Predictive risk score for isolated brain metastasis in non-small cell lung cancer
Highlight box
Key findings
• Development of a risk score for the probability of synchronous isolated brain metastasis (IBM) in patients presenting with non-small cell lung cancer (NSCLC).
What is known and what is new?
• Brain metastasis is common and currently clinical stage alone is used to justify additional brain imaging during work-up.
• This study identifies salient features associated with synchronous IBM and the risk score can aid in determining if additional brain imaging is warranted.
What is the implication, and what should change now?
• By utilizing the risk score developed in this study in clinical practice, there is now provided an evidence-based method to identify patients with NSCLC who require dedicated brain imaging in the absence of other metastatic disease.
Introduction
Lung cancer is the leading cause of cancer deaths in the United States with non-small cell lung cancer (NSCLC) the most common type (1,2). The brain is the most common site for distant metastasis, with isolated brain metastasis (IBM) accounting for 20% (3). Early detection of synchronous brain metastases is critical for patient care and can facilitate treatment. Aggressive treatment of both IBM and primary NSCLC has favorable outcomes comparable to treatment of NSCLC without brain metastases (4). However, algorithms to determine the likelihood of synchronous IBM (S-IBM) are not well established. Here we define IBM as the occurrence of one or more brain lesions without evidence of extracranial metastasis; compared to solitary brain metastasis (one brain lesions without evidence of extracranial metastasis) or single brain metastasis (one brain lesions with at least one other site of extracranial disease).
Current guidelines recommend obtaining brain imaging starting at clinical stage IIA (T2bN0) to assess for synchronous metastasis; obtaining brain imaging for clinical stage IB (T2aN0) is optional and considered for select patients who are high risk (5). These recommendations are based on limited evidence. The risk factors for synchronous and the development of brain metastasis in NSCLC has been previously described. Waqar utilized the National Cancer Database (NCDB) from 2010–2012 and identified age, histology, tumor size, grade, and nodal status as significant risk factors (6). However, they did not distinguish between IBM and brain metastasis with other concurrent metastatic sites. Another study found tumor diameter, calcium, and carcinoembryonic antigen (CEA) to be significant risk factors for screening for brain metastasis in NSCLC (7). Another identified increasing tumor size, increasing lymph node stage, adenocarcinoma, and undifferentiated cancer cell types of primary lesion as risk factors for brain metastases at initial presentation of NSCLC (8). However, both studies were limited by small sample of patients with brain metastases (N=57 and N=95 respectively). Several studies have investigated the risk factors for developing metachronous brain metastases in NSCLC. One found locally advanced NSCLC, non-squamous cell carcinoma type (especially adenocarcinoma), elevated CEA level, and lymph node metastases (especially multiple mediastinal lymph nodes) are independent risk factors for developing brain metastases (9). This may suggest that locally advanced NSCLC or having multiple risk factors at the time of initial presentation may warrant brain imaging prior to treatment. Conversely, some patients are felt to be low risk for intracranial disease and do not require preoperative imagining. One demonstrated no survival benefit associated with preoperative brain magnetic resonance imaging (MRI) among stage IA NSCLC patients receiving lung resection (10). We believe the risks of S-IBM in NSCLC remains poorly defined as are the clinical scenarios in which preoperative brain imaging is appropriate. To this researcher’s knowledge, there has not been a study that has developed a predictive risk score for S-IBM in NSCLC, making this a novel investigation.
The purpose of this study was to identify the risk factors associated with S-IBM in NSCLC using the NCDB. By performing a retrospective evaluation of patients in the NCDB, we aimed to identify the most salient demographic and clinical factors for developing brain metastasis in the absence of other metastatic disease [i.e., that would have been detected on pre-operative fludeoxyglucose-18 positron emission tomography (FDG-PET)]. We hypothesized there would be discrete clinical and demographic factors associated with S-IBM and these factors could be used to produce a predictive risk score. We present this article in accordance with the STROBE reporting checklist (available at https://jtd.amegroups.com/article/view/10.21037/jtd-23-1668/rc) (11).
