This article has an erratum available at: http://dx.doi.org/10.21037/jtd-2024-03 the article has been update on 2024-09-10 at here.
Machine learning-based prediction of off-pump coronary artery bypass grafting-associated acute kidney injury
Highlight box
Key findings
• The gradient-boosting decision tree model was deemed the best model for predicting off-pump coronary artery bypass grafting (OPCABG)-associated acute kidney injury (AKI) (OPCABG-AKI) out of a set of eight machine learning models. The addition of intraoperative time series data in addition to baseline data may improve predictive performance.
What is known and what is new?
• OPCABG is one of the major cardiac operations leading to cardiac surgery associated-acute kidney injury (CSA-AKI). Early recognition and timely intervention have important clinical significance for CSA-AKI.
• This study aimed to establish a prediction model of OPCABG-AKI through machine learning method.
What is the implication, and what should change now?
• Early recognition and timely intervention have important clinical significance for OPCABG-AKI.
Introduction
Acute kidney injury (AKI) is a serious complication after cardiac surgery (1). A meta-analysis (2) reported that the incidence of cardiac surgery-associated acute kidney injury (CSA-AKI) was approximately 26.0–28.5%. The 28- and 90-day mortality rate of patients with CSA-AKI have been shown to reach 10.7% and 30%, respectively (3). The pathogenesis of CSA-AKI is complex and includes ischemia-reperfusion injury, inflammation from surgical trauma, oxidation, and other factors (4,5). Although off-pump coronary artery bypass grafting (OPCABG) can avoid ischemia-reperfusion injury (6), the hemodynamic impact of the procedure and the possible incomplete revascularization can still increase the risk of off-pump coronary artery bypass grafting-associated acute kidney injury (OPCABG-AKI) (7-9). Therefore, it is of great clinical significance to establish an accurate prediction model for OPCABG-AKI to identify the high-risk patients (10,11). Recently, CSA-AKI has been predicted by machine learning in many studies (12-15). However, these studies included a large number of surgical types, inconsistent definitions of AKI and incorporated few intraoperative data (16). In this study, we compared the performance of eight different machine learning models to predict OPCABG-AKI, in order to select the optimal model for identifying high-risk patients early and guiding perioperative clinical decision-making. We present this article in accordance with the TRIPOD reporting checklist (available at https://jtd.amegroups.com/article/view/10.21037/jtd-24-711/rc).
Methods
Study population
The preoperative and intraoperative data of 1,041 patients who underwent OPCABG in Chest Hospital, Tianjin University from June 1, 2021 to April 30, 2023 were collected. The average age of these patients was 67.69±6.59 years, and the number of males and females was 743 and 298, respectively. After screening and excluding patients with missing data, the data of 701 patients were finally included in the dataset. The study was conducted in accordance with the Declaration of Helsinki (as revised in 2013). The study was approved by the Medical Ethics Committee of Chest Hospital, Tianjin University (No. 2021 KY-008-01). The requirement for informed consent was waived due to the retrospective nature of this study. The confidentiality and privacy of patients was guaranteed.
OPCABG-AKI diagnostic criteria
The definition of AKI was based on the serum creatinine (SCr) based Kidney Disease Improving Global Outcomes (KDIGO) criteria (17). However, the urine output criteria were not used due to lack of data. The diagnostic criteria were therefore as follows: SCr rises by ≥26.5 µmol/L (≥0.3 mg/dL) or SCr rises to ≥1.5 times compared to baseline within 7 days after surgery; or need for renal replacement therapy.
Data collection and data preprocessing
The training set comprised 80% of the dataset and the test set comprised the remaining 20% of the dataset, including baseline data and intraoperative time series data. The baseline data included the preoperative data, intraoperative variables, and discrete variable data. The indicators with a missing rate over 10% were deleted, and then the remaining values were processed. Intraoperative time series data were collected by LiDCO hemodynamic monitor (Masimo, Irvine, CA, USA), Nonin oximeter (Nonin Medical, Plymouth, MN, USA), Philips multi-parameter portable ECG monitor (Philips, Amsterdam, The Netherlands), and Ohmeda anesthesia machine (GE Healthcare, Chicago, IL, USA), and the sampling frequency was adjusted to 1 Hz. Variables with the same value were considered as outliers, and indicators with an outlier rate of more than 10% were removed, and then missing numerical variables were imputed by the upper and lower means, and modal imputation was used for categorical variables.
