The predictive value of serum fibrinogen and platelet distribution width for long-term cardiac death in acute myocardial infarction patients
Highlight box
Key findings
• In this study, we found that platelet distribution width (PDW) and fibrinogen seem to be useful as biomarkers for long-term death prediction post-myocardial infarction (MI).
What is known and what is new?
• Previous studies have shown that PDW and fibrinogen are independent risk factors for long-term major adverse cardiovascular event (MACE) in acute coronary syndrome patients.
• In this study, we demonstrate PDW and fibrinogen as useful biomarkers for long-term death prediction post-MI in our Chinese acute myocardial infarction patients.
What is the implication, and what should change now?
• This study only recorded the hard endpoint event of death post-MI, neglecting other MACE. Death was the most critical event, but other MACE were also important to evaluate the long-term prognosis of patients post-MI. Therefore, the follow-up study will record all MACE post-MI to confirm the predictive value of relevant biomarkers found in this research.
Introduction
Coronary heart disease (CHD) is the leading cause of death worldwide, with acute myocardial infarction (AMI) being its most severe manifestation (1). Currently, AMI has become the second leading cause of death in China, and its incidence is increasing year by year. The number of people living with AMI is expected to reach 23 million by 2030 (2). AMI refers to acute myocardial tissue damage resulting from coronary thrombosis and subsequent occlusion of the coronary arteries, usually triggered by the erosion or rupture of unstable atherosclerotic plaques (2). AMI can cause chest pain and arrhythmias and other complications such as heart rupture with rapid onset and disease progression, which can be life-threatening if not treated in time (3). Currently, the treatment for AMI is mainly to open the culprit vessels by percutaneous coronary intervention (PCI) (4). Although the timely and effective opening of the culprit vessels and restoration of myocardial perfusion has greatly reduced the mortality rate of these patients, persistent myocardial ischemia and myocardial-reperfusion injury still induce major adverse cardiovascular events (MACEs), of which the hard endpoint mortality rate in hospital or discharge approaches 4–6% (5,6). Therefore, AMI is still one of the major diseases threatening human life even after opening of the criminal blood vessels promptly. Identifying high-risk patients early and strengthening the treatment and management are particularly critical to improve the long-term prognosis of these patients and reduce the mortality of these patients.
Numerous prior studies have indicated that many risk factors are associated with long-term MACE post-myocardial infarction (MI), including diabetes, hyperlipidemia, and lifestyle behaviors such as smoking and mental stress (7-9). However, these traditional risk factors merely suggest a high probability of MACE occurrence but are not predictive. It is well known that biomarkers from blood have become predictors for many diseases because of their stability and easy availability, so it is urgent to find biomarkers from blood to predict the probability of MACE post-MI, especially for the hard endpoint event of death. A good biomarker should have the following features: (I) high sensitivity and specificity for prediction; (II) low sample size for point-of-care analysis; and (III) multiple functions in clinical application, such as disease-specific manifestations with routine analytical features (10,11). Previous biomarkers, such as troponin and creatine kinase, are more commonly used to diagnose MI than to determine prognosis (12). Current studies have already found many biomarkers, including noncoding RNAs, to predict long-term MACE post-MI, but these biomarkers generally require special test kits or clinical non-routine examination items, so they do not fulfill the principle of biomarker selection (13-15).
In this retrospective study, we sought to explore novel, readily available, and common clinical biomarkers possibly associated with the hard endpoint event of death post-MI after balancing the traditional risk factors. We present this article in accordance with the STARD reporting checklist (available at https://jtd.amegroups.com/article/view/10.21037/jtd-24-204/rc).
Methods
Study design and patients
This study was a retrospective case-control study. Consecutive 1,273 patients aged ≥18 years who were diagnosed with AMI in Shanghai Sixth People’s Hospital Affiliated to Shanghai Jiao Tong University School of Medicine from January 2017 to September 2019 were selected, and the follow-up period was at least 4 years. After excluding patients who had at least 1 exclusion criterion, a total of 1,059 patients with AMI were enrolled, of which 130 died from AMI, and 929 patients with AMI who did not die were used as the control group. Clinical data were collected from the initial medical exposure of patients with AMI who were hospitalized. Exclusion criteria included prior PCI or percutaneous transluminal coronary angioplasty (PTCA) therapy, acute stroke within 1-month, severe liver dysfunction, thyroid dysfunction, congenital heart disease, rheumatic or prosthetic heart disease, pulmonary artery disease, acute or chronic respiratory failure, and any diseases of non-MI that led to troponin elevation. All enrolled patients agreed to participate in the study. The study was conducted in accordance with the Declaration of Helsinki (as revised in 2013). The study was approved by the Ethics Committee of Shanghai Sixth People’s Hospital Affiliated to Shanghai Jiao Tong University School of Medicine [2021-KY-034(K)-(1)] and individual consent for this retrospective analysis was waived.
Definition and treatment of AMI
Patients have obvious clinical manifestations of chest discomfort, especially severe chest pain and radiating pain into the left arm, right arm, or neck/jaw. Some patients have no obvious chest pain, usually manifesting as stomach discomfort, vomiting, and even a shock state. Electrocardiogram (ECG) should be taken within 10 minutes in these patients. ECG shows that the corresponding lead ST-segment elevation with or without elevated levels of the blood marker high-sensitivity troponin can be a preliminary diagnosis of ST-segment elevation MI (STEMI). ST-segment depression with elevated biomarker high-sensitivity troponin is the preliminary diagnosis of non-ST-elevation MI (NSTEMI). The final diagnosis is based on coronary angiography, with complete occlusion of the infarct-related artery (IRA) diagnosed as STEMI and severe stenosis of the IRA with restricted blood flow diagnosed as NSTEMI. For patients with confirmed STEMI, the IRA will be opened within 12 hours. For NSTEMI patients, the IRA will be opened within 12, 24, and 72 hours according to risk stratification, and all AMI patients will be treated with optimal drug therapy according to the guidelines (4).
