A prediction model based on computed tomography characteristics for identifying malignant from benign sub-centimeter solid pulmonary nodules
Highlight box
Key findings
• We established a prediction model based on computed tomography (CT) features and validated its performance using data from two centers, which may help in the early diagnosis of lung cancer and improve patient prognosis.
What is known and what is new?
• Tumor-lung interface, spiculation, air bronchogram and invisible at the mediastinal window were independent predictors for distinguishing malignant from benign sub-centimeter solid pulmonary nodules (SSPNs).
• Our model has a good performance in distinguishing malignant SSPNs from benign ones.
What is the implication, and what should change now?
• SSPNs differ from traditional larger nodules both clinically and radiologically.
• The prediction model based on CT features can help distinguish between benign and malignant SSPNs.
Introduction
Lung cancer is responsible for the leading cause of cancer-related mortality. According to estimates from the Global Cancer Statistics 2020, lung cancer accounted for an estimated 1.8 million deaths in 185 countries (1). Most lung cancer cases are already in the advanced-stage when detected or diagnosed, which leads to poor prognosis and short survival time for patients. The survival rate of lung cancer patients decreases with increasing staging. The 5-year survival rate is 68–92% for stage I and 0–60% for stage II–IV (2). The wide utilization of chest computed tomography (CT) has significantly increased the detection rate of pulmonary nodules and reduced lung cancer mortality by 20% (3). The results of low-dose CT for lung cancer screening in Shanghai, China and South Korea showed that 68.3–81.09% of all detected lung cancers were in stage I (4,5). Therefore, earlier detection and diagnosis are key to improving the prognosis of lung cancer patients and reducing their mortality.
Lung cancer could appear as solid, part-solid, or ground-glass nodules or mass on CT imaging. Compared to part-solid and ground-glass nodules, lung cancer appearing as solid nodules has a higher risk of metastasis and a poorer prognosis (6). Therefore, earlier diagnosis of lung cancer presenting as solid nodules is more crucial. However, the diagnosis of sub-centimeter solid pulmonary nodules (SSPNs) is very difficult in the clinical practice. In most cases, SSPNs are benign, including intrapulmonary lymph nodes, intrapulmonary charcoal deposition, and granuloma; however, 4.5% are malignant (7). In addition, some previous studies have shown that about 10% SSPNs have a risk of lymph node metastasis or recurrence (8,9). However, due to the small diameter of SSPNs, the diagnostic value of positron emission tomography/computed tomography (PET-CT), bronchoscopy, and puncture biopsy is limited (10). Thus, it remains challenging to distinguish malignant from benign SSPNs in clinical practice.
CT examination plays an important role in the diagnosis and follow-up decision-making for these SSPNs. Many studies have investigated the value of the clinical and radiological features in distinguishing between malignant and benign pulmonary nodules. Several predictive models are available to distinguish benign and malignant nodules, such as the Mayo model (11) and the VA model (12). However, these models involved both solid and ground-glass nodules, and rarely only focused on solid nodules, especially SSPNs. This study focused on the SSPNs and collected their clinical and imaging characteristics to construct and validate a risk prediction model, including internal and external dataset from two centers. Therefore, the purpose of this study is to investigate the clinical and imaging characteristics of SSPNs and establish and validate a prediction model based on CT characteristics to assist in their early diagnosis. We present this article in accordance with the TRIPOD reporting checklist (available at https://jtd.amegroups.com/article/view/10.21037/jtd-23-1943/rc).
Methods
Patient selection
This retrospective study was conducted in accordance with the Declaration of Helsinki (as revised in 2013) and approved by the institutional ethics board of National Cancer Center/National Clinical Research Center for Cancer/Cancer Hospital, Chinese Academy of Medical Sciences and Peking Union Medical College (No. NCC2021C-283) and The Fourth Hospital of Hebei Medical University was informed and agreed with the study. Informed consent was waived as this was a retrospective study and subjects were assured of privacy and identifying information. We retrospectively collected SSPNs between January 2012 and July 2023 from National Cancer Center/National Clinical Research Center for Cancer/Cancer Hospital, Chinese Academy of Medical Sciences and Peking Union Medical College (Center 1) with the following criteria: (I) solid pulmonary nodules with mean diameter ≤10 mm; (II) malignant or benign SSPNs confirmed by surgical pathology or follow-up: all malignant and a majority of benign nodules were confirmed by surgical pathology; some SSPNs that remained stable during follow-up of ≥2 years are considered benign; (III) chest CT images with a thin slice thickness (≤1.25 mm). Exclusion criteria were as follows: (I) participants with incomplete clinical data; (II) patients with a history of malignancy in less than 5 years; (III) the pathological diagnoses were metastatic lesions. Finally, we enrolled 261 consecutive patients from Center 1, and randomly divided them at a ratio of 7:3 into two sets: the training set (n=183) and internal validation set (n=78). All of the malignant lesions were pathologically proved, including 148 (148/161, 91.9%) adenocarcinoma, five squamous cell carcinoma, one adenosquamous carcinoma, and seven neuroendocrine tumors (Table 1). Besides, we retrospectively collected 69 patients from The Fourth Hospital of Hebei Medical University (Center 2) between January 2022 and December 2022 as the external validation dataset.
