Deep learning to predict long-term mortality from plain chest X-ray in patients referred for suspected coronary artery disease
Highlight box
Key findings
• Our deep learning chest X-ray (DL-CXR) risk score robustly predicts mortality [area under the curve (AUC): 0.793, 95% confidence interval: 0.759–0.827]. Including age & coronary artery disease (CAD) enhances prediction (AUC: 0.809). External validation confirms accuracy.
What is known and what is new?
• DL can produce long-term prognostic information from CXR. In patients with suspected angina referred for coronary angiography, our DL-CXR risk score predicts long-term outcome better than age and CAD status.
What is the implication, and what should change now?
• DL supported interpretation of CXR should be tested in different clinical settings possibly to optimize risk stratification and support prevention programs.
Introduction
The chest X-ray (CXR) is a first-line tool adopted on patients referred for suspicion of cardiac conditions and possibly the most common imaging test performed in the medical field (1,2). Most CXRs are reported as non-pathologic and are used to rule out a specific clinical suspicion mostly related to the lungs. However, CXRs carry a plethora of different information, often completely undiscovered and unreported, concerning the patient’s overall and specific health status (3). When identified, this information could help make the diagnosis at the time of referral, support risk stratification of future morbidity and mortality, and guide a chain of decisions concerning lifestyle changes, screening planning, and prevention strategies. Clinicians and radiologists evaluate many CXRs during their medical career but are rarely able to follow patients in their long-term clinical developments, and, for this reason, they cannot connect and articulate the multifaceted radiographic findings at time zero with events occurring at long-term follow-up.
Artificial intelligence (AI) has recently resurged interest in the essential role that non-invasive ubiquitous and affordable imaging modalities such as CXRs may play in diagnosing pathologies and eventually helping define outcomes at follow-up. Deep learning (DL) can detect information that has been overlooked by human observers and, consequently, could strengthen image interpretation across many clinical specialties (4). Apart from the emerging potential that AI is demonstrating for the enhancement and support of diagnosis at the time of patient referral, the use of AI to define a patient’s prognosis starting from simple medical images, offers even more fascinating, and challenging, perspectives. The hypothesis that a DL model can produce long-term prognostic information from CXR has already been confirmed within a lung and prostate screening program (5). In this manuscript, we present our experience with a DL algorithm we have specifically designed for predicting, from a single projection CXR, long-term mortality of patients referred for coronary angiography to rule out the presence of coronary artery disease (CAD). We present this article in accordance with the TRIPOD reporting checklist (available at https://jtd.amegroups.com/article/view/10.21037/jtd-24-322/rc).
Methods
Imaging and data curation
The patients’ cohort included in the present study has been already used by our group to test, train, and validate a deep convolutional neural network (DCNN) solution for detecting significant CAD based on chest radiographs (6).
Data of patients referred to our institution (Spedali Civili di Brescia, Brescia, Italy) for suspected angina were retrospectively analysed. Radiographs were performed using nine different radiographic machines. Exclusion criteria included: previous percutaneous coronary revascularization, cardiac surgery, cardiac electronic devices implantation, and images with low-quality acquisition.
One-projection CXRs were included in the study. We decided to exclude lateral radiographs because not present in the entire cohort. All images had been performed during the index admission. Images storage took place in the institutional Picture Archiving and Communication System (PACS).
Coronary angiography findings were produced by experienced interventional cardiologists, prospectively collected, and stored in an electronic database at the time of investigation. Severe CAD was confirmed when at least one visually estimated coronary diameter stenosis with severity of ≥70% for non-left main disease and ≥50% for left main disease was present (6). We only used physiological assessment with fractional flow reserve (FFR) and instantaneous wave-free ratio (iFR) in coronary lesions of uncertain significance.
Demographic and clinical information concerning the patient’s age, biological sex, body mass index (BMI), angina status at time of referral were also collected (7).
For every patient included in the cohort, demographic information contained in the hospital electronic medical records (EMR) was matched with the Italian National Census data, to document follow-up status (alive, dead, and eventual death date) in August 2022.
