing or visual assistance, improving sleep quality (41-43), correcting anemia and hypoproteinemia, nutritional support, controlling infection, and standardized management of blood glucose and blood pressure (44,45). Using bispectral index (BIS) to control the depth of anesthesia and appropriate use of dexmedetomidine intraoperatively could reduce the incidence of POD as well (46).
Effectiveness of POD prediction models in patients with OPCABG
In terms of model construction, this is the first study to predict the occurrence of POD in patients with OPCABG. All the variables included in the prediction model are readily available predictors. The risk factors for POD in the OPCABG patients were identified by LASSO regression, and a prediction model was established based on these variables. The application of this model may help clinicians to identify and intervene early to prevent the occurrence of POD. However, this study was a single-center retrospective study and may be subject to bias. In the future, it is necessary to conduct multi-center prospective studies with larger sample sizes to further evaluate the clinical application efficacy of the model.
Conclusions
After reviewing the 1,258 patients who underwent OPCABG surgery, eight independent predictors of the development of POD were identified. A nomogram was constructed with these eight predictors to identify patients at high risk of developing POD. Early intervention can reduce the risk of developing POD.
Acknowledgments
Funding: This work was supported by
Footnote
Reporting Checklist: The authors have completed the TRIPOD reporting checklist. Available at https://jtd.amegroups.com/article/view/10.21037/jtd-24-578/rc
Data Sharing Statement: Available at https://jtd.amegroups.com/article/view/10.21037/jtd-24-578/dss
Peer Review File: Available at https://jtd.amegroups.com/article/view/10.21037/jtd-24-578/prf
Conflicts of Interest: All authors have completed the ICMJE uniform disclosure form (available at https://jtd.amegroups.com/article/view/10.21037/jtd-24-578/coif). The authors have no conflicts of interest to declare.
Ethical Statement: The authors are accountable for all aspects of the work in ensuring that questions related to the accuracy or integrity of any part of the work are appropriately investigated and resolved. The study was conducted in accordance with the Declaration of Helsinki (as revised in 2013) and was approved by the Ethics Committee of Tianjin University Chest Hospital (No. 2020YS-022-01). All the patients’ legal representatives signed the written informed consent form.
Open Access Statement: This is an Open Access article distributed in accordance with the Creative Commons Attribution-NonCommercial-NoDerivs 4.0 International License (CC BY-NC-ND 4.0), which permits the non-commercial replication and distribution of the article with the strict proviso that no changes or edits are made and the original work is properly cited (including links to both the formal publication through the relevant DOI and the license). See: https://creativecommons.org/licenses/by-nc-nd/4.0/.
References
- Mestres CA, Quintana E. The never-ending story: on-pump and off-pump coronary artery bypass grafting versus percutaneous coronary intervention and long-term survival. Eur J Cardiothorac Surg 2023;64:ezad269. [Crossref] [PubMed]
- Chan J, Dimagli A, Dong T, et al. Trend and early clinical outcomes of off-pump coronary artery bypass grafting in the UK. Eur J Cardiothorac Surg 2023;64:ezad272. [Crossref] [PubMed]
- Jiang Y, Xu L, Liu Y, et al. Beating-heart on-pump coronary artery bypass grafting vs. off-pump coronary artery bypass grafting: a systematic review and meta-analysis. J Thorac Dis 2021;13:4185-94. [Crossref] [PubMed]
- Kowalewski M, Pawliszak W, Malvindi PG, et al. Off-pump coronary artery bypass grafting improves short-term outcomes in high-risk patients compared with on-pump coronary artery bypass grafting: Meta-analysis. J Thorac Cardiovasc Surg 2016;151:60-77.e1-58.
