The relationship between the collagen score at the anastomotic site of esophageal squamous cell carcinoma and anastomotic leakage
Highlight box
Key findings
• The study showed that the collagen score may be an important quantitative index of atony and blood supply to the conduit that can be used to predict anastomotic leakage (ALs).
What is known and what is new?
• Many clinical pathological characteristics could serve as predictive factors for AL, but their predictive performance value is relatively low.
• This study introduced a new method for quantifying the atony and blood supply to predict AL.
What is the implication, and what should change now?
• The collagen score may be a new method for quantifying the degree of atony and blood supply to the conduit in minimally invasive esophagectomy.
Introduction
Esophageal cancer is the sixth most common malignant tumor in China, and more than 95% of patients with esophageal cancer have esophageal squamous cell carcinoma; furthermore, esophageal cancer has the fifth highest mortality among cancers, with a five-year survival rate of approximately 15–30% (1). At present, the surgical methods commonly used for subtotal esophagectomy via the right thoracic approach include the preparation of an abdominal tube stomach, gastric bypass tube neck anastomosis (i.e., the McKeown procedure), and neck-chest abdominal lymph node dissection. The McKeown procedure involves multiple cumbersome steps, including the removal of both the thoracic esophagus and part of the stomach, lymph node dissection, and reconstruction with a tubularized gastric conduit. Three incisions are needed for this procedure, including incisions on the neck, the chest, and the abdomen. Three-field (3FL) radical surgery for esophageal cancer causes significant trauma and is associated with significant postoperative morbidity. Both operational and perioperative management pose significant challenges for surgeons, among which the most serious complication is anastomotic leakage (AL) (2). Among patients who underwent 3FL surgery, the incidence of AL is higher than that of intrathoracic anastomosis due to the high position of the anastomosis resulting in greater tension as well as a more compromised blood supply (3). Thus, reducing the incidence of AL after 3FL surgery and predicting AL have become the focus of perioperative research.
Previous research has shown that strong risk factors for AL include preoperative hypoalbuminemia, tumor location, preoperative complications, and neoadjuvant therapy. Furthermore, intraoperative outcomes, including the preservation of the gastric blood supply and the performance of a tension-free anastomosis, are related to surgeons’ technical abilities and experience (4-14). If a patient is considered to be at high risk for AL after surgery, the esophageal surgeon will use a series of perioperative treatments, such as increasing retention time of drainage tubes, prolonging dependence on jejunostomy tubes, and administering antibiotics intravenously (7-9). Thus, a precise prediction model for postoperative AL in esophageal cancer patients needs to be established to reduce the perioperative morbidity associated with this complication.
In surgical procedures, close attention is devoted to the atony and rich blood supply of the gastric tube, but these two factors are subjectively assessed by the primary surgeon. Currently, there is no quantitative method for clarifying the tension and blood supply of the gastric tube. Indocyanine green fluorescence angiography has been studied, but the results have been inconsistent.
Collagen is concentrated in the submucosa (15). Collagen is the main component of the extracellular matrix and the load-bearing “scaffold” of tissues (16). The collagen structure also affects the blood supply and function of different cells (17). Type I collagen is one of the most important components for tissue stability after anastomosis (18,19). One of the microscopic changes that occurs in AL tissue is collagen structure (20). Thus, in 3FL surgery, the tension and blood supply at the anastomotic site can be quantitatively analyzed using a collagen score to assess the risk of AL occurrence.
Second harmonic generation (SHG) imaging is a technique that uses nonlinear optics to generate SHG signals from tissues. This technique is commonly used in the field of biomedical imaging to visualize collagen fibers and other non-centrosymmetric structures (21). By detecting and analyzing SHG signals, SHG imaging allows for high-resolution and label-free visualization of tissue structures (22) and thus provides valuable insights into biological processes and pathological conditions. This noninvasive imaging modality has gained popularity due to its ability to provide detailed structural information with minimal photodamage, phototoxicity, and photobleaching, thus making it a powerful tool in biomedical research and clinical applications (23). We present this article in accordance with the TRIPOD reporting checklist (available at https://jtd.amegroups.com/article/view/10.21037/jtd-24-427/rc).