Predictors
The predictors used for machine learning model development were detailed in Table S1.
Statistical analysis
A total of eight machine learning models were trained to predict OPCABG-AKI based on the baseline data only, including logistic regression (LR), support vector machine (SVM), decision tree (DT), random forest (RF), k-nearest neighbor (KNN), gradient-boosting decision tree (GBDT), adaptive boosting (AdaBoost), and eXtreme gradient boosting (XGBoost). In this study, k-fold cross-validation was adopted, and k was set to 10, that is, the original data was randomly divided into 10 parts without repeated sampling. One of them is selected as the test set at a time, and the remaining nine are used as the training set to train the model. Repeat step 2 10 times to get a model after training on each training set. Test the model against the appropriate test set, calculate and save the model’s evaluation metrics. The average of the 10 groups of test results is calculated as an estimate of the accuracy of the model and as a performance index of the model under the current k-fold cross-validation. The intraoperative time series data were extracted by means of a long short-term memory (LSTM) deep learning model. The baseline data and the extracted intraoperative features were fused by the transfer learning and fed into each of the eight machine learning models for further training. The model with the best predictive performance was identified based on the calculated accuracy and area under the curve (AUC). Afterwards, the feature importance was calculated and ranked by DT model to screen out the main risk factors. All statistical analyses were performed using SPSS version 26. The statistical analysis plan was depicted in Figure 1.
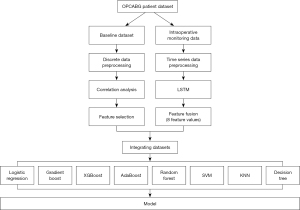
Results
Prediction of OPCABG-AKI by machine learning methods
Among 701 patients included in the study, 73 (10.4%) developed OPCABG-AKI. The patients inclusion flowchart was showed in Figure 2. Table 1 shows the performance of the models based on the baseline data only; the AUC of the GBDT model was the highest (AUC =0.739), followed by AdaBoost model (AUC =0.732), SVM model (AUC =0.731), LR model (AUC =0.717), XGBoost model (AUC =0.698), RF model (AUC =0.647), KNN model (AUC =0.578), and DT model (AUC =0.517). Table 2 shows the performance of these models when intraoperative data were added to the baseline data; the AUC of the GBDT model was again the highest (AUC =0.861), followed by RF model (AUC =0.780), XGBoost model (AUC =0.764), SVM model (AUC =0.730), AdaBoost model (AUC =0.726), LR model (AUC =0.700), KNN model (AUC =0.598) and DT model (AUC =0.550). These data demonstrate that the addition of intraoperative time series data resulted in a considerable increase in AUC; in case of the GBDT model, AUC increased by 0.122.
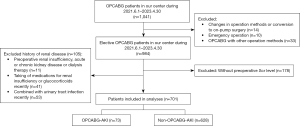
Table 1
Machine learning model | AUC | Accuracy rate |
---|---|---|
LR | 0.717 | 0.786 |
SVM | 0.731 | 0.793 |
DT | 0.517 | 0.871 |
RF | 0.647 | 0.936 |
KNN | 0.578 | 0.929 |
GBDT | 0.739 | 0.943 |
AdaBoost | 0.732 | 0.907 |
XGBoost | 0.698 | 0.936 |
AUC, area under the curve; LR, logistic regression; SVM, support vector machine; DT, decision tree; RF, random forest; KNN, k-nearest neighbor; GBDT, gradient-boosting decision tree; AdaBoost, adaptive boosting; XGBoost, eXtreme gradient boosting.
