Development of a prognostic nomogram for patients underwent extracorporeal circulation auxiliary to open cardiac surgery on hospital mortality: a retrospective cohort study
Highlight box
Key findings
• This study developed a model for predicting postoperative hospital mortality in patients underwent extracorporeal circulation auxiliary to open cardiac surgery (ECAOCS).
What is known and what is new?
• There are several preoperative risk assessment models for cardiac surgery, such as EuroSCORE II, Society of Thoracic Surgeons Predicted Risk of Mortality (STS-PROM) Score and German AV Score.
• Our study developed a model for predicting postoperative in-hospital mortality in patients undergoing extracorporeal circulation-assisted open-heart surgery by using data within 24 hours of postoperative intensive care unit (ICU) admission.
What is the implication, and what should change now?
• The model can be well used in clinical work to help doctors screen high-risk groups, take targeted treatments, save medical resources, and reduce the postoperative in-hospital mortality of ECAOCS.
Introduction
Cardiac surgery is one of the most difficult operations in the field of surgery, with complex operation, extraordinarily long surgery time, high surgical risk, many complications and poor prognosis as its most obvious labels (1-3). Extracorporeal circulation auxiliary to open cardiac surgery (ECAOCS) is performed with the use of an artificial device that replaces the pumping function of the heart and the oxygenation function of the lungs, which provides the surgeon with a better view of the operation and a longer period of time (4). However, high risk and poor prognosis are inherent disadvantages of cardiac surgery, including hemorrhage, arrhythmia, heart failure, systemic inflammatory response, and renal failure (5-10). Early recognition of these postoperative complications is an effective way to reduce postoperative mortality. Therefore, we need to identify some indicators or create a model to assess the risk of death in patients after ECAOCS.
There have been many surgical prognostic models for cardiac patients, and the more frequently applied ones are EuroSCORE and the Society of Thoracic Surgeons Predicted Risk of Mortality (STS-PROM) Score (11,12). With the help of these scoring models, physicians can accurately know the groups of patients with higher scores and higher prognostic risks, so that they can take relevant therapeutic measures earlier and reduce the incidence of adverse events. However, these models do not use indicators of the patient’s postoperative period and fail to provide immediate postoperative assessment of the patient.
Nomogram is a statistical tool that has recently been widely used in predictive modeling and is capable of integrating multiple influencing factors to assess prognosis (13). Currently, nomogram has been widely used in surgical treatment and chemotherapy of malignant tumors, treatment of chronic diseases, and prognostic evaluation of gene therapy.
We would like to create a predictive model that could evaluate immediately after a patient’s surgery based on vital signs, test markers, hemodynamics, and other factors. We believe that there are differences in each patient’s ability to tolerate ECAOCS. Evaluating patients in the immediate postoperative period gives a better prediction of outcome.
In this study, we developed a prognostic model for the risk of hospital mortality after ECAOCS based on the Medical Information Mart for Intensive Care (MIMIC)-IV database, using nomogram and other statistical methods. To the best of our knowledge, our study is the first to predict hospital mortality after ECAOCS by nomogram. We present this article in accordance with the TRIPOD reporting checklist (available at https://jtd.amegroups.com/article/view/10.21037/jtd-24-24/rc).
Methods
Data source
All patient data for this study were obtained from an international online database, the MIMIC-IV database. The MIMIC-IV is a publicly available single-center intensive care database provided by Beth Israel Deaconess Medical Center (BIDMC, Boston, MA, USA) and the Massachusetts Institute of Technology (MIT, Cambridge, MA, USA) with institutional review board approval; information was obtained for 299,712 patients admitted to various intensive care units (ICUs) at BIDMC in Boston, MA from 2008 to 2019 (14). The MIMIC database has played an integral role in advancing a large body of research in the fields of clinical informatics, epidemiology, and machine learning. The MIMIC database contains clinical information about patients, including demographic information, vital signs, laboratory results, surgical records, hemodynamic results, and nursing records. The collection of patient information and creation of the research resource were reviewed by the Institutional Review Board at the BIDMC, which granted a waiver of informed consent and approved the data-sharing initiative. Our author P.W. (ID: 10984851) passed the ethical examination of this database and gained access to it. The study was conducted in accordance with the Declaration of Helsinki (as revised in 2013).
Study population
All patients who underwent ECAOCS according to the International Classification of Diseases (ICD)-9 codes were extracted, totaling 4,852 patients. We excluded patients with multiple surgeries, age <18 years, and a lack of monitoring data >5%. Eventually 880 eligible patients were enrolled in our study. The specific screening process is shown in Figure 1.