Methods
Patients and specimens
This study was approved by the Institutional Review Committee of Fujian Cancer Hospital (No. K2023-444-01). All the participants signed a written informed consent form. This study was conducted in accordance with the Helsinki Declaration (as revised in 2013). The inclusion criteria were as follows: (I) underwent a pathological examination to confirm primary cancer of the spinal canal; (II) underwent surgery with or without preoperative neoadjuvant therapy; and (III) underwent surgery for which the surgical methods included three incisions (one each in the neck, chest, and abdomen) for the radical resection of esophageal cancer and left cervical anastomosis with gastric bypass. The exclusion criteria were as follows: (I) had undergone left or right intrathoracic anastomosis; (II) had undergone palliative surgery; or (III) had a positive margin.
Neoadjuvant therapy and surgery
The patients did not receive neoadjuvant therapy before surgery, which included neoadjuvant chemotherapy, neoadjuvant chemoradiation, and/or neoadjuvant chemotherapy/immunotherapy. After the initial diagnosis, all patients were diagnosed with multidisciplinary esophageal cancer at our hospital. All surgeries were performed by the same medical team of senior surgeons specializing in esophageal cancer surgery at our hospital. The patient was first placed in the left lateral decubitus position, and a right thoracoscopy was performed to mobilize the thoracic esophagus and dissect the lymph nodes. Next, the patient was placed in the supine position, and the left neck and abdominal incisions were made to create the gastric conduit and perform a neck anastomosis. The anastomosis was performed using an end-to-end circular mechanical approach.
Definition of AL
In this study, AL was defined as a full-thickness digestive tract defect, including a cannula, anastomotic site, anastomotic nail, or gastric tube. All ALs were diagnosed by endoscopic and/or contrast imaging.
SHG imaging and quantitative analysis of collagen
The method for using the SHG imaging system that was employed in this study has been described in a previous study (24). In brief, the system consists of a high-throughput scanning inverted Axiovert200 microscope (ZeissLSM510META; Zeiss, Oberkochen, Germany) and a mode-locked femtosecond Tis laser (110 fs, 76 MHz) that can be tuned in the range of 700 to 980 nm (CoherentMira900-F; Coherent, Santa Clara, CA, USA) (Figure S1). The SHG imaging and collagen quantitative analysis were performed by an independent observer who was not aware of the clinical results. For SHG imaging, a 63× objective with an excitation wavelength of 800 nm was used. Three 512-µm by 512-µm regions in the submucosa were randomly selected on the gastric side of each donut for SHG imaging based on hematoxylin and eosin (HE) images. Images of other unstained sequence slices were collected and compared with the HE images for histological evaluation.
After image acquisition, an independent observer who was not aware of the clinical results used MATLAB 2015B (MathWorks, Natick, MA, USA) to extract the collagen structural features (23). First, the Gaussian mixture model method was used to segment the 15 SHG image into collagen pixels and background pixels (25). The percentage area of collagen was calculated as the ratio between the number of collagen pixels in the image and the total number of pixels. Next, the binary collagen mask image was processed using the network extraction algorithm described above to track each collagen fiber in the image and identify the crosslinking points (defined as the connection points between two or more fibers) (26). Finally, 11 collagen features were extracted, including the percentage area of collagen, average number of collagen fibers per square millimeter, average fiber density and standard deviation (SD) of fiber width, average fiber width and SD of fiber width, average fiber straightness and SD of fiber straightness, cross-linking density, and average cross-linking space and SD of cross-linking space. The average of the three collagen features obtained from the SHG imaging of each sample was calculated as the final collagen feature value. As a result, 142 collagen features on the gastric side of the anastomotic “donut” were obtained.
Selection of collagen features and construction of the collagen score
Models that incorporate multiple biomarkers are more accurate than those that incorporate individual biomarkers (27,28). Least absolute shrinkage and selection operator (LASSO) regression is a widely used feature screening method for predictive model construction, especially for high-dimensional parameter problems. LASSO binary logistic regression was used to select important predictors from all the collagen features, and the predictors were weighted according to their LASSO regression coefficients. Finally, a collagen score was calculated for each patient (28). We performed LASSO regression analysis using the software package “glmnet” (Foundation for Statistical Computing Research, Vienna, Austria).