Table 1
Pathological subtypes | N |
---|---|
Benign | 100 (38.3%) |
Granuloma | 20 |
Fibroplasia | 25 |
Tuberculosis | 1 |
Organising pneumonia | 1 |
Bronchial adenoma | 5 |
Sclerosing pneumocytoma | 2 |
Pulmonary hamartoma | 25 |
Intrapulmonary lymph nodes | 11 |
Hyperplasia of lung tissue | 4 |
Follow-up | 6 |
Malignant | 161 (61.7%) |
Adenocarcinomas | 148 |
Microinvasive adenocarcinoma | 25 |
Invasive non-mucinous adenocarcinoma | 107 |
Invasive mucinous adenocarcinoma | 16 |
Squamous cell carcinomas | 5 |
Adenosquamous carcinoma | 1 |
Neuroendocrine tumors | 7 |
Carcinoid/neuroendocrine tumor | 2 |
Small cell lung carcinoma | 3 |
Large cell neuroendocrine carcinoma | 2 |
Chest CT scan technique
All CT scans were performed using 64-detector row scanners (Optima CT 660, LightSpeed VCT or Discovery CT 750 HD, General Electric Medical Systems; TOSHIBA Aquilion, TOSHIBA Medical Systems, Tokyo, Japan). To avoid the effects of respiratory artifacts, images were acquired with the patient in a fully inspiratory state. Parameters for CT scans included: a tube voltage of 120 kVp; automatic mA configurations (tube current between 200–350 mA, noise index at 13; pitch values of 0.992 or 0.984; a rotation duration of 0.5 s; and a thickness of 5 mm). For enhanced CT scans, iopromide was used as a contrast agent, injected intravenously at 300 mg/mL iodine levels, varying between 80 and 90 mL, with a 2.5 mL/s flow rate, and the images were taken 35 s after the intravenous injection. The reconstruction thicknesses were either 1.25 or 1.0 mm, spaced 0.8 mm apart by a conventional reconstruction algorithm. The images were observed in both the lung window [window width (WW) =1,600 HU; window level (WL) =−600 HU] and the mediastinal window (WW =360 HU; WL =60 HU).
Radiologic features evaluation
The clinical and radiologic features of all enrolled SSPNs were analyzed and processed by Radiology Information System/Picture Archiving and Communication Systems (RIS/PACS). The radiologic features were reviewed by two radiologists (J.N.L. and S.L.C., with 6 and 3 years of experience in diagnosing thoracic tumors, respectively). Disagreements were resolved through consultation with two senior radiologists (L.L.Q. and J.W.W., with 8 and 25 years of experience in diagnosing thoracic tumors, respectively). Radiologic features including nodule size (mean diameter), location, the tumor-lung interface, lobulation, spiculation, air bronchogram, vacuole, pleural traction, and vascular convergence were observed at the lung window; calcification and nodules invisibility were observed at the mediastinal windows.
The tumor-lung interface was defined as the border between the lesion and normal lung parenchyma, which was classified as clear and smooth, clear and rough, and unclear/halo sign. A clear and smooth tumor-lung interface was clear and flat, with or without lobation (Figure 1A). Unlike the smooth tumor-lung interface, clear and rough indicated clear but irregular or spiculated interface (Figure 1B). Unclear/halo sign demonstrated glass opacity around the lesion (Figure 1C). Lobulation is defined as part of the lesion having a wavy or scalloped surface (13). According to the ratio of the chord arc distance to chord length, the lobation sign was divided into three categories: shallow (a ratio of ≤0.2), medium (a ratio of 0.2 to 0.4), and deep (a ratio of ≥0.4) lobation (Figure 1D-1F). Spiculation was generally divided into two categories: short and long spiculation; the former fine and short, length <1 cm, width 1 mm; the latter thick and long, length 1–3 cm, width 1–2 mm (Figure 1B,1G). A vacuole is defined as a small area of round or oval air attenuation within the nodule (Figure 1B). Air bronchogram is the presence of air-filled bronchi in the nodule (Figure 1H). When a nodule is visible at the mediastinal window, it indicates that the nodule can be observed both at the lung window and the mediastinal window (Figure 1I,1J). A SSPN is invisible at the mediastinal window, it indicates that the nodule can be observed at the lung window, but not at the mediastinal window (Figure 1K,1L).
