Emergent robotic surgery conversions: improving operating room team performance through high fidelity simulations
Highlight box
Key findings
• Team-based training of emergency undocking protocols and checklists improve operating room team performance during emergency robotic undocking for bleeding.
What is known and what is new?
• Checklists are beneficial and improve outcomes in emergency situations.
• Simulations and didactic education can improve team performance and decrease critical missed steps in team implementation of protocols for emergency conversion from robotic thoracic surgery to open.
What is the implication, and what should change now?
• Thoracic surgery teams should engage in educational activities to prepare for low frequency, high fidelity events to improve patient safety.
Introduction
In the past 20 years, robotic surgery has become increasingly adopted. Nevertheless, it has sparked controversy in the areas of financial expense and patient safety. The main safety concerns pertain to intra-operative emergencies that require expedient open conversion when the surgeon is not at the bedside. Significant bleeding from vascular injury can potentially occur in all operations regardless of type. However, pulmonary resections are unique in their need for pulmonary artery (PA) dissection, during which vessel injury may lead to catastrophic bleeding in a very short timeframe. A recent review of lobectomies from the Society of Thoracic Surgeons database showed a conversion rate from robotic to open approaches of 6% (1). Conversion was associated with increased mortality, major complications, and intraoperative and postoperative transfusions (1). Prior studies focusing on gynecologic and urologic intra-operative emergencies have found that pre-defined emergency protocols have significantly improved undocking times and overall medical responses (2-4). Similarly, emergency protocol utilization during non-robotic intra-operative emergencies has led to significantly higher adherence to best practices and a significant reduction in failure of adherence to critical management steps after initial implementation (5).
There is a paucity of emergency protocols associated with robotic-assisted thoracic procedures. In addition, there is limited data on the best approach to adapting protocols to improve operating room (OR) team performance. Our hypothesis was that protocol-based training programs of standardized checklists would improve team performance during simulated emergency robotic conversion as measured by completion of critical steps and time to conversion. We produced new emergency protocols specific to robot-assisted thoracic surgery and then created two educational programs to teach the protocols: (I) a high-fidelity simulation-based educational program and (II) a video-based didactic educational program. These two educational modalities were then tested for feasibility and effectiveness.
Methods
OR team design and simulation model
A total of six different OR teams participated in the study (Teams 1–6) (Figure 1). Each OR team consisted of six members including an OR circulating registered nurse, a certified OR scrub technician, a general surgery resident [post-graduate year (PGY)-2 or -4], a thoracic surgery attending, an anesthesia resident (PGY-3 or -4), and an anesthesia attending. Teams were recruited via emails. Whenever possible, volunteers chose the times they were available to participate without knowing the difference between groups. All simulation participants completed pre-simulation surveys. The pre-simulation surveys were created by the study team to assess for the participants prior experience and confidence level in addressing emergency situations in the OR. Participants were pre-briefed and given information about the simulated patient and the KindHeart Thoracic Surgical Simulator manikin (Intuitive Surgical, Inc., Chapel Hill, NC, USA), the REALTi360 vital signs simulator (iSimulate, Albany, NY, USA). The porcine KindHeart thoracic model included a fully perfused beating heart and lungs. Injury to the great vessels led to significant simulated bleeding (Figures S1,S2).
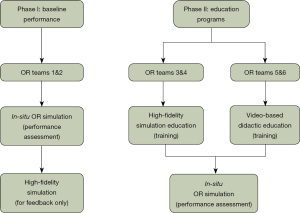
Simulation design
The simulated operative case was an elective robotic lobectomy in a patient with no known pre-operative comorbidities. The five consecutive emergency scenarios were: (I) massive hemorrhage from a pulmonary artery, (II) decision to convert to open, (III) hemodynamic instability, (IV) pulseless electrical activity (PEA) arrest, and (V) unstable ventricular fibrillation. The five scenarios were designed with an expert team including thoracic surgery and anesthesia attendings with support from simulation experts from the UMass simulation laboratory (Figure S3). To begin each high-fidelity simulation, the respective OR teams gained intravenous access in the manikin arm, intubated the manikin airway, performed preoperative briefing, and prepped and draped the KindHeart manikin. The anesthesia team had access to various simulated medications and an arterial line set up. The first scenario was initiated after the thoracic surgeon purposefully cut the pulmonary artery, with each of the five emergency scenarios occurring consecutively in order without interruption.