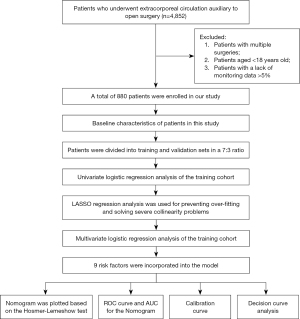
Variable extraction
All variables were extracted using the Structured Query Language (SQL), and pgAdmin4 for PostgreSQL was used as the administrative platform. The extracted variables included the following: demographic characteristics [age, body mass index (BMI) and gender], vital signs [including heart rate (HR), systolic blood pressure (sBP), mean blood pressure (mBP), and diastolic blood pressure (dBP)], laboratory test results [including anion gap (AG), bicarbonate ion (HCO3−), blood urea nitrogen (BUN), chloridion (Cl−), creatinine (Cr), glucose, sodium ion (Na+), potassium ion (K+), hematocrit (HCT), hemoglobin (Hb), red blood cell (RBC), white blood cell (WBC)], coagulation function [including international normalized ratio (INR), prothrombin time (PT), activated partial thromboplastin time (APTT), platelet (PLT)], Sequential Organ Failure Assessment (SOFA) score, Charlson comorbidities index(CCI) score, hemodynamic parameters [systolic pulmonary artery pressure (sPAP), mean pulmonary artery pressure (mPAP), diastolic pulmonary artery pressure (dPAP), central venous pressure (CVP)], concomitant disease [acute myocardial infarction (AMI), congestive heart failure (CHF), peripheral vascular disease (PVD), cerebrovascular disease (CVD), chronic obstructive pulmonary disease (COPD), diabetes, stroke]. All vital signs, laboratory results, coagulation, Sequential Organ Failure Assessment (SOFA) scores, Charlson Comorbidity Index (CCI) scores, and hemodynamic parameters were averaged over the first 24 hours after admission to the ICU.
All data were collected within the first 24 hours after admission to the ICU. If there were several reports on a variable within the first 24 hours, the mean value was chosen for analysis. In this study, data that basically obey the normal distribution or approximate normal distribution were considered as abnormal values when the sample data was greater than ± 3σ. Items with outliers and missing values greater than 5% were discarded. Items with outliers and missing values less than 5% were interpolated using multiple imputation (15). Our study used hospital death as endpoint.
Statistical analysis
Continuous variables were presented as the mean ± SD or median (interquartile range) and were compared by Student t-test or the Kruskal-Wallis U test. Categorical data are presented as numbers with proportions and were analyzed by the χ2 test. All patients were randomly divided into training and validation cohort in a ratio of 7:3. The model was constructed using data from the training group and the model was validated using the validation group.
Univariate logistic regression analyses were performed on all factors for patients in the training cohort. In order to prevent overfitting and to address the problem of severe covariance, all factors with P<0.05 in the univariate logistic regression analysis were analyzed using the least absolute shrinkage and selection operator (LASSO) regression. LASSO regression analysis is achieved by applying regularized paths to the selection of variables. A penalty term is added to the ordinary least squares method to reduce the variance of the regression coefficients by adjusting the coefficients of the penalty term, thus reducing the effect of multicollinearity and preventing overfitting of the model (16). The degree of LASSO regression complexity adjustment is controlled by the parameter λ. The larger λ is the stronger the penalty for a linear model with more variables, thus ending up with a model with fewer variables (17). When λ = min, the result of cross-validation was identified by us as the final candidate variable. The factors screened by the LASSO regression analysis were included as alternative variables in the multivariate logistic regression analysis. Multivariate logistic regression was performed using multiple distant stepwise regression with stepwise regression at forward P<0.1 and backward P<0.05.
All factors ultimately included in the model were determined based on the results of the multivariate logistic regression analysis. The finalized model is presented in the form of a nomogram. The receiver operating characteristic (ROC) curve of patients in the training cohort were plotted according to the sensitivity and specificity of the model, and the area under the curve (AUC) was calculated. Finally, the calibration of the model was evaluated by calibration curves and Hosmer-Lemeshow goodness-of-fit test (HL test). The model was quantified by decision curve analysis (DCA) to predict the net benefit of patients underwent ECAOCS at different threshold probabilities.
In order to ensure the reliability of data extraction operation, our three authors extracted data separately, and then analyzed it by STATA (version 16.0 Stata Corp, College Station, TX, USA) and R version 4.3.1 to ensure the correctness and reliability of the extracted data. We used the “glmnet” R package to fulfillment the LASSO regression model and the “rms” package to materialize the nomogram for mortality risk prediction. All tests were two-sided, and P<0.05 was considered significant.
Results
Characteristics of the patients
A total of 880 patients were finally included in the study based on the inclusion and exclusion criteria set for the study. The training cohort contained 616 patients and the validation cohort contained 264 patients. Demographic characteristics of all training cohort patients at baseline are shown in Table 1. In the training cohort, 395 (66.37%) were male and 221 (33.63%) were female. The mean age of the patients was 67.76 years. In the validation cohort, there were 186 males (65.78%) and 78 females (34.22%). The mean age of the patients was 68.65 years. Hospital mortality rate for patients in the training and validation cohorts were 8.12% (50 patients) and 7.95% (21 patients), respectively.
