A novel amino acid metabolism-related gene signature to predict the overall survival of esophageal squamous cell carcinoma patients
Highlight box
Key findings
• The amino acid metabolism-related gene (AAMRG) signature could be used to predict the prognosis of esophageal squamous cell carcinoma (ESCC) patients.
What is known, and what is new?
• An effective prognostic predictor of clinical outcomes is urgently required in ESCC treatment.
• Two AAMRGs [i.e., branched-chain amino acid transaminase 1 (BCAT1) and methylmalonic aciduria and homocystinuria type C protein (MMACHC)] were selected to develop a novel AAMRG-related gene predictor of 1- and 2-year prognostic risk in ESCC patients.
What is the implication, and what should change now?
• Our prognostic nomogram that incorporates clinical factors and BCAT1 and MMACHC gene expression showed good prognostic performance for ESCC.
Introduction
Esophageal carcinoma (EC) has a high incidence rate worldwide. With an overall 5-year survival rate ranging from 15% to 25% worldwide, EC is characterized with a high malignancy and poor prognosis (1). As the main type of EC, esophageal squamous cell carcinoma (ESCC) is known for having a low early diagnosis rate, a poor prognosis, and a low survival rate. The poor outcomes of ESCC are closely related to inconspicuous clinical symptoms in the early stage, early metastasis, and easy recurrence (2). Thus, effective prognostic markers are urgently needed to be identified to predict ESCC treatment outcomes.
Cellular metabolism reprogramming is a common feature in the progress of tumorigenesis (3-5). Besides glucose metabolism disorder, the deregulated uptake of amino acids is also a hallmark of cancer-associated metabolic changes (6,7). Amino acids are vital to support the survival and biosynthesis of mammalian cancer cells (8,9). There is accumulating evidence of a strong relationship among cancer cell growth and glutamine, serine, and glycine (7). Increased glutamine metabolism is a common metabolic alteration in cancer (10). As a potential nutrient, glutamine is considered second only to glucose in cancer in terms of it being a source of nitrogen and carbon (11). The oncogenic transcription factor (TF) Myc activates glutaminase and further regulates glutamine metabolism, and energy and reactive oxygen species homeostasis in lymphoma cells and prostate cancer cells (12). Research has also shown that proline metabolism is involved in the significant tumorigenicity and poor differentiation of ESCC (13). However, the relationship between amino acid metabolism and the prognostic outcomes of ESCC patients requires further investigation.
In this study, we explored the association between amino acid metabolism-related genes (AAMRGs) and the prognosis of ESCC patients based on data from The Cancer Genome Atlas (TCGA) database. Two differentially expressed AAMRGs [i.e., branched-chain amino acid transaminase 1 (BCAT1) and methylmalonic aciduria and homocystinuria type C protein (MMACHC)] were identified using data from TCGA and the Gene Expression Omnibus (GEO) databases, and ESCC transcriptome data from our previous research, and validated using the real-time quantitative polymerase chain reaction (RT-qPCR) method (14). The tumor subtypes of the two AAMRGs (i.e., BCAT1 and MMACHC) were evaluated by a survival analysis using Kaplan-Meier (K-M) curves. A nomogram based on the two AAMRGs (BCAT1 and MMACHC) and clinical information was further developed to predict ESCC patient survival at 1 and 2 years. A decision curve analysis (DCA) was used to evaluate the predictive efficacy of the nomogram model. We present this article in accordance with the TRIPOD reporting checklist (available at https://jtd.amegroups.com/article/view/10.21037/jtd-24-818/rc).
Methods
Data acquisition and processing
TCGA-ESCC expression matrix dataset was downloaded from TCGA dataset (https://www.cancer.gov/ccg/research/genome-sequencing/tcga), and samples with incomplete overall survival (OS) prognostic information were excluded. In total, 88 ESCC samples were included in the analysis. In addition, the expression matrix and clinical data of 653 cases of normal samples of Genotype-Tissue Expression (GTEx) esophageal tissue from the UCSC Xena database (http://Genome.ucsc.edu) were obtained and normalized into the format of fragments per kilobase per million. The count sequencing data of TCGA-ESCC dataset were normalized using the limma R package. As Table 1 shows, the GSE20347 dataset comprised the gene expression profile data of 17 ESCC tissues and adjacent tissues, while the GSE67269 dataset comprised the data of 73 ESCC tissues and adjacent tissues (15,16). The sva R package was used to standardize the GSE20347 and GSE67269 ESCC datasets, which served as the verification dataset. The expression profile data of 6 ESCC tissues and adjacent tissues from previous study by our laboratory team were used as an ESCC dataset (14).
Table 1
Items | GSE20347 | GSE67269 |
---|---|---|
Platform | Gpl571 | Gpl571 |
Sequencing type | Expression profiling by array | Expression profiling by array |
Species | Homo sapiens | Homo sapiens |
Disease | ESCC | ESCC |
Tissue | Esophageal | Esophageal |
Samples in disease group | 17 | 73 |
Samples in control group | 17 | 73 |
Reference | 20955586 | 32375686 |
ESCC, esophageal squamous cell carcinoma.
The GeneCards database (http://www.ncbi.nlm.nih.gov/geo) provides comprehensive information about human genes (17). We used the term “amino acid metabolism” as the search keyword to collect epigenetic-related genes in the GeneCards database, and based on a relevance score >5, identified 90 AAMRGs. We used “amino acid metabolism” as the search keyword in the Molecular Signatures Database (MSigDB) (https://www.gsea-msigdb.org/gsea/msigdb/) (18), and identified 258 AAMRGs from 11 related reference gene sets. In addition, we searched the relevant literature in the PubMed database (https://pubmed.ncbi.nlm.nih.gov/) and identified 374 genes related to amino acid metabolism. The AAMRGs from the three sources were merged to obtain the 629 AAMRGs analyzed in this study (19). The specific genes list is shown in table available at https://cdn.amegroups.cn/static/public/JTD-24-818-1.xls.
Single-factor prognostic screening
To evaluate the ability of the AAMRGs to predict the survival of patients with ESCC, we used TCGA-ESCC dataset as the test set, and we conducted a single-factor Cox regression analysis to screen the prognostic-related genes with the threshold set at a P value <0.05. The prognostic-related genes that met the screening threshold were included in the follow-up analysis.
Protein-protein interaction (PPI) network
A PPI network is comprised of individual proteins that interact with each other. In this study, we used the Search Tool for the Retrieval of Interacting Genes/Proteins (STRING) database (https://string-db.org/) to construct a PPI network from the identified AAMRGs, and the required minimum interaction score was set at 0.4. We visualized the PPI network model with Cytoscape software (version 3.9.1). The GeneMANIA website (http://genemania.org/) has the ability to predict the functionally similar genes of a target gene and was used to predict the AAMRGs and construct the PPI network in our study (20).