Phase I—Emergency checklist protocol development and in-situ OR simulations
The checklists and protocol (Figure S3) were created through two separate round table discussions with key stake holders as a single institution, including thoracic surgery attendings, thoracic anesthesia attendings, OR staff, and staff from the UMass department of Patient Quality and Safety. The first two simulation teams (Teams 1 and 2) were considered the baseline group and did not receive any specific training with respect to checklist protocols prior to their in-situ OR simulation. Teams 1 and 2 (baseline) each completed two simulations spaced two weeks apart. The first simulations took place in the OR, without prior knowledge of the scenarios. The second was done in a healthcare simulation center, where the main goal was discussion and feedback for each scenario. After Teams 1 and 2 completed their simulation sessions, each respective group completed an oral debriefing session and written post-simulation surveys. Latent safety threats (LSTs) were identified and further processed with failure mode and effects analysis (FMEA) with calculations of risk priority number (RPN) for each LST. The results were previously published (6). The protocol and checklists were fine-tuned by the study team following these sessions and critical checklist items were identified (Figure 1).
Phase II—Educational programs to teach emergency checklist protocols
The education program consisted of two different approaches to learning the protocols and checklists. Teams 3 and 4 underwent practice-based learning in a healthcare simulation center, whereas Teams 5 and 6 received interactive video-based sessions. During the high-fidelity lab simulation sessions completed by Teams 3 and 4, participants received feedback after completing each scenario. Standardized pre-brief sessions were performed in addition to faculty and simulation educator co-facilitated debrief sessions.
The video-based didactic session for Teams 5 and 6 was approximately one hour in length and included a PowerPoint presentation with video clips from previous simulation sessions. This presentation was designed by the study team utilizing the critical checklist items identified previously. The session was interactive and included discussion points that allowed participants to work through the checklists from their personal perspectives. During the in-situ simulations for Teams 3–6, the protocols and checklists were available in the ORs as reference. Teams 3–6 underwent their respective education programs followed by in-situ simulation in the OR after 2 weeks.
Statistical analysis
Quantitative measures
All simulations and education sessions were video recorded for accurate measurement of quantitative measures including: (I) time from decision for open conversion to attending at bedside, (II) time to removal of all robotic instruments (measured as time from initial instrument removal to last instrument removal), and (III) total undocking time (measured as time from instruction to undock to all robot arms completely undocked and stowed). Critical checklist items are listed in Figure 2. Critical checklist items were considered complete if seen or heard on video recordings of the in-situ OR simulations. All videos were scored by three different observers (B.B., K.M.D., F.L.). The percentage of critical checklist items missed was calculated for each team. The interrater reliability was measured by the level of agreement (93%) and the Kappa of 0.463, 95% confidence interval (CI): 0.116 to 0.709, P=0.01. We performed a descriptive analysis, then used an independent samples Kruskal-Wallis test, with a 95% CI, to test for statistically significant differences across the groups. The data was analyzed with SAS studio v3.81.