Table 1
Variable | Total cohort (n=880) | Derivation cohort (n=616) | Validation cohort (n=264) | P value |
---|---|---|---|---|
Age, years | 68.03±12.35 | 67.76±12.35 | 68.65±12.37 | 0.33 |
BMI, kg/m2 | 28.89±9.65 | 28.75±6.22 | 29.25±14.85 | 0.48 |
Gender | 0.07 | |||
Male | 581 (66.00) | 395 (64.12) | 186 (70.45) | |
Female | 299 (34.00) | 221 (35.88) | 78 (29.55) | |
HR, beats/min | 97.64±13.82 | 97.72±13.79 | 97.43±13.91 | 0.78 |
dBP, mmHg | 75.12±13.63 | 75.72±14.12 | 73.73±12.33 | 0.06 |
mBP, mmHg | 100.37±28.37 | 100.52±28.39 | 100.06±28.37 | 0.83 |
sBP, mmHg | 141.75±19.66 | 141.73±18.73 | 141.81±21.73 | 0.96 |
AG, mmol/L | 12.10±2.67 | 12.14±2.63 | 12.00±2.76 | 0.48 |
HCO3−, mmol/L | 23.22±2.27 | 23.22±2.25 | 23.23±2.35 | 0.94 |
BUN, mg/dL | 19.59±11.13 | 19.65±10.54 | 19.48±12.43 | 0.84 |
Cl−, mmol/L | 108.08±3.48 | 108.00±3.47 | 108.26±3.51 | 0.31 |
Cr, mg/dL | 1.13±0.98 | 1.12±0.96 | 1.15±1.01 | 0.71 |
Glucose, mg/dL | 12.45±3.27 | 12.48±3.05 | 12.39±3.76 | 0.71 |
Na+, mmol/L | 138.78±2.73 | 138.78±2.82 | 138.79±2.53 | 0.97 |
K+, mmol/L | 4.35±0.44 | 4.34±0.42 | 4.38±0.47 | 0.24 |
INR | 1.37±0.22 | 1.37±0.22 | 1.37±0.22 | 0.87 |
PT, s | 15.09±2.23 | 15.09±2.19 | 15.11±2.29 | 0.93 |
APTT, s | 36.74±14.26 | 36.45±14.36 | 37.41±14.02 | 0.36 |
HCT | 27.61±5.07 | 27.49±5.15 | 27.89±4.88 | 0.27 |
Hb, g/dL | 10.08±23.92 | 10.06±1.28 | 10.12±1.23 | 0.56 |
PLT, 109/L | 155.44±64.33 | 157.91±68.09 | 149.67±1.23 | 0.08 |
RBC, 1012/L | 3.32±0.44 | 3.32±0.44 | 3.32±0.45 | 0.85 |
WBC, 109/L | 13.26±5.52 | 13.29±5.03 | 13.19±6.511 | 0.79 |
SOFA | 3.99±2.09 | 4.04±2.15 | 3.89±1.95 | 0.31 |
CCI | 5.52±2.19 | 5.51±2.13 | 5.54±2.31 | 0.86 |
AMI | 241 (27.39) | 172 (27.92) | 69 (26.14) | 0.59 |
CHF | 317 (36.02) | 233 (37.82) | 84 (31.82) | 0.09 |
PVD | 181 (20.57) | 128 (20.78) | 53 (20.08) | 0.81 |
CVD | 113 (12.84) | 78 (12.66) | 35 (13.26) | 0.79 |
COPD | 274 (31.14) | 192 (31.17) | 82 (31.06) | 0.98 |
Diabetes | 209 (23.75) | 149 (24.19) | 60 (22.73) | 0.64 |
Stroke | 123 (13.98) | 80 (12.99) | 43 (16.29) | 0.19 |
dPAP, mmHg | 18.37±4.82 | 18.39±4.77 | 18.33±4.94 | 0.87 |
mPAP, mmHg | 24.80±5.79 | 24.80±5.65 | 24.81±6.13 | 0.99 |
sPAP, mmHg | 34.79±8.18 | 34.71±7.91 | 34.96±8.78 | 0.69 |
CVP, cmH2O | 12.39±4.81 | 12.41±4.63 | 12.37±5.22 | 0.91 |
Dead in hospital | 71 (8.07) | 50 (8.12) | 21 (7.95) | 0.94 |
Continuous variables were presented as mean ± standard deviation. Categorical data are presented as numbers with proportions. BMI, body mass index; HR, heart rate; dBP, diastolic blood pressure; mBP, mean blood pressure; sBP, systolic blood pressure; AG, anion gap; HCO3−, bicarbonate ion; BUN, blood urea nitrogen; Cl−, chloridion; Cr, creatinine; Na+, sodium ion; K+, potassium ion; INR, international normalized ratio; PT, prothrombin time; APTT, activated partial thromboplastin time; HCT, hematocrit; Hb, hemoglobin; PLT, platelet; RBC, red blood cell; WBC, white blood cell; SOFA, Sequential Organ Failure Assessment score; CCI, Charlson comorbidities index score; AMI, acute myocardial infarction; CHF, congestive heart failure; PVD, peripheral vascular disease; CVD, cerebrovascular disease; COPD, chronic obstructive pulmonary disease; dPAP, diastolic pulmonary artery pressure; mPAP, mean pulmonary artery pressure; sPAP, systolic pulmonary artery pressure; CVP, central venous pressure.
Univariate analysis in training cohort
In the training cohort, patients who died hospital after ECAOCS differed significantly from nonfatal patients in the factors HR, AG, HCO3−, BUN, Cr, glucose, Na+, INR, PT, APTT, SOFA, CCI, AMI, PVD, CVD, dPAP, mPAP, sPAP, and CVP according to the results of univariate logistic regression analysis. The detailed results are shown in Table 2.