Selection of clinicopathological features
Univariate and multivariate logistic regression analyses were performed on the following clinical and pathological features: age, sex, preoperative albumin (35 g/L was the preoperative low albumin level), neoadjuvant therapy, tumor location, posttreatment T phase, and posttreatment N phase.
Statistical analyses
All the statistical analyses were conducted using SPSS 23.0 software (IBM, Armonk, NY, USA) and R3.4.4 software. Continuous variables are represented as the mean (SD) or median (interquartile range). The Mann-Whitney U test was used to analyze the nonnormally distributed parameters. Student’s t-test was used to analyze the individual variables. The chi-square test (χ2) test and Fisher’s exact test were used to analyze the categorical variables. The significant variables in the univariate analysis were included in the multivariate analysis. The odds ratios (ORs) and 95% confidence intervals (CIs) of the risk factors were analyzed using multiple logistic regression. All the P tests were two-sided.
Results
Patient demographics and cancer characteristics
After applying the inclusion and exclusion criteria, data from a total of 938 patients who underwent 3FL radical surgery for esophageal cancer at Fujian Cancer Hospital from January 2020 to January 2023 were retrospectively analyzed. According to the diagnostic criteria for AL, 20 patients with postoperative AL were identified, yielding an incidence rate of 2.17%. These 20 patients were randomly divided into an experimental group and a validation group (with 10 patients per group). In addition, 193 patients who had never experienced AL were randomly assigned to the experimental cohort (103 patients) and the validation cohort (90 patients).
Changes in the collagen score
The LASSO logistic regression model was used to select the predictive collagen features in the main cohort, and the following three significant predictive factors from the 142 collagen features were selected: histogram mean, histogram variance, and histogram energy. Next, based on the individualized levels of each patient’s three collagen features, the following formula was derived to calculate the collagen score: collagen score = histogram variance × 0.468 – histogram mean × 0.153 – histogram energy × 0.265 (Figure 1). Representative HE and SHG images of distal and proximal donuts in the AL and non-AL patients are shown in Figure 2.
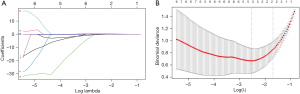
Strong risk factors for AL
In terms of the clinical pathological characteristics, univariate analysis revealed significant differences in the preoperative low albumin levels between the AL and non-AL groups in the experimental and validation cohorts (P=0.002), but no statistically significant differences were found for any other clinical pathological features (Table 1). In the multivariate analysis, no statistically significant difference in preoperative low albumin levels was observed (P=0.35) (Table 2).
Table 1
Baseline variable | Primary cohort (n=113) | Validation cohort (n=100) | |||||
---|---|---|---|---|---|---|---|
Non-AL (n=103) | AL (n=10) | P value* | Non-AL (n=90) | AL (n=10) | P value* | ||
Age, year | 0.10 | >0.99 | |||||
<65 | 52 (50.5) | 2 (20.0) | 24 (26.7) | 3 (30.0) | |||