Patients had signed informed consent to use relevant clinical information for scientific purposes. All data have been anonymized, and the study protocol has been approved by the local scientific and ethical committee (Comitato Etico-Scientifico Brescia, Protocol No. NP 4817). The study was conducted in accordance with the Declaration of Helsinki (as revised in 2013).
Algorithm settings
A DCNN was trained to detect long-term clinical outcome from the patient CXR at time of referral for coronary angiogram. The ground truth reference of follow-up status was structured using official data from the Italian census. Based on the follow-up status, we identified two groups, dead or alive at long-term follow-up.
We trained the system for binary classification (0, alive; 1 dead). Patients were randomly divided for training (70%) and DCNN model tuning (10%). We performed internal validation (model testing) with the remaining patients (20%).
Given the workload and the numerosity of training samples, we selected a DCNN pre-trained on PadChest (8), NIH ChestX-ray8 (9), CheXpert (10), and MIMIC (11) datasets. In particular, after an extensive model search, we found ResNet50 to be the most suited (12). We exploited TorchXRayVision backed up with PyTorch on Python 3.7 for the model training and validation (13). The performed hyperparameters search to minimize validation loss was carried out using a binary cross-entropy with 100 maximum epochs and early stopping to avoid overfitting. To improve robustness, data augmentation was performed by applying random geometric, brightness, and contrast transformations. All the images underwent a standard normalization between 0 and 1.
External validation
After internal validation of the DCNN, we performed external validation using a sample of 193 patients referred for chest pain to a different medical institution located in north-western Europe, the Leiden University Medical Center (Leiden, the Netherlands). Inclusion criteria in the external validation group were the same as those used for the inclusion in the original dataset.
Statistical analysis
Statistical analysis was performed using the Python scikit-learn and scipy libraries. Sensitivity, specificity, and area under the receiver operating characteristic (ROC) curve (AUC) were calculated to evaluate the binary classifier performance of the DCNN at different thresholds and achieve the highest sensitivity or the maximum sum of sensitivity and specificity. AUCs were compared using the DeLong parametric method, and 95% confidence intervals (CIs) were produced using two-sided CIs for proportions. The association between the DL-CXR risk score and follow-up all-cause mortality (primary outcome) was tested using Cox proportional hazards regression models and Kaplan-Meier (KM) statistics. The ROC AUCs were used to further assess follow-up mortality discrimination of the AI derived radiography risk score and compare it to that of already known risk-factors (age, sex, BMI, presence and extension of CAD, and angina status). The statistical significance threshold was a P value <0.05. Finally, GradCAM heat maps were generated to define the chest radiograph features supporting the different DCNN decisions (14).
Results
Patients
A total of 6,031 patients was randomly divided for model training (71%; n=4,259) and model fine-tuning/model validation (10%; n=602). Internal clinical validation (model testing) was performed with the remaining patients (19%; n=1,170). Demographic and clinical information, including presence and eventual degree of CAD, are presented in Table 1.
Table 1
Clinical data | Overall cohort (N=6,031) | Validation cohort (N=1,170) | |||
---|---|---|---|---|---|
Low risk (N=234) | Moderate risk (N=206) | High risk (N=318) | Very high risk (N=412) | ||
Age (years), mean ± SD | 68±12 | 69±12 | 71±11 | 67±11 | 64±12 |
Biological sex (male) (%) | 67 | 65 | 63 | 69 | 75 |
BMI (kg/m2), mean ± SD | 26±5 | 26±5 | 26±5 | 26±4 | 27±4 |
CAD (%) | |||||
No-CAD/non-severe CAD | 44 | 41 | 46 | 44 | 47 |
One vessel severe CAD | 27 | 26 | 29 | 28 | 23 |
Two vessels severe CAD | 16 | 18 | 15 | 18 | 17 |
Three vessels severe CAD | 12 | 14 | 11 | 10 | 13 |
Left main CAD | 1 | 1 | 1 | 0 | 0 |
DL-CXR, deep learning chest X-ray; SD, standard deviation; BMI, body mass index; CAD, coronary artery disease.