Methods
NCDB
This study was performed using the NCDB, a hospital-based tumor registry. The NCDB captures data from hospitals that are accredited by the American College of Surgeons Commission on Cancer (CoC) and includes more than 70% of all newly diagnosed cancer cases nationwide. The NCDB is a joint project of the CoC between the American College of Surgeons and the American Cancer Society. The CoC’s NCDB and the hospitals participating in the CoC NCDB were the source of the de-identified data used in this study. They have not verified and aren’t responsible for the statistical validity of the data analysis of the conclusions derived by the authors.
Study population and variables
The 2020 iteration [2016–2020] of the NCDB was queried and inclusion criteria was all adult patients with NSCLC. Patients were excluded if they were younger than 18 years of age, had documented metastatic disease to any other organ system (bone, liver, lung, or “other”), or was missing critical information. Patients were then primarily categorized as cM0 or IBM (Figure 1).
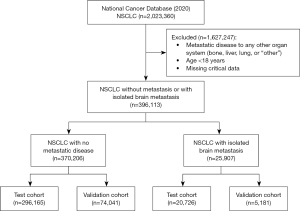
Other patient variables included in our analysis were age, sex, race, type of institution, Charlson/Deyo score, and socioeconomic status (as a product of income and rate of high school graduation). Disease characteristics included clinical T (cT) stage, clinical N (cN) stage, histology (adenocarcinoma, squamous cell carcinoma, or other), and tumor grade (well differentiated, moderately differentiated, poorly differentiated, undifferentiated, or unknown).
Statistical analysis
The cohort of patients was randomly divided into 2 groups: 80% of patients were used as a “test” cohort to develop a risk model, and the remaining 20% were used as a “validation” cohort of the risk model. Using the test cohort, we first performed statistical comparison of demographic and clinical factors between the two groups (no brain metastasis vs. IBM) using the Wilcoxon rank sum test for continuous variables and Chi-square for categorical data. Demographics and clinical characteristics are described using median and interquartile range (IQR) for continuous variables, and frequency and percentages for categorical variables. To determine any independent association of these factors on the occurrence of IBM, we also performed multivariable logistic regression. Covariates for the logistic regression were based on backwards selection from bivariate modeling. This logistic model was used to perform marginal standardization analysis to estimate the actual risk of IBM for various clinical scenarios. We then created a risk score to estimate the risk of IBM using the nomolog plugin for STATA (12). The nomogram values were rounded to the nearest integer and described as the “risk score”. We performed a receiver operating characteristic (ROC) analysis of the risk score (on both the test and the validation cohorts) and reported the accuracy of the integer rounded risk score as the area under the curve (AUC) or “c-statistic”. The relationship between risk score and rate of IBM was determined using marginal standardization analysis.
Statistical analysis was performed using STATA MP (Version 17.0, College Station, TX, USA). Statistical significance was set at a P value ≤0.05. The study was determined to be exempt from Institution Review Board review because all the data was de-identified. The study was conducted in accordance with the Declaration of Helsinki (as revised in 2013).
Results
Our study population consisted of 396,113 patients: 25,907 patients with IBM and 370,206 patients with no metastatic disease. The study population was randomly divided into test and validation cohorts, which were similar. Among the test cohort, IBM was associated with several demographic and clinical characteristics in bivariate comparison (Table 1). Bivariate analysis demonstrated that age, histology, grade, cT stage, and cN stage were all statistically significant factors associated with IBM.