RNA-microRNA (miRNA) and messenger RNA (mRNA)-TF prediction networks
The ENCORI database (https://rnasysu.com/encori/) was used to search for miRNA targets through high-throughput CLIP-Seq experimental data and degradome experimental data, and it provided a variety of visual interfaces for exploring miRNA targets. The database contains a wealth of data of miRNA-long noncoding RNA (lncRNA), miRNA-mRNA, miRNA-RNA, and RNA-lncRNA. We used the ENCORI database to predict the miRNAs interacting with the AAMRGs, and then screened the miRNAs with more than four supporting databases in the results (21). Cytoscape software was used to establish the mRNA-miRNA interaction network. Next, CHIPBase database (version 3.0) (https://rna.sysu.wsu.cn/chipbase/) identified thousands of binding motif matrices and their binding sites from ChIP-seq data of DNA-binding proteins, and predicted the transcriptional regulatory relationship between millions of TFs and genes. Then CHIPBase database was used to predict the TFs interacting with the AAMRGs and the TFs with more than 10 supporting documents were then screened out (22). Cytoscape software was used to establish the mRNA-TF network.
Consistency clustering and differential expression analysis
The differently expressed genes (DEGs) that were identified in both the ESCC group and adjacent normal group of TCGA_GTEx-ESCC dataset, ESCC dataset, and GEO_ESCC dataset were considered important genes and included in the further analysis. The DEGs were screened according to the following filter criteria: (log2|fold change| >0, P value <0.05). Genes with log2fold change >0 and P value <0.05 were upregulated differentially expressed genes (upregulated genes), and genes with log2fold change <0 and P value <0.05 were downregulated differentially expressed genes (downregulated genes). We used the consensus clustering method of the ConsensusClusterPlus R package to identify different ESCC disease subtypes based on the AAMRGs. In the process, the number of clusters was set between 2 and 8, and 80% of the total samples were extracted for 1,000 repetitions. We selected “km” for the clusterAlg parameter and “Euclidean” for the distance parameter. We then divided the count expression profile data processed by TCGA-ESCC dataset into cluster 1 group and cluster 2 group for the differential analysis. The DEGs were screened from cluster 1 and cluster 2 and displayed in a volcano map.
Functional analysis of the DEGs
To further explore the biological function, a Gene Ontology (GO) analysis and a Kyoto Encyclopedia of Genes and Genomes (KEGG) analysis were performed with the DEGs in the cluster 1 and cluster 2 groups. A gene set enrichment analysis (GSEA) was performed using the GSEA online tool (http://software.broadinstitute.org/gsea/index.jsp). A gene set variation analysis (GSVA) was performed using the GSVA R package (version 1.30.0).
Cox prediction model
To examine the clinical prognostic value of the identified AAMRGs on ESCC, we performed a univariate Cox regression analysis of the expression levels of the AAMRGs in TCGA-ESCC dataset and clinical variables, such as age and gender. We also drew a forest diagram to display the result. Genes with a P value <0.1 were selected for inclusion in the multivariate Cox regression analysis, and a multivariate Cox regression model was constructed. Based on the results of the multivariate Cox regression analysis, we established a nomogram to predict the 1- and 2-year probability of no disease progression in ESCC patients. Finally, we used calibration curves to evaluate the accuracy and resolution of the nomogram. A DCA is a simple method for evaluating clinical predictive models, diagnostic tests, and molecular markers. We used the ggDCA R package to draw a DCA diagram and evaluate the predictive effect of the nomogram model on the 1- and 2-year survival outcomes of the ESCC patients.
RNA isolation and quantitative RT-qPCR assays
Twenty-one ESCC tissues and adjacent tissues from ESCC patients were obtained from Fujian Cancer Hospital July 2020 to August 2023. The clinical information of ESCC patients is presented in Table S1. The expression of BCAT1 and MMACHC of 21 ESCC and adjacent tissues was detected by RT-qPCR. Total RNA was isolated using TRIzol reagent (Thermo Fisher Scientific, Waltham, MA, USA). The complementary DNA (cDNA) was synthesized using the RevertAidTM First Strand cDNA Synthesis Kit (Thermo Fisher Scientific). RT-qPCR was performed on a StepOne Real-Time PCR system (Thermo Fisher Scientific). The relative gene expression levels were quantified using the 2−ΔΔCT method. The study was conducted in accordance with the Declaration of Helsinki (as revised in 2013). This study was approved by the Ethics Committee of Fujian Cancer Hospital (No. K2021-027-01). Individual consent for this retrospective analysis was waived.
Statistical analysis
All the data processing and analyses were completed using R software (version 4.2.2). The continuous variables were presented as the mean ± standard deviation. The t-test was used to compare the differences between the normally distributed variables in the two groups, and the Wilcoxon rank-sum test was used to compare the differences between the continuous variables. The prognostic K-M curve and Cox regression model were constructed using the survival R package.
Results
Technical roadmap
The technical roadmap is shown in Figure 1. The GSE20347 and GSE67269 ESCC datasets were merged to obtain the GEO_ESCC dataset, and the data were processed to remove the batch effect. After the removal of the batch effect, the dataset was compared by a distribution boxplot and principal component analysis (PCA) graph (Figure 2A-2D). To evaluate the ability of the AAMRGs to predict the survival of ESCC patients, TCGA-ESCC dataset was used as the test set. The 18 prognosis-related genes screened by the univariate Cox regression analysis were included in a subsequent analysis. A PPI network, mRNA-miRNA interaction network, and mRNA-TF interaction network of the 18 prognosis-related genes were constructed to evaluate the internal connection with other molecule and predict function.
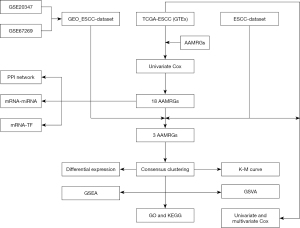
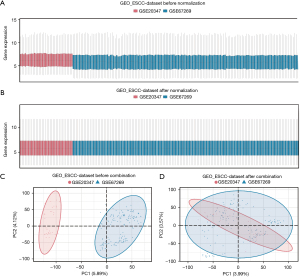
Among the 18 prognosis-related genes, two DEGs from the ESCC group and the normal group in TCGA_GTEx-ESCC, ESCC, and GEO_GEO datasets were selected and screened for the subsequent analysis. Consensus clustering was performed based on the expression matrix of the two prognosis-related DEGs in TCGA-ESCC dataset, and finally, two ESCC disease subtypes (k=2) (cluster 1 and cluster 2) were identified. A difference analysis of TCGA-ESCC dataset between the cluster 1 and cluster 2 groups was performed. Next, a GSEA, GSVA, GO analysis, and KEGG analysis were performed. Finally, the expression of the two prognosis-related DEGs and clinical variables, such as age and gender, from TCGA-ESCC dataset were used to construct a Cox model.
