The consistently up-regulated expression of NLRP3 in severe asthma patients from mRNA microarray and ovalbumin-induced mouse model of asthma
Highlight box
Key findings
• Through bioinformatics analysis, a plethora of differentially expressed genes (DEGs) have been identified, potentially influencing the development of severe asthma (SA). Notably, the investigation highlights the nucleotide-binding oligomerization domain-like receptor family pyrin domain containing 3 (NLRP3) as a potential key player in asthma pathogenesis, suggesting its prospective utility as a biomarker for SA.
What is known and what is new?
• Bioinformatics analysis has been employed to identify DEGs associated with asthma.
• The current study presents a comprehensive analysis of DEGs related to SA using bioinformatics methods. This study highlights NLRP3 as a potentially pivotal player in asthma pathogenesis, indicating its prospective utility as a biomarker specifically for SA.
What is the implication, and what should change now?
• The identification of NLRP3 as a potential pivotal player in asthma pathogenesis suggests a new avenue for research and therapeutic interventions targeting SA.
• Researchers and clinicians should prioritize further investigation into the role of NLRP3 in asthma pathogenesis, including experimental validation of its function and potential as a biomarker.
Introduction
Asthma is one of the most common chronic respiratory diseases in the world (1,2), with 358 million asthma patients worldwide, and the prevalence of asthma increased by 12.6% from 1900 to 2019 (3). Severe asthma (SA) is defined as “asthma that is uncontrolled, despite adherence with maximal optimized high-dose inhaled corticosteroids with long-acting beta-agonist (LABA) treatment and management of contributory factors, or that requires high-dose treatment to maintain good symptom control and reduce the risk of exacerbations” (4). Only 5% of the asthma patient population is diagnosed with SA, yet 50% of the healthcare system’s asthma treatment cost burden attributes to the treatment of SA (5). Uncontrolled exacerbation of symptoms often occurs in SA patients, such as episodic expiratory dyspnea and wheezing, along with a decline in lung function and excessive immune responses (6).
The lungs’ innate immune responses are set in motion when pathogens, allergens and other irritants activate extracellular and intracellular pattern recognition receptors (PRRs) (7,8). The nucleotide-binding oligomerization domain-like receptor family pyrin domain containing 3 (NLRP3), an intracellular PRR, plays a crucial role in recognizing microbial motifs, endogenous danger signals, and environmental irritants. This recognition leads to the formation and activation of the NLRP3 inflammasome. The NLRP3 inflammasome formation results in caspase 1-dependent release of the pro-inflammatory cytokines IL-1β and IL-18, along with the occurrence of pyroptosis (8).
Overactivation of the NLRP3 inflammasome can result in excessive inflammation, tissue damage, and the development of chronic inflammatory diseases (9,10). Study using NLRP3-deficient mice in an ovalbumin (OVA)-induced allergic airway inflammation model has shown a reduction in eosinophil influx, airway hyperreactivity, inflammation, and goblet cell accumulation (11). This is accompanied by reduced IL-1β expression in the airway compared to wild-type littermates, indicating the involvement of NLRP3 in asthma pathogenesis (12).
The mechanisms underlying SA in clinical patients remain unclear. However, in-depth exploration of big data holds the potential to lay the groundwork for future research. This study aimed to identify genes relevant to SA pathogenesis by comparing mRNA microarray datasets of SA patients and healthy controls from Gene Expression Omnibus (GEO) (13). Following the identification of NLRP3 as a likely relevant gene, we conducted immunohistochemistry (IHC), quantitative reverse transcription polymerase chain reaction (qRT-PCR) and Western Blot studies on lung tissues from OVA-induced model mice to confirm the relevance of NLRP3 in SA. We present this article in accordance with the ARRIVE reporting checklist (available at https://jtd.amegroups.com/article/view/10.21037/jtd-24-567/rc).
Methods
Acquisition of RNA information
GEO (http://www.ncbi.nlm.nih.gov/geo) is an open repository for functional genomics data, encompassing gene expression data, chips, and microarrays. In this study, the gene expression dataset (GSE76262) was retrieved from GEO, specifically utilizing the Affymetrix GPL570 platform and the Affymetrix Human Genome U133 Plus 2.0 Array (14). Probes were transformed into the corresponding gene symbols based on the platform’s annotation information. The GSE7626 dataset comprises 93 induced sputum samples from patients with SA and 21 samples from healthy controls (15). The study was conducted in accordance with the Declaration of Helsinki (as revised in 2013).