Diagnoses and definitions
Hypertension is diagnosed based on systolic blood pressure and/or diastolic blood pressure ≥140/90 mmHg or previous diagnosis and current anti-hypertensive drug treatment. The diagnosis of diabetes mellitus is based on fasting for at least 8 hours with blood glucose ≥7.0 mmol/L or 2-hour oral glucose tolerance test (OGTT) blood glucose ≥11.1 mmol/L or random blood glucose ≥11.1 mmol/L or the patient has a history of diabetes mellitus and is currently being treated with anti-hyperglycemic drugs. Dyslipidemia can be diagnosed when the total cholesterol level is ≥6.22 mmol/L, the low-density cholesterol level is ≥4.14 mmol/L, the high-density cholesterol level is ≥1.55 or ≤1.04 mmol/L or the triglyceride level is ≥2.26 mmol/L. Chronic kidney disease is diagnosed based on the corresponding guideline diagnostic criteria, while the diagnosis of tumors mainly refers to the patient’s past medical history. Other diseases, including lung diseases and other cardiovascular diseases, are all diagnosed based on the corresponding guideline diagnostic criteria. Patients with the above conditions were considered positive and calculated as a percentage.
Biochemical markers examination
Venous blood samples were obtained upon admission from each AMI patient and analyzed for biochemical markers, lipids, and blood glucose. The assays were performed according to the corresponding kit instructions.
Statistical analysis
Categorical variables were presented as percentages, and unadjusted categorical variables between two groups were compared using χ2 or Fisher’s exact test or the Cochrane Mantel-Haenszel (CMH) test (where applicable). Continuous variables were expressed as the mean ± standard deviation (SD) or median [interquartile range (IQR)]. The Shapiro-Wilk test was used to confirm whether the continuous data were normally distributed. If the continuous variables were normally distributed, an independent sample t-test was used for comparison. For non-normally distributed data, the Wilcoxon rank sum test was used.
The propensity score method was used to mitigate the influence of nonrandom selection bias and confounding variables of patients in the MI-death group and MI-no-death group. The individual propensity score is calculated as the individual covariates of the conditional probability of death given the presence of AMI. To count the scores, a logistic regression model with the following covariates was observed: (I) demographic variables such as age, sex, height, weight, body mass index (BMI), systolic blood pressure, diastolic blood pressure, smoking, and drinking; (II) clinical variables such as diabetes, hypertension, chronic kidney disease, tumor and percentage of STEMI. The nearest neighbor matching (NNM) method without calipers was applied to find pairs. Based on the propensity score, forward logistic regression analysis was used in the MI-no-dead group to find the sample with the closest score to the MI-death group sample and form a pair.
Cox proportional hazard regression analyses were examined on those significant variables calculated by paired t-test or Wilcoxon rank-sum test. Standard error, hazard ratio (HR), and 95% confidence interval (CI) were recorded. A receiver operating characteristic (ROC) curve was created to estimate the predictive value of those significant variables found by Cox proportional hazard regression analysis. Kaplan-Meier curve with log-rank test was performed to compare the proportion of patients with low and high platelet distribution width (PDW) and fibrinogen having survival during follow-up. Statistical significance was defined as P<0.05. All statistical analyses were calculated using SPSS 26.0 statistical software.
Results
The selected population of this study included patients with AMI from 1st January 2017 to 30th September 2019. According to the exclusion criteria, the final total number of patients entered into the cohort was 1,059. A total of 929 patients with AMI did not have a hard end-point of death, which was the control group, and a total of 130 people with MI-related death were the case group. After propensity score matching, a total of 116 pairs of balanced cohorts were matched.
Comparison of baseline demographic and clinical variables before and after propensity score matching
The baseline data of the patients in the two groups before and after matching are shown in Table 1. As many as 7 covariates were dramatically different between the two groups before matching. However, only 1 covariate remained significantly different between the two groups after propensity score matching.
Table 1
Variables | Unmatched analysis | Propensity score matched analysis | |||||
---|---|---|---|---|---|---|---|
Death (n=130) | Survival (n=929) | P value | Death (n=116) | Survival (n=116) | P value | ||
Age (years) | 74.0 (66.0–83.0) | 65.0 (56.0–73.0) | <0.001 | 74.0 (66.0–83.0) | 71.5 (60.0–80.0) | 0.045 | |
Sex (male, %) | 75.4 | 80.4 | 0.18 | 76.7 | 78.4 | 0.75 | |
Height (cm) | 170.0 (160.0–172.8) | 170.0 (164.0–173.0) | 0.16 | 170.0 (160.0–172.0) | 168.0 (160.0–172.0) | 0.79 | |
Weight (kg) | 65.5 (59.3–72.0) | 70.0 (60.0–77.0) | 0.004 | 65.5 (59.3–70.0) | 65.0 (60.0–75.0) | 0.48 | |
BMI (kg/m2) | 23.6 (21.1–25.7) | 24.2 (22.3–26.6) | 0.02 | 23.6 (21.4–25.5) | 24.0 (21.3–26.1) | 0.35 | |
SBP (mmHg) | 131.0 (109.0–147.0) | 130.0 (114.0–147.0) | 0.39 | 131.0 (108.0–148.0) | 125.0 (111.0–144.0) | 0.92 | |
DBP (mmHg) | 78.0 (67.0–86.0) | 79.0 (69.0–88.0) | 0.06 | 78.0 (66.0–86.0) | 74.0 (66.0–84.0) | 0.37 | |
Smoking (%) | 62.3 | 52.5 | 0.04 | 50.0 | 49.1 | 0.90 | |
Drinking (%) | 6.9 | 10.7 | 0.19 | 7.8 | 9.5 | 0.64 | |
Diabetes (%) | 47.7 | 29.5 | <0.001 | 46.6 | 46.6 | >0.99 | |
Hypertension (%) | 76.9 | 62.1 | 0.001 | 75.9 | 69.0 | 0.24 | |
Chronic kidney disease (%) | 13.1 | 1.1 | <0.001 | 7.8 | 7.8 | >0.99 | |
Tumor (%) | 2.3 | 0.6 | 0.09 | 1.7 | 2.6 | >0.99 | |
STEMI (%) | 65.4 | 68.0 | 0.55 | 68.1 | 63.8 | 0.49 |
Continuous variables are presented as median with interquartile ranges for data with non-normal distributions. BMI, body mass index; SBP, systolic blood pressure; DBP, diastolic blood pressure; STEMI, ST-segment elevation myocardial infarction.