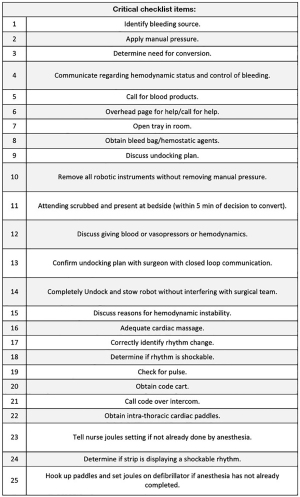
Qualitative measures
Participants completed pre- and post-surveys for each simulation and education session (Appendices 1,2). Likert scales were used and later converted to numerical values for statistical analysis (7,8). The study was conducted in accordance with the Declaration of Helsinki (as revised in 2013). This study was approved by the institutional review board at the University of Massachusetts Chan Medical School (No. H00022131) and informed consent was obtained from all simulation participants prior to study initiation.
Results
Between April 2021 and December 2021, six different OR teams underwent a total of 50 simulated scenarios in 10 sessions. Thirty of the scenarios were completed in the OR and 20 in the simulation laboratory. There was a total of 36 participants. All except for one participant were based in the same institution. Due to unforeseen circumstances, last-minute substitutions occurred for two nurses and one anesthesia attending. Three thoracic surgeons participated. Each surgeon was on two teams with the same intervention i.e., one surgeon was in Teams 1 and 2, another in Teams 3 and 4, and an additional in Teams 5 and 6.
Objective performance evaluations
The two baseline OR teams (Teams 1 and 2) completed 90% (average 22.5/25) of the critical check list items (Table 1). In contrast, after either simulation or didactic education, Teams 3–6 successfully completed an average of 99% (24.75/25) of critical tasks (P=0.08). The improvement was statistically significant at the level of 0.10. A significance level of 0.10 was selected due to our small sample size and the relevance of this study for informing future work (9). After simulation training, Teams 3 and 4 had no critical misses. The two OR teams (5 and 6) who completed the didactic video-based education sessions achieved an average of 98% (24.5/25) critical checklist points. Team 1 had one critical miss, due to lack of discussion of undocking plan. Team 2 had the highest number of critical misses, which included lack of discussion of undocking plan, failure to maintain manual pressure on the bleed while removing robotic instruments, lack of closed loop communication between the surgery and nursing team members during robot undocking, and physical interference with surgical team during the robotic stowing process. Team 5’s one critical miss was failure to recognize shockable rhythm.
Table 1
Team | Phase and education type | Time from decision to convert to attending at bedsidea | P valueb | Total time to remove all robotic instrumentsa | P valueb | Total undocking timea | P valueb | Total number of critical checklist points completed (n=25) | Percentage of critical checklist complete | P valueb |
---|---|---|---|---|---|---|---|---|---|---|
1 | Phase I: baseline | 174 | 232 | 46 | 24 | 96.0 | ||||
2 | Phase I: baseline | 90 | 89 | 214 | 21 | 84.0 | ||||
1+2 | Baseline mean | 132 | 160.5 | 130 | 22.5 | 90.0 | ||||
3 | Phase II: simulation | 193 | 266 | 31 | 25 | 100.0 | ||||
4 | Phase II: simulation | 125 | 229 | 66 | 25 | 100.0 | ||||
3+4 | Simulation mean | 159 | 247.5 | 48.5 | 25 | 100.0 | ||||
5 | Phase II: didactic | 91 | 227 | 37 | 24 | 96.0 | ||||
6 | Phase II: didactic | 120 | 251 | 32 | 25 | 100.0 | ||||
5+6 | Didactic mean | 105.5 | 239 | 34.5 | 24.5 | 98.0 | ||||
1–6 | Mean overall | 132.2 | 0.64 | 215.7 | 0.36 | 71 | 0.17 | 24 | 96.0 | 0.08 |
a, times displayed as seconds; b, baseline vs. any education (simulation or didactic).
Time to task completion
In all simulations, the surgical attendings were scrubbed and present at the bedside in less than 200 seconds from the decision to convert from robotic to open (Table 1). The observed time to bedside ranged from 90–193 seconds, with a mean of 132.2 seconds. Total time to remove all robotic instruments ranged from 89–266 seconds, with a mean of 215.7 seconds. Total undocking time ranged from 31–214 seconds, with a mean 71 seconds (Table 1).