Table 1
Characteristics | No metastasis | Isolated brain metastasis | P value |
---|---|---|---|
Age (years) | 70 [18–90] | 66 [18–90] | <0.001 |
Male (versus female) | 179,040 (48.4) | 12,953 (50.0) | <0.001 |
Caucasian | 306,587 (82.8) | 20,098 (77.6) | <0.001 |
Insurance status | <0.001 | ||
Not insured | 4,977 (1.3) | 910 (3.5) | |
Private insurance | 79,273 (21.4) | 7,285 (28.1) | |
Medicaid | 23,270 (6.3) | 3,181 (12.3) | |
Medicare | 250,211 (67.6) | 13,602 (52.5) | |
Other | 8,842 (2.4) | 599 (2.3) | |
Unknown | 3,633 (1.0) | 330 (1.3) | |
Academic program | 195,274 (52.7) | 14,231 (54.9) | <0.001 |
Histology | <0.001 | ||
Adenocarcinoma | 190,068 (51.3) | 18,494 (71.4) | |
Squamous carcinoma | 128,569 (34.7) | 3,796 (14.7) | |
Other | 51,569 (13.9) | 3,617 (14.0) | |
Grade | <0.001 | ||
Well-differentiated | 29,319 (7.9) | 356 (1.4) | |
Moderately differentiated | 72,926 (19.7) | 2,246 (8.7) | |
Poorly differentiated | 67,908 (18.3) | 6,443 (24.9) | |
Undifferentiated | 1,539 (0.4) | 170 (0.7) | |
Cannot be assessed/unknown | 198,514 (53.6) | 16,692 (64.4) | |
Clinical T-stage | <0.001 | ||
cT1 | 113,464 (30.6) | 3,157 (12.2) | |
cT2 | 54,726 (14.8) | 4,887 (18.9) | |
cT3 | 27,523 (7.4) | 2,919 (11.3) | |
cT4 | 22,307 (6.0) | 2,764 (10.7) | |
Missing | 152,186 (41.1) | 12,180 (47.0) | |
Clinical N-stage | <0.001 | ||
cN0 | 157,785 (42.6) | 4,664 (18.0) | |
cN1 | 15,422 (4.2) | 1,764 (6.8) | |
cN2 | 39,400 (10.6) | 5,853 (22.6) | |
cN3 | 12,809 (3.5) | 2,132 (8.2) | |
Missing | 144,790 (39.1) | 11,494 (44.4) | |
Charlson/Deyo comorbidity score | <0.001 | ||
0 | 203,991(55.1) | 16,632 (64.2) | |
1 | 91,024 (24.6) | 5,275 (20.4) | |
2 | 40,938 (11.1) | 2,212 (8.5) | |
≥3 | 34,253 (9.3) | 1,788 (6.9) |
Data shown includes median and interquartile range for continuous variables or n (%) for categorical variables. Differences calculated using chi-square or rank sum test.
Using variables associated with IBM in bivariate comparison, we performed a multivariable logistic regression to determine which factors were independently associated with IBM (Table 2). This analysis showed younger age groups were independently associated with higher risk of IBM. Increasing tumor grade was also associated with IBM. With adenocarcinoma as reference, squamous carcinoma [odds ratio (OR) =0.23, 95% confidence interval (CI): 0.22–0.24] and other histology (OR =0.69, 95% CI: 0.65–0.73) were less likely to demonstrate IBM. As with bivariate analysis, increasing cT stage and cN stage were both associated with increased risk of IBM.
Table 2
Characteristic | Odds ratio | 95% confidence interval | P value |
---|---|---|---|
Age group (years) | |||
18–49 | 1.27 | 1.14–1.42 | <0.001 |
50–59 | 1.30 | 1.23–1.38 | <0.001 |
60–69 | 1.00 | ||
70–79 | 0.68 | 0.64–0.71 | <0.001 |
80–89 | 0.52 | 0.49–0.56 | <0.001 |
≥90 | 0.38 | 0.29–0.49 | <0.001 |
Male gender | 1.05 | 1.01–1.10 | 0.013 |
Charlson/Deyo comorbidity score | |||
0 | 1.00 | ||
1 | 0.79 | 0.74–0.83 | <0.001 |
2 | 0.78 | 0.72–0.85 | <0.001 |
≥3 | 0.79 | 0.72–0.87 | <0.001 |
Histology | |||
Adenocarcinoma | 1.00 | ||
Squamous cell carcinoma | 0.23 | 0.22–0.24 | <0.001 |
Other | 0.69 | 0.65–0.73 | <0.001 |
Grade | |||
Well-differentiated | 1.00 | ||
Moderately differentiated | 2.34 | 1.97–2.80 | <0.001 |
Poorly differentiated | 4.37 | 3.69–5.17 | <0.001 |
Undifferentiated | 4.48 | 3.41–5.88 | <0.001 |
Grade cannot be assessed/unknown | 4.95 | 4.18–5.86 | <0.001 |
Clinical stage group | |||
cT1N0 | 1.00 | ||
cT1N1 | 5.72 | 4.98–6.56 | <0.001 |
cT1N2 | 6.93 | 6.26–7.67 | <0.001 |
cT1N3 | 7.43 | 6.44–8.56 | <0.001 |
cT2N0 | 4.16 | 3.82–4.53 | <0.001 |
cT2N1 | 11.2 | 10.0–12.6 | <0.001 |
cT2N2 | 11.4 | 10.3–12.5 | <0.001 |
cT2N3 | 10.1 | 8.85–11.6 | <0.001 |
cT3N0 | 5.50 | 4.94–6.11 | <0.001 |
cT3N1 | 8.62 | 7.46–9.95 | <0.001 |
cT3N2 | 11.3 | 10.2–12.5 | <0.001 |
cT3N3 | 10.0 | 8.61–11.6 | <0.001 |
cT4N0 | 6.25 | 5.55–7.04 | <0.001 |
cT4N1 | 9.98 | 8.44–11.8 | <0.001 |
cT4N2 | 11.5 | 10.4–12.7 | <0.001 |
cT4N3 | 13.1 | 11.4–15.0 | <0.001 |
To demonstrate the clinical relevance of this analysis, we performed a margin estimation analysis, which estimates the risk of IBM for each combination of clinical tumor and nodal staging categories (Table 3). As expected, the rate of IBM increased with increasing cT stage and cN stage. Given the strong association of IBM with histology type, we also performed subgroup estimates for patients with adenocarcinoma and squamous cell cancer separately (Table 4).