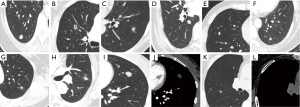
Pathological diagnosis
The pathological diagnosis and categorization of SSPNs were confirmed based on the new World Health Organization (WHO) pulmonary tumor classification, 2021 edition (14). Surgical samples served as the basis for the ultimate pathological assessments. All histological preparations and analyses were conducted by two experienced pathologists, each having over two decades of expertise in pathological diagnoses. Disagreements were resolved and settled either through collective agreement or following discussions with a third pathologist.
Statistical analysis
All statistical analyses were performed with the SPSS software (version 26.0). Continuous variables were analyzed with the independent samples t-test and the Wilcoxon rank-sum test. The categorical variables were compared with the χ2 test and Fisher’s exact test. Measurement data were presented as the mean ± standard deviation (SD) or median (25th, 75th). Count data were expressed as percentages. The bilateral P value <0.05 was considered statistically significant. Variables with P<0.05 in the univariate analysis were included in the multivariable logistic regression analysis to determine the independent risk factors. A receiver operating characteristic (ROC) curve was used to evaluate the performance of the model. Moreover, the model was validated by the internal set and external set, respectively.
Results
Clinical and radiological characteristics of SSPNs in the training set and independent risk factors for malignant SSPNs
The clinical and radiological characteristics of enrolled SSPNs in the training set are demonstrated in Table 2. The training cohort consisted of 183 patients: 73 males (57.6±11.6 years) and 110 females (54.3±9.1 years). In this study, seven clinical and eleven CT features were analyzed. The univariate analysis demonstrated significant differences in the tumor-lung interface (P<0.001), lobulation (P=0.003), spiculation (P<0.001), air bronchogram (P=0.001), vacuole (P=0.006), pleural traction (P=0.002), and invisible at the mediastinal window (P=0.04) between benign and malignant groups. Multivariable logistic regression analysis showed that characteristics of the tumor-lung interface (P=0.002), spiculation (P=0.04), air bronchogram (P=0.047), and invisible at the mediastinal window (P=0.003) were independent risk factors of malignant SSPNs. The AUCs of the independent predictors for differentiating benign and malignant SSPNs ranged from 0.546 to 0.690 (Figure 2).
Table 2
Characteristics | Benign (n=70) | Malignant (n=113) | P value | Multivariate logistic regression analysis | |
---|---|---|---|---|---|
OR | P value | ||||
Gender, n (%) | 0.77 | – | – | ||
Male | 27 (38.6) | 46 (40.7) | – | – | |
Female | 43 (61.4) | 67 (59.3) | – | – | |
Age (years), mean ± SD | 54.5±10.2 | 56.3±10.3 | 0.25 | – | – |
Symptom, n (%) | 20 (28.6) | 21 (18.6) | 0.12 | – | – |
Smoking history, n (%) | 13 (18.6) | 27 (23.9) | 0.40 | – | – |
Family cancer history, n (%) | 26 (37.1) | 40 (35.4) | 0.81 | – | – |
Abnormal CEA level, n (%) | 1 (1.4) | 5 (4.4) | 0.41 | – | – |
Previous history of extra-thoracic cancer 5 years ago, n (%) | 0 (0.0) | 2 (1.8) | 0.53 | – | – |
Size (mm), median (25th, 75th) | 8.0 (7.0–9.5) | 8.5 (7.3–10.0) | 0.12 | – | – |
Location, n (%) | 0.86 | – | – | ||
LUL | 17 (24.3) | 30 (26.5) | – | – | |
RUL | 17 (24.3) | 23 (20.4) | – | – | |
RML | 8 (11.4) | 11 (9.7) | – | – | |
LLL | 11 (15.7) | 24 (21.2) | – | – | |
RLL | 17 (24.3) | 25 (22.1) | – | – | |
The tumor-lung interface, n (%) | <0.001* | – | 0.002* | ||
Clear and smooth | 34 (48.6) | 4 (3.5) | Reference | ||
Clear and rough | 25 (35.7) | 91 (80.5) | 40.853 | 0.001* | |
