The prognostic analysis and a machine-learning based disease-specific survival state model in pulmonary large-cell neuroendocrine carcinomas
Highlight box
Key findings
• The gradient boosting decision tree (GBDT) showed promising performance in predicting 2-year survival, which can serve as a valuable reference for its clinical diagnosis and treatment in pulmonary large-cell neuroendocrine carcinoma (PLCNEC).
What is known and what is new?
• PLCNEC is a rare and highly aggressive tumor characterized by early distant metastasis and recurrence, leading to a dismal prognosis. Due to limited availability of clinical data, there is currently no robust predictive model for accurately assessing patient survival outcomes.
• This study presents the first application of machine learning techniques to construct a survival prediction model for PLCNEC, and by inputting the clinical characteristic data into the trained model, we can easily predict the patient’s 2-year survival status.
What is the implication, and what should change now?
• The implementation of early treatment and intervention is crucial for patients with PLCNEC, while the evaluation of clinical characteristics plays a significant role in determining prognosis.
Introduction
Pulmonary large-cell neuroendocrine carcinoma (PLCNEC), representing 1–3% of all primary lung cancers, is a rare and aggressive tumor with a poor prognosis and high recurrence rate, originating from argyrophilic cells in lung and bronchial mucosa (1). According to the 2015 World Health Organization (WHO) classification criteria, large-cell neuroendocrine carcinoma (LCNEC) is classified as a neuroendocrine (NE) tumor with three standard NE markers (synaptophysin, chromogranin A, and CD56). Its microscopic features include large tumor cells, abundant eosinophilic cytoplasm, obvious polymorphism, distinct nucleoli, high mitosis rate (>10/2 mm2), and frequent necrosis (2). Similar to small cell lung cancer (SCLC), PLCNEC also tends to occur in elderly men and is closely related to smoking and prone to distant metastasis, making it difficult to distinguish the two due to the high mitotic number and expression of NE-related protein (3). Compared with other lung cancers, PLCNEC is prone to develop early distant metastases and exhibits a poorer prognosis, with an overall 5-year survival rate of approximately between 15% and 57%, and recurrence and progression largely occur within the 2-year follow-up (4,5). The molecular study has identified two major types of PLCNEC: one type has genomic features of SCLC, characterized by retinoblastoma 1 (RB1) and tumor protein p53 (TP53) alterations, and the other type is similar to non-small cell lung cancer (NSCLC), characterized primarily by alterations typical of smoking-associated adenocarcinoma [serine/threonine kinase 11 (STK11), Kelch-like ECH-associated protein 1 (KEAP1), Kirsten rat sarcoma (KRAS)] and the absence of RB1 alterations (6). Because of the clinical similarity of PLCNEC and SCLC, the etoposide/platinum combination has been the gold standard first-line treatment for advanced PLCNECs for years. However, in recent years, it has been widely proposed that “NSCLC-like” advanced LCNECs should be treated both in first- and second-line settings, with chemotherapy regimens used for the treatment of NSCLC (7). Due to its rarity and biological heterogeneity, there is still no consensus on the most appropriate therapeutic approach.
Machine learning is the process of fitting predictive models to data or identifying informative groupings within data by approximating or imitating humans’ ability to recognize patterns objectively (8). This emerging artificial intelligence technology has been increasingly utilized in the biomedical field, particularly for making accurate predictions of target outcomes with insufficient experimental data, which can guide future clinical research.
To establish a reliable and stable prognostic model for patients with PLCNEC, the current study utilized machine learning techniques and the Surveillance, Epidemiology, and End Results (SEER) database. Firstly, clinical characteristics and treatment information of PLCNEC patients were extracted from the SEER database to identify related prognostic risk factors. Subsequently, a predictive model was developed using machine learning algorithms, incorporating various prognostic factors such as age, race, laterality, tumor size, American Joint Committee on Cancer-tumor node metastasis staging (AJCC-TNM) stage, and Treatment & Organ Transfer Information. Our study findings may guide clinical treatment decisions and offer valuable insights for future research. We present this article in accordance with the TRIPOD reporting checklist (available at https://jtd.amegroups.com/article/view/10.21037/jtd-23-1927/rc).