Comparison of routine blood and biochemical markers before and after propensity score matching
The general statistics of routine blood and biochemical markers of the two groups are shown in Table 2. Before matching, 29 parameters were significantly different between the two groups, as listed in Table 2. After propensity score matching, only 21 parameters were significantly different between the two groups, including white blood cell count, lymphocyte%, eosinophil%, lymphocyte count, monocyte count, eosinophil count, mean corpuscular hemoglobin, mean corpuscular hemoglobin concentration, red blood cell distribution width, PDW, mean platelet volume, glucose, creatinine, urea nitrogen, uric acid, lipoprotein α, thrombin time, fibrinogen, D-dimer, C-reactive protein and procalcitonin.
Table 2
Variables | Unmatched analysis | Propensity score matched analysis | |||||
---|---|---|---|---|---|---|---|
Death (n=130) | Survival (n=929) | P value | Death (n=116) | Survival (n=116) | P value | ||
Hemoglobin (g/L) | 129.000 (112.000–143.000) | 139.500 (128.000–150.000) | <0.001 | 131.000 (112.300–145.000) | 135.500 (121.000–142.000) | 0.36 | |
Red blood cell (1012/L) | 4.255 (3.810–4.775) | 4.580 (4.210–4.960) | <0.001 | 4.315 (3.853–4.800) | 4.415 (4.010–4.725) | 0.55 | |
Platelet (109/L) | 209.500 (166.000–257.000) | 214.000 (178.250–252.750) | 0.16 | 208.000 (166.500–259.250) | 199.000 (169.500–241.250) | 0.71 | |
White blood cell (109/L) | 9.950 (7.900–13.275) | 9.500 (7.700–11.800) | 0.06 | 10.200 (8.225–13.600) | 9.200 (7.300–11.300) | 0.03 | |
Neutrophil (%) | 80.900 (73.300–87.100) | 79.000 (71.225–85.600) | 0.04 | 81.000 (73.300–87.100) | 77.550 (68.700–85.675) | 0.08 | |
Lymphocyte (%) | 11.800 (7.525–17.875) | 14.100 (9.600–20.600) | 0.001 | 11.800 (7.525–18.200) | 15.100 (9.300–23.100) | 0.01 | |
Monocyte (%) | 5.300 (3.800–7.400) | 5.000 (3.500–6.700) | 0.048 | 5.150 (3.775–7.325) | 5.200 (3.400–7.000) | 0.34 | |
Eosinophil (%) | 0.100 (0.000–0.725) | 0.400 (0.100–1.100) | <0.001 | 0.100 (0.000–0.700) | 0.400 (0.100–1.100) | 0.008 | |
Neutrophil (109/L) | 8.000 (5.600–11.000) | 7.500 (5.700–9.600) | 0.10 | 8.100 (5.800–11.400) | 7.000 (5.000–9.700) | 0.051 | |
Lymphocyte (109/L) | 1.150 (0.800–1.525) | 1.300 (1.000–1.800) | 0.001 | 1.150 (0.800–1.625) | 1.400 (1.000–1.800) | 0.03 | |
Monocyte (109/L) | 0.500 (0.400–0.800) | 0.500 (0.300–0.600) | <0.001 | 0.600 (0.400–0.800) | 0.400 (0.300–0.600) | 0.001 | |
Eosinophil (109/L) | 0.020 (0.000–0.063) | 0.040 (0.010-0.900) | <0.001 | 0.015 (0.000–0.060) | 0.040 (0.010–0.090) | 0.02 | |
Hematocrit (%) | 38.500 (33.750–42.925) | 41.500 (38.200–44.400) | <0.001 | 39.400 (33.950–43.300) | 39.900 (36.800–42.600) | 0.58 | |
Mean corpuscular volume (fL) | 90.750 (87.900–94.600) | 90.400 (87.200–92.900) | 0.23 | 90.650 (87.825–93.750) | 90.900 (87.600–94.000) | 0.58 | |
Mean corpuscular hemoglobin (Pg) | 30.200 (29.100–31.200) | 30.400 (29.500–31.400) | 0.08 | 30.200 (29.100–31.200) | 30.500 (29.500–31.800) | 0.04 | |
Mean corpuscular hemoglobin concentration (g/L) | 333.000 (323.500–339.000) | 337.000 (329.000–343.000) | <0.001 | 333.000 (323.500–339.000) | 336.000 (327.000–343.000) | 0.03 | |
Red blood cell distribution width (%) | 13.550 (13.000–14.025) | 13.000 (12.500–13.300) | <0.001 | 13.300 (13.000–14.000) | 13.000 (12.900–14.000) | 0.03 | |
Plateletcrit (%) | 0.225 (0.190–0.273) | 0.230 (0.200–0.275) | 0.22 | 0.225 (0.190–0.273) | 0.220 (0.190–0.260) | 0.48 | |
Platelet distribution width (%) | 13.600 (12.000–15.625) | 12.800 (11.700–14.625) | 0.008 | 13.500 (12.100–15.400) | 12.500 (11.500–14.200) | 0.007 | |
Mean platelet volume (fL) | 11.200 (10.500–12.000) | 10.900 (10.300–11.600) | 0.02 | 11.200 (10.500–12.000) | 10.800 (10.300–11.600) | 0.02 | |
Kalium (mmol/L) | 4.000 (3.500–4.300) | 3.900 (3.600–4.200) | 0.09 | 4.000 (3.500–4.300) | 3.900 (3.500–4.275) | 0.72 | |
Natrium (mmol/L) | 139.500 (137.000–142.000) | 140.000 (138.000–142.000) | 0.09 | 140.000 (136.250-142.000) | 140.000 (138.000–142.000) | 0.27 | |
Chlorinum (mmol/L) | 101.000 (97.000–103.000) | 102.000 (100.000–104.000) | 0.004 | 101.000 (97.000–103.000) | 102.000 (99.000–105.000) | 0.08 | |