Table 3
Clinical stage group | Predictive margin, % | 95% confidence interval (%) |
---|---|---|
cT1N0 | 0.96 | 0.89–1.03 |
cT1N1 | 5.24 | 4.62–5.85 |
cT1N2 | 6.30 | 5.79–6.77 |
cT1N3 | 6.70 | 5.90–7.49 |
cT2N0 | 3.90 | 3.63–4.11 |
cT2N1 | 9.80 | 8.99–10.6 |
cT2N2 | 9.90 | 9.31–10.48 |
cT2N3 | 8.92 | 8.00–9.83 |
cT3N0 | 5.05 | 4.62–5.47 |
cT3N1 | 7.70 | 6.75–8.64 |
cT3N2 | 9.85 | 9.18–10.5 |
cT3N3 | 8.83 | 7.80–9.86 |
cT4N0 | 5.70 | 5.16–6.24 |
cT4N1 | 8.80 | 7.56–10.0 |
cT4N2 | 9.98 | 9.32–10.7 |
cT4N3 | 11.3 | 10.1–12.4 |
Data are presented as predicted percent values and 95% confidence interval for isolated brain metastasis.
Table 4
Clinical stage group | Adenocarcinoma | Squamous cell cancer | |||
---|---|---|---|---|---|
Predictive margin, % | 95% confidence interval, % | Predictive margin, % | 95% confidence interval, % | ||
cT1N0 | 1.90 | 1.76–2.05 | 0.44 | 0.40–0.48 | |
cT1N1 | 9.80 | 8.75–10.8 | 2.50 | 2.15–2.78 | |
cT1N2 | 11.6 | 10.8–12.4 | 3.00 | 2.69–3.25 | |
cT1N3 | 12.3 | 11.0–13.6 | 3.20 | 2.76–3.60 | |
cT2N0 | 7.40 | 6.92–7.82 | 1.80 | 1.66–1.96 | |
cT2N1 | 17.3 | 16.0–18.6 | 4.70 | 4.27–5.14 | |
cT2N2 | 17.5 | 16.6–18.3 | 4.80 | 4.40–5.11 | |
cT2N3 | 15.9 | 14.5–17.4 | 4.30 | 3.78–4.76 | |
cT3N0 | 9.40 | 8.71–10.2 | 2.40 | 2.14–2.60 | |
cT3N1 | 13.9 | 12.4 - 15.5 | 3.70 | 3.17–4.15 | |
cT3N2 | 17.3 | 16.4–18.4 | 4.70 | 4.35–5.11 | |
cT3N3 | 15.8 | 14.2–17.4 | 4.20 | 3.68–4.77 | |
cT4N0 | 10.6 | 9.70–11.5 | 2.70 | 2.40–2.97 | |
cT4N1 | 15.7 | 13.7–17.7 | 4.20 | 3.58–4.84 | |
cT4N2 | 17.6 | 16.5–18.7 | 4.80 | 4.42–5.18 | |
cT4N3 | 19.5 | 17.8–21.3 | 5.40 | 4.83–6.05 |
Using the logistic regression from Table 2, we created a scoring system to determine the risk of IBM. This scoring system used cT stage, cN stage, age, comorbidity, histology, and tumor grade, as shown in Table 5. ROC analysis showed that the scoring system has excellent discrimination of IBM in the test cohort (AUC =0.806). We then applied the scoring system to the separate validation cohort, which also showed excellent discrimination in ROC analysis (AUC =0.805). The relationship of risk score and predicted risk of IBM is shown in Table 6.