Table 2
Variables | Univariate analysis | ||
---|---|---|---|
OR | 95% CI | P value | |
Age, years | 0.99 | 0.97–1.02 | 0.96 |
BMI, kg/m2 | 1.04 | 0.99–1.08 | 0.08 |
Gender | 1.72 | 0.97–3.09 | 0.07 |
HR, beats/min | 1.02 | 1.01–1.04 | 0.02 |
dBP, mmHg | 1.01 | 0.98–1.02 | 0.69 |
mBP, mmHg | 1.01 | 0.99–1.01 | 0.10 |
sBP, mmHg | 1.01 | 0.99–1.02 | 0.19 |
AG, mmol/L | 1.4 | 1.26–1.55 | <0.001 |
HCO3−, mmol/L | 0.73 | 0.64–0.84 | <0.001 |
BUN, mg/dL | 1.06 | 1.04–1.08 | <0.001 |
Cl−, mmol/L | 0.95 | 0.88–1.02 | 0.22 |
Cr, mg/dL | 1.57 | 1.29–1.91 | <0.001 |
Glucose, mg/dL | 1.02 | 1.01–1.03 | <0.001 |
Na+, mmol/L | 1.14 | 1.03–1.26 | 0.009 |
K+, mmol/L | 0.86 | 0.43–1.73 | 0.68 |
INR | 4.65 | 1.74–12.39 | 0.002 |
PT, s | 1.16 | 1.05–1.29 | 0.003 |
APTT, s | 1.05 | 1.04–1.07 | <0.001 |
HCT | 1.02 | 0.97–1.08 | 0.38 |
Hb, g/dL | 1.02 | 0.81–1.27 | 0.89 |
PLT, 109/L | 1 | 0.99–1.01 | 0.06 |
RBC, 1012/L | 1.22 | 0.64–2.32 | 0.55 |
WBC, 109/L | 0.95 | 0.89–1.01 | 0.13 |
SOFA | 1.21 | 1.08–1.36 | 0.001 |
CCI | 1.37 | 1.19–1.56 | <0.001 |
AMI | 2.38 | 1.33–4.29 | 0.004 |
CHF | 1.72 | 0.96–3.07 | 0.07 |
PVD | 2.83 | 1.55–5.17 | 0.001 |
CVD | 2.71 | 1.37–5.36 | 0.004 |
COPD | 1.04 | 0.56–1.94 | 0.90 |
Diabetes | 0.87 | 0.44–1.75 | 0.71 |
Stroke | 1.31 | 0.59–2.89 | 0.51 |
dPAP, mmHg | 1.17 | 1.10–1.23 | <0.001 |
mPAP, mmHg | 1.12 | 1.07–1.17 | <0.001 |
sPAP, mmHg | 1.07 | 1.04–1.10 | <0.001 |
CVP, cmH2O | 1.11 | 1.05–1.17 | <0.001 |
OR, odds ratio; CI, confidence interval; BMI, body mass index; HR, heart rate; dBP, diastolic blood pressure; mBP, mean blood pressure; sBP, systolic blood pressure; AG, anion gap; HCO3−, bicarbonate ion; BUN, blood urea nitrogen; Cl−, chloridion; Cr, creatinine; Na+, sodium ion; K+, potassium ion; INR, international normalized ratio; PT, prothrombin time; APTT, activated partial thromboplastin time; HCT, hematocrit; Hb, hemoglobin; PLT, platelet; RBC, red blood cell; WBC, white blood cell; SOFA, Sequential Organ Failure Assessment score; CCI, Charlson comorbidities index score; AMI, acute myocardial infarction; CHF, congestive heart failure; PVD, peripheral vascular disease; CVD, cerebrovascular disease; COPD, chronic obstructive pulmonary disease; dPAP, diastolic pulmonary artery pressure; mPAP, mean pulmonary artery pressure; sPAP, systolic pulmonary artery pressure; CVP, central venous pressure.
Selection of the risk predictors
In order to obtain a more streamlined model while avoiding covariance and overfitting, we performed LASSO regression analyses on the factors with significant differences in the univariate logistic regression analyses. Nineteen factors with significant differences in the univariate logistic regression analyses were analyzed by LASSO regression. We chose the factors incorporated at λ = min as the output. According to the results of the LASSO regression analysis in Figure 2, a total of nine factors were included in the final model and they were AG, CVP, glucose, Cr, APTT, HCO3−, CVD, AMI, PVD. The above nine factors were subjected to multifactor regression analysis. Multivariate logistic regression was performed using multiple distant stepwise regression with stepwise regression at forward P<0.1 and backward P<0.05. The results are shown in Table 3. Nine factors were ultimately included in the final model, namely: AG (OR: 0.14; 95% CI: 0.005–0.28), CVP (OR: 0.06; 95% CI: 0.04–0.12), glucose (OR: 0.009; 95% CI: 0.001–0.018), Cr (OR: 0.23; 95% CI: −0.03 to 0.49), APTT (OR: 0.05; 95% CI: 0.029–0.064), HCO3− (OR: −0.17; 95% CI: −0.33 to −0.02), CVD (OR: 1.13; 95% CI: 0.29–1.97), AMI (OR: 0.96; 95% CI: 0.23–1.69), PVD (OR: 1.19; 95% CI: 0.44–1.95). Based on this model, we constructed a nomogram to predict hospital mortality in patients after ECAOCS. We randomly selected a patient, labeled factor scores in the nomogram, and finally calculated total score and risk of death (Figure 3). This patient with an AG level of 16, a CVP of 13.03, a glucose level of 126.5, a Cr level of 2.8, an APTT level of 31.63, a HCO3− level of 19.67, PVD, AMI but no CVD had a total score of 318 points, which corresponded to an approximately 37% hospital survival probability.