Methods
Data source and data extraction
The clinical data of patients diagnosed with PLCNEC from 2010–2020 were obtained from the SEER database accessed using the Case Listing Session of SEER*Stat software version 8.4.1. Among the databases provided by the SEER program, the “SEER Research Data, 17 Registries, Nov 2022 Sub (2000–2020)” database and “Site recode: International Classification of Diseases for Oncology 3 (ICD-O-3)—lung and bronchus, histologic type ICD-O-3 = 8013, year of diagnosis ≥2010” were selected. The exclusion criteria were as follows: (I) information about AJCC-TNM, AJCC-Stage, tumor size, and metastasis was unclear; (II) survival time was less than 1 month; (III) the tumor stage was M1 or IV but no clear site of distant metastasis was provided. A total of 2,897 patients were finally recruited for the follow-up study analysis (Figure 1). The clinicopathological data collected from 30 patients diagnosed with PLCNEC between 2010 and 2022 at The First Affiliated Hospital of Sun Yat-sen University were used as external test data to validate the accuracy of the trained machine. The follow-up period was extended until November 30, 2023. The study was conducted in accordance with the Declaration of Helsinki (as revised in 2013).
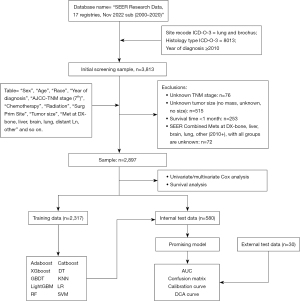
Statistical analysis
The Kaplan-Meier method was utilized to analyze the estimated survival rates at different time points for various subgroups. Univariate and multivariate Cox regression analyses were employed to identify risk or favorable factors influencing the survival status of PLCNEC patients using a hazard ratio (HR) with a 95% confidence interval (CI). The primary outcome was disease-specific survival (DSS), defined as death caused by PLCNEC. The secondary outcome was overall survival (OS), defined as death from any cause, including PLCNEC. All data were analyzed using IBM SPSS Statistics (version 27.0.1). A P value of less than 0.05 was considered statistically significant.
Ten machine learning algorithms were employed, including Adaboost, Catboost, XGboost, decision tree (DT), gradient boosting decision tree (GBDT), k-nearest neighbor (KNN), light gradient boosting machine (LightGBM), logistic regression (LR), random forest (RF), and support vector machine (SVM). All PLCNEC patients were randomly divided into training and testing sets in an 80:20 ratio before building machine learning models. The training and test sets were partitioned using the train_test_split module from the Scikit-learn (Sklearn) library in Python, ensuring random allocation. The performance of each algorithm was evaluated in the test set using metrics such as accuracy, precision, sensitivity, F1 score, and receiver operating characteristic (ROC) curve. Furthermore, a confusion matrix, calibration curve, and decision curve analysis (DCA) were established to visually and comprehensively assess the validity and clinical benefits of the models. All tests were performed using Python version 3.10.
Results
Baseline clinicopathological characteristics of PLCNEC patients
The distribution of baseline clinical characteristics of patients in the LCNEC group is shown in Table 1. Among the enrolled LCNEC patients, 1,161 were aged <65 years, and 1,736 were aged ≥65 years. There were significantly more women than men (59.9% vs. 40.1%), and the majority of the patients were white (82.6%). For tumor size comparison, the results were as follows: the proportion of patients with a tumor diameter of <5 cm was significantly higher than that of patients with a tumor diameter of ≥5 cm (70.0% vs. 30.0%). Regarding the AJCC cancer staging, the proportion of patients in T1 + T2 and N0 + N1 stages was higher (59.7% vs. 38.3%, 55.2% vs. 43.1%), but nearly one-half of the patients developed distant organ metastases (M1, 42.1%) during the progression of the disease. In terms of tumor treatment, 40.4% of patients underwent prime site surgery and only 17% of patients underwent radiotherapy pre- or post-surgery. Meanwhile, 55.5% of patients received chemotherapy. For OS, it was found that the vast majority of patients (79.9%) survived less than 3 years, and death was the main outcome (72.7%). The proportion of DSS was 61.5%, suggesting a poor prognosis of PLCNEC patients.