Table 2
Machine learning model | AUC | Accuracy rate |
---|---|---|
LR | 0.700 | 0.893 |
SVM | 0.730 | 0.900 |
DT | 0.550 | 0.836 |
RF | 0.780 | 0.921 |
KNN | 0.598 | 0.936 |
GBDT | 0.861 | 0.936 |
AdaBoost | 0.726 | 0.914 |
XGBoost | 0.764 | 0.936 |
AUC, area under the curve; LR, logistic regression; SVM, support vector machine; DT, decision tree; RF, random forest; KNN, k-nearest neighbor; GBDT, gradient-boosting decision tree; AdaBoost, adaptive boosting; XGBoost, eXtreme gradient boosting.
Interpretability of machine learning models in AKI forecasting
The top 20 features of the GBDT model for feature importance are shown in Figure 3. Use of insulin aspart is the most important feature of OPCABG-AKI, followed by use of acarbose, spironolactone, alfentanil, dezocine, levosimendan, clindamycin, history of myocardial infarction, sex, cerebral tissue oxygen saturation (SctO2; left), SctO2 (right), lactate, creatine kinase (CK), troponin, heart rate, family history of surgery, clopidogrel, age, systemic vascular resistance (SVR), and angiotensin receptor blockers (ARBs).
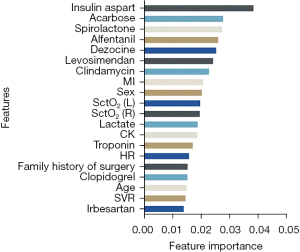
Discussion
CSA-AKI is a common complication after cardiac surgery with an incidence rate of up to 1 in 3 patients (18-22), which can lead to increased postoperative mortality, length of hospitalization time, and healthcare costs (23-25). In a meta-analysis including 86 randomized controlled trials (RCTs) and 25,855 patients, 5,082 (20%) patients developed CSA-AKI (11). In addition, the development of kidney disease is accompanied by a high incidence of gastrointestinal bleeding and surgical re-exploration for bleeding, respiratory tract infections, and sepsis (26,27). Therefore, the accurate prediction of CSA-AKI before surgery and early detection of high-risk patients can help clinicians to strengthen the physiological and hemodynamic monitoring and provide personalized fluid management at an early stage, which intends to optimize the systemic and renal perfusion in the high-risk patients and reduce the risk of CSA-AKI (28). A number of machine learning models have been developed to predict CSA-AKI. However, there are still no guidelines to recommend predictive models (29). Lee et al. (30) showed that XGBoost outperformed traditional LR or risk scores in prediction of CSA-AKI in their study. In another study, an integrated model (RF + XGBoost) was shown with the best performance in prediction of CSA-AKI. However, most models have been limited by heterogeneity of surgery types included, inconsistent definitions of AKI, and different completeness of perioperative data collection. Our present study, which specifically investigated AKI in the setting of OPCABG, determined that a GBDT model had the best predictive performance. Prediction could even be improved further by incorporating intraoperative time series data.
The occurrence of CSA-AKI depends on the patients’ preoperative condition and intraoperative physiological processes that are detrimental to the kidneys, including hypotension, low cardiac output syndrome, intraoperative catecholamine surge, decreased vasomotor responsiveness, and others (31). Both preoperative and intraoperative factors are helpful in prediction of CSA-AKI. Intraoperative features reflect intraoperative physiological changes in the heart. Tseng et al. (32) emphasized the value of intraoperative features in prediction of CSA-AKI. A machine learning algorithm was proposed in a single-center cohort study that reclassified approximately 40% of surgical patients; the patients were predicted as low-risk AKI with a preoperative model yet were classified as high-risk after incorporating intraoperative characteristics (33). However, processing intraoperative time series data as the minimum, maximum, average, and short- and long-term variability may result in the loss of the useful information (34). The advantage of our present study was the combination of machine learning and deep learning. Intraoperative features were extracted by a LS-LSTM deep learning model based on intraoperative time series data. Baseline data were subsequently fused with complex features of intraoperative continuous data by means of migration learning, and the integrated data were fed into the machine learning model to predict OPCABG-AKI. Compared with the use of preoperative data alone, the data fusion of baseline data and intraoperative continuous data with complex features improved the accuracy of the GBDT model in the prediction of OPCABG-AKI.