Finding differentially expressed genes (DEGs)
DEGs between SA and healthy control samples were identified using GEO2R (http://www.ncbi.nlm.nih.gov/geo/geo2r). GEO2R is an interactive web tool that enables users to compare two or more datasets within a GEO series to identify DEGs across experimental conditions. The adjusted P value (Padj) and the Benjamini and Hochberg false discovery rate were employed to strike a balance between the discovery of statistically significant genes and the control of false-positives. The criteria used for screening DEGs were as follows: an Padj<0.05 and a log2fold change (FC) ≥1 (15).
Gene Ontology (GO) and Kyoto Encyclopedia of Genes and Genomes (KEGG) enrichment analyses of DEGs
To delve deeper into the potential mechanisms of the DEGs, we utilized the Bioconductor package and Cluster Profiler package in the R language (16). This facilitated GO and KEGG pathway analyses on the target genes identified in the previous step. The GO analysis encompasses the cellular component (CC), molecular function (MF), and biological process (BP) of the potential target genes.
Gene cluster identification and protein-protein interaction (PPI) network analysis
PPI network was constructed using the Search Tool for the Retrieval of Interacting Genes (STRING; http://string-db.org) online database, version 11.0. Analyzing functional interactions between proteins can provide insights into the mechanisms underlying the generation or development of diseases. In this study, the PPI network of DEGs was established using the STRING database, considering interactions with a combined score greater than 0.4 as statistically significant. Cytoscape (version 3.8.1) was employed to visualize molecular interaction networks. The Molecular Complex Detection (MCODE) plugin (version 1.4.2) in Cytoscape was utilized to identify densely connected regions. The selection criteria were as follows: degree cut-off =2, node score cut-off =0.2, Max depth =100 and k-score =2. The detailed methods are provided in a previous study (17).
Animal model and ethics statements
Specific-pathogen-free Female C57BL/6 mice, aged 6–8 weeks and weighing 18–20 g, were procured. Forty mice were randomly allocated into two groups (n=20 per group): a control group and an OVA group (OVA, chicken egg albumin, grade V; Sigma, St. Louis, MO, USA). Sensitization and airway challenge procedures are detailed in our previous report (18). Briefly, OVA-induced asthma mice were sensitized to OVA plus Al (OH) via intraperitoneal injection (1, 7, 14 d), and then challenged by intranasal administration (24, 25 d) plus aerosol inhalation (26–28 d) with OVA solution orderly. Normal saline (NS) was used in the control group. The mouse procedures outlined in this study received approval from the Research Ethics Committee of Guangdong Provincial People’s Hospital, Guangdong Academy of Medical Sciences (approval No. GDREC2019219A). All procedures were conducted following the Guide for the Care and Use of Laboratory Animals. A protocol was prepared before the study without registration.
RNA extraction and qRT-PCR experiment
Lung tissues were processed by means of a syringe plunger and stainless-steel filter mesh to obtain intrapulmonary airways carefully. Total RNA extraction was carried out following the TRIzol protocol (Ambion, Austin, TX, USA), and subsequently, reverse transcription into cDNA was performed using the PrimeScriptTM RT Master Mix (Takara, Kusatsu, Japan). Real-time PCR was conducted using TB Green Premix Ex TaqTM II (Takara) on a Roche Light Cycler 480II system. The normalization of target gene expression was performed relative to GADPH using the 2−ΔΔCt method (19).
Western blot analysis
Radio immunoprecipitation assay (RIPA) lysis buffer (P0013C, Beyotime, Shanghai, China) was used to extract total protein from the tissues. 10% SDS-PAGE gels were used to separate the tissue lysates, transferred to a polyvinylidene fluoride (PVDF) membrane, and then probed with anti-NLRP3 (ab263899, Abcam, Cambridge, UK; 1:1,000) and anti-glyceraldehyde-3-phosphate dehydrogenase (GAPDH) (abs132004, Absin, 1:1,000) antibodies, followed by incubation with secondary antibodies (CAT#7074, CST, 1:2,500). The proteins were ultimately detected using the Chemiluminescent HRP Substrate (CAT#0100, Millipore, Burlington, VT, USA) (20).
IHC
Murine lungs were fixed in 4% paraformaldehyde (Sigma) for 24 hours. Subsequently, the tissues were paraffin-embedded (Sigma) and sectioned into 5-µm slices. For immunohistochemical staining, lung tissues were subjected to incubation with a primary antibody against NLRP3 (1:100; Abcam), as described in a previous report (21). Each specimen was independently scored by two observers and a histopathologist.