PPI, mRNA-miRNA, and mRNA-TF networks
To evaluate the ability of the AAMRGs to predict the survival of ESCC patients, we used TCGA-ESCC dataset as the test set, and performed a single-factor Cox regression analysis to screen the prognosis-related genes. The 18 prognosis-related genes (i.e., ASMT, ATF3, BCAT1, BECN1, EEFSEC, GLUL, HGD, KYAT1, MMACHC, PAH, PKHD1, PSMC6, RPL17, RPL37, RRAGB, SAT1, SLC6A15, and TARS2) were selected and analyzed based on the PPIs. The required minimum interaction score of the STRING database was set at 0.400, and eight AAMRGs [i.e., BCAT1, GLUL, HGD, KYAT1 (CCBL1), PAH, RPL17, RPL37, and SLC6A15] were included in the PPI network (Figure 3A).
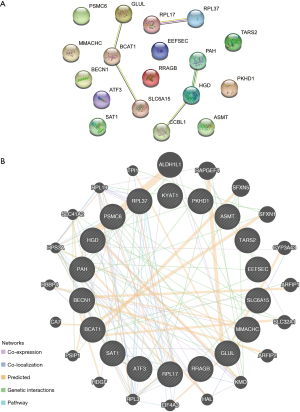
To identify the functionally similar genes through the GeneMANIA website, an interaction network of these 18 AAMRGs was constructed; the co-expression, co-localization, predicted relationship, gene interaction relationship, pathway connection, were represented by the different colored lines (Figure 3B). The mRNA-miRNA prediction network of AAMRGs is shown in Figure 4A, and the list is presented in Table 2. To predict the TFs that interacted with the AAMRGs, a mRNA-TF interaction network was visualized (Figure 4B). The interaction network comprised 14 mRNAs (i.e., BCAT1, BECN1, GLUL, HGD, MMACHC, PAH, PKHD1, PSMC6, RPL17, RPL37, RRAGB, SAT1, SLC6A15, and TARS2) and 36 TFs (Table 3).
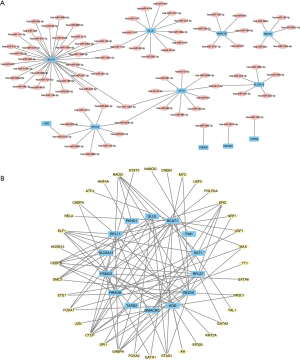
Table 2
mRNA | miRNA |
---|---|
ATF3 | hsa-let-7a-5p, hsa-let-7d-5p, hsa-miR-98-5p, hsa-miR-10a-5p, hsa-let-7i-5p, hsa-miR-485-3p, hsa-miR-526b-5p, hsa-miR-641, hsa-miR-361-3p, hsa-miR-450b-5p, hsa-miR-1224-5p |
BCAT1 | hsa-miR-19a-3p, hsa-miR-32-5p, hsa-miR-92a-3p, hsa-miR-105-5p, hsa-miR-106a-5p, hsa-miR-196a-5p, hsa-miR-7-5p, hsa-miR-199b-5p, hsa-miR-215-5p, hsa-miR-124-3p, hsa-miR-141-3p, hsa-miR-186-5p, hsa-miR-381-3p, hsa-miR-335-5p, hsa-miR-495-3p, hsa-miR-498, hsa-miR-520d-5p, hsa-miR-499a-5p, hsa-miR-493-3p, hsa-miR-92b-3p, hsa-miR-579-3p, hsa-miR-620, hsa-miR-140-3p, hsa-miR-340-5p, hsa-miR-501-3p, hsa-miR-888-5p, hsa-miR-873-5p, hsa-miR-942-5p, hsa-miR-1224-5p, hsa-miR-513b-5p, hsa-miR-1276, hsa-miR-3163, hsa-miR-500b-5p, hsa-miR-4429, hsa-miR-506-5p |
BECN1 | hsa-miR-136-5p, hsa-miR-302c-3p, hsa-miR-520e, hsa-miR-130a-5p, hsa-miR-23c |
EEFSEC | hsa-miR-3619-5p |
GLUL | hsa-let-7b-5p, hsa-miR-24-3p, hsa-miR-7-5p, hsa-miR-429, hsa-miR-515-5p, hsa-miR-193a-5p, hsa-miR-582-3p, hsa-miR-1323, hsa-miR-1321, hsa-miR-1911-5p, hsa-miR-4784, hsa-miR-5194 |
HGD | hsa-miR-513c-5p |
MMACHC | hsa-miR-139-5p, hsa-miR-194-5p, hsa-miR-577, hsa-miR-769-5p, hsa-miR-665, hsa-miR-4731-5p |
PSMC6 | hsa-miR-382-3p |
RPL37 | hsa-miR-92a-3p, hsa-miR-205-5p, hsa-miR-150-5p, hsa-miR-526b-3p, hsa-miR-450b-5p, hsa-miR-513c-5p, hsa-miR-1913, hsa-miR-670-5p, hsa-miR-3064-5p |
SLC6A15 | hsa-let-7i-5p, hsa-miR-125b-5p, hsa-miR-372-3p, hsa-miR-409-3p, hsa-miR-497-5p, hsa-miR-505-3p |
TARS2 | hsa-miR-195-5p |
mRNA, messenger RNA; miRNA, microRNA.
Table 3
mRNA | TF |
---|---|
BCAT1 | ATF4, CEBPB, CTCF, GATA1, GATA2, MAX, MYC, RAD21, RELA, SMC3, SPI1, STAG1, TAL1, USF1 |
BECN1 | CREB1, CTCF, ELF1, ETS1, GABPA, MAX, MYC, NR3C1, NRF1, RAD21, SMC3, SPI1, STAG1 |
GLUL | CTCF, FOXA1, FOXA2, RAD21, AR, SMC3, STAG1 |
HGD | FOXA1, FOXA2, HNF4A, HOXB13, JUN, NR3C1, AR, CEBPB, EP300 |
MMACHC | ELF1, ERG |
PAH | NANOG |
PKHD1 | CTCF, FOXA1, RAD21 |
PSMC6 | ERG, GABPA, GATA1, GATA2, GATA6, POLR2A, STAT3 |
RPL17 | CEBPA, CEBPB, CTCF, ELF1, ERG, GABPA, KMT2A, NRF1, RAD21, SMC3, STAG1 |
RPL37 | ELF1, ERG, ETS1, FOXA1, GABPA, SPI1, CEBPB |
RRAGB | CEBPA, CEBPB, CTCF, MAX, USF1, USF2 |
SAT1 | ELF1, ERG, GABPA, RAD21, CTCF |
SLC6A15 | CEBPB |
TARS2 | CTCF, ELF1, GABPA, YY1 |
mRNA, messenger RNA; TF, transcription factor.