DCNN
Patients were stratified according to DL-based interpretation quartiles (DL-CXR risk score). Median follow-up was 6.1 years [interquartile range (IQR), 3.3–8.7 years] with a KM overall estimated mortality of 21.9% (95% CI: 19.2–24.5%) (Figure 1A) (15). Estimated mortality increased significantly according to the DL-CXR risk score (low-risk 5%, moderate 17%, high 29%, very high 46%; P<0.001) (Figure 1B). At Cox regression, DL-CXR risk score [P<0.005; hazard ratio (HR): 3.30; 95% CI: 2.35–4.64], age (P<0.005; HR: 1.8; 95% CI: 1.1–2.1), presence of severe CAD (P<0.005; HR: 1.3; 95% CI: 1.0–1.7), and angina status (P=0.03; HR: 0.8; 95% CI: 0.7–1.0) were independent predictors of long-term follow-up mortality. Sex (P=0.4) and BMI (P=0.9) were not mortality predictors (Figure 2).
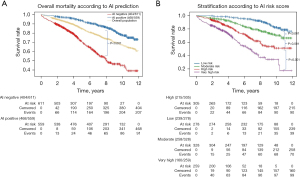
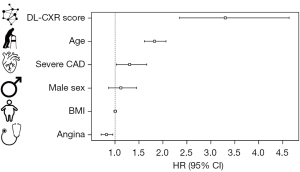
The DL-CXR risk score AUC was 0.793 (95% CI: 0.75–0.82, sensitivity 78%, specificity 68%), significantly better than that achieved when using coronary angiography findings (AUC: 0.569, 95% CI: 0.52–0.61) (P<0.001) and age (AUC 0.735, 95% CI: 0.69–0.77) (P<0.004) as predictors. The DL-CXR mortality prediction model improved when age and CAD status at the referral time were included (AUC 0.809; 95% CI: 0.77–0.84) (Figure 3) (15). Table 2 summarizes the confusion matrix of the model including DL-CXR score, age, and CAD status.
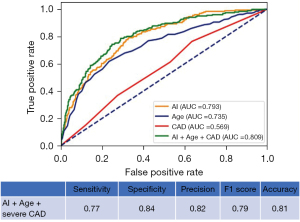
Table 2
CAD status | Predicted positive | Predicted negative |
---|---|---|
Severe CAD | 834 (TP) | 123 (FN) |
Absence of severe CAD | 84 (FP) | 129 (TN) |
The model includes DL-CXR score, CAD status, and age. Metrics: areas under the curve: 0.81; sensitivity: 87%; specificity: 61%; precision: 91%; negative predictive value: 49%. CAD, coronary artery disease; TP, true positive; FP, false positive; FN, false negative; TN, true negative; DL-CXR, deep learning chest X-ray.
External validation with 193 patients confirmed the DL-CXR risk score prediction performance (AUC: 0.71; 95% CI: 0.49–0.92; sensitivity 0.838; specificity 0.338). KM survival analysis revealed a graded association between DL-CXR risk score categories and mortality (P=0.04) (Figure S1).
Heat maps and nomogram for mortality prediction
Attention maps were generated using the DCNN that demonstrated the highest performance, specifically the one incorporating the DL-CXR risk score, CAD status, and age. Heat map activations predominantly focused on areas including the cardiac silhouette, left ventricular apex, pulmonary bases, pulmonary parenchyma, costophrenic sinuses, pulmonary hila, thoracic aorta, neck/supra-aortic vessels, and the clavicle region. Interactive nomograms, derived from the Cox regression analysis, are presented as illustrative cases. These nomograms enable the prediction of mortality risks based on the DL-CXR score, CAD status, and age (Figure S2).
Discussion
Our study shows that: (I) the DCNN model predicted long-term (6-year) overall mortality from a single CXR view performed in patients referred for coronary angiogram for suspected angina pectoris; (II) DL-based prediction of long-term mortality outperformed the prediction based on age, sex and CAD presence/severity; (III) the intrinsic generalizability of the model is supported by the fact that the dataset covered many years of clinical routine, in a large academic hospital, where CXRs are performed using different radiographic equipment; (IV) the DL-CXR performance has been confirmed with validation, internal and external, on patients referred for a similar reason (chest pain, CAD suspicion) to a different medical facility in a different European country; (V) although our results are encouraging, the DCNN has not been tested on a broader population without chest pain, with a lower prevalence of CAD, with a less complex comorbidity profile who may have a lower long-term mortality rate.