Table 1
Characteristics | SEER data (N=2,897), n (%) | External data (N=30), n (%) | P |
---|---|---|---|
Age (years) | 0.003 | ||
<65 | 1,161 (46.0) | 20 (66.7) | |
≥65 | 1,736 (54.0) | 10 (33.3) | |
Sex | <0.001 | ||
Male | 1,332 (40.1) | 26 (86.7) | |
Female | 1,565 (59.9) | 4 (13.3) | |
Race | <0.001 | ||
Black | 372 (12.8) | 0 | |
White | 2,392 (82.6) | 0 | |
Others | 128 (4.4) | 30 (100.0) | |
Unknown | 5 (0.2) | 0 | |
T | 0.02 | ||
T1 | 892 (30.8) | 3 (10.0) | |
T2 | 836 (28.9) | 10 (33.3) | |
T3 | 529 (18.3) | 11 (36.7) | |
T4 | 580 (20.0) | 5 (16.7) | |
Tx | 60 (2.1) | 1 (1.5) | |
N | 0.68 | ||
N0 | 1,309 (45.2) | 10 (33.3) | |
N1 | 291 (10.0) | 4 (13.3) | |
N2 | 894 (30.9) | 9 (30.0) | |
N3 | 354 (12.2) | 5 (16.7) | |
Nx | 49 (1.7) | 2 (6.7) | |
M | 0.61 | ||
M0 | 1,677 (57.9) | 16 (53.3) | |
M1 | 1,220 (42.1) | 14 (46.7) | |
Stage | 0.82 | ||
I | 664 (22.9) | 8 (26.7) | |
II | 378 (13.0) | 3 (10.0) | |
III | 635 (21.9) | 5 (16.7) | |
IV | 1,220 (42.1) | 14 (46.7) | |
Tumor size (cm) | 0.60 | ||
<3 | 1,173 (40.5) | 13 (43.3) | |
3 to <5 | 855 (29.5) | 5 (16.7) | |
5–7 | 414 (14.3) | 6 (20.0) | |
>7 | 455 (15.7) | 6 (20.0) | |
Laterality | 0.94 | ||
Left | 1,197 (41.3) | 12 (40.0) | |
Right | 1,669 (57.6) | 18 (60.0) | |
Paired site/bilateral | 29 (1.0) | 0 | |
Unknown | 2 (0.1) | 0 | |
Primary surgery | 0.28 | ||
No | 1,728 (59.6) | 15 (50.0) | |
Yes | 1,169 (40.4) | 15 (50.0) | |
Chemotherapy | 0.80 | ||
No | 1,288 (44.5) | 14 (46.7) | |
Yes | 1,609 (55.5) | 16 (53.3) | |
Surgery and radiotherapy | 0.13 | ||
No | 2,404 (83.0) | 28 (93.3) | |
Yes | 493 (17.0) | 2 (6.7) | |
Bone metastasis | 0.89 | ||
No | 2,439 (84.2) | 25 (83.3) | |
Yes | 458 (15.8) | 5 (16.7) | |
Brain metastasis | 0.29 | ||
No | 2,395 (82.7) | 27 (90.0) | |
Yes | 502 (17.3) | 3 (10.0) | |
Liver metastasis | 0.87 | ||
No | 2,482 (85.7) | 26 (86.7) | |
Yes | 415 (14.3) | 4 (13.3) | |
Lung metastasis | 0.03 | ||
No | 2,634 (90.9) | 24 (80.0) | |
Yes | 263 (9.1) | 6 (20.0) | |
Other metastasis | 0.29 | ||
No | 2,085 (72.0) | 19 (63.3) | |
Yes | 812 (28.0) | 11 (36.7) | |
Survival time (years) | 0.04 | ||
<1 | 1,511 (52.2) | 12 (40.0) | |
1 to <3 | 803 (27.7) | 7 (23.3) | |
3–5 | 283 (9.8) | 3 (10.0) | |
>5 | 300 (10.4) | 8 (26.7) | |
Disease-specific survival | 0.02 | ||
Alive or dead of other cause | 1,114 (38.5) | 14 (46.7) | |
Dead | 1,783 (61.5) | 16 (53.3) |
SEER, Surveillance, Epidemiology, and End Results; TNM, tumor node metastasis.
Estimated survival rate in all patients
The estimated survival rates based on DSS for the 2,897 enrolled PLCNEC patients are presented in Table 2. The median survival time for the overall group was only 17 months, which further decreased to 7 months in stage IV. Only 41.4% of patients survive beyond 2 years. As anticipated, survival rates from stage I to IV declined gradually, with a significant drop when tumors metastasized to distant organs and tissues at stage IV, where the 2-year survival rate was 13.1%.