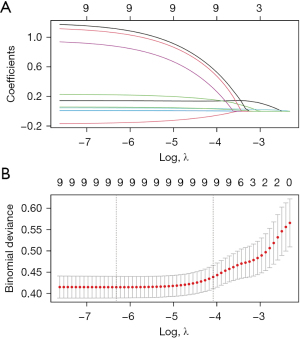
Table 3
Variables | Multivariate analysis | ||
---|---|---|---|
OR | 95% CI | P value | |
AG, mmol/L | 0.14 | 0.005–0.28 | 0.04 |
HCO3−, mmol/L | −0.17 | −0.33 to −0.02 | 0.03 |
Cr, mg/dL | 0.23 | −0.03 to 0.49 | 0.08 |
Glucose, mg/dL | 0.009 | 0.001–0.018 | 0.04 |
APTT, s | 0.05 | 0.029–0.064 | <0.001 |
AMI | 0.96 | 0.23–1.69 | 0.01 |
PVD | 1.19 | 0.44–1.95 | 0.002 |
CVD | 1.13 | 0.29–1.97 | 0.008 |
CVP | 0.06 | 0.04–0.12 | 0.048 |
LASSO, least absolute shrinkage and selection operator; OR, odds ratio; CI, confidence interval; AG, anion gap; HCO3−, bicarbonate ion; Cr, creatinine; APTT, activated partial thromboplastin time; AMI, acute myocardial infarction; PVD, peripheral vascular disease; CVD, cerebrovascular disease; CVP, central venous pressure.
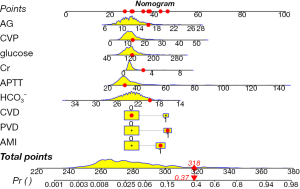
Verification of the predictive value of the nomogram
Based on the AUC obtained from the ROC analysis, the predictive power of the model in the training cohort was 0.8892 and 0.8848 in the validation cohort (Figure 4). The results of the decision curves showed that the model’s fit for predicting hospital mortality after ECAOCS was high in both the training cohort and the validation cohort. The results of the HL test were P=0.11 and P=0.34, respectively (Figure 5). DCA showed that the threshold probability of net gain from applying our model exceeded 60% in the training cohort and reached 80% in the validation cohort (Figure 6).
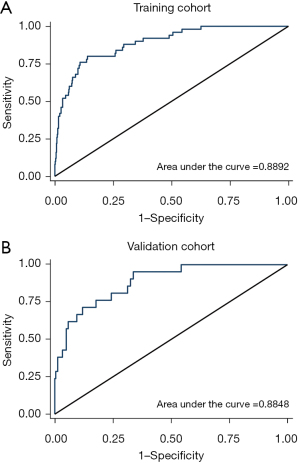
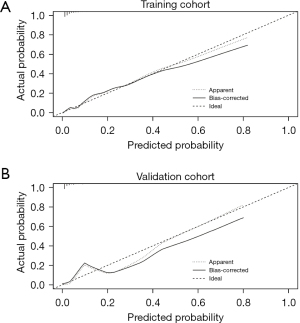
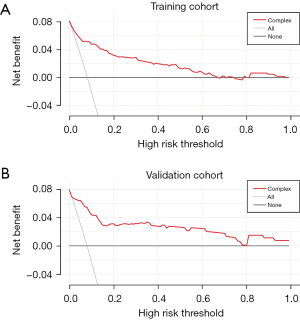
Discussion
Cardiac and large vessel surgery has long been considered one of the most difficult types of surgery in the field of surgery. At the same time, the vast majority of patients after ECAOCS need to be admitted to the ICU for postoperative special care. In order to better predict hospital mortality in patients underwent ECAOCS and to allow early intervention in patients at high risk of death, we need to develop a predictive model to help clinicians identify these patients at high risk of death in the postoperative period.
There are systems in place to specifically assess the surgical risk prior to cardiac surgery, such as the EuroSCORE, STS-PROM Score and German AV Score (18). EuroSCORE is a scoring system based on a database of nearly 20,000 consecutive cardiac surgery patients from 128 hospitals in eight European countries, which aims to find high-risk factors affecting the prognosis of cardiac surgery and to predict the risk of death in cardiac surgery patients by calculating a score for each patient (19). This scoring system has received widespread attention upon its release, and has been used in a large number of applications for preoperative risk assessment of coronary artery bypass grafting, heart valve surgery, and other surgeries with good predictive results (20-22). However, as this scoring system becomes widely used, some of its flaws are gradually discovered. One of the more prominent flaws is that this scoring system overestimates a patient’s mortality after cardiac surgery, and this flaw has been demonstrated in several studies (19,23,24). Therefore, in order to improve the predictive accuracy of EuroSCORE, the researchers have developed a more precise version through more in-depth studies: EuroSCORE II. Compared to EuroSCORE, EuroSCORE II provides better prediction of surgical mortality and offers greater flexibility in the inclusiveness of surgery (25,26). As we all know, no model is perfect and EuroSCORE II still has its shortcomings, such as the powerful discrimination (11).