≥65 | 51 (49.5) | 8 (80.0) | 66 (73.3) | 7 (70.0) | |||
Sex | 0.73 | 0.34 | |||||
Female | 29 (28.2) | 2 (20.0) | 47 (52.2) | 7 (70.0) | |||
Male | 74 (71.8) | 8 (80.0) | 43 (47.8) | 3 (30.0) | |||
Preoperative Alb, g/L | 0.002 | 0.002 | |||||
≥35 | 99 (96.1) | 6 (60.0) | 83 (92.2) | 5 (50.0) | |||
<35 | 4 (3.9) | 4 (40.0) | 7 (7.8) | 5 (50.0) | |||
Primary site | 0.17 | 0.31 | |||||
Upper | 9 (8.7) | 1 (10.0) | 8 (8.9) | 2 (20.0) | |||
Middle | 53 (51.5) | 8 (80.0) | 45 (50.0) | 6 (60.0) | |||
Lower | 41 (39.8) | 1 (10.0) | 37 (41.1) | 2 (20.0) | |||
Neoadjuvant therapy | 0.74 | 0.09 | |||||
No | 52 (50.5) | 6 (60.0) | 55 (61.1) | 3 (30.0) | |||
Yes | 51 (49.5) | 4 (40.0) | 35 (38.9) | 7 (70.0) | |||
pT stage | 0.99 | 0.56 | |||||
0 | 13 (12.6) | 1 (10.0) | 7 (7.8) | 1 (10.0) | |||
1 | 23 (22.3) | 2 (20.0) | 18 (20.0) | 4 (40.0) | |||
2 | 7 (6.8) | 1 (10.0) | 12 (13.3) | 1 (10.0) | |||
3 | 52 (50.5) | 5 (50.0) | 43 (47.8) | 4 (40.0) | |||
4 | 8 (7.8) | 1 (10.0) | 10 (11.1) | 0 (0.0) | |||
pT stage | >0.99 | 0.32 | |||||
0–2 | 43 (41.7) | 4 (40.0) | 37 (41.1) | 6 (60.0) | |||
3–4 | 60 (58.3) | 6 (60.0) | 53 (58.9) | 4 (40.0) | |||
pN stage | 0.51 | 0.32 | |||||
N0 | 56 (54.4) | 4 (40.0) | 44 (48.9) | 7 (70.0) | |||
N+ | 47 (45.6) | 6 (60.0) | 46 (51.1) | 3 (30.0) | |||
pN stage | 0.58 | 0.19 | |||||
0 | 56 (54.4) | 4 (40.0) | 44 (48.9) | 7 (70.0) | |||
1 | 22 (21.4) | 2 (20.0) | 23 (25.6) | 0 (0.0) | |||
2 | 17 (16.5) | 2 (20.0) | 16 (17.8) | 3 (30.0) | |||
3 | 8 (7.8) | 2 (20.0) | 7 (7.8) | 0 (0.0) | |||
Collagen score | 1.494 [1.038] | 6.437 [2.527] | <0.001 | 1.781 [2.059] | 2.589 [2.554] | <0.001 |
Data are presented as n (%) or mean [SD]. *, χ2 test or Fisher’s exact test. AL, anastomotic leakage; Alb, albumin; pT stage, pathological T stage; pN stage, pathological N stage; SD, standard deviation.
Table 2
Baseline variable | Univariate logistic regression | Multivariate logistic regression | |||
---|---|---|---|---|---|
OR (95% CI) | P value | OR (95% CI) | P value | ||
Age, years | |||||
<65 | Reference | ||||
≥65 | 4.078 (0.826–20.138) | 0.08 | |||
Sex | |||||
Female | Reference | ||||
Male | 1.568 (0.314–7.826) | 0.58 | |||
Preoperative Alb, g/L | |||||
≥35 | Reference | – | |||
<35 | 16.5 (3.290–82.743) | 0.001 | – | 0.35 | |
Primary site | |||||
Upper | Reference | ||||
Middle | 1.358 (0.151–12.207) | 0.78 | |||
Lower | 0.220 (0.013–3.849) | 0.30 | |||
Neoadjuvant therapy | |||||
No | Reference | ||||
Yes | 0.680 (0.181–2.552) | 0.57 | |||
pT stage | |||||
0–2 | Reference | ||||
3–4 | 1.075 (0.286–4.042) | 0.92 | |||
pN stage | |||||
N0 | Reference | ||||
N+ | 1.787 (0.476–6.712) | 0.39 | |||
Collagen score | 0.131 (0.036–0.474) | 0.002 | 0.131 (0.036–0.474) | 0.002 |
OR, odds ratio; CI, confidence interval; Alb, albumin; pT stage, pathological T stage; pN stage, pathological N stage.
In terms of the collagen score, the results of the univariate analysis showed that in the experimental cohort, the collagen score [mean (SD)] of the AL patients was lower than that of the non-AL patients [1.494 (1.038) vs. 6.437 (2.527), P<0.001] (Table 1). The areas under the curve (AUCs) of the experimental and validation cohorts were 0.978 (95% CI: 0.931–0.996) and 0.900 (95% CI: 0.824–0.951), respectively (Figure 3).