Table 2
Tumor stage | Median survival time (months) | Survival rate of different years (%) | ||||
---|---|---|---|---|---|---|
0.5 year | 1 year | 2 years | 3 years | 5 years | ||
Overall | 17.0 | 73.6 | 56.6 | 41.4 | 34.1 | 28.4 |
I | – | 97.2 | 91.3 | 79.7 | 71.9 | 62.3 |
II | 35.0 | 91.7 | 78.9 | 56.1 | 49.2 | 44.6 |
III | 20.0 | 81.6 | 63.8 | 42.8 | 31.7 | 23.2 |
IV | 7.0 | 50.6 | 25.9 | 13.1 | 7.3 | 4.0 |
Survival analysis
Kaplan-Meier curves, representing various demographic and clinical characteristics, are presented in Figure 2. Patients aged ≥65 years, male gender, and those with distant organ metastasis such as bone, liver, and brain metastasis exhibited a significantly poorer prognosis, while primary surgery significantly improved the survival time, consistent with the results obtained from Cox regression analysis. Additionally, the poorer survival outcomes of patients were positively correlated with an increase in the AJCC-TNM stage level. Intriguingly, the survival curve for patients receiving chemotherapy did not align with our expectations, initially showing a better prognosis with a survival time of approximately <12 months but later exhibiting the opposite trend. This discrepancy may be attributed to the fact that most patients receiving chemotherapy were in stage III–IV, which inherently has a shorter survival time. Therefore, further clinical studies are warranted to validate these findings. Nonetheless, the prognostic impact of primary site laterality and race was insignificant.
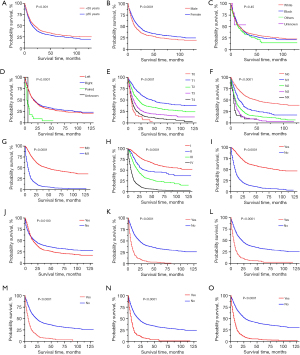
Univariate and multivariate Cox analyses
To investigate the risk factors influencing survival and prognosis in patients with poorly differentiated PLCNEC, univariate Cox analysis was initially performed to identify prognostic variables. The results showed that age (P=0.002), sex (P<0.001), AJCC cancer staging (P<0.001), tumor size (P<0.001), laterality (P<0.001), primary surgery (P<0.001), chemotherapy (P=0.01), and distant metastasis (P<0.001) were related factors affecting survival time (Table 3). Multivariate Cox regression analysis revealed that age (HR 1.262, 95% CI: 1.144–1.391, P<0.001), sex (HR 1.168, 95% CI: 1.063–1.284, P=0.001), N1 (HR 1.389, 95% CI: 1.166–1.656, P<0.001), N2 (HR 1.433, 95% CI: 1.245–1.649, P<0.001), N3 (HR 1.638, 95% CI: 1.386–1.937, P<0.001), NX (HR 1.464, 95% CI: 1.036–2.070, P=0.03), TNM stages II (HR 1.725, 95% CI: 1.383–2.151, P<0.001), III (HR 2.048, 95% CI: 1.635–2.564, P<0.001), IV (HR 3.801, 95% CI: 3.005–4.807, P<0.001), primary surgery (HR 0.481, 95% CI: 0.413–0.559, P<0.001), chemotherapy (HR 0.450, 95% CI: 0.404–0.501, P<0.001), bone metastasis (HR 1.284, 95% CI: 1.124–1.466, P<0.001), liver metastasis (HR 1.223, 95% CI: 1.069–1.399, P=0.003), and brain metastasis (HR 1.167, 95% CI: 1.023–1.331, P=0.02) were independent factors affecting survival. Specifically, male gender, age ≥65 years old, advanced N category or TNM stage, and bone, liver, and brain metastases were associated with a poorer prognosis, whereas primary surgery and chemotherapy significantly improved patient outcomes.