Gene expression verification and prognostic analysis
Among the 18 AAMRGs, the DEGs of the ESCC group and the normal group in TCGA_GTEx-ESCC, ESCC, and GEO_GEO datasets were explored. A total of two genes (i.e., BCAT1 and MMACHC) were differentially expressed in the two groups (Figure 5A-5C). Both the BCAT1 and MMACHC genes were more upregulated in the ESCC tissues than the normal tissues in the three databases. However, the expression of SAT1 was inconsistent across the three databases, and was thus not included in the follow-up analysis. In the survival analysis, the expression of MMACHC was found to be positively associated with the survival time of the ESCC patients (P=0.02). A high expression of BCAT1 appeared to be associated with longer OS in the ESCC patients although this was not statistically significant (P=0.17) (Figure 5D,5E).
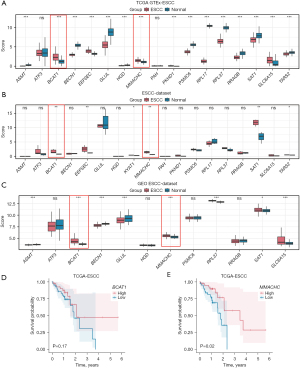
Consensus clustering to construct the disease subtypes of ESCC
To explore the expression differences of the two AAMRGs (i.e., BCAT1 and MMACHC) in the ESCC patients in TCGA-ESCC dataset, the “ConsensusClusterPlus” R package was used to identify the different disease subtypes related to ESCC. Two ESCC disease subtypes (cluster 1 and cluster 2) were identified (Figure 6A). ESCC disease subtype 1 (cluster 1) comprised 53 samples, while ESCC disease subtype 2 (cluster 2) comprised 35 samples. The results showed the consistent cluster cumulative distribution function (CDF) and different clusters (Figure 6B). A delta plot was generated of the area under the CDF curve for the number of classes (Figure 6C). The K-M curve of TCGA-ESCC dataset for ESCC disease subtypes (cluster 1 and cluster 2) was plotted (Figure 6D). It showed that the difference in the survival time between cluster 1 and cluster 2 was statistically significant (P=0.043), and the prognosis of cluster 1 was worse than that of cluster 2. A heatmap was generated to display the expression levels of these two AAMRGs in cluster 1 and cluster 2 in TCGA-ESCC dataset (Figure 6E).
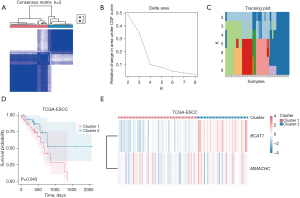
GSEA and GSVA of the ESCC disease subtypes
A total of 1,246 DEGs were identified in the ESCC and normal groups using TCGA-ESCC dataset, of which 506 DEGs were significantly upregulated and 740 DEGs were significantly downregulated (Figure 7A). A GSEA was performed, and the results showed that the genes in TCGA-ESCC dataset were significantly enriched in the PI3K/AKT signaling pathway (P<0.001), pre-Notch expression and processing (P=0.004), the TGF-β signaling pathway (P=0.005), the Hippo signaling regulation pathway (P=0.03), the MAPK family signaling cascades (P=0.03), and other pathways (Figure 7B-7G and Table 4).
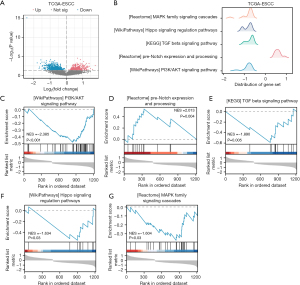
Table 4
ID | Enrichment score | NES | P value |
---|---|---|---|
Wp PI3K/AKT signaling pathway | 0.48 | 2.38 | <0.001 |
Wp focal adhesion PI3K/AKT/mTOR signaling pathway | 0.47 | 2.3 | <0.001 |
Reactome signaling by TGF-β family members | 0.59 | 1.95 | <0.001 |
Reactome pre-Notch expression and processing | 0.53 | 2.01 | <0.001 |
KEGG TGF-β signaling pathway | 0.59 | 1.89 | 0.01 |
Wp Hippo-merlin signaling dysregulation | 0.57 | 1.76 | 0.01 |
Wp Hippo signaling regulation pathways | 0.55 | 1.63 | 0.03 |
Reactome MAPK family signaling cascades | 0.37 | 1.6 | 0.03 |
Wp TGF-β receptor signaling in skeletal dysplasia | 0.62 | 1.59 | 0.04 |
GSEA, gene set enrichment analysis; NES, normalized enrichment score; KEGG, Kyoto Encyclopedia of Genes and Genomes analysis.
To explore the difference in the hallmark gene set between the ESCC disease subtypes, a GSVA was performed using TCGA-ESCC dataset. In total, five hallmark gene sets were found to differ between the cluster 1 and cluster 2 groups (Figure 8A and Table 5). Figure 8B shows a group comparison chart of the five hallmark gene sets, including the pathway of angiogenesis, epithelial-mesenchymal transition, peroxisome, coagulation, and ultraviolet (UV) response DNA damage (DN).
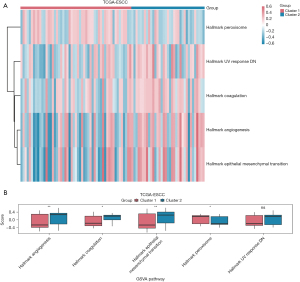
Table 5
Ontology | Log2fold change | P value |
---|---|---|
Hallmark angiogenesis | 0.24 | <0.001 |
Hallmark epithelial-mesenchymal transition | 0.23 | <0.001 |
Hallmark peroxisome | 0.15 | <0.001 |
Hallmark coagulation | 0.15 | 0.01 |
Hallmark UV response DN | 0.14 | 0.02 |
GSVA, gene set variation analysis; UV, ultraviolet; DN, DNA damage.