- Tian L, Wang H, Jia Y, et al. Effect of percutaneous cerebral oximetry-guided anaesthetic management on postoperative delirium in older adults undergoing off-pump coronary artery bypass grafting: study protocol for a single-centre prospective randomised controlled trial in a tertiary academic hospital in China. BMJ Open 2023;13:e076419. [Crossref] [PubMed]
- Ma Y, Sui D, Yang S, et al. Optimal postoperative delirium prediction after coronary artery bypass grafting surgery: a prospective cohort study. Front Cardiovasc Med 2023;10:1251617. [Crossref] [PubMed]
- Zhang Y, Wan DH, Chen M, et al. Automated machine learning-based model for the prediction of delirium in patients after surgery for degenerative spinal disease. CNS Neurosci Ther 2023;29:282-95. [Crossref] [PubMed]
- Hu XY, Liu H, Zhao X, et al. Automated machine learning-based model predicts postoperative delirium using readily extractable perioperative collected electronic data. CNS Neurosci Ther 2022;28:608-18. [Crossref] [PubMed]
- Aldecoa C, Bettelli G, Bilotta F, et al. European Society of Anaesthesiology evidence-based and consensus-based guideline on postoperative delirium. Eur J Anaesthesiol 2017;34:192-214. [Crossref] [PubMed]
- Meco M, Giustiniano E, Cecconi M, et al. Pharmacological prevention of postoperative delirium in patients undergoing cardiac surgery: a bayesian network meta-analysis. J Anesth 2023;37:294-310. [Crossref] [PubMed]
- Yang T, Yang H, Liu Y, et al. Postoperative delirium prediction after cardiac surgery using machine learning models. Comput Biol Med 2024;169:107818. [Crossref] [PubMed]
- Zhao X, Li J, Xie X, et al. Online interpretable dynamic prediction models for postoperative delirium after cardiac surgery under cardiopulmonary bypass developed based on machine learning algorithms: A retrospective cohort study. J Psychosom Res 2024;176:111553. [Crossref] [PubMed]
- Lee S, Jung DE, Park D, et al. Intraoperative neurological pupil index and postoperative delirium and neurologic adverse events after cardiac surgery: an observational study. Sci Rep 2023;13:13838. [Crossref] [PubMed]
- Khalifa C, Robert A, Cappe M, et al. Serum Neurofilament Light and Postoperative Delirium in Cardiac Surgery: A Preplanned Secondary Analysis of a Prospective Observational Study. Anesthesiology 2024;140:950-62. [Crossref] [PubMed]
- Namirembe GE, Baker S, Albanese M, et al. Association Between Postoperative Delirium and Long-Term Subjective Cognitive Decline in Older Patients Undergoing Cardiac Surgery: A Secondary Analysis of the Minimizing Intensive Care Unit Neurological Dysfunction with Dexmedetomidine-Induced Sleep Trial. J Cardiothorac Vasc Anesth 2023;37:1700-6. [Crossref] [PubMed]
- Dogan TD, Guttenthaler V, Zimmermann A, et al. Functional intervention following cardiac surgery to prevent postoperative delirium in older patients (FEEL WELL study). J Intensive Care 2023;11:62. [Crossref] [PubMed]
- Zhou C, Qu X, Wang L, et al. Knowledge, attitude, and practice regarding postoperative delirium among cardiac surgery nurses: A cross-sectional multi-centre study. J Clin Nurs 2023;32:5046-55. [Crossref] [PubMed]
- Li T, Li J, Yuan L, et al. Effect of Regional vs General Anesthesia on Incidence of Postoperative Delirium in Older Patients Undergoing Hip Fracture Surgery: The RAGA Randomized Trial. JAMA 2022;327:50-8. [Crossref] [PubMed]
- Patel M, Onwochei DN, Desai N. Influence of perioperative dexmedetomidine on the incidence of postoperative delirium in adult patients undergoing cardiac surgery. Br J Anaesth 2022;129:67-83. [Crossref] [PubMed]
- ChSECC. White book of Chinese cardiovascular surgery and extracorporeal circulation in 2022. Chin J ECC 2022;21. Available online: https://link.cnki.net/doi/10.13498/j.cnki.chin.j.ecc.2023.04.2
- Cai S, Li J, Gao J, et al. Prediction models for postoperative delirium after cardiac surgery: Systematic review and critical appraisal. Int J Nurs Stud 2022;136:104340. [Crossref] [PubMed]
- Igwe EO, Nealon J, O'Shaughnessy P, et al. Incidence of postoperative delirium in older adults undergoing surgical procedures: A systematic literature review and meta-analysis. Worldviews Evid Based Nurs 2023;20:220-37. [Crossref] [PubMed]
- Wiredu K, Mueller A, McKay TB, et al. Sex Differences in the Incidence of Postoperative Delirium after Cardiac Surgery: A Pooled Analyses of Clinical Trials. Anesthesiology 2023;139:540-2. [Crossref] [PubMed]
- DeBolt CL, Gao Y, Sutter N, et al. The association of post-operative delirium with patient-reported outcomes and mortality after lung transplantation. Clin Transplant 2021;35:e14275. [Crossref] [PubMed]
- Moskowitz EE, Overbey DM, Jones TS, et al. Post-operative delirium is associated with increased 5-year mortality. Am J Surg 2017;214:1036-8. [Crossref] [PubMed]