Table 5
Characteristic | Score |
---|---|
Clinical T-stage | |
cT1 | 0 |
cT2 | 6 |
cT3 | 6 |
cT4 | 7 |
Clinical N-stage | |
cN0 | 0 |
cN1+ | 7 |
Age group (years) | |
18–49 | 6 |
50–59 | 7 |
60–69 | 5 |
70–79 | 2 |
80–89 | 1 |
≥90 | 0 |
Charlson/Deyo comorbidity score | |
0 | 1 |
≥1 | 0 |
Histology | |
Adenocarcinoma | 9 |
Squamous carcinoma | 0 |
Other | 7 |
Grade | |
Well-differentiated | 0 |
Moderately differentiated | 5 |
Undifferentiated | 9 |
Grade cannot be assessed/unknown | 10 |
Table 6
Score | Predicted rate, % | Lower 95% CI, % | Upper 95% CI, % |
---|---|---|---|
10 | 0.3 | 0.1 | 0.5 |
11 | 0.4 | 0.2 | 0.5 |
12 | 0.4 | 0.2 | 0.5 |
13 | 0.8 | 0.5 | 1.0 |
14 | 0.6 | 0.4 | 0.9 |
15 | 0.5 | 0.3 | 0.7 |
16 | 0.8 | 0.6 | 1.0 |
17 | 1.2 | 1.0 | 1.4 |
18 | 1.6 | 1.3 | 1.8 |
19 | 1.6 | 1.3 | 1.8 |
20 | 1.7 | 1.4 | 1.9 |
21 | 2.0 | 1.7 | 2.2 |
22 | 2.4 | 2.1 | 2.6 |
23 | 3.0 | 2.6 | 3.4 |
24 | 3.4 | 3.1 | 3.8 |
25 | 4.0 | 3.7 | 4.3 |
26 | 5.3 | 4.9 | 5.7 |
27 | 6.7 | 6.2 | 7.2 |
28 | 7.5 | 6.9 | 8.0 |
29 | 9.5 | 8.8 | 10.2 |
30 | 9.8 | 9.0 | 10.5 |
31 | 11.7 | 10.9 | 12.5 |
32 | 15.4 | 14.5 | 16.4 |
33 | 16.4 | 15.3 | 17.4 |
34 | 17.0 | 16.0 | 18.0 |
35 | 18.9 | 17.7 | 20.2 |
36 | 20.0 | 18.3 | 21.6 |
37 | 21.0 | 19.6 | 22.4 |
38 | 24.8 | 23.5 | 26.2 |
39 | 26.4 | 24.8 | 28.0 |
40 | 27.2 | 25.3 | 29.2 |
41 | 29.5 | 25.6 | 33.3 |
CI, confidence interval.
Discussion
Current guideline-based recommendations on obtaining brain imaging to assess for synchronous brain metastases in NSCLC is based on limited evidence (5). Additionally, some evidence suggests obtaining preoperative brain imagining in low-risk patients is unnecessary and has shown to demonstrate no survival benefit (10). This study suggests discrete clinicopathologic factors associated with S-IBM. We believe these factors can be used to discriminate patients with elevated risk of IBM.
Accurate clinical staging is imperative for the treatment of NSCLC. Guideline directed care necessitates an accurate understanding of a patient’s burden of disease. Contemporary imaging modalities have improved the accuracy of clinical staging and improved the detection of metastatic disease. Arguably, the largest contribution to improved accuracy in clinical stage is utilization of FDG-PET. Several studies have shown FDG-PET to be useful in diagnosing malignant pulmonary lesions with estimated sensitivity and specificity to be 96.8 and 77.8% respectively, to have superiority in detecting mediastinal lymph node metastases compared to computed tomography (CT) alone, and to be useful in the detection of distant metastasis, particularly bone and adrenal lesions (13,14). Unfortunately, FDG-PET is unable to accurately image the brain due to high physiologic uptake of FDG by brain parenchyma (14). Therefore, additional brain imaging is recommended for select patients. This can increase cost of and time required to accurately stage a patient. This study specifically looks at patients without other metastatic sites, which simulates a situation seen by many surgeons evaluating patients for lung resection. By eliminating unnecessary tests, providers may be able to reduce time from diagnosis to intervention, which has been associated with improved survival among patients receiving lung resection for cancer (15).