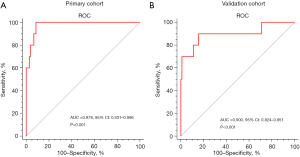
Discussion
At present, there are various methods for accessing gastroesophageal anastomosis, including hand-sewing and mechanical anastomosis. End-to-side circular anastomosis is a mainstream anastomosis method and has been adopted at many centers. Strong risk factors for AL include intraoperative factors and patient comorbidities (e.g., diabetes and peripheral vascular disease). Many centers have conducted similar studies on the clinical and pathological characteristics of patients in the past.
Due to the high anastomotic position, poor tension, and inadequate blood supply at the anastomotic site during 3FL surgery for esophageal cancer, postoperative AL has long been considered one of the most serious complications (2,3). During surgery, close attention should be devoted to the establishment of the gastric tube. The formation of the gastric tube determines its length and the preservation of blood supply. However, there is currently no reliable quantitative detection method for measuring the tension and blood supply at the gastroesophageal anastomosis site.
As the main component of the extracellular matrix, collagen provides integrity and structure in healthy tissues (29). Collagen also affects the biological activity of surrounding cells, such as the blood supply, tissue repair, and tissue development (30). Thus, maintaining normal collagen content and structure is crucial for wound healing and stabilizing tissue function. Generally, there is no difference in collagen levels in the human stomach (26). However, in surgical procedures and the production of a gastric tube, the probability of postoperative AL is determined by the protection of the mucosal vascular network under the gastric tube and the quality of the blood supply to the gastric tube at the anastomotic site.
In this study, we found a significant correlation between the collagen score (determined based on SHG imaging and a quantitative analysis) and AL. Using LASSO regression, we constructed a formula for the collagen score using three collagen features of gastric donuts and compared these three collagen features between AL and non-AL patients on the gastric side of all patients. We found significant differences in the following three collagen characteristics of gastric donuts: histogram mean, histogram variance, and histogram energy. We calculated the collagen score of the patients and found sufficient differentiation in the main cohort (AUC: 0.901) and validation cohort (AUC: 0.886). Therefore, the collagen score may serve as a new clinical biomarker to accurately predict the AL of postoperative 3FL surgery patients.
In this study, based on the univariate analysis, preoperative albumin and collagen scores were identified as independent predictors of AL. A low preoperative albumin level is an independent risk factor for AL. Tumors can lead to increased metabolism, reduced dietary intake, and impaired liver protein synthesis in patients, which ultimately affects tissue healing and increases the risk of postoperative AL. However, in the multivariate analysis, no statistically significant difference in preoperative low albumin values was detected between the two groups of patients. However, this analysis was limited by the low incidence of AL at our center (20/938, 2.13%). The results of the multivariate analysis revealed that the collagen score based on collagen protein was an independent risk factor for AL, which suggests that collagen can serve as a predictor of AL. Thus, based on the collagen score constructed in this study, postoperative nutritional support and delayed feeding should be strengthened for patients with low scores.
This study had several limitations. This was a single-center retrospective study with a small sample size, and the sample size of positive patients was small, so the results may be biased. In addition, the patients included in this study were all patients in southern China, and they all had esophageal squamous cell carcinoma; therefore, this study should be expanded to a prospective international multicenter clinical study in the future. In addition, this study did not stratify patients according to whether neoadjuvant therapy was completed. In future research, patients should be stratified based on whether neoadjuvant therapy was completed and based on the strategy of neoadjuvant therapy applied. Furthermore, future studies should include larger sample sizes.
Conclusions
The collagen score constructed in this study was shown to be related to AL. The collagen score can be used to predict postoperative AL in esophageal cancer patients who underwent 3FL surgery.