Unclear/halo sign | 11 (15.7) | 18 (15.9) | 17.206 | 0.01* | |
Lobulation, n (%) | 0.003* | – | 0.57 | ||
None | 24 (34.3) | 23 (20.4) | Reference | ||
Shallow | 7 (10.0) | 6 (5.3) | 0.903 | 0.92 | |
Medium | 6 (8.6) | 2 (1.8) | 0.225 | 0.22 | |
Deep | 33 (47.1) | 82 (72.6) | 1.148 | 0.79 | |
Spiculation, n (%) | <0.001* | – | 0.04* | ||
None | 62 (88.6) | 66 (58.4) | Reference | ||
Short | 7 (10.0) | 47 (41.6) | 3.638 | 0.01* | |
Long | 1 (1.4) | 0 (0.0) | 0.000 | >0.99 | |
Air bronchogram, n (%) | 2 (2.9) | 24 (21.2) | 0.001* | 5.005 | 0.047* |
Vacuole, n (%) | 6 (8.6) | 28(24.8) | 0.006* | 3.094 | 0.051 |
Pleural traction, n (%) | 20 (28.6) | 58 (51.3) | 0.002* | 1.505 | 0.35 |
Vascular convergence, n (%) | 1 (1.4) | 11 (9.7) | 0.06 | – | – |
Calcification, n (%) | 2 (2.9) | 0 (0.0) | 0.15 | – | – |
Invisible at the mediastinal window, n (%) | 1 (1.4) | 12 (10.6) | 0.04* | 88.599 | 0.003* |
The differences were assessed by the independent samples t-test and Wilcoxon Rank Sum test or Pearson χ2 test and Fisher’s exact test, as appropriate. *, P<0.05. SSPNs, sub-centimeter solid pulmonary nodules; OR, odds ratio; SD, standard deviation; CEA, carcinoembryonic antigen; LUL, left upper lobe; RUL, right upper lobe; RML, right middle lobe; LLL, left lower lobe; RLL, right lower lobe.
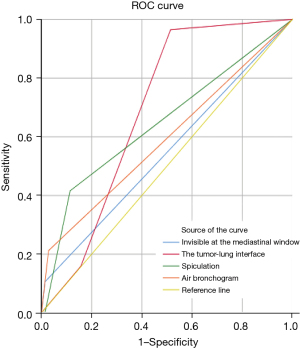
Model construction and its internal and external validation
The clinical and radiological characteristics of SSPNs in the internal and external validation sets are demonstrated in the Tables 3,4. The prediction model for distinguishing benign and malignant SSPNs was established based on the results of multivariable logistic regression analysis.
Table 3
Characteristics | Benign (n=30) | Malignant (n=48) | P value |
---|---|---|---|
Gender, n (%) | 0.57 | ||
Male | 13 (43.3) | 24 (50.0) | |
Female | 17 (56.7) | 24 (50.0) | |
Age (years), mean ± SD | 55.7±11.6 | 57.5±8.7 | 0.44 |
Symptom, n (%) | 1 (3.3) | 13 (27.1) | 0.008* |
Smoking history, n (%) | 7 (23.3) | 13 (27.1) | 0.71 |
Family cancer history, n (%) | 10 (33.3) | 15 (31.2) | 0.85 |
Abnormal CEA level, n (%) | 1 (3.3) | 4 (8.3) | 0.64 |
Previous history of extra-thoracic cancer 5 years ago, n (%) | 0 (0.0) | 2 (4.2) | 0.52 |
Size (mm), median (25th, 75th) | 8.3 (6.4–9.6) | 9.0 (7.6–10.0) | 0.045* |
Location, n (%) | 0.45 | ||
LUL | 3 (10.0) | 11 (22.9) | |
RUL | 8 (26.7) | 8 (16.7) | |
RML | 3 (10.0) | 2 (4.2) | |
LLL | 8 (26.7) | 15 (31.3) | |
RLL | 8 (26.7) | 12 (25.0) | |
The tumor-lung interface, n (%) | <0.001* | ||
Clear and smooth | 14 (46.7) | 2 (4.2) | |
Clear and rough | 12 (40.0) | 37 (77.1) | |
Unclear/halo sign | 4 (13.3) | 9 (18.8) | |
Lobulation, n (%) | <0.001* | ||
None | 10 (33.3) | 2 (4.2) | |
Shallow | 9 (30.0) | 3 (6.3) | |
Medium | 1 (3.3) | 4 (8.3) | |
Deep | 10 (33.3) | 39 (81.3) | |
Spiculation, n (%) | 0.02* | ||
None | 23 (76.7) | 28 (58.3) | |
Short | 5 (16.7) | 20 (41.7) | |
Long | 2 (6.7) | 0 (0.0) | |
Air bronchogram, n (%) | 2 (6.7) | 17 (35.4) | 0.004* |
Vacuole, n (%) | 2 (6.7) | 12 (25.0) | 0.04* |
Pleural traction, n (%) | 8 (26.7) | 28 (58.3) | 0.006* |
Vascular convergence, n (%) | 0 (0.0) | 3 (6.3) | 0.28 |
Calcification, n (%) | 1 (3.3) | 1 (2.1) | >0.99 |
Invisible at the mediastinal window, n (%) | 2 (6.7) | 3 (6.3) | >0.99 |
The differences were assessed by the independent samples t-test and Wilcoxon Rank Sum test or Pearson χ2 test and Fisher’s exact test, as appropriate. *, P<0.05. SSPNs, sub-centimeter solid pulmonary nodules; SD, standard deviation; CEA, carcinoembryonic antigen; LUL, left upper lobe; RUL, right upper lobe; RML, right middle lobe; LLL, left lower lobe; RLL, right lower lobe.