Discussion
In a study of in-situ OR simulations of thoracic surgery robotic emergency scenarios, we found that introduction of checklists and protocols through high-fidelity laboratory simulations and interactive presentations improved team performance and decreased critical missed steps. Participant survey responses (Appendices 1,2) from the teams who underwent education demonstrated increased confidence and understanding of their role during intraoperative robotic crises. Almost all participants (94%) agreed pre- and post-simulation that high-fidelity simulations of intraoperative emergencies are beneficial and provide a helpful training opportunity to practice high-stakes, low-frequency events. The complete survey results were previously published (6). High-fidelity simulations allowed for hands-on team-based learning that may be superior to didactic learning. Although only four teams were in the education arm (phase II, Teams 3–6), the two teams who underwent high-fidelity simulation learning (Teams 3 and 4) successfully accomplished all critical tasks. Performance after video-based didactic learning sessions was also superior to the baseline teams. However, there was one critical miss related to rhythm recognition. In all in-situ simulations, the time of surgeon to bedside was less than 200 seconds. Most critical failures in the baseline groups were related to team collaboration, including closed loop communication, discussion of undocking plans, and removal of robotic arms at the appropriate time without interfering with the surgical team.
Published studies regarding the compared efficacy of teaching emergency checklists with simulation-based versus video-based didactic educational programs are limited, especially in the context of robotic surgery. Nevertheless, a pilot study has shown that simulation training may be more effective than didactic education for teaching the management of abdominal compartment syndrome (10). A simulation-based curriculum has also been successfully used to teach the “situation, perspective, invitation, knowledge, empathy, summary” (SPIKES) protocol for delivering bad news (11). A group in 2021 demonstrated that an in-situ simulation program, initially with video-assisted thoracoscopic surgery (VATS) and later switched to robotic-assisted thoracoscopic surgery (RATS), was a feasible program at their institution (12). Their methods differed slightly in that simulation scenarios were constructed from root-cause analysis of cases discussed at Morbidity & Mortality conferences, as opposed to systems issues identified from simulation debriefing sessions. In addition, only two scenarios, hemorrhage, and contralateral pneumothorax with acute hypoxia, were included in their simulations. The checklist available to the team was “Code Red”, which was initially designed by Cerfolio et al. in 2016 (13). The participants were assessed on technical skills, non-technical skills for surgeons (NOTECHS), and stress management. In a separate study conducted in 2018, Ballas et al. created an educational program for gynecologic surgery that used formative simulation and debriefing followed by a lecture (2). In the study, only the surgeons were active participants. The other roles, like circulators and scrub techs, were fulfilled by embedded standardized persons. The implementation of their curriculum led to greater confidence, baseline knowledge assessments and performance of critical actions (such as undocking time) by the surgeon (2).
The benefits of emergency checklists have been demonstrated in aviation, aeronautics, as well as medicine (12,14). In a landmark study on OR checklists by Haynes et al., a multicenter study found that the physical availability of checklists reduced the rate of death from 1.5% to 0.8% (P=0.003), while decreasing the rate of inpatient complications from 11% to 7.0% (P<0.001) (5). In our study, physical copies of emergency robotic undocking protocols were available to all team members in phase II. This can be more permanently displayed in the format of a poster, a proposed version of which is visible in Figure 3.
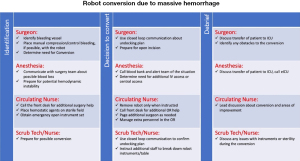
It is possible that having the checklist readily available improves performance. However, the team interaction and skills required for emergency undocking and resuscitation of thoracic surgery patients in lateral positioning are complex. Hands-on skills like expedient undocking of the robot can only be learned through practice. Similarly, closed-loop communication during emergency situations is also a learned skill, not easily accomplished as a checklist item. At the same time, overall familiarity with the sequence of responses to unexpected catastrophic bleeding is essential. Ultimately, both team-based simulations and checklists are important in the success of an OR team’s response to catastrophic bleeding during robotic surgery.