CXR is a routine first-line diagnostic armamentarium in patients referred for suspected cardiopulmonary conditions. CXR contains a plethora of information about the patient’s general health, such as body habitus, cardiovascular condition, pulmonary status [lung congestion, interstitial lung disease, chronic obstructive pulmonary disease (COPD)], and the bones/skeletal condition, including mineral density. All this information is related to the presence of comorbidities and a frailty profile that may impact follow-up survival (16-20) and may support the fact that DL-read plain CXR may predict patients’ outcomes better than any single risk factor taken alone. The DL-CXR risk could be a surrogate for frailty and other comorbidities and could be capturing unmeasured confounders, such as chronic pulmonary disease, osteoporosis, and many other comorbidities that may suggest worse prognosis and frailty.
We have previously demonstrated that DL-read CXRs could be used to pre-test significant CAD probability in patients referred for suspected angina (6). A DCNN adequately trained in identifying severe CAD, using coronary angiography as ground truth, can detect the presence of severe CAD from a one-projection CXR and distinguish among patients with different degrees of CAD severity (6). Starting from this assumption, we have focused the present study on the same patient cohort previously referred for coronary angiography and have trained, this time, our DCNN to predict mortality outcomes at long-term follow-up. In a prognostic study, Lu et al. have used a large cohort of patients (over 50,000) from the Prostate, Lung, Colorectal, and Ovarian Cancer Screening Trial (PLCO) to train and validate a DCNN to predict long-term mortality from chest radiograms. System performance was externally validated using data from the screening radiography arm of the National Lung Screening (NLS) Trial (5). Using DCNN interpretation of diagnostic findings and standard risk factors, Lu et al. could identify patients with an increased risk of 6- and 12-year mortality. The DL-CXR risk score exhibited a graded association with mortality, ranging from 2.7% in the low-risk group to 33.9% in the very high-risk group (5). The DL-CXR risk score achieved AUC values of 0.75 for 12-year mortality in the internal testing set and 0.68 for 6-year mortality in the external testing set (5). In the same patient population, the same group has more recently confirmed that the DL-CXR risk has a graded association with lung disease mortality after adjustment for risk factors, including age, smoking, and radiologic findings (with HR up to 11) (21). Our study, conducted in a cohort with a high prevalence of severe CAD (over 50%), further confirms this graded association. All quartiles of DL-CXR risk scores showed higher mortality rates, possibly due to the complex comorbid profile of our patients, particularly those with severe CAD, which led to poorer outcomes compared to patients in a cancer prevention program.
As emerging from our analysis and previously confirmed by Lu et al., the DCNN interpretation of the CXRs allows us to identify a prognostic value that is complementary to broadly used risk factors for follow-up mortality (5). It has already been proposed that substituting biological age measures for chronological age could improve the performance of existing risk scores (22). Raghu et al. and more recently Mitsuyama et al. have proposed an AI/DL-based estimate of biological age starting from a CXR (22,23). The CXR age predicts longevity and the presence of various chronic diseases beyond chronological age (23,24). There are possibly some common activation areas that can be identified within the radiographic image, like the mediastinum width, the aortic knob calcification and aortic tortuosity, the cardiac silhouette geometrical ratios, the bone and muscle density, and so on that modify with age. All these parameters could actually be used as surrogates for biological age and act together, to support a single estimator of the biological age and overall health status, for the AI-based prediction of chronic conditions, outcomes after interventions, and long-term events (25-30). Our logistic regression and ROC statistics findings confirm that the AI/DL-based CXR-score can predict long-term mortality significantly better than chronological age.
Apart from the prediction of long-term outcomes, DL evaluation of emergency department CXRs has been used to identify patients referred for acute chest pain syndrome who are at risk for a 30-day composite adverse outcome, including acute coronary syndrome, pulmonary embolism, aortic dissection, and overall mortality (31). The DL tool improved the prediction of these acute adverse outcomes beyond age, sex, and conventional troponin/D-dimer positivity (31). In a first referral setting, the CXR-score could help clinicians to defer unnecessary additional, more invasive investigations in 14% of the referred patients (31).