Table 4
Variables | Benign (n=41) | Malignant (n=28) |
---|---|---|
The tumor-lung interface, n (%) | ||
Clear and smooth | 30 (73.2) | 2 (7.1) |
Clear and rough | 9 (22.0) | 22 (78.6) |
Unclear/halo sign | 2 (4.9) | 4 (14.3) |
Spiculation, n (%) | ||
None | 35 (85.4) | 11 (39.3) |
Short | 6 (14.6) | 17 (60.7) |
Long | 0 (0.0) | 0 (0.0) |
Air bronchogram, n (%) | 0 (0.0) | 3 (10.7) |
Invisible at the mediastinal window, n (%) | 4 (9.8) | 1 (3.6) |
SSPNs, sub-centimeter solid pulmonary nodules.
ROC analysis revealed that the model’s area under the curve (AUC) value was 0.875 [95% confidence interval (CI): 0.818, 0.933] for the training set (Figure 3). The AUCs in the internal and external validation set were 0.781 (95% CI: 0.664, 0.897) and 0.873 (95% CI: 0.791, 0.955), respectively; the sensitivity and specificity were 66.7% and 83.3% for the internal validation data, and 100.0% and 61.0% for the external validation data, respectively (Figures 4,5).
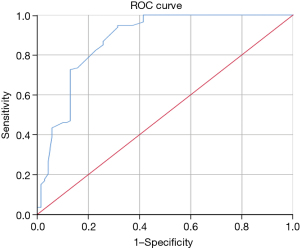
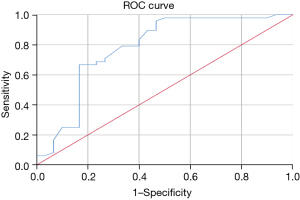
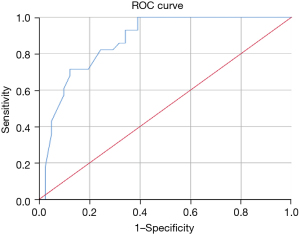
Discussion
The differentiation between benign and malignant SSPNs remains difficult in clinical practice. Earlier diagnosis could improve patient survival and prognosis. In this study, we explored on CT and clinical features of SSPNs and established and validated a prediction model to assist in distinguishing malignant and benign SSPNs. Multivariable logistic regression analysis showed that the tumor-lung interface, spiculation, air bronchogram, and invisible at the mediastinal window were independent risk factors of malignant SSPNs. The AUCs in the internal and external validation set were (0.781; 95% CI: 0.664, 0.897) and (0.873; 95% CI: 0.791, 0.955), respectively; the sensitivity and specificity were 66.7% and 83.3% for the internal validation data, and 100.0% and 61.0% for the external validation data, respectively.
In this study, a clear and rough tumor-lung interface conferred a 40.9-fold increased risk of malignancy than a clear and smooth nodule, which is much higher than a previous study (15). The previous study showed that poorly defined border increased the risk of lung cancer by 6.6-fold. Perhaps it is because this study only included SSPNs, while the previous study included both ground-glass nodules and solid nodules. Halo sign presents as an area of ground-glass attenuation surrounding a solid pulmonary nodule and it was usually considered pulmonary hemorrhage (16). In this study, the incidence of halo sign was slightly higher in malignant SSPNs, which may be caused by tumor invasion of the surrounding tissue or a lepidic pattern of the tumor margins. Therefore, a small nodule with a halo sign should be alert to the possibility of malignancy.