In recent years, with the rapid development of computer technology such as machine learning, clinicians’ capacity to process and analyze massive amounts of clinical data has been greatly improved (27). At the same time, with the spread of electronic medical records and the establishment of large medical databases, it has become possible to carry out more in-depth studies of clinical problems. In addition, with the assistance of machine learning, newly developed prediction models have a more accurate and reliable ability to predict the occurrence and prognosis of diseases than previous models (28,29).
In this study, we developed a predictive model for predicting hospital mortality in postoperative patients by applying statistical methods such as LASSO regression analysis and nomogram, using a variety of clinical data from the MIMIC IV database of patients underwent ECAOCS in the first 24 hours after surgery. Through multivariate logistic regression analysis and LASSO regression analysis, we finally developed a predictive model with nine factors: AG, CVP, glucose, Cr, APTT, HCO3−, CVD, AMI, PVD. Finally, our model was validated by several metrics such as AUC, calibration curve, HL test and DCA. The final validation result is that our prediction model possesses a strong ability to predict postoperative hospital death in patients underwent ECAOCS.
Our study found that patients with CVD, AMI, and PVD had a higher risk of death after ECAOCS. The results of a study suggest that there are substantial associations between cardiovascular diseases and CVD and these associations have a significant impact on clinical outcomes (30). AMI is one of the most serious and life-threatening diseases. Myocardial injury is exacerbated during intraoperative cardiac arrest in patients with a past history of AMI and myocardial ischaemia and reperfusion after aortic opening can further exacerbate the degree of myocardial injury (31,32). PVD is one of the manifestations of systemic vascular ageing and can in some ways reflect the current state of the patient’s systemic vasculature (33,34). PVD may be a warning of systemic cardiovascular events.
ECAOCS cause electrolyte imbalance in patients to some extent due to heavy bleeding, continuous vasoactive drug administration, ventilator-assisted respiration, and large fluid intake. Previous studies have also shown that patients with metabolic acidosis have a significantly higher risk of death than normal patients (35,36). A retrospective study designed to examine the relationship between blood glucose and all-cause mortality, cardiovascular disease in patients without diabetes who presented to the emergency department showed that patients with disturbed blood glucose levels at the time of admission had a higher risk of death than patients with normal glucose tolerance. Patients with hyperglycemia had almost twice the risk of long-term mortality and more than double the risk of cardiovascular events compared with normal glucose tolerance patients (37). A retrospective observational study conducted at the US Academic Medical Centre examined acute kidney injury after cardiac surgery and developed a predictive model to predict acute kidney injury after cardiac surgery. In this model serum creatinine levels after cardiac surgery were highly correlated with acute kidney injury (38). Meanwhile, another study found that the severity of AKI was associated with increased short- and long-term mortality in cardiac ICU patients (39). A meta-analysis found that elevated central venous pressure was associated with increased mortality in critically ill patients, which is consistent with our previous studies (40). Coagulation abnormality is a high-risk factor for increased intraoperative and postoperative mortality in cardiac surgery (41,42). Bleeding due to coagulation abnormalities is something surgeons do not want to see during and after ECAOCS, and severe coagulation abnormalities can lead directly to surgical failure.
Our model predicts postoperative hospital mortality in patients underwent cardiac surgery by using factors that are routinely monitored and readily available after the patient’s admission to the ICU and has yielded satisfactory results, but our model still has some limitations. Firstly, our model is only internally validated in the same database and not externally in other databases. Therefore, the predictive power of the model needs to be validated in other databases in the future. Secondly, our study was an observational retrospective study and the use of the models identified in this study in customized treatment of patients needs to be further explored. Thirdly, since the data used to construct the predictive models in this study came from public databases, some of the factors we wanted to use were not available, which brings irreparable regret to the study. Finally, in order to ensure that the study included the most original data, we excluded from the study all patients with more than 5% missing data. This stringent screening criterion resulted in a large number of patients being discarded, so the sample size of the study was therefore severely reduced and bias was inevitable. All these shortcomings need to be continued to be improved in future studies, so that the model can better serve the clinical work.
Conclusions
Our study developed a model for predicting postoperative hospital mortality in patients underwent ECAOCS by incorporating AG, CVP, glucose, Cr, APTT, HCO3−, CVD, AMI, and PVD from the first 24 hours after admission to the ICU, and verified the validity of the model by using analytical methods, such as AUC, calibration curve, and decision curve. The model can be well used in clinical work to help doctors screen high-risk groups, take targeted treatments, save medical resources, and reduce the postoperative in-hospital mortality of ECAOCS.
Acknowledgments
Funding: This work was supported by
Footnote
Reporting Checklist: The authors have completed the TRIPOD reporting checklist. Available at https://jtd.amegroups.com/article/view/10.21037/jtd-24-24/rc
Peer Review File: Available at https://jtd.amegroups.com/article/view/10.21037/jtd-24-24/prf
Conflicts of Interest: All authors have completed the ICMJE uniform disclosure form (available at https://jtd.amegroups.com/article/view/10.21037/jtd-24-24/coif). The authors have no conflicts of interest to declare.