GO and KEGG analyses
To explore the potential biological function of the DEGs between the cluster 1 and cluster 2 groups in TCGA-ESCC dataset, GO and KEGG analyses were performed. Separate GO gene functional enrichment analyses for the cluster 1 and cluster 2 groups of TCGA-ESCC dataset were conducted (Table 6), as well as KEGG functional enrichment analyses (Table 7). The top 20 pathways with the smallest p values for both GO and KEGG, representing their respective up- and downregulation, are presented in Figure 9A,9B.
Table 6
Ontology | Log2fold change | P value |
---|---|---|
GO BP apoptotic process involved in heart morphogenesis | −0.32 | <0.001 |
GO BP atrial cardiac muscle tissue development | −0.26 | <0.001 |
GO BP endocardial cushion development | −0.19 | <0.001 |
GO BP endocardial cushion morphogenesis | −0.2 | <0.001 |
GO BP histone H3 K14 acetylation | 0.14 | <0.001 |
GO BP indole containing compound biosynthetic process | 0.22 | <0.001 |
GO BP indole containing compound metabolic process | 0.15 | <0.001 |
GO BP L arginine transmembrane transport | −0.18 | <0.001 |
GO BP maintenance of protein location in extracellular region | −0.29 | <0.001 |
GO BP muscle cell fate commitment | −0.2 | <0.001 |
GO BP N terminal protein amino acid acetylation | 0.18 | <0.001 |
GO BP norepinephrine transport | −0.2 | <0.001 |
GO BP pharyngeal arch artery morphogenesis | −0.25 | <0.001 |
GO BP pharyngeal system development | −0.18 | <0.001 |
GO BP positive regulation of bmp signaling pathway | −0.19 | <0.001 |
GO BP positive regulation of cytoplasmic translational initiation | 0.23 | <0.001 |
GO BP positive regulation of Golgi to plasma membrane protein transport | 0.21 | <0.001 |
GO BP preassembly of GPI anchor in ER membrane | 0.26 | <0.001 |
GO BP protein linear polyubiquitination | 0.19 | 0.01 |
GO BP regulation of chondrocyte development | −0.38 | <0.001 |
GO BP regulation of glomerulus development | −0.29 | <0.001 |
GO BP regulation of lymphangiogenesis | −0.3 | <0.001 |
GO BP regulation of mitotic spindle assembly | 0.17 | <0.001 |
GO BP regulation of type I interferon mediated signaling pathway | 0.14 | <0.001 |
GO BP righting reflex | −0.22 | <0.001 |
GO BP serotonin metabolic process | 0.16 | 0.01 |
GO BP sinoatrial node development | −0.24 | <0.001 |
GO CC alveolar lamellar body | 0.26 | <0.001 |
GO CC facit collagen trimer | −0.33 | <0.001 |
GO CC FHF complex | 0.28 | <0.001 |
GO CC protein phosphatase type 1 complex | 0.15 | 0.01 |
GO CC ripoptosome | 0.25 | 0.01 |
GO CC ubiquitin conjugating enzyme complex | 0.19 | 0.01 |
GO MF basic amino acid transmembrane transporter activity | −0.16 | <0.001 |
GO MF connexin binding | 0.19 | 0.01 |
GO MF delta catenin binding | −0.23 | <0.001 |
GO MF G quadruplex RNA binding | 0.22 | <0.001 |
GO MF L arginine transmembrane transporter activity | −0.19 | <0.001 |
GO MF P type calcium transporter activity | 0.24 | <0.001 |
GO MF retinyl palmitate esterase activity | 0.29 | <0.001 |
GO, Gene Ontology; BP, biological progress; CC, cellular component; MF, molecular function.
Table 7
Ontology | Log2fold change | P value |
---|---|---|
KEGG glycosaminoglycan biosynthesis chondroitin sulfate | −0.19 | 0.01 |
KEGG nicotinate and nicotinamide metabolism | −0.1 | 0.02 |
KEGG peroxisome | 0.10 | 0.03 |
KEGG valine leucine and isoleucine biosynthesis | −0.14 | 0.03 |
KEGG GPI anchor biosynthesis | 0.11 | 0.03 |
KEGG ECM receptor interaction | −0.15 | 0.04 |
KEGG ubiquitin mediated proteolysis | 0.08 | 0.04 |
KEGG glycosphingolipid biosynthesis globo series | −0.12 | 0.04 |
KEGG non-small cell lung cancer | 0.08 | 0.05 |
KEGG O glycan biosynthesis | −0.11 | 0.05 |
KEGG, Kyoto Encyclopedia of Genes and Genomes; GPI, glycosylphosphatidylinositol; ECM, extracellular matrix.
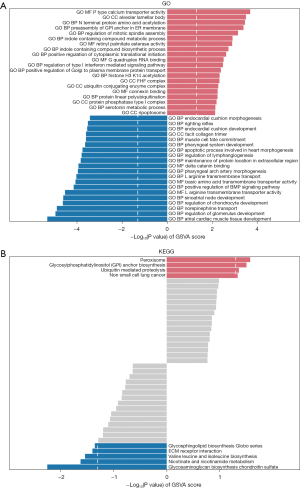
Cox model construction
We also performed a statistical analysis of the clinical information of the ESCC patients in TCGA-ESCC dataset (Table 8). We then performed univariate and multivariate Cox regression analyses of TCGA-ESCC dataset to analyze the expression level of the two AAMRGs (i.e., BCAT1 and MMACHC) and clinical variables, such as age, gender, pathological stage, clinical T, N, and M stage, and clinical variables. For survival analysis, we first performed a univariate Cox regression analysis (Table 9) to examine the expression of these two AAMRGs and the clinical variables, and we drew a forest plot (Figure 10A). Factors with a P value <0.10 in the univariate Cox regression were then included in the multivariate Cox regression analysis. In the regression analysis, a multi-factor Cox regression model was constructed (Table 9), a nomogram analysis of the genes included in the multi-factor Cox regression model was then performed to examine the predictive ability of the model, and a nomogram was drawn (Figure 10B). In addition, a 1- and 2-year prognostic calibration analysis was performed of the nomogram of the multivariate Cox regression model and a calibration curve was drawn (Figure 10C,10D). Notably, the blue line, which corresponded to 1-year survival, was closer to the gray ideal situation line, indicating that the prediction effect of the 1-year model was slightly better than that of the 2-year model. We performed a DCA to evaluate the ability of the constructed Cox regression prognostic model to predict 1- and 2-year survival (Figure 10E,10F). The blue line of the 2-year representative model was generally higher than the “all positive” red line and the “all negative” gray line, indicating that the model had a good prediction effect at 1 and 2 years.