Glucose (mmol/L) | 8.085 (6.210–11.565) | 6.590 (5.640–8.300) | <0.001 | 8.200 (6.188–11.805) | 6.910 (5.983–9.315) | 0.02 | |
Glycated hemoglobin (%) | 6.500 (6.000–7.500) | 6.000 (5.600–7.000) | <0.001 | 6.450 (5.900–7.730) | 6.350 (5.600–7.530) | 0.14 | |
Alanine aminotransferase (U/L) | 39.000 (23.000–66.000) | 37.000 (25.000–55.000) | 0.31 | 41.000 (23.000–69.000) | 35.000 (26.250–51.500) | 0.18 | |
Aspartic aminotransferase (U/L) | 77.000 (40.000–284.000) | 87.000 (38.000–183.000) | 0.29 | 83.000 (43.000–284.000) | 72.500 (37.750–149.250) | 0.06 | |
Alkaline phosphatase (mmol/L) | 78.000 (62.000–90.000) | 73.000 (60.000–89.000) | 0.17 | 77.000 (61.000–89.000) | 75.000 (61.000–92.000) | 0.98 | |
Creatinine (μmol/L) | 106.000 (81.250–170.250) | 73.000 (62.000–87.000) | <0.001 | 101.000 (80.250–161.750) | 79.000 (65.000–108.000) | <0.001 | |
Urea nitrogen (mmol/L) | 9.700 (6.700–14.700) | 5.900 (4.800–7.500) | <0.001 | 9.200 (6.500–12.800) | 6.750 (5.600–9.750) | <0.001 | |
Uric acid (μmol/L) | 413.000 (353.000–531.000) | 351.000 (293.000–418.500) | <0.001 | 413.000 (354.000–531.000) | 349.500 (277.000–428.750) | <0.001 | |
Triglyceride (mmol/L) | 1.125 (0.840–1.653) | 1.440 (1.030–2.100) | <0.001 | 1.70 (0.850–1.670) | 1.210 (0.960–2.000) | 0.054 | |
Total cholesterol (mmol/L) | 4.540 (3.973–5.255) | 4.760 (4.023–5.510) | 0.14 | 4.550 (3.980–5.280) | 4.480 (3.620–5.670) | 0.69 | |
High-density lipoprotein (mmol/L) | 1.090 (0.870–1.300) | 1.010 (0.840–1.220) | 0.10 | 1.090 (0.880–1.270) | 1.080 (0.860–1.290) | 0.82 | |
Low-density lipoprotein (mmol/L) | 2.705 (2.178–3.523) | 3.010 (2.360–3.600) | 0.04 | 2.770 (2.180–3.560) | 2.840 (2.170–3.550) | 0.64 | |
Apolipoprotein A1 (mmol/L) | 0.940 (0.810–1.060) | 0.970 (0.880–1.070) | 0.04 | 0.940 (0.810–1.050) | 0.890 (0.810–1.010) | 0.33 | |
Apolipoprotein B (mmol/L) | 0.800 (0.650–0.990) | 0.860 (0.700–1.030) | 0.04 | 0.820 (0.660–1.000) | 0.890 (0.670–1.120) | 0.09 | |
Apolipoprotein E (mmol/L) | 4.090 (3.325–5.205) | 4.020 (3.320–4.903) | 0.66 | 4.120 (3.360–5.300) | 3.630 (2.980–5.010) | 0.10 | |
Lipoprotein α (mmol/L) | 16.500 (8.650–31.450) | 14.900 (7.500–28.200) | 0.22 | 16.700 (8.900–31.600) | 12.100 (6.700–22.100) | 0.02 | |
Prothrombin time (s) | 13.550 (12.050–16.150) | 13.500 (11.700–15.600) | 0.13 | 13.500 (11.925–16.175) | 13.550 (11.800–16.800) | 0.94 | |
International normalized ratio | 1.180 (1.050–1.390) | 1.180 (1.018–1.353) | 0.12 | 1.180 (1.040–1.360) | 1.180 (1.030–1.438) | 0.75 | |
Activated partial thromboplastin (s) | 34.750 (29.680–99.430) | 47.600 (27.800–140.000) | 0.26 | 34.700 (30.000–94.000) | 39.200 (29.200–131.350) | 0.44 | |
Thrombin time (s) | 17.300 (16.330–19.480) | 18.500 (16.900–110.000) | 0.001 | 17.200 (16.400–19.500) | 18.900 (17.730–74.900) | <0.001 | |
Fibrinogen (g/L) | 3.604 (2.684–4.375) | 2.708 (2.353–3.144) | <0.001 | 3.587 (2.677–4.260) | 2.804 (2.324–3.194) | <0.001 | |
D-dimer (mg/L) | 0.951 (0.496–3.118) | 0.350 (0.200–0.698) | <0.001 | 1.065 (0.495–3.235) | 0.440 (0.223–0.885) | <0.001 | |
C-reactive protein (mg/L) | 16.330 (3.230–64.240) | 3.570 (2.500–9.380) | <0.001 | 13.800 (2.670–64.240) | 3.930 (2.500–11.530) | <0.001 | |
Procalcitonin (ng/mL) | 0.130 (0.060–0.540) | 0.050 (0.050–0.070) | <0.001 | 0.120 (0.050–0.450) | 0.050 (0.040–0.090) | <0.001 |
Continuous variables are presented as median with interquartile ranges for data with non-normal distributions.
Comparison of myocardial enzyme profile, heart failure markers, and ejection fraction (EF) before and after propensity score matching
Before propensity score matching, B-type natriuretic peptide precursor (pro-BNP), brain natriuretic peptide (BNP), lactate dehydrogenase, myoglobin, and EF were remarkably different between both groups. After propensity score matching, all these parameters were still significantly different between the two groups (P<0.05). The levels of pro-BNP, BNP, lactate dehydrogenase, and myoglobin were significantly higher in the case group than in the control group, while the EF value showed an opposite trend. The general statistics of the myocardial enzyme profile, heart failure markers, and EF of the two groups are shown in Table 3.