Our findings have some clinical implications. For patients with risk factors of postoperative AKI, although the preoperative factors are difficult to be addressed, the attending surgeons and anesthesiologists would regard these patients as high-risk individuals, focus on their renal function, actively give intervention measures to protect the kidney, closely monitor the hemodynamics, minimize the use of nephrotoxic drugs, and use goal-directed hemodynamic treatment, which would not only ensure effective renal perfusion but also avoid fluid overload. Preoperative variables reflected the baseline characteristics of patients, while intraoperative variables were more closely related to the management of patients by surgeons and anesthesiologists. The intraoperative variables were intraoperative real-time monitoring, and corresponding intervention measures could be taken in time to avoid the occurrence of postoperative AKI. Both of the preoperative baseline characteristics and the intraoperative real-time interventions have significant associations with postoperative AKI.
Previously identified risk factors for CSA-AKI, such as diabetes mellitus, preoperative renal function, age, type of surgery, duration of surgery, left ventricular ejection fraction, body mass index, and hypertension (14,22,35), were not included in the top 20 features for predicting OPCABG-AKI in this study. The main risk factors screened in this study included insulin aspart, acarbose, spironolactone, alfentanil, dezocine, levosimendan, clindamycin, history of myocardial infarction, gender, and others. A total of nine of the top 20 features in the importance of features were medicines used before and during surgery, among which the top five features were all medicines, which suggested that the related drugs can affect the renal function after cardiac surgery.
However, there are certain limitations to our study. Our study only included one single-center nature, and the number of AKI events is relatively small, and there was a lack of external validation and prospective validation. Different stages of AKI were not studied in this study, limited clinical significance. Future studies should include multi-center data, conduct prospective design, and follow up to verify the prediction effect of the model. It may be more beneficial to design observation endpoints for different degrees of AKI to guide clinical practice. Besides, the intra procedural data is lacking, like number of anastomoses, type of grafts. We will include more intra procedural data in our study in the future.
Conclusions
This study demonstrates the applicability of machine learning in predicting the development of OPCABG-AKI. Despite the previous models with AUC >0.7 can be helpful in predicting the risk of AKI following OPCABG, the prediction performance of the present GBDT model is much better. Moreover, the data fusion of preoperative and intraoperative characteristics improved the prediction performance of the GBDT model. The GBDT model may assist clinicians in the risk stratification and clinical decision-making for OPCABG-AKI in these patients who are undergoing OPCABG.
Acknowledgments
Funding: This work was supported by
Footnote
Reporting Checklist: The authors have completed the TRIPOD reporting checklist. Available at https://jtd.amegroups.com/article/view/10.21037/jtd-24-711/rc
Data Sharing Statement: Available at https://jtd.amegroups.com/article/view/10.21037/jtd-24-711/dss
Peer Review File: Available at https://jtd.amegroups.com/article/view/10.21037/jtd-24-711/prf
Conflicts of Interest: All authors have completed the ICMJE uniform disclosure form (available at https://jtd.amegroups.com/article/view/10.21037/jtd-24-711/coif). J.M.A. serves as an unpaid editorial board member of Journal of Thoracic Disease from October 2023 to September 2025. The other authors have no conflicts of interest to declare.
Ethical Statement: The authors are accountable for all aspects of the work in ensuring that questions related to the accuracy or integrity of any part of the work are appropriately investigated and resolved. The study was conducted in accordance with the Declaration of Helsinki (as revised in 2013). The study was approved by the Medical Ethics Committee of Chest Hospital, Tianjin University (No. 2021 KY-008-01). The requirement for informed consent was waived due to the retrospective nature of this study.
Open Access Statement: This is an Open Access article distributed in accordance with the Creative Commons Attribution-NonCommercial-NoDerivs 4.0 International License (CC BY-NC-ND 4.0), which permits the non-commercial replication and distribution of the article with the strict proviso that no changes or edits are made and the original work is properly cited (including links to both the formal publication through the relevant DOI and the license). See: https://creativecommons.org/licenses/by-nc-nd/4.0/.