- Tse L, Schwarz SK, Bowering JB, et al. Incidence of and Risk Factors for Delirium After Cardiac Surgery at a Quaternary Care Center: A Retrospective Cohort Study. J Cardiothorac Vasc Anesth 2015;29:1472-9. [Crossref] [PubMed]
- Brown CH 4th, Max L, LaFlam A, et al. The Association Between Preoperative Frailty and Postoperative Delirium After Cardiac Surgery. Anesth Analg 2016;123:430-5. [Crossref] [PubMed]
- Kotfis K, Szylińska A, Listewnik M, et al. Early delirium after cardiac surgery: an analysis of incidence and risk factors in elderly (≥65 years) and very elderly (≥80 years) patients. Clin Interv Aging 2018;13:1061-70. [Crossref] [PubMed]
- Koster S, Hensens AG, Schuurmans MJ, et al. Prediction of delirium after cardiac surgery and the use of a risk checklist. Eur J Cardiovasc Nurs 2013;12:284-92. [Crossref] [PubMed]
- de la Varga-Martínez O, Gómez-Pesquera E, Muñoz-Moreno MF, et al. Development and validation of a delirium risk prediction preoperative model for cardiac surgery patients (DELIPRECAS): An observational multicentre study. J Clin Anesth 2021;69:110158. [Crossref] [PubMed]
- Kumar AK, Jayant A, Arya VK, et al. Delirium after cardiac surgery: A pilot study from a single tertiary referral center. Ann Card Anaesth 2017;20:76-82. [Crossref] [PubMed]
- Price CC, Garvan C, Hizel LP, et al. Delayed Recall and Working Memory MMSE Domains Predict Delirium following Cardiac Surgery. J Alzheimers Dis 2017;59:1027-35. [Crossref] [PubMed]
- Bukhbinder AS, Hinojosa M, Harris K, et al. Population-Based Mini-Mental State Examination Norms in Adults of Mexican Heritage in the Cameron County Hispanic Cohort. J Alzheimers Dis 2023;92:1323-39. [Crossref] [PubMed]
- Feeney J, Savva GM, O'Regan C, et al. Measurement Error, Reliability, and Minimum Detectable Change in the Mini-Mental State Examination, Montreal Cognitive Assessment, and Color Trails Test among Community Living Middle-Aged and Older Adults. J Alzheimers Dis 2016;53:1107-14. [Crossref] [PubMed]
- Greaves D, Psaltis PJ, Davis DHJ, et al. Risk Factors for Delirium and Cognitive Decline Following Coronary Artery Bypass Grafting Surgery: A Systematic Review and Meta-Analysis. J Am Heart Assoc 2020;9:e017275. [Crossref] [PubMed]
- Hirsch J, DePalma G, Tsai TT, et al. Impact of intraoperative hypotension and blood pressure fluctuations on early postoperative delirium after non-cardiac surgery. Br J Anaesth 2015;115:418-26. [Crossref] [PubMed]
- Iizuka Y, Yoshinaga K, Takahashi K, et al. Association between Plasma Ascorbic Acid Levels and Postoperative Delirium in Older Patients Undergoing Cardiovascular Surgery: A Prospective Observational Study. J Cardiovasc Dev Dis 2023;10:293. [Crossref] [PubMed]
- Meng L, Xiao J, Gudelunas K, et al. Association of intraoperative cerebral and muscular tissue oxygen saturation with postoperative complications and length of hospital stay after major spine surgery: an observational study. Br J Anaesth 2017;118:551-62. [Crossref] [PubMed]
- Cai S, Latour JM, Lin Y, et al. Preoperative cardiac function parameters as valuable predictors for nurses to recognise delirium after cardiac surgery: A prospective cohort study. Eur J Cardiovasc Nurs 2020;19:310-9. [Crossref] [PubMed]
- Enomoto K, Kosaka S, Kimura T, et al. Prevention of postoperative delirium after cardiovascular surgery: A team-based approach. J Thorac Cardiovasc Surg 2023;165:1873-1881.e2. [Crossref] [PubMed]
- Rubin FH, Neal K, Fenlon K, et al. Sustainability and scalability of the hospital elder life program at a community hospital. J Am Geriatr Soc 2011;59:359-65. [Crossref] [PubMed]
- O'Gara BP, Mueller A, Gasangwa DVI, et al. Prevention of Early Postoperative Decline: A Randomized, Controlled Feasibility Trial of Perioperative Cognitive Training. Anesth Analg 2020;130:586-95. [Crossref] [PubMed]
- Xue X, Wang P, Wang J, et al. Preoperative individualized education intervention reduces delirium after cardiac surgery: a randomized controlled study. J Thorac Dis 2020;12:2188-96. [Crossref] [PubMed]
- van der Zanden V, Beishuizen SJ, Swart LM, et al. The Effect of Treatment of Anemia with Blood Transfusion on Delirium: A Systematic Review. J Am Geriatr Soc 2017;65:728-37. [Crossref] [PubMed]
- Olofsson B, Stenvall M, Lundström M, et al. Malnutrition in hip fracture patients: an intervention study. J Clin Nurs 2007;16:2027-38. [Crossref] [PubMed]
- Aldecoa C, Bettelli G, Bilotta F, et al. Update of the European Society of Anaesthesiology and Intensive Care Medicine evidence-based and consensus-based guideline on postoperative delirium in adult patients. Eur J Anaesthesiol 2024;41:81-108. [Crossref] [PubMed]