Table 8
Characteristics | Overall (n=88) |
---|---|
OS event | |
Dead | 27 (30.68) |
Alive | 61 (69.32) |
Age (years) | |
≥65 | 22 (25.00) |
<65 | 66 (75.00) |
Sex | |
Male | 76 (86.36) |
Female | 12 (13.64) |
Pathology stage | |
Stage I & II | 55 (62.50) |
Stage III & IV | 31 (35.23) |
T stage | |
T1 | 7 (7.95) |
T2 | 29 (32.95) |
T3 | 46 (52.27) |
T4 | 4 (4.55) |
M stage | |
M0 | 78 (88.64) |
M1 | 4 (4.55) |
N stage | |
N0 & N1 | 76 (86.36) |
N2 & N3 | 9 (10.23) |
Data are presented as n (%). ESCC, esophageal squamous cell carcinoma; TCGA, The Cancer Genome Atlas; OS, overall survival.
Table 9
Characteristics | Univariate Cox | Multivariate Cox | |||
---|---|---|---|---|---|
HR (95% CI) | P | HR (95% CI) | P | ||
Age (years) | |||||
≥65 | 1.5 (0.617–3.67) | 0.37 | 2.12 (0.7–6.43) | 0.18 | |
<65 | |||||
Sex | |||||
Male | 6.17 (0.833–45.7) | 0.07 | |||
Female | |||||
Pathology stage | |||||
Stage III & IV | 2.97 (1.33–6.66) | 0.008 | 2.73 (0.975–7.65) | 0.06 | |
Stage I & II | |||||
T stage | |||||
T2 | 0.973 (0.204–4.64) | 0.97 | |||
T3 | 0.926 (0.205–4.19) | 0.92 | |||
T4 | 3.86 (0.629–23.6) | 0.15 | |||
T1 | |||||
M stage | |||||
M1 | 2.29 (0.667–7.88) | 0.19 | |||
M0 | |||||
N stage | |||||
N2 & N3 | 3.68 (1.43–9.46) | 0.007 | 2.5 (0.82–7.65) | 0.11 | |
N0 & N1 | |||||
BCAT1 | 0.465 (0.232–0.93) | 0.03 | 0.645 (0.293–1.42) | 0.28 | |
MMACHC | 0.159 (0.0334–0.762) | 0.02 | 0.144 (0.02–1.04) | 0.05 |
HR, hazard ratio; ESCC, esophageal squamous cell carcinoma; CI, confidence interval.
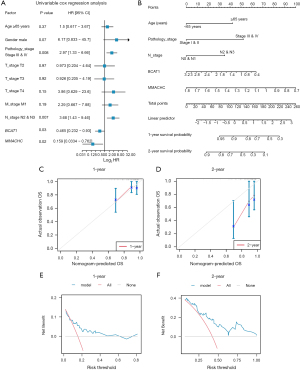
The genes involved in the risk signature
To explore the potential ESCC cancer risk-related genes, the expression of the BCAT1 and MMACHC risk signature genes were further validated. As Figure 11 shows, the expression of both BCAT1 and MMACHC was upregulated in tumors. This finding aligned with our bioinformatics results, which suggests that these genes might serve as innovative biomarkers for ESCC prognosis.
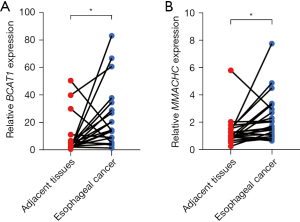
Discussion
ESCC remains a significant public health challenge globally and is characterized by an aggressive nature and a high mortality rate. Despite advancements in diagnostic techniques and treatment modalities, the prognosis of ESCC patients remains poor, and patients have a 5-year survival rate ranging from 15% to 25% (1). Metabolic reprogramming, which is a crucial hallmark of malignancy, undergoes changes in metabolic patterns to meet energy demands during tumor progression, and heterogeneous metabolic subtypes can be observed in local invasive lesions (7,23). Several recent studies have shed light on the intricate connection between amino acid metabolism and EC, particularly the pivotal role of amino acid metabolism in tumor growth and progression (24-26). This study sought to develop a comprehensive prognostic model of ESCC based on the prognostic expression of AAMRGs. Our model combined clinical variables, histopathological data, and BCAT1 and MMACHC expression levels, and advanced statistical methods were used to enhance its predictive accuracy.
This study focused on AAMRGs, and the prognosis of ESCC patients was screened by a univariate Cox regression analysis. In the GEO training set, we first identified 18 prognostic AAMRGs and then built a predictor model comprising two AAMRGs (i.e., BCAT1 and MMACHC) through the integration of least absolute shrinkage and selection operator regression and Cox regression analyses. Moreover, a nomogram was established and calibration plots were used to evaluate whether the nomogram was accurate in the prediction of 1- and 2-year OS. The expression of BCAT1 and MMACHC was higher in the ESCC tissues than the normal control tissues. These results were further confirmed by the RT-qPCR analysis.
We performed consensus clustering (k=2) based on the expression matrix from TCGA-ESCC data set, and finally identified two ESCC disease subtypes that were closely correlated with clinical prognosis (cluster 1 and cluster 2). Patients in the ESCC disease subtype of cluster 1 had a worse prognosis than that of those in cluster 2. In total, 1,246 DEGs between the ESCC disease subtypes were identified and were highly enriched in the PI3K/AKT signaling pathway, TGF-β signaling pathway, and Hippo signaling regulation pathway. Research has shown that the PI3K/AKT signaling pathway is involved in ESCC cell proliferation and survival and is closely related with the progression of chemoresistance in ESCC (27,28). It has been reported that PI3K inhibitors improve responses to chemotherapy in ESCC (29). The Hippo signaling pathway has been shown to preserve the equilibrium between cell proliferation and apoptosis through the meticulous control of factors, including metabolic signals, cell-cell interactions, and mechanical stimuli (30). Thus, any disruption in the Hippo signaling pathway could lead to the onset and advancement of tumors (31). Hippo pathway dysfunction is highly correlated with a poor-prognosis subtype of EC (32). The over-activation of Hippo/YAP signaling might play an important role in the carcinogenic process and progression of ESCC (33). The profound role of TGF-β in early embryonic development, organ formation, immune regulation, tissue repair, and maintaining adult homeostasis has been extensively recognized (34). In healthy and early-stage cancer cells, the TGF-β signaling pathway engages in tumor-suppressing activities, such as inducing cell-cycle arrest and promoting apoptosis. Conversely, when activated in advanced-stage cancers, TGF-β signaling pathway encourages tumor growth, including metastasis and resistance to chemotherapy (35).