The increasing use of medical imaging has contributed to the increased cost of lung cancer work-up and management. As lung cancer is the 2nd most common cancer worldwide, reducing unnecessary testing could have significant impact on health care expenditures (16). The predictive risk score described in this manuscript could be used to identify which patients for whom additional brain imaging is appropriate. Although the decision to perform radiographic studies is a multifactorial clinical decision, this analysis suggests that a score of 23 is a reasonable threshold to trigger dedicated brain imaging. This threshold is based on current guidelines recommending obtaining brain imaging starting at clinical stage IIA, which we estimated a risk of IBM of approximately 7% among patients with adenocarcinoma. Our risk score estimates a score of 22 is associated with a predicted rate of IBM of 2.4% (95% CI: 2.1–2.6%), which we believe is a reasonable acceptable risk to forgo preoperative brain imaging.
This study differs from previous literature on the subject of brain metastasis in several ways. A notable aspect is our investigation of S-IBM while the majority of current studies have investigated the development of metachronous IBM. It is suspected these studies are evaluating risk for developing brain metastasis in order to assess post-treatment progression and survival. However, our motivation is to provide an evidence-based method to systematize work-up and care, especially among patients who are being considered for surgery (i.e., without other extra-thoracic metastasis).
Another area our study differs from previous literature is our evaluation of IBM risk associated with cT and cN stages and our utilization of those stages in our risk score. We found the rate of IBM increased with increasing cT stage and cN stage and allowed our risk score to account for the risk associated with T and N stage combination. The literature varies regarding risk associated with T and N staging as well as utilizing T stage or tumor diameter for study parameters. Multiple studies found an association with increased risk of brain metastases with increased tumor size, with cut-offs ranging from 5–6 cm, while others found no correlation between tumor size or stage and brain metastasis (7-9). Some that utilized T staging found an increase in risk of brain metastasis associated with higher T staging, while other studies have combined the stages in their analyses (17,18). Additionally, one found disagreement regarding risk associated with T stage or tumor size in a literature review and concluded lymph node metastases to be an independent risk factor for brain metastases (9). We believe a system that uses T stage in combination with other factors allows for a more individualized risk assessment.
Another controversial area in the literature is the association of different primary cancer histology types with the risk of brain metastases. Several studies suggest that non-squamous cell carcinoma, especially adenocarcinoma, to be an independent risk factor for brain metastases (6,8,9,17-19). Similarly, our study found a strong association with histology type and IBM with adenocarcinoma being the most prominent and squamous cell having a lower associated risk. However, some studies found no correlation between histology and brain metastases (3,20). This discrepancy is likely attributed to the method of brain metastases assessment and diagnosis. Sørensen et al. demonstrated 29% of those with lung adenocarcinoma with brain metastasis found on autopsy were not diagnosed clinically, suggesting a high rate of under diagnosis (21).
There are many studies that have been published on prediction models for NSCLC, however this study differs by utilizing risk factors to predict the probability of IBM at the time of diagnosis of NSCLC and guide if additional brain imaging is warranted. One review found numerous studies that have developed a risk score or nomogram used to predict survival, prognosis, or lymph node metastasis (22). One commonly used model is the World Health Organization Performance Status (WHO-PS) which is the Eastern Cooperative Oncology Group Performance Status (ECOG-PS) scale which is mainly used to assess prognosis; however, it is based on subjective measurements and can have wide variability between evaluators (22). To this researcher’s knowledge, this is the only study that has produced a risk score to predict synchronous IBM, which could aid clinicians in deciding if additional brain imaging is warranted in questionable cases. Additionally, these previous nomograms were constructed from relatively small sample sizes (ranging from 192 to 535), compared to this study with a sample size of 396,113 patients with 25,907 of those patients with IBM (22).