Statistical analyses
Data dispersion was expressed using the mean and standard deviation (SD). Statistical analysis for the data was conducted using SPSS 25.0 software. A two-tailed Student’s t-test was applied to compare two independent groups. A significance level of P<0.05 was considered statistically significant (22).
Results
Analysis of differential expression genes
After analyzing the data consistency and standardizing the results, 545 genes in SA patients were expressed significantly differently compared to healthy controls, with 172 upregulated and 373 downregulated. The volcano plot (Figure 1A), the principal component analysis (Figure 1B), heat map (Figure 1C) and table available at https://cdn.amegroups.cn/static/public/JTD-24-567-1.xlsx displays the results of this analysis. Table 1 illustrates the distribution of DEGs.
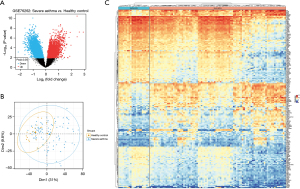
Table 1
Gene symbol | Padj value | t | b | Log2FC |
---|---|---|---|---|
Upregulated | ||||
IL1RL1 | <0.001 | 5.79 | 8.00 | 2.93 |
CLC | <0.001 | 5.80 | 8.04 | 2.62 |
IL18R1 | <0.001 | 7.34 | 15.06 | 2.45 |
PRSS33 | 0.002 | 4.35 | 2.29 | 1.97 |
LINC01366 | 0.002 | 4.49 | 2.77 | 1.66 |
EDN1 | <0.001 | 5.83 | 8.15 | 1.61 |
VEGFA | <0.001 | 5.62 | 7.27 | 1.57 |
IRAK3 | <0.001 | 5.21 | 5.56 | 1.5 |
NLRP3 | 0.001 | 4.40 | 2.44 | 1.35 |
FFAR3 | 0.004 | 3.92 | 0.77 | 1.31 |
Downregulated | ||||
EIF1AY | 0.009 | −3.47 | −0.62 | −2.14 |
SCD | 0.001 | −4.54 | 2.99 | −1.76 |
PPIC | 0.003 | −4.09 | 1.37 | −1.66 |
RPS4Y1 | 0.006 | −3.65 | −0.07 | −1.63 |
MYO5A | <0.001 | −5.18 | 5.44 | −1.62 |
TDRD3 | 0.005 | −3.77 | 0.30 | −1.58 |
SPARC | <0.001 | −4.68 | 3.49 | −1.53 |
HDDC2 | 0.002 | −4.41 | 2.49 | −1.5 |
SUCLG2 | <0.001 | −5.17 | 5.40 | −1.45 |
ADTRP | 0.002 | −4.30 | 2.08 | −1.4 |
With |log2FC| ≥1, false discovery rate <0.05, and Padj<0.05 in SA group compared to HC. FC, fold change; SA, severe asthma; HC, healthy control; Padj, adjusted P value.
KEGG and GO enrichment analyses of DEGs
Functional and pathway enrichment analyses were conducted using the clusterProfiler package to gain insights into the biological classification of DEGs. The results of GO analysis indicated that changes in BP of DEGs were significantly enriched in positive regulation of cytokine production, T cell activation, negative regulation of the immune system process, regulation of response to cytokine stimulus, and regulation of cytokine-mediated signaling pathway (Figure 2A). The CC of DEGs was primarily enriched in the external side of the plasma membrane, membrane microdomain, membrane raft, secretory granule membrane, and specific granule membrane (Figure 2B). Changes in MF were mainly enriched in enzyme inhibitor activity, cytokine binding, cytokine receptor activity, peptidase regulator activity, and endopeptidase inhibitor activity (Figure 2C). Furthermore, KEGG pathway analysis revealed that the up-regulated DEGs were predominantly enriched in the Pi3k-Akt, TNF, chemokine, cytokine-cytokine receptor interaction, and viral protein interaction with cytokine and cytokine receptor pathways (Figure 2D). Meanwhile, our analysis suggests NLRP3 involvement in pathways associated with pyroptosis, c-type lectin receptor signaling, and NOD-like receptor signaling (online tables available at https://cdn.amegroups.cn/static/public/JTD-24-567-2.xlsx and https://cdn.amegroups.cn/static/public/JTD-24-567-3.xlsx).