Ethical Statement: The authors are accountable for all aspects of the work in ensuring that questions related to the accuracy or integrity of any part of the work are appropriately investigated and resolved. The study was conducted in accordance with the Declaration of Helsinki (as revised in 2013).
Open Access Statement: This is an Open Access article distributed in accordance with the Creative Commons Attribution-NonCommercial-NoDerivs 4.0 International License (CC BY-NC-ND 4.0), which permits the non-commercial replication and distribution of the article with the strict proviso that no changes or edits are made and the original work is properly cited (including links to both the formal publication through the relevant DOI and the license). See: https://creativecommons.org/licenses/by-nc-nd/4.0/.
References
- Coisne A, Lancellotti P, Habib G, et al. ACC/AHA and ESC/EACTS Guidelines for the Management of Valvular Heart Diseases: JACC Guideline Comparison. J Am Coll Cardiol 2023;82:721-34. [Crossref] [PubMed]
- Peters AS, Duggan JP, Trachiotis GD, et al. Epidemiology of Valvular Heart Disease. Surg Clin North Am 2022;102:517-28. [Crossref] [PubMed]
- Coffey S, Roberts-Thomson R, Brown A, et al. Global epidemiology of valvular heart disease. Nat Rev Cardiol 2021;18:853-64. [Crossref] [PubMed]
- Task Force Members. 2019 EACTS/EACTA/EBCP guidelines on cardiopulmonary bypass in adult cardiac surgery. Br J Anaesth 2019;123:713-57. [Crossref] [PubMed]
- Bartoszko J, Karkouti K. Managing the coagulopathy associated with cardiopulmonary bypass. J Thromb Haemost 2021;19:617-32. [Crossref] [PubMed]
- Ma HP, Xu WF, Yu J, et al. Heparin sensitivity and postoperative blood loss in patients undergoing cardiac surgery with cardiopulmonary bypass. Eur J Anaesthesiol 2020;37:162-9. [Crossref] [PubMed]
- Nguyen M, Stiel L, Guilloteau A, et al. Leukocyte cell population data in patients with cardiac surgery and cardiopulmonary bypass: A potential readily available tool to monitor immunity. Front Immunol 2022;13:1101937. [Crossref] [PubMed]
- Ranucci M, Ambrogi F, Pistuddi V. Cardiac surgery associated acute kidney injury and the role of cardiopulmonary bypass technique. J Thorac Cardiovasc Surg 2019;157:301. [Crossref] [PubMed]
- Squiccimarro E, Stasi A, Lorusso R, et al. Narrative review of the systemic inflammatory reaction to cardiac surgery and cardiopulmonary bypass. Artif Organs 2022;46:568-77. [Crossref] [PubMed]
- Massoth C, Zarbock A, Meersch M. Acute Kidney Injury in Cardiac Surgery. Crit Care Clin 2021;37:267-78. [Crossref] [PubMed]
- Nashef SA, Roques F, Sharples LD, et al. EuroSCORE II. Eur J Cardiothorac Surg 2012;41:734-44; discussion 744-5. [Crossref] [PubMed]
- O'Brien SM, Shahian DM, Filardo G, et al. The Society of Thoracic Surgeons 2008 cardiac surgery risk models: part 2--isolated valve surgery. Ann Thorac Surg 2009;88:S23-42. [Crossref] [PubMed]
- Balachandran VP, Gonen M, Smith JJ, et al. Nomograms in oncology: more than meets the eye. Lancet Oncol 2015;16:e173-80. [Crossref] [PubMed]
- Johnson AEW, Bulgarelli L, Shen L, et al. MIMIC-IV, a freely accessible electronic health record dataset. Sci Data 2023;10:1. [Crossref] [PubMed]
- Hayati Rezvan P, Lee KJ, Simpson JA. The rise of multiple imputation: a review of the reporting and implementation of the method in medical research. BMC Med Res Methodol 2015;15:30. [Crossref] [PubMed]
- Friedman J, Hastie T, Tibshirani R. Regularization Paths for Generalized Linear Models via Coordinate Descent. J Stat Softw 2010;33:1-22. [Crossref] [PubMed]
- Mullah MAS, Hanley JA, Benedetti A. LASSO type penalized spline regression for binary data. BMC Med Res Methodol 2021;21:83. [Crossref] [PubMed]
- Raith EP, Udy AA, Bailey M, et al. Prognostic Accuracy of the SOFA Score, SIRS Criteria, and qSOFA Score for In-Hospital Mortality Among Adults With Suspected Infection Admitted to the Intensive Care Unit. JAMA 2017;317:290-300. [Crossref] [PubMed]
- Gogbashian A, Sedrakyan A, Treasure T. EuroSCORE: a systematic review of international performance. Eur J Cardiothorac Surg 2004;25:695-700. [Crossref] [PubMed]
- Abildstrøm SZ, Hvelplund A, Rasmussen S, et al. Prognostic information in administrative co-morbidity data following coronary artery bypass grafting. Eur J Cardiothorac Surg 2010;38:573-6. [Crossref] [PubMed]