It should be noted that SSPNs with clear and smooth tumor-lung interfaces are not enough to be considered as benign, and they may be highly malignant. Proximately 20% to 30% of pulmonary nodules with well-defined, and smooth borders are malignant (17). In a recent study, Hu et al. (18) reported two cases of SSPNs manifesting smooth edges were small cell lung cancers (SCLCs), which diverge from those of larger SCLCs. In this study, six malignant SSPNs with clear and smooth borders tended to be ball or oval. One was SCLC, and the remaining five were adenocarcinomas. Thus, a sub-centimeter nodule with a clear and well-defined border is not definitely benign, and it is also necessary to observe other malignant imaging features and the growth rate for a comprehensive assessment.
As reported, spiculation signs or air bronchogram effectively distinguish benign and malignant nodules (19,20). In this study, SSPNs with short spiculation signs or air bronchogram are prone to be malignant. Generally, solid nodules are visible at the mediastinal window, while, invisible at the mediastinal window is an independent predictor for malignant SSPNs in this study. It could be a result of a lepidic growth pattern or the gradual accumulation of cells during the growth. Moreover, compared with previous studies, located in the upper lobe (15,21-24), larger size (19), and lobulation (25) were not strongly correlated with malignancy. Generally, older age and abnormal carcinoembryonic antigen (CEA) are related to malignant nodules (25,26), however, in this study, neither age nor CEA level is independent predictor. The possible reasons for the results are as follows: compared to traditional larger lung cancers, the small size of SSPNs, makes these features less notable; the different characteristics of the participants enrolled. These results suggest that small nodules differ from traditional larger nodules both clinically and radiologically.
There are overlaps between benign and malignant SSPNs (27,28). The presence of lobulation or spiculation in granuloma nodules usually confuses our judgment, which makes the diagnoses more challenging and intricate. Because of excavating numerous quantitative imaging features with high throughput of radiomics and automatically learning features at multiple levels of abstraction of deep learning (29,30), some scholars have attempted to apply them for establishing prediction models in the recent studies (23,28,31,32). Their prediction models have achieved relatively good results in predicting malignancy or distinguishing between benign and malignant lesions. Nonetheless, due to poor reproducibility in radiomics, makes them difficult to be widely applied in clinical practice (33). Hence, we believe traditional imaging is still critical.
Due to the low probability of malignancy but high degree of malignancy of solid nodules, the clinical method for SSPNs mainly relies on careful attention on CT follow-up observation for further treatment. Various guidelines recommend 12-month follow-up intervals for solid nodules <6 mm, 6–12 months follow-up intervals for solid nodules 6–8 mm, and 3 months for solid nodules ≥8 mm of patients with high-risk factors (34-36). A retrospective study discovered solid nodules maintaining stability of within a 3-month follow-up interval is not strong evidence for the diagnosis of benign nodules and requires continuous attention (37). Therefore, in clinical practice, for suspected SSPNs, the observation window should be appropriately extended, and early diagnosis and surgical treatment should be carried out.
There were several limitations in this study. Firstly, the study was retrospective with relatively small sample size. Secondly, this study did not include genetic mutations information. Finally, only one external validation dataset was available in this study and more validations should be conducted.
Conclusions
In summary, tumor-lung interface, spiculation, air bronchogram and invisible at the mediastinal window are independent predictors for distinguishing malignant from benign SSPNs. This study established a prediction model based on CT features and validated its performance using data from two centers, which may help in the early diagnosis of lung cancer and improve patient prognosis.
Acknowledgments
Funding: This work was supported by
Footnote
Reporting Checklist: The authors have completed the TRIPOD reporting checklist. Available at https://jtd.amegroups.com/article/view/10.21037/jtd-23-1943/rc
Data Sharing Statement: Available at https://jtd.amegroups.com/article/view/10.21037/jtd-23-1943/dss
Peer Review File: Available at https://jtd.amegroups.com/article/view/10.21037/jtd-23-1943/prf
Conflicts of Interest: All authors have completed the ICMJE uniform disclosure form (available at https://jtd.amegroups.com/article/view/10.21037/jtd-23-1943/coif). The authors have no conflicts of interest to declare.
Ethical Statement: The authors are accountable for all aspects of the work in ensuring that questions related to the accuracy or integrity of any part of the work are appropriately investigated and resolved. This retrospective study was conducted in accordance with the Declaration of Helsinki (as revised in 2013) and approved by the institutional ethics board of National Cancer Center/National Clinical Research Center for Cancer/Cancer Hospital, Chinese Academy of Medical Sciences and Peking Union Medical College (NCC2021C-283) and The Fourth Hospital of Hebei Medical University was informed and agreed with the study. Informed consent was waived as this was a retrospective study and subjects were assured of privacy and identifying information.