It should be emphasized that the DL-based CXR score should not be extrapolated from the overall clinical setting, and additional clinical information concerning the patient’s demography and health status may help optimize the image-based AI/DL prediction of events in the acute setting and during long-term follow-up. Kolossváry et al. have reported a significant improvement in the prediction of 30-day cardiovascular adverse events in a model that included, simultaneously, age, sex, biomarkers (troponin), and AI-based CXR score (from an AUC of 0.80 in the sole CXR-score model to an AUC of 0.85 in the CXR-score, sex, age, troponin model) (31). Raghu et al. have shown only a slight improvement in the prediction of 12-year follow-up mortality, from an AUC of 0.75 to an AUC of 0.78, when adding to the CXR-score based model information concerning documented risk factors (age, sex, smoking category, diabetes, hypertension, obesity, underweight, past myocardial infarction, past stroke, and past cancer) and reported CXR pathologic findings (such as e lung nodule, significant atelectasis, pleural plaque or effusion, lymphadenopathy, chest wall or bony lesion, COPD/emphysema, cardiomegaly or other cardiovascular abnormality, and lung fibrosis) (23). The impact of known pathologic conditions, such as CAD, on follow-up mortality has been documented and should be considered when designing DL-based prediction models (24). In our cohort, all patients underwent coronary angiography, allowing for stratification based on CAD presence and severity. As expected, in our Cox regression model, presence of severe CAD had a more significant prediction of follow-up mortality when compared to angina status. This is possibly since severe CAD is a factor influencing death (quoad vitam), while angina pectoris is a negative factor affecting quality of life (quoad valitudinem).
Intriguingly, the outcome prediction based on CXR was more pronounced than that based on CAD classification alone. It is worth noting that our model did not account for patients who received intensified medical management following coronary angiography. This oversight might have contributed to the relatively low predictive power of CAD severity on long-term outcomes that could be attributed to the mitigating effect of timely and appropriate treatment post-coronary angiography, including necessary invasive interventions (32). Moreover, cardiovascular disease accounts for only a third of overall mortality in Italy and the Netherlands. Consequently, as we were addressing overall mortality, adding age and CAD status only marginally improved the CXR score’s performance.
Finally, previously proposed mortality risk scores, built on linear models (such as logistic and linear regression), explainable tree-based models, and more complex DL models, have shown good accuracy, with AUCs reaching 0.90 in the prediction of long-term mortality, by including many demographics, laboratory, questionnaires, and at times epigenetic and gene expression data for the identification of so-called biological clocks (33-37). Although we believe that DL models that incorporate additional demographic and clinical data, including multiple additional imaging and laboratory tests, may have a more accurate prognostic value and may be able to detect changes in risk that can occur at different time points and result from external human-driven interventions, we should be aware of the budgetary and organizational costs vs. accuracy trade-offs. In this light, the main aim of our research was to support the fact that the DCNN can extract from a simple, affordable, and ubiquitous investigation, such as the CXR performed at the time of referral in different settings, the “hidden fingerprints” of long-term outcomes that are embedded in the image, and that the DCNN performance is independent of additional demographic or clinical information.