This study is similar to previous literature regarding brain metastases in finding that younger age is associated with risk of IBM. We found age less than 60 years had increased occurrence of IBM. Multiple studies also found patient age of younger than 60–70 years was associated with risk of brain metastases (3,9,17,20). Additionally, we found a decrease in occurrence of IBM with increasing age. Similarly, Dimitropoulos et al. found the incidence of brain metastases was reduced with increasing age (20). It is unclear why younger age is associated with higher brain metastases risk, it has been suggested that it may be due to cerebrovascular microenvironments, differences in expression of biomarkers, or better performance statuses and associated longer survival in young patients (9).
Limitations
This study has several limitations. The use of a large retrospective database is limited by its accuracy and has not been adjudicated. Specifically, we cannot guarantee the accuracy of clinical stage reported in the data or know the technique used to define it. This database also lacks other important clinical information such as patient symptoms, which could strongly associate with the presence of metastasis and might alter the accuracy or utility of our risk score. Furthermore, we do not have any information about how brain metastases were evaluated, including whether institutions routinely offered head CT or brain MRI. This may have affected the accuracy of the clinical stage. Another limitation is inherent to risk score methodology, which may under or overestimate the risk of metastasis due to the effect of variables not included in the model.
There may exist several factors associated with metastasis not included in the analysis, one of them being genetic changes. Molecular testing has recently become critical in the management of NSCLC with the detection of mutations and translocations (EGFR, BRAF, ALK, ROS1 for some examples) that have led to targeted therapy (23). This database lacked information regarding molecular testing and could not be included in the analysis, however it is an area that future investigation in could lead to refinement and expansion of utilization of the risk score. Genetic changes and other unmeasured variables may affect the components of the risk score and its generalizability.
Conclusions
The risk score presented here integrates clinical and pathologic features to provide an estimate of risk of IBM at the time of workup. This may facilitate individualized evaluation of patients with NSCLC and reduce cost associated with accurately defining patient clinical stage.
Acknowledgments
Funding: None.
Footnote
Reporting Checklist: The authors have completed the STROBE reporting checklist. Available at https://jtd.amegroups.com/article/view/10.21037/jtd-23-1668/rc
Peer Review File: Available at https://jtd.amegroups.com/article/view/10.21037/jtd-23-1668/prf
Conflicts of Interest: All authors have completed the ICMJE uniform disclosure form (available at https://jtd.amegroups.com/article/view/10.21037/jtd-23-1668/coif). D.S.B. receives consulting fees from Novartis, Bristol-Myers Squibb, Mirati Therapeutics, Eli Lilly, AstraZeneca, Syneos Health, Amgen, and Daiichi Sankyo and independent research funding from AstraZeneca. Philip Linden receives grant funding from Zimmer Biomet. C.W.T. is a consultant for Atricure, Bristol Myers Sqibb, AstraZeneca and Zimmer Biomet. He has also received grant funding from Zimmer Biomet and research support from Medtronic. The other authors have no conflicts of interest to declare.
Ethical Statement: The authors are accountable for all aspects of the work in ensuring that questions related to accuracy or integrity of any part of the work are appropriately investigated and resolved. The study was determined to be exempt from Institution Review Board review because all the data was de-identified. The study was conducted in accordance with the Declaration of Helsinki (as revised in 2013).
Open Access Statement: This is an Open Access article distributed in accordance with the Creative Commons Attribution-NonCommercial-NoDerivs 4.0 International License (CC BY-NC-ND 4.0), which permits the non-commercial replication and distribution of the article with the strict proviso that no changes or edits are made and the original work is properly cited (including links to both the formal publication through the relevant DOI and the license). See: https://creativecommons.org/licenses/by-nc-nd/4.0/.