Open Access Statement: This is an Open Access article distributed in accordance with the Creative Commons Attribution-NonCommercial-NoDerivs 4.0 International License (CC BY-NC-ND 4.0), which permits the non-commercial replication and distribution of the article with the strict proviso that no changes or edits are made and the original work is properly cited (including links to both the formal publication through the relevant DOI and the license). See: https://creativecommons.org/licenses/by-nc-nd/4.0/.
References
- Sung H, Ferlay J, Siegel RL, et al. Global Cancer Statistics 2020: GLOBOCAN Estimates of Incidence and Mortality Worldwide for 36 Cancers in 185 Countries. CA Cancer J Clin 2021;71:209-49. [Crossref] [PubMed]
- Detterbeck FC, Boffa DJ, Kim AW, et al. The Eighth Edition Lung Cancer Stage Classification. Chest 2017;151:193-203.
- National Lung Screening Trial Research Team. Reduced lung-cancer mortality with low-dose computed tomographic screening. N Engl J Med 2011;365:395-409. [Crossref] [PubMed]
- Fan L, Wang Y, Zhou Y, et al. Lung Cancer Screening with Low-Dose CT: Baseline Screening Results in Shanghai. Acad Radiol 2019;26:1283-91. [Crossref] [PubMed]
- Kim YW, Kang HR, Kwon BS, et al. Low-dose chest computed tomographic screening and invasive diagnosis of pulmonary nodules for lung cancer in never-smokers. Eur Respir J 2020;56:2000177. [Crossref] [PubMed]
- Hattori A, Suzuki K, Matsunaga T, et al. What is the appropriate operative strategy for radiologically solid tumours in subcentimetre lung cancer patients?†. Eur J Cardiothorac Surg 2015;47:244-9. [Crossref] [PubMed]
- Henschke CI, Yankelevitz DF, Mirtcheva R, et al. CT screening for lung cancer: frequency and significance of part-solid and nonsolid nodules. AJR Am J Roentgenol 2002;178:1053-7. [Crossref] [PubMed]
- Sakurai H, Nakagawa K, Watanabe S, et al. Clinicopathologic features of resected subcentimeter lung cancer. Ann Thorac Surg 2015;99:1731-8. [Crossref] [PubMed]
- Sun K, You A, Wang B, et al. Clinical T1aN0M0 lung cancer: differences in clinicopathological patterns and oncological outcomes based on the findings on high-resolution computed tomography. Eur Radiol 2021;31:7353-62. [Crossref] [PubMed]
- Mazzone PJ, Lam L. Evaluating the Patient With a Pulmonary Nodule: A Review. JAMA 2022;327:264-73. [Crossref] [PubMed]
- Swensen SJ, Silverstein MD, Ilstrup DM, et al. The probability of malignancy in solitary pulmonary nodules. Application to small radiologically indeterminate nodules. Arch Intern Med 1997;157:849-55. [Crossref] [PubMed]
- Gould MK, Ananth L, Barnett PG, et al. A clinical model to estimate the pretest probability of lung cancer in patients with solitary pulmonary nodules. Chest 2007;131:383-8. [Crossref] [PubMed]
- Chu ZG, Li WJ, Fu BJ, et al. CT Characteristics for Predicting Invasiveness in Pulmonary Pure Ground-Glass Nodules. AJR Am J Roentgenol 2020;215:351-8. [Crossref] [PubMed]
- WHO Classification of Tumours Editorial Board. Thoracic Tumours. WHO Classification of Tumours. 5th edition. 2021. Available online: https://tumourclassification.iarc.who.int/
- Liu Y, Wang H, Li Q, et al. Radiologic Features of Small Pulmonary Nodules and Lung Cancer Risk in the National Lung Screening Trial: A Nested Case-Control Study. Radiology 2018;286:298-306. [Crossref] [PubMed]
- Parrón M, Torres I, Pardo M, et al. The halo sign in computed tomography images: differential diagnosis and correlation with pathology findings. Arch Bronconeumol 2008;44:386-92. [Crossref] [PubMed]
- Wahidi MM, Govert JA, Goudar RK, et al. Evidence for the treatment of patients with pulmonary nodules: when is it lung cancer?: ACCP evidence-based clinical practice guidelines (2nd edition). Chest 2007;132:94S-107S.