Table 3
Factors | Univariate analysis | Multivariate analysis | |||
---|---|---|---|---|---|
χ2 | P | HR (95% CI) | P | ||
Age | 9.423 | 0.002 | 1.262 (1.144–1.391) | <0.001 | |
Sex | 23.084 | <0.001 | 1.168 (1.063–1.284) | 0.001 | |
Race | 1.600 | 0.20 | – | – | |
T | 296.045 | <0.001 | – | – | |
T0 | – | – | Reference | ||
T1 | – | – | 0.666 (0.486–0.913) | 0.01 | |
T2 | – | – | 0.920 (0.675–1.254) | 0.59 | |
T3 | – | – | 1.056 (0.773–1.442) | 0.73 | |
T4 | – | – | 1.094 (0.804–1.488) | 0.56 | |
N | 550.451 | <0.001 | – | – | |
N0 | – | – | Reference | ||
N1 | – | – | 1.389 (1.166–1.656) | <0.001 | |
N2 | – | – | 1.433 (1.245–1.649) | <0.001 | |
N3 | – | – | 1.638 (1.386–1.937) | <0.001 | |
NX | – | – | 1.464 (1.036–2.070) | 0.03 | |
M | 1,029.510 | <0.001 | – | – | |
Stage | 994.573 | <0.001 | – | – | |
I | – | – | Reference | ||
II | – | – | 1.725 (1.383–2.151) | <0.001 | |
III | – | – | 2.048 (1.635–2.564) | <0.001 | |
IV | – | – | 3.801 (3.005–4.807) | <0.001 | |
Tumor size (cm) | 226.800 | <0.001 | – | – | |
Laterality | 26.314 | <0.001 | – | – | |
Primary surgery | 833.403 | <0.001 | 0.481 (0.413–0.559) | <0.001 | |
Chemotherapy | 6.386 | 0.01 | 0.450 (0.404–0.501) | <0.001 | |
Surgery and radiotherapy | 4.037 | 0.045 | – | – | |
Distant metastasis | 1,029.510 | <0.001 | – | – | |
Bone metastasis | 87.728 | <0.001 | 1.284 (1.124–1.466) | <0.001 | |
Brain metastasis | 61.087 | <0.001 | 1.167 (1.023–1.331) | 0.02 | |
Liver metastasis | 60.284 | <0.001 | 1.223 (1.069–1.399) | 0.003 | |
Lung metastasis | 60.284 | <0.001 | – | – | |
Other metastasis | 155.728 | <0.001 | – | – |
PLENEC, pulmonary large-cell neuroendocrine carcinoma; HR, hazard ratio; CI, confidence interval; TNM, tumor node metastasis.
Machine learning-based model for the 2-year survival prediction
Construction of the machine-learning model
Considering that the median survival time of patients with PLCNEC in this study was only 17 months, and a significant decrease in survival rate was observed after 2 years, the 2-year survival status was finally selected as the target for machine learning prediction. This approach aims to enable early assessment and timely intervention by predicting patient outcomes through inputting the clinical characteristic data (such as age, sex, tumor stage, treatment, etc.) into the trained model. Furthermore, since all investigated clinical features are integral to clinical diagnosis and treatment, all features were incorporated into the training model. The performance of these 10 algorithms is presented in Table 4. For the test data set, SVM (0.848), Adaboost (0.799), GBDT (0.796), LR (0.793), and KNN (0.777) had the highest sensitivities, where LR (AUC =0.834), Adaboost (AUC =0.831), GBDT (AUC =0.831), KNN (AUC =0.822), and Catboost (AUC =0.793) were the most effective predictive models. The ROC curves of the 10 models are depicted in Figure 3A. The present study focused primarily on sensitivity in high-risk patients who may experience death after 2 years from tumor onset. Additionally, we aimed for the indicators used to evaluate each model’s results to not fall below an acceptable threshold. Although Adaboost and GBDT exhibited similar and highest sensitivity and AUC values, GBDT had superior performance in accuracy, precision, and F1-score, making it the preferred predictive model. Moreover, based on the importance scores assigned to each variable in the GBDT analysis (Figure 3B), it was found that surgery, AJCC-TNM classification, tumor stage, and chemotherapy were among the top six variables that significantly explained the 2-year survival status.
Table 4
Models | Accuracy | Precision | Sensitive | F1-score | AUC |
---|---|---|---|---|---|
Adaboost | 0.762 | 0.765 | 0.799 | 0.782 | 0.831 |
Catboost | 0.736 | 0.745 | 0.767 | 0.756 | 0.793 |
DT | 0.672 | 0.718 | 0.634 | 0.674 | 0.651 |
GBDT | 0.776 | 0.786 | 0.796 | 0.791 | 0.831 |
KNN | 0.772 | 0.792 | 0.777 | 0.784 | 0.822 |
LightGBM | 0.760 | 0.776 | 0.774 | 0.775 | 0.809 |
LR | 0.767 | 0.775 | 0.793 | 0.784 | 0.834 |
RF | 0.726 | 0.736 | 0.757 | 0.746 | 0.791 |
SVM | 0.757 | 0.736 | 0.848 | 0.788 | 0.792 |
XGboost | 0.709 | 0.732 | 0.715 | 0.723 | 0.756 |
DT, decision tree; GBDT, gradient boosting decision tree; KNN, k-nearest neighbor; LightGBM, light gradient boosting machine; LR, logistic regression; RF, random forest; SVM, support vector machine; AUC, area under the curve.