Table 3
Variables | Unmatched analysis | Propensity score matched analysis | |||||
---|---|---|---|---|---|---|---|
Death (n=130) | Survival (n=929) | P value | Death (n=116) | Survival (n=116) | P value | ||
Troponin I (μg/L) | 29.390 (6.770–80.000) | 22.430 (6.030–71.180) | 0.18 | 27.360 (6.580–78.760) | 16.570 (4.390–41.810) | 0.02 | |
B-type natriuretic peptide (pg/mL) | 725.500 (251.500–1,851.000) |
134.000 (44.250–362.000) |
<0.001 | 625.000 (157.500–1,586.250) |
238.000 (119.000–538.000) |
<0.001 | |
B-type natriuretic peptide precursor (pg/mL) | 9,905.000 (3,284.000–22,034.000) |
1,198.500 (528.150–2,587.000) |
<0.001 | 8,644.000 (3,035.000–15,824.000) |
1,978.090 (803.170–3,912.250) |
<0.001 | |
Lactate dehydrogenase (U/L) | 693.500 (444.500–1,158.250) |
554.500 (355.000–803.000) |
<0.001 | 713.500 (430.500–1,158.250) |
564.000 (372.000–763.250) |
0.006 | |
Creatine kinase (U/L) | 1,101.000 (340.250–2,948.750) |
1,281.500 (404.500–2,587.000) |
0.79 | 1,186.000 (373.500–3,086.000) |
1,289.000 (402.500–2,566.250) |
0.67 | |
Creatine kinase isoenzyme (U/L) | 83.250 (32.175–223.025) |
115.750 (28.900–240.475) |
0.57 | 87.350 (32.175–223.025) |
105.550 (26.600–228.300) |
0.87 | |
Myoglobin (μg/L) | 383.500 (145.750–1,227.500) |
235.600 (49.100–1,147.350) |
0.002 | 400.200 (137.000–1,212.000) |
254.000 (64.900–889.400) |
0.03 | |
Ejection fraction (%) | 50.000 (42.000–55.500) |
58.000 (52.000–62.000) |
<0.001 | 50.000 (42.000–56.000) |
58.000 (53.000–62.000) |
<0.001 |
Continuous variables are presented as median with interquartile ranges for data with non-normal distributions.
HR for MI-related death using Cox proportional hazard regression analysis
Cox regression analysis was performed on all parameters that were dramatically different by univariate analysis between the two groups after matching. Risk factors with a significant difference for MI-related death included PDW (HR =1.210, 95% CI: 1.080–1.356, P=0.001), fibrinogen (HR =1.218, 95% CI: 1.027–1.444, P=0.02), and EF (HR =0.954, 95% CI: 0.929–0.981, P=0.001) (shown in Table 4).
Table 4
Variables | B value | SE | Hazard ratio | 95% CI | P value |
---|---|---|---|---|---|
Platelet distribution width | 0.191 | 0.058 | 1.210 | 1.080–1.356 | 0.001 |
Fibrinogen | 0.197 | 0.087 | 1.218 | 1.027–1.444 | 0.02 |
Ejection fraction | −0.047 | 0.014 | 0.954 | 0.929–0.981 | 0.001 |
HRs, hazard ratios; MI, myocardial infarction; SE, standard error; CI, confidence interval.
ROC analysis of statistically significant parameters to predict MI-related death
PDW, fibrinogen, and EF value were analyzed by ROC curve to assess the predictive value of those parameters for MI-related death. The area under the curve (AUC) of the PDW was 0.604 (95% CI: 0.531–0.677, P=0.007), the optimal threshold of the PDW value was 13.05%, the AUC of fibrinogen was 0.684 (95% CI: 0.614–0.754, P<0.001), the optimal threshold of the fibrinogen level was 3.562 g/L, the AUC of the EF value was 0.734 (95% CI: 0.663–0.805, P<0.001), and the optimal threshold of the EF value was 55.5% (shown in Table 5, Figure 1).
Table 5
Variables | ROC-AUC (95% CI) | P value | Cut-off (sensitivity, specificity) |
---|---|---|---|
Platelet distribution width (%) | 0.604 (0.531–0.677) | 0.007 | 13.05 (0.561, 0.635) |
Fibrinogen (g/L) | 0.684 (0.614–0.754) | <0.001 | 3.562 (0.518, 0.843) |
Ejection fraction (%) | 0.734 (0.663–0.805) | <0.001 | 55.5 (0.747, 0.664) |
ROC, receiver operating characteristic; MI, myocardial infarction; AUC, area under the curve; CI, confidence interval.
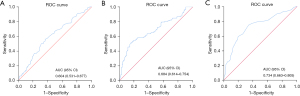
Kaplan-Meier curve was performed to compare the proportion of survived patients with low (below cut-off) and high (above cut-off) PDW and fibrinogen having survival during follow-up
By Kaplan-Meier analysis, the rate of patients who had a PDW ≥13.05% showed a higher MI-related death throughout the follow-up period than those who had a PDW <13.05% (shown in Figure 2). Similarly, Kaplan-Meier analysis showed that patients with fibrinogen ≥3.562 g/L had a higher mortality associated with MI-related death than those with <3.562 g/L (shown in Figure 3).
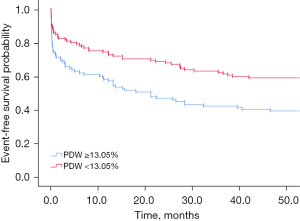
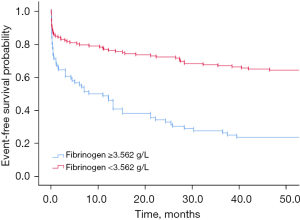
Discussion
In this study, after mitigating the influence of non-randomly selected bias and confounding variables as much as possible by propensity score matching, we demonstrated for the first time that PDW and fibrinogen were associated with the hard endpoint event death of AMI. Moreover, ROC analysis indicated that the AUC of PDW was 0.604, with 56.1% sensitivity and 63.5% specificity, and the AUC of fibrinogen was 0.684, with 51.8% sensitivity and 84.3% specificity. Therefore, we provide two new biochemical markers that are important for predicting the long-term hard endpoint of death post-MI.