A large part of OR team performance is dependent on each team member’s experience. For example, a new surgical scrub technologist may not be as effective as a seasoned technologist dedicated to robotic thoracic surgeries. While some institutions have teams that are exclusively dedicated to certain specialties, others do not. At our institution, there is a limited number of OR staff members who routinely help with thoracic surgery cases. At times, robotic thoracic operations are done with OR teams without significant experience with thoracic surgeries. The difference in staff and anesthesia experience cannot be always controlled in simulations. Team 1 from phase I was composed of OR staff members with thoracic surgical experience. As a result, they performed well with only one critical miss despite lack of preparation.
Although the study was not designed to address the frequency of education or how experienced participants are, there is evidence that education (either simulation or video-based) did improve team performance overall. Emergent conversions during robotic operations are low frequency events. In an analysis of Society of Thoracic Surgery data by Servais et al., 6% of robotic surgeries ended with conversion to open approach of which 17.9% were converted emergently (1). Given the infrequency, simulations learning would still have a role in improving outcomes even in institutions with dedicated specialty teams. Simulation can also help to address logistical questions such as which team member ought to be responsible for the checklist in an emergency scenario.
One of the disadvantages of simulation learning is the resources and staff time requirement. We demonstrated that simulations of complex undocking scenarios can be accomplished without significant time investment. Simulations for each team in the laboratory lasted for approximately two hours. If there is adequate preparation, three teams can complete simulations in one day. If simulation learning is not feasible, then interactive video-based learning can still be performed, which requires less resources. Finally, an approach that maximizes long term learning and maintenance of skills may include simulations followed by periodic video-based learning at regular intervals.
There are several limitations in our study. We performed a single-institution investigation, considering educational methods in the context of one surgical specialty. Our results may not be applicable to all robotic practices. In addition, only three thoracic surgeons and five anesthesiologists were involved in the six OR simulations. As a result, there may be an over-estimation in surgeon performance. However, the surgeons did not cross intervention groups. There was one surgeon for phase I, and two for phase II. The surgeons in phase II participated in teams that received either simulation training or video-based learning. Although 50 simulation scenarios were carried out, only 6 OR teams participated in the study. Because of the low number of teams, our results in critical misses only trended toward statistical significance. The study does not address whether simulation or video-based learning is more effective. Finally, we did not follow the participants’ knowledge after the in-situ simulations and therefore cannot conclude how frequently the team-based learning should be performed.
Future research should include simulations in a multi-institutional, multi-specialty collaboration. Current literature is limited to mostly single-institution studies involving only one surgical specialty. The available conclusions have been limited to expert opinions of single surgeons. Therefore, expansion of simulation education research in robotic surgery is crucial, as any robotic surgery could result in catastrophic bleeding. With more simulation data, further refinement can be made to the standardized protocol, leading to implementation of society-based guidelines.
Conclusions
Emergency checklist protocols can be used to improve thoracic surgery team adherence to best practices in the context of high-stakes, low-frequency events. In-situ and lab-based simulations of emergency scenarios followed by debriefing, video analysis, and round table discussion can be used to develop an emergency checklist protocol for emergent undocking of the robot in the context of massive hemorrhage and subsequent clinical decompensation. This study shows that targeted educational programs, whether didactic or simulation based, can feasibly and effectively be used to teach OR teams these checklist protocols. Further studies are needed to optimize emergency undocking protocols and elucidate the role of simulation and video-based learning in other surgical specialties.
Acknowledgments
Deceased author: The authors would like to acknowledge and thank Dr. Bryce Bludevich, who was the true force behind the study. She was an essential part of this project from its conception until the very end. The simulations would not have been possible without her dedication and ingenuity. We mourn her untimely passing just before the submission of the manuscript.