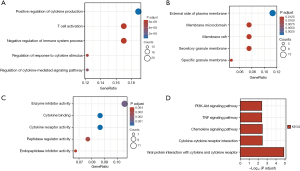
Construction of PPI network
We depicted the construction of the PPI network for DEGs in (Figure 3A), offering a clear visualization of the intricate relationships among these genes. Utilizing cytoscape, we identified the network’s most significant module, as depicted in (Figure 3B). Strategically selecting 21 DEGs as hub genes, based on their substantial degrees ≥10, highlighted their crucial roles within the network. Notably, NLRP3 emerged as the most highly connected, exhibiting three significant interactions.
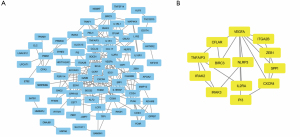
The expression of NLRP3 in mice
Timeline of animal experiments in (Figure 4). IHC was used to detect NLRP3 expression in OVA-induced asthma mice and the control group (Figure 5A). The intensity of NLRP3 expression was significantly higher in the OVA-induced asthma mice compared to the control group. Similarly, the mRNA expression of NLRP3 in OVA-induced asthma mice was also significantly higher than that in the control group (P=0.02, Figure 5B). Subsequently, the selected biomarker NLRP3 was validated in OVA-induced asthma lung samples using Western blot. The results demonstrated that the expression levels of NLRP3 in the lungs of OVA-induced mice were significantly higher than those in the control group (P=0.02, Figure 5C). Flow chart for the study (Figure 6).
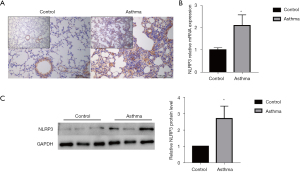
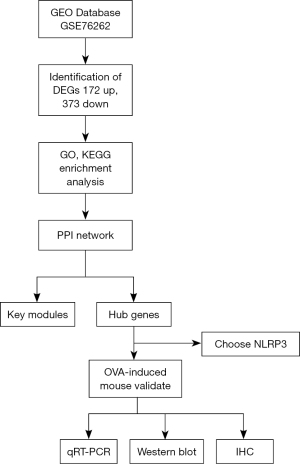
Discussion
A considerable body of research supports the utility of employing bioinformatics methods to screen DEGs and analyze known gene interaction networks and pathways. We have identified that NLRP3 is significantly overexpressed in SA patients and OVA-induced asthma mice, compared with healthy controls, which aligns with existing literature on the implications of NLRP3 in asthma pathogenesis (23). Notably, downregulation of NLRP3 through the administration of NLRP3 inhibitors in asthma models has been associated with constrained AHR and reduced pulmonary inflammation, suggesting a potential therapeutic avenue, indicating that NLRP3 may be associated with asthma aggressiveness (24,25).
To identify DEGs for severe cases, comparing SA vs. non-SA may be more appropriate, however, due to samples restricted by GSE76262 from GEO databases, it is difficult to conduct the above analysis. Then, according to the demographic and clinical characteristics of the patients described in the original article, we chose samples meeting the SA criteria to compare with healthy control.
In the context of innate immune responses, our analysis suggests the involvement of NLRP3 in pathways related to pyroptosis, c-type lectin receptor signaling, and NOD-like receptor signaling. These findings highlight potential mechanisms that may contribute to the progression of asthma. Research indicates that exposure to pathogens, allergens, cigarette smoke, and other noxious stimuli in the asthmatic airway can trigger the generation of reactive oxygen species (ROS), cytokines, and neutrophil extracellular traps (NETs) (4). This, in turn, activates the NLRP3 inflammasome in infiltrating eosinophils, neutrophils, macrophages, and airway epithelial cells. The heightened release of IL-1β and IL-18 follows, leading to increased infiltration of Th1, Th2, and/or Th17 cells. These events contribute to pathological consequences, including mucus hypersecretion, airway hyperresponsiveness (AHR), and airway remodeling (24).
To investigate complex and diverse syndromes like SA, it is essential to employ appropriate animal model systems. These models should not only facilitate in-depth mechanistic studies of SA pathogenesis but also serve as a platform for the preclinical assessment of various therapeutic drugs. In recent decades, numerous mouse models of SA have been created and validated. Proposed subtypes of SA comprise the following categories: viral infection exacerbated asthma; Aspergillus sp. infection exacerbated asthma; multiple allergen exposure exacerbated asthma; chronic allergen exposure exacerbated asthma; IFN-γ-dependent SA; non-allergic steroid resistant SA; high neutrophilic Th17-induced SA; high eosinophilic (Th2 high) SA; IL-13-mediated SA. Various induction protocols have been employed in these models, and most of them have undergone testing for steroid resistance (26).