Here, our results showed that inflammation-related markers such as C-reactive protein, white blood cell count (16), erythrocyte-related indicators such as red blood cell distribution width (17), partial coagulation-related indicators such as D-dimer (18), and some biochemical markers such as creatinine, uric acid, and lipoprotein α were all significantly increased in the MI-related death group (19-21), and the elevation of these biomarkers were all confirmed to be associated with long-term MACE post-MI in previous studies. In addition, the biomarkers related to myocardial injury post-MI, such as pro-BNP, BNP, and lactate dehydrogenase, were all significantly higher in the death group before and after propensity score matching, indicating that the more severe myocardial injury post-MI, the higher the probability of long-term death post-MI, which is consistent with previous studies (22,23). Moreover, cardiac ultrasound results demonstrated that the worse EF value of patients post-MI, the higher risk of long-term hard endpoint death, which is also consistent with the conclusions of a prior study (24). The previously confirmed risk biomarkers were retained in the current study, which in turn proves the reliability of the current study.
All biomarkers that differed between the two groups after propensity score matching were included in the Cox regression model, suggesting that PDW and fibrinogen were two independent risk factors for predicting the hard endpoint of death post-MI. Among them, for every 1% increase in the PDW, the hard endpoint event of death post-MI increased by 1.21 times, and for every 1 g/L increase in fibrinogen, the hard endpoint event of death post-MI increased by 1.218 times.
The formation process of AMI is plaque rupture in the coronary vessels, which allows contact with platelets to form a thrombus and leads to coronary artery occlusion. Platelet activation and the active substance released by those platelets play an important role in the pathogenesis of AMI (25,26). Larger platelets with higher pro-thrombotic status lead to increasing platelet activity and release more active substances such as thromboxane A2 glycoprotein IIb–IIIa receptor (27,28). PDW is not influenced by platelet swelling in vitro, so it better reflects the volume and function of platelets (29). Higher PDW value refers to stronger platelet activation and more active substances of platelets are released, leading to a higher risk of thrombosis (30). Therefore, many studies have suggested that PDW is associated with the occurrence of AMI. In addition, PDW was associated with Gensini scores, suggesting a correlation with severity in acute coronary syndrome (ACS) patients (31). In our study, PDW was found to be an independent predictor of the hard endpoint of death post-MI, which may correlate with the strength of thrombosis in patients with AMI and the severity of AMI development. Currently, only a few studies have shown that PDW is related to long-term prognosis post-MI. A study has suggested that PDW is related to long-term MACE in ACS patients, but it has no predictive value for the long-term hard endpoint of death in ACS patients (32). This may be relevant to the study population included. In addition, prior research has pointed out that PDW is associated with death during hospitalization in MI patients (33). Furthermore, one study showed that PDW could predict one-year death in ACS population, but our study has a larger sample size and longer follow-up time, so our conclusion is more reliable and better to reflect PDW as a predictor of higher risk of long-term death in the Chinese AMI population (34). In summary, PDW may be one of the expected indicators of the hard endpoint of long-term death in AMI patients.
Fibrinogen is a precursor of fibrin, which is a key component of platelet cross-linking and blood clot formation and has a definite role in arterial thrombosis (35). Many previous studies have confirmed that fibrinogen is an important risk factor for AMI and stroke (36,37). However, the results of this study suggested that plasma fibrinogen was associated with long-term hard endpoint death events post-MI and was an important predictor. Previous studies have shown that plasma fibrinogen concentrations are associated with long-term mortality of CHD (38,39), but few studies have focused on fibrinogen concentration correlation with MACE post-MI. A recent study showed that plasma fibrinogen was associated with 6-month MACE in patients with ACS (40). In addition, research confirmed that fibrinogen concentration was a predictor biomarker for 2-year MACE in patients with STEMI (41). However, the patients selected in these studies are not consistent with that of our study, and the follow-up period of our study is longer. Although previous report has already shown that plasma fibrinogen levels are correlated with long-term prognosis in patients with previous MI at the 10-year follow-up study (42), the sample size in our research is significantly larger, and we also mitigate the influence of nonrandom selection bias and confounding variables through the propensity score matching method. And the samples selected in our study were all with opening of the criminal blood vessels, therefore the patient’s treatment strategies in our study are more in line with guideline recommendations. The conclusions of prior studies also show the reliability of the conclusion of our study, and to the best of knowledge, this is the first study to demonstrate the correlation between fibrinogen and the hard endpoint of long-term death post-MI in our Chinese population. In addition to thrombosis formation, fibrinogen can also exacerbate the inflammatory response post-MI (43). Moreover, when fibrinogen converts to fibrin, it can aggravate myocardial injury after reperfusion (43). Together, the elevated plasma fibrinogen in AMI patients may further promote the inflammatory response post-MI and reperfusion injury after opening criminal blood vessels, which may explain why fibrinogen is a predictor for the hard endpoint of long-term death post-MI.
In this study, the propensity score method is used to mitigate the impact of data bias and confounding factors between the two groups and reduce selection bias. However, due to the sample size, one covariate still had statistically significant differences between the two groups after propensity matching, but most covariates showed no significant differences after matching. The follow-up study will further confirm the conclusions of this study by increasing the sample size. In addition, this study only recorded the hard endpoint event of death post-MI, neglecting other MACE. The hard endpoint event post-MI was the most critical, but other MACE were also important to evaluate the long-term prognosis of patients post-MI. Similarly, the follow-up study will record all relevant MACE post-MI events to confirm the predictive value of relevant biomarkers found in this research.
Conclusions
Our study showed that increased levels of PDW and fibrinogen appeared to be associated with an increased risk of death post-MI, and these two biomarkers might be expected to predict the risk of death post-MI. Prospective, randomized controlled studies should be designed to further verify the correlation. Other traditional long-term predictive biomarkers post-MI were still retained in our study, suggesting that these traditional predictive indicators should not be ignored.