In our study, the expression of BCAT1 and MMACHC was upregulated in the ESCC subtype cases (cluster 2), which had a longer survival time. This finding shows the importance of the genes in predicting ESCC clinical outcomes. BCAT1, which is primarily involved in amino acid metabolism, has been identified as a key player in the metabolic reprogramming of cancer cells (36). Recent studies have provided valuable insights into the function of BCAT1 in various malignant tumors. For example, it has been reported that the overexpression of BCAT1 in breast cancer cells leads to enhanced tumor growth and resistance to chemotherapy (37). Similarly, BCAT1 also plays a significant role in the metabolic alterations associated with glioblastoma, influencing tumor aggressiveness, and patient prognosis (38,39). In gastric cancer, the BCAT1 mutation enhances BCAT1 enzymatic activity and accelerates cell growth, motility, and tumor development (36). Previous studies have shown that BCAT1 could serve as a biomarker for the early detection of malignant tumors and a target for therapeutic interventions.
A previous study demonstrated that MMACHC mutations might lead to inherited metabolic disorders. Emerging evidence suggests that MMACHC is a critical player in cancer biology, which has implications for tumor metabolism, growth, and response to treatment. Changes in MMACHC expression and hormone receptor status in breast cancer indicate its potential role in hormone-driven breast cancer pathogenesis. The methylation of the MMACHC gene induces the inactivation of the MMACHC gene and further results in increased tumorigenicity (40). High methylation levels in MMACHC have also been observed in melanoma and medulloblastoma (41). No study has explored the role of MMACHC in ESCC disease; however, the bioinformatics analysis and RT-qPCR results of this study both validated the high expression of MMACHC in ESCC tissues. Our results also showed a positive relationship between MMACHC and ESCC.
There are several limitations in this study. First, besides the ESCC data set, the data analyzed in this study were obtained from public databases. Second, this study only examined a small number of ESCC samples, which represents this study’s main limitation. In addition, the 5-year prognostic model needs to be supplemented in future on the premise of sufficient ESCC cases. Third, the functional mechanisms of the signature need to be explored by in vivo and in vitro experiments.
Conclusions
Generally, this work identified a prognostic signature comprising two AAMRGs (i.e., BCAT1 and MMACHC) for the prediction of the 1- and 2-year OS of ESCC patients. This constructed signature will provide aids for individualized treatment.
Acknowledgments
We are very grateful to databases such as TCGA and the GEO for the data provided. We would also like to thank reviewers and editors for their insightful comments.
Funding: This work was supported in part by grants from
Footnote
Reporting Checklist: The authors have completed the TRIPOD reporting checklist. Available at https://jtd.amegroups.com/article/view/10.21037/jtd-24-818/rc
Data Sharing Statement: Available at https://jtd.amegroups.com/article/view/10.21037/jtd-24-818/dss
Peer Review File: Available at https://jtd.amegroups.com/article/view/10.21037/jtd-24-818/prf
Conflicts of Interest: All authors have completed the ICMJE uniform disclosure form (available at https://jtd.amegroups.com/article/view/10.21037/jtd-24-818/coif). The authors have no conflicts of interest to declare.
Ethical Statement: The authors are accountable for all aspects of the work in ensuring that questions related to the accuracy or integrity of any part of the work are appropriately investigated and resolved. The study was conducted in accordance with the Declaration of Helsinki (as revised in 2013). This study was approved by the Ethics Committee of Fujian Cancer Hospital (No. K2021-027-01). Individual consent for this retrospective analysis was waived.
Open Access Statement: This is an Open Access article distributed in accordance with the Creative Commons Attribution-NonCommercial-NoDerivs 4.0 International License (CC BY-NC-ND 4.0), which permits the non-commercial replication and distribution of the article with the strict proviso that no changes or edits are made and the original work is properly cited (including links to both the formal publication through the relevant DOI and the license). See: https://creativecommons.org/licenses/by-nc-nd/4.0/.
References
- Chen W, Zheng R, Baade PD, et al. Cancer statistics in China, 2015. CA Cancer J Clin 2016;66:115-32. [Crossref] [PubMed]
- Pennathur A, Gibson MK, Jobe BA, et al. Oesophageal carcinoma. Lancet 2013;381:400-12. [Crossref] [PubMed]
- Jin HR, Wang J, Wang ZJ, et al. Lipid metabolic reprogramming in tumor microenvironment: from mechanisms to therapeutics. J Hematol Oncol 2023;16:103. [Crossref] [PubMed]
- Yang Y, Huangfu L, Li H, et al. Research progress of hyperthermia in tumor therapy by influencing metabolic reprogramming of tumor cells. Int J Hyperthermia 2023;40:2270654. [Crossref] [PubMed]
- Koundouros N, Poulogiannis G. Reprogramming of fatty acid metabolism in cancer. Br J Cancer 2020;122:4-22. [Crossref] [PubMed]
- Pavlova NN, Thompson CB. The Emerging Hallmarks of Cancer Metabolism. Cell Metab 2016;23:27-47. [Crossref] [PubMed]
- Li Z, Zhang H. Reprogramming of glucose, fatty acid and amino acid metabolism for cancer progression. Cell Mol Life Sci 2016;73:377-92. [Crossref] [PubMed]
- Lieu EL, Nguyen T, Rhyne S, et al. Amino acids in cancer. Exp Mol Med 2020;52:15-30. [Crossref] [PubMed]
- Geeraerts SL, Heylen E, De Keersmaecker K, et al. The ins and outs of serine and glycine metabolism in cancer. Nat Metab 2021;3:131-41. [Crossref] [PubMed]
- Altman BJ, Stine ZE, Dang CV. From Krebs to clinic: glutamine metabolism to cancer therapy. Nat Rev Cancer 2016;16:619-34. [Crossref] [PubMed]
- Kodama M, Nakayama KI. A second Warburg-like effect in cancer metabolism: The metabolic shift of glutamine-derived nitrogen: A shift in glutamine-derived nitrogen metabolism from glutaminolysis to de novo nucleotide biosynthesis contributes to malignant evolution of cancer. Bioessays 2020;42:e2000169. [Crossref] [PubMed]
- Gao P, Tchernyshyov I, Chang TC, et al. c-Myc suppression of miR-23a/b enhances mitochondrial glutaminase expression and glutamine metabolism. Nature 2009;458:762-5. [Crossref] [PubMed]
- Togashi Y, Arao T, Kato H, et al. Frequent amplification of ORAOV1 gene in esophageal squamous cell cancer promotes an aggressive phenotype via proline metabolism and ROS production. Oncotarget 2014;5:2962-73. [Crossref] [PubMed]
- Lin Z, Chen L, Wu T, et al. Prognostic Value of SPOCD1 in Esophageal Squamous Cell Carcinoma: A Comprehensive Study Based on Bioinformatics and Validation. Front Genet 2022;13:872026. [Crossref] [PubMed]
- Hu N, Clifford RJ, Yang HH, et al. Genome wide analysis of DNA copy number neutral loss of heterozygosity (CNNLOH) and its relation to gene expression in esophageal squamous cell carcinoma. BMC Genomics 2010;11:576. [Crossref] [PubMed]
- Yang H, Su H, Hu N, et al. Integrated analysis of genome-wide miRNAs and targeted gene expression in esophageal squamous cell carcinoma (ESCC) and relation to prognosis. BMC Cancer 2020;20:388. [Crossref] [PubMed]
- Stelzer G, Rosen N, Plaschkes I, et al. The GeneCards Suite: From Gene Data Mining to Disease Genome Sequence Analyses. Curr Protoc Bioinformatics 2016;54:1.30.1-1.30.33.