Acknowledgments
Funding: This study was supported by
Footnote
Reporting Checklist: The authors have completed the TRIPOD reporting checklist. Available at https://jtd.amegroups.com/article/view/10.21037/jtd-24-427/rc
Data Sharing Statement: Available at https://jtd.amegroups.com/article/view/10.21037/jtd-24-427/dss
Peer Review File: Available at https://jtd.amegroups.com/article/view/10.21037/jtd-24-427/prf
Conflicts of Interest: All authors have completed the ICMJE uniform disclosure form (available at https://jtd.amegroups.com/article/view/10.21037/jtd-24-427/coif). The authors have no conflicts of interest to declare.
Ethical Statement: The authors are accountable for all aspects of the work in ensuring that questions related to the accuracy or integrity of any part of the work are appropriately investigated and resolved. This study was approved by the Institutional Review Committee of Fujian Cancer Hospital (No. K2023-444-01). All the participants signed a written informed consent form. This study was conducted in accordance with the Helsinki Declaration (as revised in 2013).
Open Access Statement: This is an Open Access article distributed in accordance with the Creative Commons Attribution-NonCommercial-NoDerivs 4.0 International License (CC BY-NC-ND 4.0), which permits the non-commercial replication and distribution of the article with the strict proviso that no changes or edits are made and the original work is properly cited (including links to both the formal publication through the relevant DOI and the license). See: https://creativecommons.org/licenses/by-nc-nd/4.0/.
References
- Sung H, Ferlay J, Siegel RL, et al. Global Cancer Statistics 2020: GLOBOCAN Estimates of Incidence and Mortality Worldwide for 36 Cancers in 185 Countries. CA Cancer J Clin 2021;71:209-49. [Crossref] [PubMed]
- Takeuchi H, Miyata H, Gotoh M, et al. A risk model for esophagectomy using data of 5354 patients included in a Japanese nationwide web-based database. Ann Surg 2014;260:259-66. [Crossref] [PubMed]
- Altorki N, Kent M, Ferrara C, et al. Three-field lymph node dissection for squamous cell and adenocarcinoma of the esophagus. Ann Surg 2002;236:177-83. [Crossref] [PubMed]
- Honda M, Kuriyama A, Noma H, et al. Hand-sewn versus mechanical esophagogastric anastomosis after esophagectomy: a systematic review and meta-analysis. Ann Surg 2013;257:238-48. [Crossref] [PubMed]
- Yoshida N, Baba Y, Watanabe M, et al. Triangulating stapling technique covered with the pedicled omental flap for esophagogastric anastomosis: a safe anastomosis with fewer complications. J Am Coll Surg 2015;220:e13-6. [Crossref] [PubMed]
- Anegg U, Lindenmann J, Maier A, et al. Influence of route of gastric transposition on oxygen supply at cervical oesophagogastric anastomoses. Br J Surg 2008;95:344-9. [Crossref] [PubMed]
- Yamasaki M, Miyata H, Yasuda T, et al. Impact of the route of reconstruction on post-operative morbidity and malnutrition after esophagectomy: a multicenter cohort study. World J Surg 2015;39:433-40. [Crossref] [PubMed]
- Zhai C, Liu Y, Li W, et al. A comparison of short-term outcomes between Ivor-Lewis and McKeown minimally invasive esophagectomy. J Thorac Dis 2015;7:2352-8. [Crossref] [PubMed]
- Van Daele E, Van de Putte D, Ceelen W, et al. Risk factors and consequences of anastomotic leakage after Ivor Lewis oesophagectomy. Interact Cardiovasc Thorac Surg 2016;22:32-7. [Crossref] [PubMed]
- Tsutsui S, Moriguchi S, Morita M, et al. Multivariate analysis of postoperative complications after esophageal resection. Ann Thorac Surg 1992;53:1052-6. [Crossref] [PubMed]
- Fischer C, Lingsma H, Hardwick R, et al. Risk adjustment models for short-term outcomes after surgical resection for oesophagogastric cancer. Br J Surg 2016;103:105-16. [Crossref] [PubMed]