Funding: This work was supported by
Footnote
Data Sharing Statement: Available at https://jtd.amegroups.com/article/view/10.21037/jtd-24-291/dss
Peer Review File: Available at https://jtd.amegroups.com/article/view/10.21037/jtd-24-291/prf
Conflicts of Interest: All authors have completed the ICMJE uniform disclosure form, except for author Bryce Bludevich, who is deceased (available at https://jtd.amegroups.com/article/view/10.21037/jtd-24-291/coif). K.U. reports grant funding from the Intuitive Foundation to his institution. The other authors have no conflicts of interest to declare.
Ethical Statement:
Open Access Statement: This is an Open Access article distributed in accordance with the Creative Commons Attribution-NonCommercial-NoDerivs 4.0 International License (CC BY-NC-ND 4.0), which permits the non-commercial replication and distribution of the article with the strict proviso that no changes or edits are made and the original work is properly cited (including links to both the formal publication through the relevant DOI and the license). See: https://creativecommons.org/licenses/by-nc-nd/4.0/.
References
- Servais EL, Miller DL, Thibault D, et al. Conversion to Thoracotomy During Thoracoscopic vs Robotic Lobectomy: Predictors and Outcomes. Ann Thorac Surg 2022;114:409-17. [Crossref] [PubMed]
- Ballas D, Cesta M, Roulette GD, et al. Emergency Undocking in Robotic Surgery: A Simulation Curriculum. J Vis Exp 2018;57286. [PubMed]
- Ballas DA, Cesta M, Gothard D, et al. Emergency Undocking Curriculum in Robotic Surgery. Cureus 2019;11:e4321. [PubMed]
- O’Sullivan OE, O’Sullivan S, Hewitt M, et al. Da Vinci robot emergency undocking protocol. J Robot Surg 2016;10:251-3. [Crossref] [PubMed]
- Haynes AB, Weiser TG, Berry WR, et al. A surgical safety checklist to reduce morbidity and mortality in a global population. N Engl J Med 2009;360:491-9. [Crossref] [PubMed]
- Bludevich BM, Buettner H, Hazeltine M, et al. Use of In-Situ Simulation Based Clinical Systems Test of Thoracic Robotic Surgery Emergencies. J Surg Res 2022;276:37-47. [Crossref] [PubMed]
- Jamieson S. Likert scales: how to (ab)use them. Med Educ 2004;38:1217-8. [Crossref] [PubMed]
- Sullivan GM, Artino AR Jr. Analyzing and interpreting data from likert-type scales. J Grad Med Educ 2013;5:541-2. [Crossref] [PubMed]
- Thiese MS, Ronna B, Ott U. P value interpretations and considerations. J Thorac Dis 2016;8:E928-31. [Crossref] [PubMed]
- Saraswat A, Bach J, Watson WD, et al. A pilot study examining experiential learning vs didactic education of abdominal compartment syndrome. Am J Surg 2017;214:358-64. [Crossref] [PubMed]
- Ghoneim N, Dariya V, Guffey D, et al. Teaching NICU Fellows How to Relay Difficult News Using a Simulation-Based Curriculum: Does Comfort Lead to Competence? Teach Learn Med 2019;31:207-21. [Crossref] [PubMed]
- Baste JM, Bottet B, Selim J, et al. Implementation of simulation-based crisis training in robotic thoracic surgery: how to improve safety and performance? J Thorac Dis 2021;13:S26-34. [Crossref] [PubMed]
- Cerfolio RJ, Bess KM, Wei B, et al. Incidence, Results, and Our Current Intraoperative Technique to Control Major Vascular Injuries During Minimally Invasive Robotic Thoracic Surgery. Ann Thorac Surg 2016;102:394-9. [Crossref] [PubMed]
- Elmezzi K, Deering S. Checklists in emergencies. Semin Perinatol 2019;43:18-21. [Crossref] [PubMed]