- Liberzon A, Birger C, Thorvaldsdóttir H, et al. The Molecular Signatures Database (MSigDB) hallmark gene set collection. Cell Syst 2015;1:417-25. [Crossref] [PubMed]
- Su J, Tian X, Zhang Z, et al. A novel amino acid metabolism-related gene risk signature for predicting prognosis in clear cell renal cell carcinoma. Front Oncol 2022;12:1019949. [Crossref] [PubMed]
- Franz M, Rodriguez H, Lopes C, et al. GeneMANIA update 2018. Nucleic Acids Res 2018;46:W60-4. [Crossref] [PubMed]
- Li JH, Liu S, Zhou H, et al. starBase v2.0: decoding miRNA-ceRNA, miRNA-ncRNA and protein-RNA interaction networks from large-scale CLIP-Seq data. Nucleic Acids Res 2014;42:D92-7. [Crossref] [PubMed]
- Zhou KR, Liu S, Sun WJ, et al. ChIPBase v2.0: decoding transcriptional regulatory networks of non-coding RNAs and protein-coding genes from ChIP-seq data. Nucleic Acids Res 2017;45:D43-50. [Crossref] [PubMed]
- Wang J, Zhang W, Liu C, et al. Reprogramming of Lipid Metabolism Mediates Crosstalk, Remodeling, and Intervention of Microenvironment Components in Breast Cancer. Int J Biol Sci 2024;20:1884-904. [Crossref] [PubMed]
- Li X, Zhao L, Wei M, et al. Serum metabolomics analysis for the progression of esophageal squamous cell carcinoma. J Cancer 2021;12:3190-7. [Crossref] [PubMed]
- Taherizadeh M, Khoshnia M, Shams S, et al. Plasma Changes of Branched-Chain Amino Acid in Patients with Esophageal Cancer. Middle East J Dig Dis 2021;13:49-53. [Crossref] [PubMed]
- Yang XL, Wang P, Ye H, et al. Untargeted serum metabolomics reveals potential biomarkers and metabolic pathways associated with esophageal cancer. Front Oncol 2022;12:938234. [Crossref] [PubMed]
- Luo Q, Du R, Liu W, et al. PI3K/Akt/mTOR Signaling Pathway: Role in Esophageal Squamous Cell Carcinoma, Regulatory Mechanisms and Opportunities for Targeted Therapy. Front Oncol 2022;12:852383. [Crossref] [PubMed]
- Wang L, Zhang Z, Yu X, et al. SOX9/miR-203a axis drives PI3K/AKT signaling to promote esophageal cancer progression. Cancer Lett 2020;468:14-26. [Crossref] [PubMed]
- Duan SF, Zhang MM, Zhang X, et al. HA-ADT suppresses esophageal squamous cell carcinoma progression via apoptosis promotion and autophagy inhibition. Exp Cell Res 2022;420:113341. [Crossref] [PubMed]
- Lee U, Cho EY, Jho EH. Regulation of Hippo signaling by metabolic pathways in cancer. Biochim Biophys Acta Mol Cell Res 2022;1869:119201. [Crossref] [PubMed]
- Zanconato F, Cordenonsi M, Piccolo S. YAP/TAZ at the Roots of Cancer. Cancer Cell 2016;29:783-803. [Crossref] [PubMed]
- Mai Z, Yuan J, Yang H, et al. Inactivation of Hippo pathway characterizes a poor-prognosis subtype of esophageal cancer. JCI Insight 2022;7:e155218. [Crossref] [PubMed]
- Zhou X, Li Y, Wang W, et al. Regulation of Hippo/YAP signaling and Esophageal Squamous Carcinoma progression by an E3 ubiquitin ligase PARK2. Theranostics 2020;10:9443-57. [Crossref] [PubMed]
- Peng D, Fu M, Wang M, et al. Targeting TGF-β signal transduction for fibrosis and cancer therapy. Mol Cancer 2022;21:104. [Crossref] [PubMed]
- Colak S, Ten Dijke P. Targeting TGF-β Signaling in Cancer. Trends Cancer 2017;3:56-71. [Crossref] [PubMed]
- Qian L, Li N, Lu XC, et al. Enhanced BCAT1 activity and BCAA metabolism promotes RhoC activity in cancer progression. Nat Metab 2023;5:1159-73. [Crossref] [PubMed]
- Thewes V, Simon R, Hlevnjak M, et al. The branched-chain amino acid transaminase 1 sustains growth of antiestrogen-resistant and ERα-negative breast cancer. Oncogene 2017;36:4124-34. [Crossref] [PubMed]
- Panosyan EH, Lasky JL, Lin HJ, et al. Clinical aggressiveness of malignant gliomas is linked to augmented metabolism of amino acids. J Neurooncol 2016;128:57-66. [Crossref] [PubMed]
- Cho HR, Jeon H, Park CK, et al. BCAT1 is a New MR Imaging-related Biomarker for Prognosis Prediction in IDH1-wildtype Glioblastoma Patients. Sci Rep 2017;7:17740. [Crossref] [PubMed]
- Loewy AD, Niles KM, Anastasio N, et al. Epigenetic modification of the gene for the vitamin B(12) chaperone MMACHC can result in increased tumorigenicity and methionine dependence. Mol Genet Metab 2009;96:261-7. [Crossref] [PubMed]
- Barretina J, Caponigro G, Stransky N, et al. The Cancer Cell Line Encyclopedia enables predictive modelling of anticancer drug sensitivity. Nature 2012;483:603-7. [Crossref] [PubMed]
(English Language Editor: L. Huleatt)