Acknowledgments
Funding: This research was funded by
Footnote
Reporting Checklist: The authors have completed the STARD reporting checklist. Available at https://jtd.amegroups.com/article/view/10.21037/jtd-24-204/rc
Data Sharing Statement: Available at https://jtd.amegroups.com/article/view/10.21037/jtd-24-204/dss
Peer Review File: Available at https://jtd.amegroups.com/article/view/10.21037/jtd-24-204/prf
Conflicts of Interest: All authors have completed the ICMJE uniform disclosure form (available at https://jtd.amegroups.com/article/view/10.21037/jtd-24-204/coif). The authors have no conflicts of interest to declare.
Ethical Statement: The authors are accountable for all aspects of the work in ensuring that questions related to the accuracy or integrity of any part of the work are appropriately investigated and resolved. The study was conducted in accordance with the Declaration of Helsinki (as revised in 2013). The study was approved by the Ethics Committee of Shanghai Sixth People’s Hospital Affiliated to Shanghai Jiao Tong University School of Medicine [No. 2021-KY-034(K)-(1)] and individual consent for this retrospective analysis was waived.
Open Access Statement: This is an Open Access article distributed in accordance with the Creative Commons Attribution-NonCommercial-NoDerivs 4.0 International License (CC BY-NC-ND 4.0), which permits the non-commercial replication and distribution of the article with the strict proviso that no changes or edits are made and the original work is properly cited (including links to both the formal publication through the relevant DOI and the license). See: https://creativecommons.org/licenses/by-nc-nd/4.0/.
References
- Schwalm JD, McKee M, Huffman MD, et al. Resource Effective Strategies to Prevent and Treat Cardiovascular Disease. Circulation 2016;133:742-55. [Crossref] [PubMed]
- Lin X, Green JC, Xian H, et al. Holiday and weekend effects on mortality for acute myocardial infarction in Shanxi, China: a cross-sectional study. Int J Public Health 2020;65:847-57. [Crossref] [PubMed]
- Pollard TJ. The acute myocardial infarction. Prim Care 2000;27:631-49. vi. [Crossref] [PubMed]
- Byrne RA, Rossello X, Coughlan JJ, et al. 2023 ESC Guidelines for the management of acute coronary syndromes. Eur Heart J 2023;44:3720-826. [Crossref] [PubMed]
- Mandelzweig L, Battler A, Boyko V, et al. The second Euro Heart Survey on acute coronary syndromes: Characteristics, treatment, and outcome of patients with ACS in Europe and the Mediterranean Basin in 2004. Eur Heart J 2006;27:2285-93. [Crossref] [PubMed]
- Groenland FTW, Neleman T, Kakar H, et al. Intravascular ultrasound-guided versus coronary angiography-guided percutaneous coronary intervention in patients with acute myocardial infarction: A systematic review and meta-analysis. Int J Cardiol 2022;353:35-42. [Crossref] [PubMed]
- Gouda P, Savu A, Bainey KR, et al. Long-term risk of death and recurrent cardiovascular events following acute coronary syndromes. PLoS One 2021;16:e0254008. [Crossref] [PubMed]
- Boggon R, Timmis A, Hemingway H, et al. Smoking cessation interventions following acute coronary syndrome: a missed opportunity? Eur J Prev Cardiol 2014;21:767-73. [Crossref] [PubMed]
- Sullivan S, Young A, Garcia M, et al. Sex differences in vascular response to mental stress and adverse cardiovascular events among patients with ischemic heart disease. Arterioscler Thromb Vasc Biol 2023;43:e112-e120. [Crossref] [PubMed]
- Liu C, Zhao Z, Fan J, et al. Quantification of circulating Mycobacterium tuberculosis antigen peptides allows rapid diagnosis of active disease and treatment monitoring. Proc Natl Acad Sci U S A 2017;114:3969-74. [Crossref] [PubMed]
- Ngo TTM, Moufarrej MN, Rasmussen MH, et al. Noninvasive blood tests for fetal development predict gestational age and preterm delivery. Science 2018;360:1133-6. [Crossref] [PubMed]
- Xu W, Wang L, Zhang R, et al. Diagnosis and prognosis of myocardial infarction on a plasmonic chip. Nat Commun 2020;11:1654. [Crossref] [PubMed]
- Deng XN, Wang XY, Yu HY, et al. Admission macrophage migration inhibitory factor predicts long-term prognosis in patients with ST-elevation myocardial infarction. Eur Heart J Qual Care Clin Outcomes 2018;4:208-19. [PubMed]
- Li R, Jin J, Liu E, et al. A novel circulating biomarker lnc-MALAT1 for acute myocardial infarction: Its relationship with disease risk, features, cytokines, and major adverse cardiovascular events. J Clin Lab Anal 2022;36:e24771. [Crossref] [PubMed]
- Sardu C, D'Onofrio N, Torella M, et al. Pericoronary fat inflammation and Major Adverse Cardiac Events (MACE) in prediabetic patients with acute myocardial infarction: effects of metformin. Cardiovasc Diabetol 2019;18:126. [Crossref] [PubMed]
- Fiechter M, Ghadri JR, Jaguszewski M, et al. Impact of inflammation on adverse cardiovascular events in patients with acute coronary syndromes. J Cardiovasc Med (Hagerstown) 2013;14:807-14. [Crossref] [PubMed]
- Dabbah S, Hammerman H, Markiewicz W, et al. Relation between red cell distribution width and clinical outcomes after acute myocardial infarction. Am J Cardiol 2010;105:312-7. [Crossref] [PubMed]
- Kikkert WJ, Claessen BE, Stone GW, et al. D-dimer levels predict ischemic and hemorrhagic outcomes after acute myocardial infarction: a HORIZONS-AMI biomarker substudy. J Thromb Thrombolysis 2014;37:155-64. [Crossref] [PubMed]