OVA-induced asthma mice help to define the pathological mechanisms of SA (26-28). Our OVA-induced asthma mice model with lung tissue tested using qRT-PCR, western-blot and IHC methods approved parts of the above results. We observed an increase in NLRP3 expression in the lung tissues at both the mRNA and protein levels. These observations suggest a potential role for the NLRP3 inflammasome in the development of SA. More evidence should be provided to prove the role of NLRP3 in asthma except for its expression level, such as, lung RNA-seq results of OVA-induced mouse, hematoxylin and eosin (HE), periodic acid-Schiff (PAS), AHR, bronchoalveolar lavage fluid (BALF) cells, etc. In addition, another drawback is that we cannot be sure that the OVA-induced asthma mouse model that we used matched the clinical characteristics of patients with SA completely.
The ongoing advancement and utilization of improved in vivo models for SA, coupled with complementary human studies utilizing physiologically relevant in vitro models, are crucial for the development of personalized medicine. These efforts collectively aim to address the evolving needs of the increasing population of individuals with SA.
Conclusions
We employed mRNA microarray data from human SA patients, along with mRNA and protein analysis from the OVA-induced asthma mice model, to identify DEGs when compared with a healthy control. We reveal that the NLRP3 gene may play a crucial role in airway inflammation of SA which may help to further elucidate the potential biomarker for this condition.
Acknowledgments
We thank all the investigators for their excellent assistance in this clinical research.
Funding: The research was funded by
Footnote
Reporting Checklist: The authors have completed the ARRIVE reporting checklist. Available at https://jtd.amegroups.com/article/view/10.21037/jtd-24-567/rc
Peer Review File: Available at https://jtd.amegroups.com/article/view/10.21037/jtd-24-567/prf
Conflicts of Interest: All authors have completed the ICMJE uniform disclosure form (available at https://jtd.amegroups.com/article/view/10.21037/jtd-24-567/coif). The authors have no conflicts of interest to declare.
Ethical Statement:
Open Access Statement: This is an Open Access article distributed in accordance with the Creative Commons Attribution-NonCommercial-NoDerivs 4.0 International License (CC BY-NC-ND 4.0), which permits the non-commercial replication and distribution of the article with the strict proviso that no changes or edits are made and the original work is properly cited (including links to both the formal publication through the relevant DOI and the license). See: https://creativecommons.org/licenses/by-nc-nd/4.0/.
References
- Kirby T. Living with severe asthma since childhood. Lancet Respir Med 2021;9:950-1. [Crossref] [PubMed]
- Butler CC, Yu LM, Dorward J, et al. Doxycycline for community treatment of suspected COVID-19 in people at high risk of adverse outcomes in the UK (PRINCIPLE): a randomised, controlled, open-label, adaptive platform trial. Lancet Respir Med 2021;9:1010-20. [Crossref] [PubMed]
- Boulet LP, Reddel HK, Bateman E, et al. The Global Initiative for Asthma (GINA): 25 years later. Eur Respir J 2019;54:1900598. [Crossref] [PubMed]
- Porsbjerg C, Melén E, Lehtimäki L, et al. Asthma. Lancet 2023;401:858-73. [Crossref] [PubMed]
- Mosnaim G. Asthma in Adults. N Engl J Med 2023;389:1023-31. [Crossref] [PubMed]
- Huang K, Yang T, Xu J, et al. Prevalence, risk factors, and management of asthma in China: a national cross-sectional study. Lancet 2019;394:407-18. [Crossref] [PubMed]
- Medzhitov R, Janeway CA Jr. Innate immunity: the virtues of a nonclonal system of recognition. Cell 1997;91:295-8. [Crossref] [PubMed]
- Theofani E, Semitekolou M, Morianos I, et al. Targeting NLRP3 Inflammasome Activation in Severe Asthma. J Clin Med 2019;8:1615. [Crossref] [PubMed]