Limitations
The DCNN has been trained, tested, and validated on patients referred for invasive coronary angiography, considering a clinical suspect of angina. Critical patients’ selection biases limit our findings. The population carries a specific burden of comorbidities that is not necessarily present in other patients’ groups and may have impacted events occurrence. For this reason, the DCNN should also be validated in a broader population of healthy people that are asymptomatic for chest pain. Moreover, because of the study’s retrospective nature and many additional budgetary/logistical limitations, a limited amount of clinical information, derived from the study cohort, has been adopted. Although it is indeed true that, apart from very basic demographic and clinical information we do miss important additional data such as renal function, echocardiographic parameters, comorbidities and several more, we do have included in our analysis very specific and unique information concerning the presence and extension of CAD, confirmed by invasive studies. That said, we are aware that in the future, to achieve a more accurate and personalized non-static event prediction and clinical utility of the model, the CXR-score will need to be integrated with additional demographic, clinical, and possibly medical imaging, laboratory test findings, time-dependent events, and human interventions. Those implementations may impact the model’s explainability, sustainability, and fairness (applicability in different and less structured healthcare environments). Indeed, it is not clear yet if even very simple information, such as smoking history, COPD, previous myocardial infarction, that have all a proven impact upon survival, are actually represented in the CXR and adequately detected by the algorithm and kept into account for the risk scoring. Finally, making clarity within the “black box” of the DL algorithm’s decision-making process is not an easy task, and the suboptimal explainability of the model may hinder its application in the healthcare sector. Although the produced attention maps may partly enhance the CXR areas most often involved in the mortality prediction, we still do not know why the model assigns a specific score and makes a particular prediction. We will need to address this issue in the future by investigating the cause of death and resorting to techniques around explainable AI/DL that help clarify complex DL models. Finally, although we could confirm the performance of our outcome prediction model at external validation, the AUC did drop from 0.79 to 0.71, which is not insignificant. This could be due to the small external testing dataset, or also to overfitting to the training dataset. This finding should encourage additional external validation, including larger cohort of patients with different and less marked comorbidity profiles.
Conclusions
CXRs are routinely performed in patients referred for different conditions and healthy subjects for screening. This simple and affordable imaging modality should be considered for risk stratification, including the prediction of long-term mortality. The DL-CXR risk score predicted long-term overall mortality of patients with suspected angina pectoris referred for invasive investigation. Internal and external validation on patients in two different European regions (north and south of Europe) are satisfactory. The DL-CXR risk score provided a long-term mortality risk stratification superior to that achieved when using CAD status information, sex, and chronological age. The DL-CXR risk score could be used to support screening and prevention. It should be remarked that our findings and our model, in its present form, cannot be extended to patients’ cohorts with different risk profiles, including healthy individuals. In the future, the DL-CXR risk score could be used as an adjunctive armamentarium to estimate, together with a plethora of additional tests, the risk of follow-up adverse events and to guide, in this way, anticipated actions to change unhealthy lifestyles and trigger a prompt referral to additional and more specific investigations, whenever clinically sound. A DL-CXR model that effectively predicts cardiovascular mortality in ostensibly healthy individuals could be invaluable in guiding clinical strategies to alter cardiovascular outcomes.
Acknowledgments
This work has been presented as an oral abstract at the 2023 European Society of Cardiology Congressm (Amsterdam, 25-28 August 2023).
Funding: None.
Footnote
Reporting Checklist: The authors have completed the TRIPOD reporting checklist. Available at https://jtd.amegroups.com/article/view/10.21037/jtd-24-322/rc
Data Sharing Statement: Available at https://jtd.amegroups.com/article/view/10.21037/jtd-24-322/dss
Peer Review File: Available at https://jtd.amegroups.com/article/view/10.21037/jtd-24-322/prf
Conflicts of Interest: All authors have completed the ICMJE uniform disclosure form (available at https://jtd.amegroups.com/article/view/10.21037/jtd-24-322/coif). G.D. serves as an unpaid editorial board member of Journal of Thoracic Disease from February 2023 to January 2025. The other authors have no conflicts of interest to declare.
Ethical Statement: The authors are accountable for all aspects of the work in ensuring that questions related to the accuracy or integrity of any part of the work are appropriately investigated and resolved. Patients had signed informed consent to use relevant clinical information for scientific purposes. All data have been anonymized, and the study protocol has been approved by the local scientific and ethical committee (Comitato Etico-Scientifico Brescia, Protocol No. NP 4817). The study was conducted in accordance with the Declaration of Helsinki (as revised in 2013).
Open Access Statement: This is an Open Access article distributed in accordance with the Creative Commons Attribution-NonCommercial-NoDerivs 4.0 International License (CC BY-NC-ND 4.0), which permits the non-commercial replication and distribution of the article with the strict proviso that no changes or edits are made and the original work is properly cited (including links to both the formal publication through the relevant DOI and the license). See: https://creativecommons.org/licenses/by-nc-nd/4.0/.