References
- Siegel RL, Miller KD, Fuchs HE, et al. Cancer statistics, 2022. CA Cancer J Clin 2022;72:7-33. [Crossref] [PubMed]
- American Lung Association. (n.d.). Lung Cancer Additional Measures - Lung Cancer Trends Brief. Available online: https://www.lung.org/research/trends-in-lung-disease/lung-cancer-trends-brief/lung-cancer-additional-measures
- Ceresoli GL, Reni M, Chiesa G, et al. Brain metastases in locally advanced nonsmall cell lung carcinoma after multimodality treatment: risk factors analysis. Cancer 2002;95:605-12. [Crossref] [PubMed]
- Hu C, Chang EL, Hassenbusch SJ 3rd, et al. Nonsmall cell lung cancer presenting with synchronous solitary brain metastasis. Cancer 2006;106:1998-2004. [Crossref] [PubMed]
- National Comprehensive Cancer Network. Non-small Cell Lung Cancer (Version 5.2022-September 26, 2022). Available online: https://www.nccn.org/professionals/physician_gls/pdf/nscl.pdf
- Waqar SN, Samson PP, Robinson CG, et al. Non-small-cell Lung Cancer With Brain Metastasis at Presentation. Clin Lung Cancer 2018;19:e373-9. [Crossref] [PubMed]
- He J, Wang X, Xiao R, et al. Risk factors for brain metastases from non-small-cell lung cancer: A protocol for observational study. Medicine (Baltimore) 2021;100:e24724. [Crossref] [PubMed]
- Mujoomdar A, Austin JH, Malhotra R, et al. Clinical predictors of metastatic disease to the brain from non-small cell lung carcinoma: primary tumor size, cell type, and lymph node metastases. Radiology 2007;242:882-8. [Crossref] [PubMed]
- An N, Jing W, Wang H, et al. Risk factors for brain metastases in patients with non-small-cell lung cancer. Cancer Med 2018;7:6357-64. [Crossref] [PubMed]
- Nam JG, Hong H, Choi SH, et al. No Prognostic Impact of Staging Brain MRI in Patients with Stage IA Non-Small Cell Lung Cancer. Radiology 2022;303:632-43. [Crossref] [PubMed]
- von Elm E, Altman DG, Egger M, et al. The Strengthening the Reporting of Observational Studies in Epidemiology (STROBE) Statement: guidelines for reporting observational studies. Int J Surg 2014;12:1495-9. [Crossref] [PubMed]
- Zlotnik A, Abraira V. A General-purpose Nomogram Generator for Predictive Logistic Regression Models. Stata J 2015;15:537-46. [Crossref]
- Cuaron J, Dunphy M, Rimner A. Role of FDG-PET scans in staging, response assessment, and follow-up care for non-small cell lung cancer. Front Oncol 2012;2:208. [PubMed]
- Farsad M. FDG PET/CT in the Staging of Lung Cancer. Curr Radiopharm 2020;13:195-203. [Crossref] [PubMed]
- Cao L, Linden PA, Biswas T, et al. Modeling the COVID Pandemic: Do Delays in Surgery Justify Using Stereotactic Radiation to Treat Low-Risk Early Stage Non-Small Cell Lung Cancer? J Surg Res 2023;283:532-9. [Crossref] [PubMed]
- WCRF International. (2022, April 14). Lung cancer statistics | World Cancer Research Fund International. Available online: https://www.wcrf.org/cancer-trends/lung-cancer-statistics/
- Bajard A, Westeel V, Dubiez A, et al. Multivariate analysis of factors predictive of brain metastases in localised non-small cell lung carcinoma. Lung Cancer 2004;45:317-23. [Crossref] [PubMed]
- Won YW, Joo J, Yun T, et al. A nomogram to predict brain metastasis as the first relapse in curatively resected non-small cell lung cancer patients. Lung Cancer 2015;88:201-7. [Crossref] [PubMed]
- Li J. Risk factor of brain metastases and its influence on patient prognosis after complete resection of non-small cell lung cancer. Am J Transl Res 2022;14:406-13. [PubMed]
- Dimitropoulos C, Hillas G, Nikolakopoulou S, et al. Prophylactic cranial irradiation in non-small cell lung cancer patients: who might be the candidates? Cancer Manag Res 2011;3:287-94. [PubMed]
- Sørensen JB, Hansen HH, Hansen M, et al. Brain metastases in adenocarcinoma of the lung: frequency, risk groups, and prognosis. J Clin Oncol 1988;6:1474-80. [Crossref] [PubMed]
- Wang Y, Lin X, Sun D. A narrative review of prognosis prediction models for non-small cell lung cancer: what kind of predictors should be selected and how to improve models? Ann Transl Med 2021;9:1597. [Crossref] [PubMed]
- Imyanitov EN, Iyevleva AG, Levchenko EV. Molecular testing and targeted therapy for non-small cell lung cancer: Current status and perspectives. Crit Rev Oncol Hematol 2021;157:103194. [Crossref] [PubMed]