References
- Rasmussen SB, Boyko Y, Ranucci M, et al. Cardiac surgery-Associated acute kidney injury - A narrative review. Perfusion 2023; Epub ahead of print. [Crossref]
- Yu Y, Li C, Zhu S, et al. Diagnosis, pathophysiology and preventive strategies for cardiac surgery-associated acute kidney injury: a narrative review. Eur J Med Res 2023;28:45. [Crossref] [PubMed]
- von Groote T, Sadjadi M, Zarbock A. Acute kidney injury after cardiac surgery. Curr Opin Anaesthesiol 2024;37:35-41. [Crossref] [PubMed]
- Milam AJ, Liang C, Mi J, et al. Derivation and Validation of Clinical Phenotypes of the Cardiopulmonary Bypass-Induced Inflammatory Response. Anesth Analg 2023;136:507-17. [Crossref] [PubMed]
- Fan X, Shao Z, Gao S, et al. Clinical characteristics and risk factors of cardiac surgery associated-acute kidney injury progressed to chronic kidney disease in adults: A retrospective, observational cohort study. Front Cardiovasc Med 2023;10:1108538. [Crossref] [PubMed]
- Gaudino M, Angelini GD, Antoniades C, et al. Off-Pump Coronary Artery Bypass Grafting: 30 Years of Debate. J Am Heart Assoc 2018;7:e009934. [Crossref] [PubMed]
- Takaki J, Morinaga J, Sadanaga T, et al. Renal Biomarkers in the Early Detection of Acute Kidney Injury After Off-Pump Coronary Artery Bypass Grafting. Circ J 2024;88:951-8. [Crossref] [PubMed]
- Chen Shoulei, Luo Cheng, Fang Chen, et al. Development and Validation of a Novel Nomogram for Predicting Perioperative Acute Kidney Injury Following Isolated Off-Pump Coronary Artery Bypass Grafting Surgery. Heart Surg Forum 2023;26:E832-41. [Crossref] [PubMed]
- Ko SH, Song JW, Shim JK, et al. Low Intraoperative Cerebral Oxygen Saturation Is Associated with Acute Kidney Injury after Off-Pump Coronary Artery Bypass. J Clin Med 2023;12:359. [Crossref] [PubMed]
- Wang KC, Moore AE. Cardiac Surgery-Associated Acute Kidney Injury in Adults: Clinical Outcomes, Prevention Strategies, and Future Therapies in the Postoperative Period. Nephrol Nurs J 2023;50:321-32.
- Hariri G, Collet L, Duarte L, et al. Prevention of cardiac surgery-associated acute kidney injury: a systematic review and meta-analysis of non-pharmacological interventions. Crit Care 2023;27:354. [Crossref] [PubMed]
- Thongprayoon C, Pattharanitima P, Kattah AG, et al. Explainable Preoperative Automated Machine Learning Prediction Model for Cardiac Surgery-Associated Acute Kidney Injury. J Clin Med 2022;11:6264. [Crossref] [PubMed]
- Nagy M, Onder AM, Rosen D, et al. Predicting pediatric cardiac surgery-associated acute kidney injury using machine learning. Pediatr Nephrol 2024;39:1263-70. [Crossref] [PubMed]
- Li Q, Lv H, Chen Y, et al. Development and Validation of a Machine Learning Predictive Model for Cardiac Surgery-Associated Acute Kidney Injury. J Clin Med 2023;12:1166.