References
- Knuuti J, Wijns W, Saraste A, et al. 2019 ESC Guidelines for the diagnosis and management of chronic coronary syndromes. Eur Heart J 2020;41:407-77. [Crossref] [PubMed]
- Ron E. Cancer risks from medical radiation. Health Phys 2003;85:47-59. [Crossref] [PubMed]
- Bader AS, Rubinowitz AN, Gange CP Jr, et al. Imaging in the Evaluation of Chest Pain in the Primary Care Setting, Part 1: Cardiovascular Etiologies. Am J Med 2020;133:1033-8. [Crossref] [PubMed]
- Seah JCY, Tang CHM, Buchlak QD, et al. Effect of a comprehensive deep-learning model on the accuracy of chest x-ray interpretation by radiologists: a retrospective, multireader multicase study. Lancet Digit Health 2021;3:e496-506. [Crossref] [PubMed]
- Lu MT, Ivanov A, Mayrhofer T, et al. Deep Learning to Assess Long-term Mortality From Chest Radiographs. JAMA Netw Open 2019;2:e197416. [Crossref] [PubMed]
- D'Ancona G, Massussi M, Savardi M, et al. Deep learning to detect significant coronary artery disease from plain chest radiographs AI4CAD. Int J Cardiol 2023;370:435-41. [Crossref] [PubMed]
- Genders TS, Steyerberg EW, Alkadhi H, et al. A clinical prediction rule for the diagnosis of coronary artery disease: validation, updating, and extension. Eur Heart J 2011;32:1316-30. [Crossref] [PubMed]
- Bustos A, Pertusa A, Salinas JM, et al. PadChest: A large chest x-ray image dataset with multi-label annotated reports. Med Image Anal 2020;66:101797. [Crossref] [PubMed]
- Wang X, Peng Y, Lu L, et al. ChestX-Ray8: Hospital-Scale Chest X-Ray Database and Benchmarks on Weakly-Supervised Classification and Localization of Common Thorax Diseases. 2017 IEEE Conference on Computer Vision and Pattern Recognition (CVPR); 21-26 July 2017; Honolulu, HI, USA. IEEE; 2017:3462-71.
- Irvin J, Rajpurkar P, Ko M, et al. CheXpert: A Large Chest Radiograph Dataset with Uncertainty Labels and Expert Comparison. Proceedings of the AAAI Conference on Artificial Intelligence. 2019;33:590-7. [Crossref]
- Johnson AEW, Pollard TJ, Berkowitz SJ, et al. MIMIC-CXR, a de-identified publicly available database of chest radiographs with free-text reports. Sci Data 2019;6:317. [Crossref] [PubMed]
- He K, Zhang X, Ren S, et al. Deep Residual Learning for Image Recognition. 2016 IEEE Conference on Computer Vision and Pattern Recognition (CVPR); 27-30 June 2016; Las Vegas, NV, USA. IEEE; 2016:770-8.
- Cohen JP, Viviano JD, Bertin P, et al. TorchXRayVision: A library of chest X-ray datasets and models. arXiv:2111.00595 [Preprint]. 2021. Available online: https://doi.org/
10.48550 /arXiv.2111.00595 - Selvaraju RR, Cogswell M, Das A, et al. Grad-CAM: Visual Explanations from Deep Networks via Gradient-Based Localization. 2017 IEEE International Conference on Computer Vision (ICCV); 22-29 October 2017; Venice, Italy. IEEE; 2017:618-26.
- D'ancona G, Savardi M, Massussi M, et al. Deep learning to predict long-term mortality from plain chest radiographs in patients referred for suspected angina, Eur Heart J 2023;44:ehad655.1464.