- Kechagias A, van Rossum PSN, Ruurda JP, et al. Ischemic Conditioning of the Stomach in the Prevention of Esophagogastric Anastomotic Leakage After Esophagectomy. Ann Thorac Surg 2016;101:1614-23. [Crossref] [PubMed]
- Zehetner J, DeMeester SR, Alicuben ET, et al. Intraoperative Assessment of Perfusion of the Gastric Graft and Correlation With Anastomotic Leaks After Esophagectomy. Ann Surg 2015;262:74-8. [Crossref] [PubMed]
- Yamana I, Takeno S, Shibata R, et al. Is the Geriatric Nutritional Risk Index a Significant Predictor of Postoperative Complications in Patients with Esophageal Cancer Undergoing Esophagectomy? Eur Surg Res 2015;55:35-42. [Crossref] [PubMed]
- Maier F, Siri S, Santos S, et al. The heterogeneous morphology of networked collagen in distal colon and rectum of mice quantified via nonlinear microscopy. J Mech Behav Biomed Mater 2021;113:104116. [Crossref] [PubMed]
- Siri S, Zhao Y, Maier F, et al. The Macro- and Micro-Mechanics of the Colon and Rectum I: Experimental Evidence. Bioengineering (Basel) 2020;7:130. [Crossref] [PubMed]
- Baranzini N, Pulze L, Tettamanti G, et al. HvRNASET2 Regulate Connective Tissue and Collagen I Remodeling During Wound Healing Process. Front Physiol 2021;12:632506. [Crossref] [PubMed]
- Xi G, Wang Q, Zhan H, et al. Automated classification of breast cancer histologic grade using multiphoton microscopy and generative adversarial networks. J Phys D: Appl Phys 2023;56:01540.
- Meng J, Wang G, Zhou L, et al. Mapping variation of extracellular matrix in human keloid scar by label-free multiphoton imaging and machine learning. J Biomed Opt 2023;28:045001. [Crossref] [PubMed]
- Edomskis P, Goudberg MR, Sparreboom CL, et al. Matrix metalloproteinase-9 in relation to patients with complications after colorectal surgery: a systematic review. Int J Colorectal Dis 2021;36:1-10. [Crossref] [PubMed]
- Zhan H, Chen S, Gao F, et al. AutoFibroNet: A deep learning and multi-photon microscopy-derived automated network for liver fibrosis quantification in MAFLD. Aliment Pharmacol Ther 2023;58:573-84. [Crossref] [PubMed]
- Dong S, Wang H, Ji H, et al. Development and validation of a collagen signature to predict the prognosis of patients with stage II/III colorectal cancer. iScience 2023;26:106746. [Crossref] [PubMed]
- Jiang W, Wang H, Chen W, et al. Association of collagen deep learning classifier with prognosis and chemotherapy benefits in stage II-III colon cancer. Bioeng Transl Med 2023;8:e10526. [Crossref] [PubMed]
- Yan J, Zheng X, Liu Z, et al. Multiphoton imaging provides a superior optical biopsy to that of confocal laser endomicroscopy imaging for colorectal lesions. Endoscopy 2019;51:174-8. [Crossref] [PubMed]
- Dempster AP. Maximum likelihood from incomplete data via the EM algorithm. J R Stat Soc 1977;39:1-38.
- Wang G, Zhan H, Luo T, et al. Automated ovarian cancer identification using end-to-end deep learning and second harmonic generation imaging. IEEE J Sel Top Quantum Electron 2023;29:7200609.
- Jiang Y, Jin C, Yu H, et al. Development and Validation of a Deep Learning CT Signature to Predict Survival and Chemotherapy Benefit in Gastric Cancer: A Multicenter, Retrospective Study. Ann Surg 2021;274:e1153-61. [Crossref] [PubMed]
- Huang YQ, Liang CH, He L, et al. Development and Validation of a Radiomics Nomogram for Preoperative Prediction of Lymph Node Metastasis in Colorectal Cancer. J Clin Oncol 2016;34:2157-64. [Crossref] [PubMed]
- Sauerbrei W, Boulesteix AL, Binder H. Stability investigations of multivariable regression models derived from low- and high-dimensional data. J Biopharm Stat 2011;21:1206-31. [Crossref] [PubMed]
- Kisling A, Lust RM, Katwa LC. What is the role of peptide fragments of collagen I and IV in health and disease? Life Sci 2019;228:30-4. [Crossref] [PubMed]