- Fan R, Qin W, Zhang H, et al. Machine learning in the prediction of cardiac surgery associated acute kidney injury with early postoperative biomarkers. Front Surg 2023;10:1048431. [Crossref] [PubMed]
- Brown JK, Shaw AD, Mythen MG, et al. Adult Cardiac Surgery-Associated Acute Kidney Injury: Joint Consensus Report. J Cardiothorac Vasc Anesth 2023;37:1579-90. [Crossref] [PubMed]
- Khwaja A. KDIGO clinical practice guidelines for acute kidney injury. Nephron Clin Pract 2012;120:c179-84. [Crossref] [PubMed]
- Bell J, Sartipy U, Holzmann MJ, et al. The Association Between Acute Kidney Injury and Mortality After Coronary Artery Bypass Grafting Was Similar in Women and Men. J Cardiothorac Vasc Anesth 2022;36:962-70. [Crossref] [PubMed]
- Chan MJ, Lee CC, Chen SW, et al. Effect of different surgical type of coronary artery bypass grafting on kidney injury: A propensity score analysis. Medicine (Baltimore) 2017;96:e8395. [Crossref] [PubMed]
- Yue Z, Yan-Meng G, Ji-Zhuang L. Prediction model for acute kidney injury after coronary artery bypass grafting: a retrospective study. Int Urol Nephrol 2019;51:1605-11. [Crossref] [PubMed]
- Chen JJ, Chang CH, Wu VC, et al. Long-Term Outcomes of Acute Kidney Injury After Different Types of Cardiac Surgeries: A Population-Based Study. J Am Heart Assoc 2021;10:e019718. [Crossref] [PubMed]
- Wang Y, Bellomo R. Cardiac surgery-associated acute kidney injury: risk factors, pathophysiology and treatment. Nat Rev Nephrol 2017;13:697-711. [Crossref] [PubMed]
- Biteker M, Dayan A, Tekkeşin Aİ, et al. Incidence, risk factors, and outcomes of perioperative acute kidney injury in noncardiac and nonvascular surgery. Am J Surg 2014;207:53-9. [Crossref] [PubMed]
- Ortega-Loubon C, Fernández-Molina M, Carrascal-Hinojal Y, et al. Cardiac surgery-associated acute kidney injury. Ann Card Anaesth 2016;19:687-98. [Crossref] [PubMed]
- Mao H, Katz N, Ariyanon W, et al. Cardiac surgery-associated acute kidney injury. Cardiorenal Med 2013;3:178-99. [Crossref] [PubMed]
- Ejmalian A, Aghaei A, Nabavi S, et al. Prediction of Acute Kidney Injury After Cardiac Surgery Using Interpretable Machine Learning. Anesth Pain Med 2022;12:e127140. [Crossref] [PubMed]
- Sear JW. Kidney dysfunction in the postoperative period. Br J Anaesth 2005;95:20-32. [Crossref] [PubMed]
- Sun LY, Chung AM, Farkouh ME, et al. Defining an Intraoperative Hypotension Threshold in Association with Stroke in Cardiac Surgery. Anesthesiology 2018;129:440-7. [Crossref] [PubMed]
- Vives M, Hernandez A, Parramon F, et al. Acute kidney injury after cardiac surgery: prevalence, impact and management challenges. Int J Nephrol Renovasc Dis 2019;12:153-66. [Crossref] [PubMed]
- Lee HC, Yoon HK, Nam K, et al. Derivation and Validation of Machine Learning Approaches to Predict Acute Kidney Injury after Cardiac Surgery. J Clin Med 2018;7:322. [Crossref] [PubMed]
- Petrosyan Y, Mesana TG, Sun LY. Prediction of acute kidney injury risk after cardiac surgery: using a hybrid machine learning algorithm. BMC Med Inform Decis Mak 2022;22:137. [Crossref] [PubMed]
- Tseng PY, Chen YT, Wang CH, et al. Prediction of the development of acute kidney injury following cardiac surgery by machine learning. Crit Care 2020;24:478. [Crossref] [PubMed]
- Adhikari L, Ozrazgat-Baslanti T, Ruppert M, et al. Improved predictive models for acute kidney injury with IDEA: Intraoperative Data Embedded Analytics. PLoS One 2019;14:e0214904. [Crossref] [PubMed]
- Saria S, Rajani AK, Gould J, et al. Integration of early physiological responses predicts later illness severity in preterm infants. Sci Transl Med 2010;2:48ra65. [Crossref] [PubMed]
- Huen SC, Parikh CR. Predicting acute kidney injury after cardiac surgery: a systematic review. Ann Thorac Surg 2012;93:337-47. [Crossref] [PubMed]
(English Language Editor: J. Jones)