- Clarson LE, Bajpai R, Whittle R, et al. Interstitial lung disease is a risk factor for ischaemic heart disease and myocardial infarction. Heart 2020;106:916-22. [Crossref] [PubMed]
- Boschetto P, Beghé B, Fabbri LM, et al. Link between chronic obstructive pulmonary disease and coronary artery disease: implication for clinical practice. Respirology 2012;17:422-31. [Crossref] [PubMed]
- Zhang Y, He B, Wang H, et al. Associations between bone mineral density and coronary artery disease: a meta-analysis of cross-sectional studies. Arch Osteoporos 2020;15:24. [Crossref] [PubMed]
- Kumar DA, Anburajan M. The role of hip and chest radiographs in osteoporotic evaluation among south Indian women population: a comparative scenario with DXA. J Endocrinol Invest 2014;37:429-40. [Crossref] [PubMed]
- Zaman MJ, Sanders J, Crook AM, et al. Cardiothoracic ratio within the "normal" range independently predicts mortality in patients undergoing coronary angiography. Heart 2007;93:491-4. [Crossref] [PubMed]
- Weiss J, Raghu VK, Bontempi D, et al. Deep learning to estimate lung disease mortality from chest radiographs. Nat Commun 2023;14:2797. [Crossref] [PubMed]
- Grundy SM. Coronary plaque as a replacement for age as a risk factor in global risk assessment. Am J Cardiol 2001;88:8E-11E. [Crossref] [PubMed]
- Raghu VK, Weiss J, Hoffmann U, et al. Deep Learning to Estimate Biological Age From Chest Radiographs. JACC Cardiovasc Imaging 2021;14:2226-36. [Crossref] [PubMed]
- Mitsuyama Y, Matsumoto T, Tatekawa H, et al. Chest radiography as a biomarker of ageing: artificial intelligence-based, multi-institutional model development and validation in Japan. Lancet Healthy Longev 2023;4:e478-86. [Crossref] [PubMed]
- Pyrros A, Borstelmann SM, Mantravadi R, et al. Opportunistic detection of type 2 diabetes using deep learning from frontal chest radiographs. Nat Commun 2023;14:4039. [Crossref] [PubMed]
- Ueda D, Matsumoto T, Ehara S, et al. Artificial intelligence-based model to classify cardiac functions from chest radiographs: a multi-institutional, retrospective model development and validation study. Lancet Digit Health 2023;5:e525-33. [Crossref] [PubMed]
- Ryu J, Eom S, Kim HC, et al. Chest X-ray-based opportunistic screening of sarcopenia using deep learning. J Cachexia Sarcopenia Muscle 2023;14:418-28. [Crossref] [PubMed]
- Raghu VK, Moonsamy P, Sundt TM, et al. Deep Learning to Predict Mortality After Cardiothoracic Surgery Using Preoperative Chest Radiographs. Ann Thorac Surg 2023;115:257-64. [Crossref] [PubMed]
- Jang M, Kim M, Bae SJ, et al. Opportunistic Osteoporosis Screening Using Chest Radiographs With Deep Learning: Development and External Validation With a Cohort Dataset. J Bone Miner Res 2022;37:369-77. [Crossref] [PubMed]
- Ieki H, Ito K, Saji M, et al. Deep learning-based age estimation from chest X-rays indicates cardiovascular prognosis. Commun Med (Lond) 2022;2:159. [Crossref] [PubMed]
- Kolossváry M, Raghu VK, Nagurney JT, et al. Deep Learning Analysis of Chest Radiographs to Triage Patients with Acute Chest Pain Syndrome. Radiology 2023;306:e221926. [Crossref] [PubMed]
- Hanson CA, Lu E, Ghumman SS, et al. Long-term outcomes in patients with normal coronary arteries, nonobstructive, or obstructive coronary artery disease on invasive coronary angiography. Clin Cardiol 2021;44:1286-95. [Crossref] [PubMed]
- Qiu W, Chen H, Dincer AB, et al. Interpretable machine learning prediction of all-cause mortality. Commun Med (Lond) 2022;2:125. [Crossref] [PubMed]
- Ganna A, Ingelsson E. 5 year mortality predictors in 498,103 UK Biobank participants: a prospective population-based study. Lancet 2015;386:533-40. [Crossref] [PubMed]
- Horne BD, May HT, Muhlestein JB, et al. Exceptional mortality prediction by risk scores from common laboratory tests. Am J Med 2009;122:550-8. [Crossref] [PubMed]
- Liu Z, Kuo PL, Horvath S, et al. A new aging measure captures morbidity and mortality risk across diverse subpopulations from NHANES IV: A cohort study. PLoS Med 2018;15:e1002718. [Crossref] [PubMed]
- Horvath S, Raj K. DNA methylation-based biomarkers and the epigenetic clock theory of ageing. Nat Rev Genet 2018;19:371-84. [Crossref] [PubMed]