- Hu B, Ren W, Feng Z, et al. Correlation between CT imaging characteristics and pathological diagnosis for subcentimeter pulmonary nodules. Thorac Cancer 2022;13:1067-75. [Crossref] [PubMed]
- She Y, Zhao L, Dai C, et al. Development and validation of a nomogram to estimate the pretest probability of cancer in Chinese patients with solid solitary pulmonary nodules: A multi-institutional study. J Surg Oncol 2017;116:756-62. [Crossref] [PubMed]
- Jacob M, Romano J, Ara Jo D, et al. Predicting lung nodules malignancy. Pulmonology 2022;28:454-60. [Crossref] [PubMed]
- Chen X, Feng B, Chen Y, et al. A CT-based radiomics nomogram for prediction of lung adenocarcinomas and granulomatous lesions in patient with solitary sub-centimeter solid nodules. Cancer Imaging 2020;20:45. [Crossref] [PubMed]
- Kinsey CM, Estepar RS, Zhao Y, et al. Invasive adenocarcinoma of the lung is associated with the upper lung regions. Lung Cancer 2014;84:145-50. [Crossref] [PubMed]
- Wang S, Zhou L, Li X, et al. A Novel Deep Learning Model to Distinguish Malignant Versus Benign Solid Lung Nodules. Med Sci Monit 2022;28:e936830. [Crossref] [PubMed]
- Chu ZG, Zhang Y, Li WJ, et al. Primary solid lung cancerous nodules with different sizes: computed tomography features and their variations. BMC Cancer 2019;19:1060. [Crossref] [PubMed]
- Liu M, Zhou Z, Liu F, et al. CT and CEA-based machine learning model for predicting malignant pulmonary nodules. Cancer Sci 2022;113:4363-73. [Crossref] [PubMed]
- Zhang R, Tian P, Chen B, et al. Predicting Lung Cancer Risk of Incidental Solid and Subsolid Pulmonary Nodules in Different Sizes. Cancer Manag Res 2020;12:8057-66. [Crossref] [PubMed]
- He C, Liu J, Li Y, et al. Quantitative parameters of enhanced dual-energy computed tomography for differentiating lung cancers from benign lesions in solid pulmonary nodules. Front Oncol 2022;12:1027985. [Crossref] [PubMed]
- Feng B, Chen X, Chen Y, et al. Radiomics nomogram for preoperative differentiation of lung tuberculoma from adenocarcinoma in solitary pulmonary solid nodule. Eur J Radiol 2020;128:109022. [Crossref] [PubMed]
- Lambin P, Leijenaar RTH, Deist TM, et al. Radiomics: the bridge between medical imaging and personalized medicine. Nat Rev Clin Oncol 2017;14:749-62. [Crossref] [PubMed]
- Sun W, Zheng B, Qian W. Automatic feature learning using multichannel ROI based on deep structured algorithms for computerized lung cancer diagnosis. Comput Biol Med 2017;89:530-9. [Crossref] [PubMed]
- Xu Y, Lu L. Application of Radiomics in Predicting the Malignancy of Pulmonary Nodules in Different Sizes. AJR Am J Roentgenol 2019;213:1213-20. [Crossref] [PubMed]
- Zhang R, Sun H, Chen B, et al. Developing of risk models for small solid and subsolid pulmonary nodules based on clinical and quantitative radiomics features. J Thorac Dis 2021;13:4156-68. [Crossref] [PubMed]
- Wu G, Jochems A, Refaee T, et al. Structural and functional radiomics for lung cancer. Eur J Nucl Med Mol Imaging 2021;48:3961-74. [Crossref] [PubMed]
- MacMahon H, Naidich DP, Goo JM, et al. Guidelines for Management of Incidental Pulmonary Nodules Detected on CT Images: From the Fleischner Society 2017. Radiology 2017;284:228-43. [Crossref] [PubMed]
- American College of Radiology. Lung-RADS. 2022 Assessment Categories. 2022.11. Available online: https://www.acr.org/Clinical-Resources/Reporting-and-Data-Systems/Lung-Rads
- Wood DE, Kazerooni EA, Aberle D. National Comprehensive Cancer Network (NCCN) Clinical Practice Guidelines in Oncology. Guidelines Version 1.2023 Lung Cancer Screening. 2022.10.26. Available online: https://nccnchina.org.cn/
- Byrne SC, Hammer MM. Malignant Nodules Detected on Lung Cancer Screening CT: Yield of Short-Term Follow-Up CT in Showing Nodule Growth. AJR Am J Roentgenol 2022;219:735-41. [Crossref] [PubMed]