- Barili F, Di Gregorio O, Capo A, et al. Aortic valve replacement: reliability of EuroSCORE in predicting early outcomes. Int J Cardiol 2010;144:343-5. [Crossref] [PubMed]
- Gummert JF, Funkat A, Osswald B, et al. EuroSCORE overestimates the risk of cardiac surgery: results from the national registry of the German Society of Thoracic and Cardiovascular Surgery. Clin Res Cardiol 2009;98:363-9. [Crossref] [PubMed]
- Bhatti F, Grayson AD, Grotte G, et al. The logistic EuroSCORE in cardiac surgery: how well does it predict operative risk? Heart 2006;92:1817-20. [Crossref] [PubMed]
- Karthik S, Srinivasan AK, Grayson AD, et al. Limitations of additive EuroSCORE for measuring risk stratified mortality in combined coronary and valve surgery. Eur J Cardiothorac Surg 2004;26:318-22. [Crossref] [PubMed]
- Ad N, Holmes SD, Patel J, et al. Comparison of EuroSCORE II, Original EuroSCORE, and The Society of Thoracic Surgeons Risk Score in Cardiac Surgery Patients. Ann Thorac Surg 2016;102:573-9. [Crossref] [PubMed]
- Guida P, Mastro F, Scrascia G, et al. Performance of the European System for Cardiac Operative Risk Evaluation II: a meta-analysis of 22 studies involving 145,592 cardiac surgery procedures. J Thorac Cardiovasc Surg 2014;148:3049-57.e1. [Crossref] [PubMed]
- Hu R, Zhou S, Liu Y, et al. Margin-Based Pareto Ensemble Pruning: An Ensemble Pruning Algorithm That Learns to Search Optimized Ensembles. Comput Intell Neurosci 2019;2019:7560872. [Crossref] [PubMed]
- Kim HY, Lampertico P, Nam JY, et al. An artificial intelligence model to predict hepatocellular carcinoma risk in Korean and Caucasian patients with chronic hepatitis B. J Hepatol 2022;76:311-8. [Crossref] [PubMed]
- Khurshid S, Friedman S, Reeder C, et al. ECG-Based Deep Learning and Clinical Risk Factors to Predict Atrial Fibrillation. Circulation 2022;145:122-33. [Crossref] [PubMed]
- Nakai M, Iwanaga Y, Sumita Y, et al. Associations among cardiovascular and cerebrovascular diseases: Analysis of the nationwide claims-based JROAD-DPC dataset. PLoS One 2022;17:e0264390. [Crossref] [PubMed]
- Kadesjö E, Roos A, Siddiqui A, et al. Acute versus chronic myocardial injury and long-term outcomes. Heart 2019;105:1905-12. [Crossref] [PubMed]
- Algoet M, Janssens S, Himmelreich U, et al. Myocardial ischemia-reperfusion injury and the influence of inflammation. Trends Cardiovasc Med 2023;33:357-66. [Crossref] [PubMed]
- Leopold JA. Editorial commentary: Premature peripheral arterial disease: A consequence of accelerated vascular aging due to less than ideal cardiovascular health? Trends Cardiovasc Med 2021;31:359-60. [Crossref] [PubMed]
- Santoro L, Flex A, Nesci A, et al. Association between peripheral arterial disease and cardiovascular risk factors: role of ultrasonography versus ankle-brachial index. Eur Rev Med Pharmacol Sci 2018;22:3160-5. [PubMed]
- Jung B, Rimmele T, Le Goff C, et al. Severe metabolic or mixed acidemia on intensive care unit admission: incidence, prognosis and administration of buffer therapy. A prospective, multiple-center study. Crit Care 2011;15:R238. [Crossref] [PubMed]
- Fujii T, Udy AA, Nichol A, et al. Incidence and management of metabolic acidosis with sodium bicarbonate in the ICU: An international observational study. Crit Care 2021;25:45. [Crossref] [PubMed]
- Djupsjö C, Kuhl J, Andersson T, et al. Admission glucose as a prognostic marker for all-cause mortality and cardiovascular disease. Cardiovasc Diabetol 2022;21:258. [Crossref] [PubMed]
- Demirjian S, Bashour CA, Shaw A, et al. Predictive Accuracy of a Perioperative Laboratory Test-Based Prediction Model for Moderate to Severe Acute Kidney Injury After Cardiac Surgery. JAMA 2022;327:956-64. [Crossref] [PubMed]
- Jentzer JC, Breen T, Sidhu M, et al. Epidemiology and outcomes of acute kidney injury in cardiac intensive care unit patients. J Crit Care 2020;60:127-34. [Crossref] [PubMed]
- Chen CY, Zhou Y, Wang P, et al. Elevated central venous pressure is associated with increased mortality and acute kidney injury in critically ill patients: a meta-analysis. Crit Care 2020;24:80. [Crossref] [PubMed]
- Mumford AD, Harris J, Plummer Z, et al. Near-patient coagulation testing to predict bleeding after cardiac surgery: a cohort study. Res Pract Thromb Haemost 2017;1:242-51. [Crossref] [PubMed]
- Guzzetta NA, Allen NN, Wilson EC, et al. Excessive postoperative bleeding and outcomes in neonates undergoing cardiopulmonary bypass. Anesth Analg 2015;120:405-10. [Crossref] [PubMed]