- Uluganyan M, Karaca G, Ulutas TK, et al. The Impact of Admission Serum Creatinine Derived Estimated Glomerular Filtration Rate on Major Adverse Cardiac Events in ST-Segment Elevation Myocardial Infarction Patients Undergoing Primary Percutaneous Coronary Intervention. J Clin Med Res 2016;8:325-30. [Crossref] [PubMed]
- von Lueder TG, Girerd N, Atar D, et al. Serum uric acid is associated with mortality and heart failure hospitalizations in patients with complicated myocardial infarction: findings from the High-Risk Myocardial Infarction Database Initiative. Eur J Heart Fail 2015;17:1144-51. [Crossref] [PubMed]
- Bittner VA, Szarek M, Aylward PE, et al. Effect of Alirocumab on Lipoprotein(a) and Cardiovascular Risk After Acute Coronary Syndrome. J Am Coll Cardiol 2020;75:133-44. [Crossref] [PubMed]
- Li N, Chen R, Li J, et al. Prognostic significance of serial N-terminal pro-B-type natriuretic peptide levels in patients with acute myocardial infarction: A prospective study. Am Heart J 2023;262:90-9. [Crossref] [PubMed]
- Wei S, Mao L, Liu B, et al. Serum biomarkers and the prognosis of AMI patients. Herz 2014;39:384-9. [Crossref] [PubMed]
- Halkin A, Stone GW, Dixon SR, et al. Impact and determinants of left ventricular function in patients undergoing primary percutaneous coronary intervention in acute myocardial infarction. Am J Cardiol 2005;96:325-31. [Crossref] [PubMed]
- Coppinger JA, Cagney G, Toomey S, et al. Characterization of the proteins released from activated platelets leads to localization of novel platelet proteins in human atherosclerotic lesions. Blood 2004;103:2096-104. [Crossref] [PubMed]
- Gawaz M, Langer H, May AE. Platelets in inflammation and atherogenesis. J Clin Invest 2005;115:3378-84. [Crossref] [PubMed]
- Kamath S, Blann AD, Lip GY. Platelet activation: assessment and quantification. Eur Heart J 2001;22:1561-71. [Crossref] [PubMed]
- Giles H, Smith RE, Martin JF. Platelet glycoprotein IIb-IIIa and size are increased in acute myocardial infarction. Eur J Clin Invest 1994;24:69-72. [Crossref] [PubMed]
- Vagdatli E, Gounari E, Lazaridou E, et al. Platelet distribution width: a simple, practical and specific marker of activation of coagulation. Hippokratia 2010;14:28-32. [PubMed]
- Verdoia M, Barbieri L, Schaffer A, et al. Platelet distribution width and the risk of periprocedural myocardial infarction in patients undergoing percutaneous coronary intervention. J Thromb Thrombolysis 2014;37:345-52. [Crossref] [PubMed]
- Bekler A, Ozkan MT, Tenekecioglu E, et al. Increased Platelet Distribution Width Is Associated With Severity of Coronary Artery Disease in Patients With Acute Coronary Syndrome. Angiology 2015;66:638-43. [Crossref] [PubMed]
- Ulucan Ş, Keser A, Kaya Z, et al. Association between PDW and Long Term Major Adverse Cardiac Events in Patients with Acute Coronary Syndrome. Heart Lung Circ 2016;25:29-34. [Crossref] [PubMed]
- Bae MH, Lee JH, Yang DH, et al. White blood cell, hemoglobin and platelet distribution width as short-term prognostic markers in patients with acute myocardial infarction. J Korean Med Sci 2014;29:519-26. [Crossref] [PubMed]
- Timóteo AT, Papoila AL, Lousinha A, et al. Predictive impact on medium-term mortality of hematological parameters in Acute Coronary Syndromes: added value on top of GRACE risk score. Eur Heart J Acute Cardiovasc Care 2015;4:172-9. [Crossref] [PubMed]
- Ang L, Mahmud E. Monitoring oral antiplatelet therapy: is it justified? Ther Adv Cardiovasc Dis 2008;2:485-96. [Crossref] [PubMed]
- Maresca G, Di Blasio A, Marchioli R, et al. Measuring plasma fibrinogen to predict stroke and myocardial infarction: an update. Arterioscler Thromb Vasc Biol 1999;19:1368-77. [Crossref] [PubMed]
- Wilhelmsen L, Svärdsudd K, Korsan-Bengtsen K, et al. Fibrinogen as a risk factor for stroke and myocardial infarction. N Engl J Med 1984;311:501-5. [Crossref] [PubMed]
- Arnau Vives MA, Rueda Soriano J, Martínez Dolz LV, et al. Prognostic value of fibrinogen in patients admitted with suspected unstable angina and non-q-wave myocardial infarction. Rev Esp Cardiol 2002;55:622-30. [Crossref] [PubMed]
- Fibrinogen Studies Collaboration. Plasma fibrinogen level and the risk of major cardiovascular diseases and nonvascular mortality: an individual participant meta-analysis. JAMA 2005;294:1799-809. [PubMed]
- Mahmud E, Ramsis M, Behnamfar O, et al. Effect of Serum Fibrinogen, Total Stent Length, and Type of Acute Coronary Syndrome on 6-Month Major Adverse Cardiovascular Events and Bleeding After Percutaneous Coronary Intervention. Am J Cardiol 2016;117:1575-81. [Crossref] [PubMed]
- Ang L, Behnamfar O, Palakodeti S, et al. Elevated Baseline Serum Fibrinogen: Effect on 2-Year Major Adverse Cardiovascular Events Following Percutaneous Coronary Intervention. J Am Heart Assoc 2017;6:e006580. [Crossref] [PubMed]
- Retterstol L, Kierulf P, Pedersen JC, et al. Plasma fibrinogen level and long-term prognosis in Norwegian middle-aged patients with previous myocardial infarction. A 10 year follow-up study. J Intern Med 2001;249:511-8. [Crossref] [PubMed]
- Zacharowski K, Zacharowski P, Reingruber S, et al. Fibrin(ogen) and its fragments in the pathophysiology and treatment of myocardial infarction. J Mol Med (Berl) 2006;84:469-77. [Crossref] [PubMed]