- Pinkerton JW, Kim RY, Robertson AAB, et al. Inflammasomes in the lung. Mol Immunol 2017;86:44-55. [Crossref] [PubMed]
- Rathinam VA, Fitzgerald KA. Inflammasome Complexes: Emerging Mechanisms and Effector Functions. Cell 2016;165:792-800. [Crossref] [PubMed]
- Besnard AG, Guillou N, Tschopp J, et al. NLRP3 inflammasome is required in murine asthma in the absence of aluminum adjuvant. Allergy 2011;66:1047-57. [Crossref] [PubMed]
- Qi X, Gurung P, Malireddi RK, et al. Critical role of caspase-8-mediated IL-1 signaling in promoting Th2 responses during asthma pathogenesis. Mucosal Immunol 2017;10:128-38. [Crossref] [PubMed]
- Hou Y, Zhang Q, Pang W, et al. YTHDC1-mediated augmentation of miR-30d in repressing pancreatic tumorigenesis via attenuation of RUNX1-induced transcriptional activation of Warburg effect. Cell Death Differ 2021;28:3105-24. [Crossref] [PubMed]
- Kuo CS, Pavlidis S, Loza M, et al. T-helper cell type 2 (Th2) and non-Th2 molecular phenotypes of asthma using sputum transcriptomics in U-BIOPRED. Eur Respir J 2017;49:1602135. [Crossref] [PubMed]
- Li L, Lei Q, Zhang S, et al. Screening and identification of key biomarkers in hepatocellular carcinoma: Evidence from bioinformatic analysis. Oncol Rep 2017;38:2607-18. [Crossref] [PubMed]
- Yu G, Wang LG, Han Y, et al. clusterProfiler: an R package for comparing biological themes among gene clusters. OMICS 2012;16:284-7. [Crossref] [PubMed]
- Chen D, Wu W, Yi L, et al. A Potential circRNA-miRNA-mRNA Regulatory Network in Asthmatic Airway Epithelial Cells Identified by Integrated Analysis of Microarray Datasets. Front Mol Biosci 2021;8:703307. [Crossref] [PubMed]
- Wu J, Xu J, Cai C, et al. Ag85B DNA vaccine suppresses airway inflammation in a murine model of asthma. Respir Res 2009;10:51. [Crossref] [PubMed]
- Dai R, Yu Y, Yan G, et al. Intratracheal administration of adipose derived mesenchymal stem cells alleviates chronic asthma in a mouse model. BMC Pulm Med 2018;18:131. [Crossref] [PubMed]
- Shumin Z, Luying Z, Senlin L, et al. Ambient particulate matter-associated autophagy alleviates pulmonary inflammation induced by Platanus pollen protein 3 (Pla3). Sci Total Environ 2021;758:143696. [Crossref] [PubMed]
- Venosa A, Cowman S, Katzen J, et al. Role of CCR2(+) Myeloid Cells in Inflammation Responses Driven by Expression of a Surfactant Protein-C Mutant in the Alveolar Epithelium. Front Immunol 2021;12:665818. [Crossref] [PubMed]
- Wang F, Li Z, Lyu FJ, et al. The therapeutic effect of stem cells from human exfoliated deciduous teeth on a rat model of tracheal fistula. Stem Cell Res Ther 2022;13:310. [Crossref] [PubMed]
- Hu J, Wang J, Li C, et al. Fructose-1,6-bisphosphatase aggravates oxidative stress-induced apoptosis in asthma by suppressing the Nrf2 pathway. J Cell Mol Med 2021;25:5001-14. [Crossref] [PubMed]
- Rossios C, Pavlidis S, Hoda U, et al. Sputum transcriptomics reveal upregulation of IL-1 receptor family members in patients with severe asthma. J Allergy Clin Immunol 2018;141:560-70. [Crossref] [PubMed]
- Vande Walle L, Lamkanfi M. Drugging the NLRP3 inflammasome: from signalling mechanisms to therapeutic targets. Nat Rev Drug Discov 2024;23:43-66. [Crossref] [PubMed]
- Gubernatorova EO, Namakanova OA, Tumanov AV, et al. Mouse models of severe asthma for evaluation of therapeutic cytokine targeting. Immunol Lett 2019;207:73-83. [Crossref] [PubMed]
- Hong JY, Chung Y, Steenrod J, et al. Macrophage activation state determines the response to rhinovirus infection in a mouse model of allergic asthma. Respir Res 2014;15:63. [Crossref] [PubMed]
- Ravanetti L, Dijkhuis A, Sabogal Pineros YS, et al. An early innate response underlies severe influenza-induced exacerbations of asthma in a novel steroid-insensitive and anti-IL-5-responsive mouse model. Allergy 2017;72:737-53. [Crossref] [PubMed]