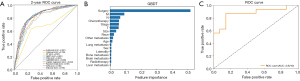
Validation of GBDT model
The ROC curve predicted by the trained GBDT on the external validation set is presented in Figure 3C. Next, the performance of the GBDT model in predicting the test set was evaluated using confusion curves, revealing an accuracy rate of 77.59% with correct predictions for the internal data set (Figure 4A) and 83.33% for the external data set (Figure 4B). To test the accuracy and stability of the prediction model, the confusion matrix and calibration curve were constructed for the internal and external validation cohorts, respectively. The horizontal and vertical coordinates of the calibration curve represent the predicted probability of the model and the actual target outcome, respectively. The closer the curve aligns with the diagonal line, the greater the proximity exists between the predicted outcome and actual observations. The results demonstrated that the prediction ability of GBDT remained stable and consistent across different data sets (Figure 4C,4D). DCA is a forthright approach to assess the clinical utility of predictive models, incorporating patient preferences or decision makers’ input into the analysis, whose x-axis represents the threshold probability of mortality and the y-axis represents the net benefit rate. In each plot, the horizontal dashed line denotes no deaths, and the intersecting solid line corresponds to different target outcomes where all patients experience mortality, and the curve above them illustrates the clinical decision curve of the prediction model at 2 years. As shown in Figure 4E,4F, each curve was predominantly distributed along the two reference lines, without any apparent intersection with them, underscoring the model’s great clinical utility for predicting survival outcomes among PLCNEC patients.
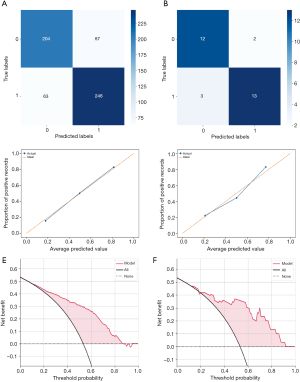
Discussion
PLCNEC is a rare disease characterized by high malignancy and unfavorable prognosis. The baseline demographic and clinical characteristics were obtained from 2,897 patients who were meticulously selected from a substantial number of PLCNEC cases in the SEER database. Multivariate Cox regression and survival analyses identified both favorable and risk factors that significantly impact the survival and prognosis of PLCNEC patients. Additionally, various machine learning algorithms were employed to train and test different models for predicting 2-year survival, with GBDT demonstrating the most promising results with a high AUC value, accuracy, precision, sensitivity, and F1 score.
Our data showed that male gender, age ≥65 years old, and the presence of bone, liver, or brain metastases were associated with a poorer prognosis. However, primary surgery and chemotherapy significantly improved patient outcomes. Consistent with previous reports, PLCNEC exhibited a higher incidence rate among males and old individuals, which may be associated with male smoking behavior and the duration of smoking (9,10). Due to the rarity of PLCNEC, the efficacy of early surgical intervention is yet to be fully validated with prospective studies. Nonetheless, existing research suggests that the extirpation of tumors with lobectomy or pneumonectomy is associated with improved OS (11), especially in early and locally advanced diseases (12,13). Barbara et al. found that after complete resection of LCNEC, half of the patients recurred mostly within the first 2 years of follow-up (14). Therefore, adjuvant chemotherapy is frequently utilized in clinical practice to postpone tumor recurrence post-surgery, and a plethora of data has also substantiated its efficacy; however, it may only be efficacious for patients with stage II–III, as no significant improvement has been reported for stage IA (14-16). Due to two major molecular types of PLCNEC (SCLC-RB1 and TP53 alterations and NSCLC-KRAS alteration and RB1 absence), the selection of its chemotherapy regimen remains controversial (7). Currently, its chemotherapy consists of either NSCLC-based regimen, such as pemetrexed, gemcitabine, and paclitaxel, or SCLC-based treatment that incorporates etoposide (16). Sun et al. reported a response rate of 73% when using SCLC regimens of cisplatin and etoposide, compared with a response of 50% with NSCLC regimens of pemetrexed, gefitinib, or erlotinib (17). However, a different study found that NSCLC-based treatment showed greater efficacy (18). Overall, the determination of the standard treatment for PLCNEC patients at different stages remains inconclusive, necessitating further large-scale prospective clinical studies in the future.
PLCNEC and SCLC are the two types of high-grade NE carcinomas of the lung with a poor prognosis, and the application of immune checkpoint inhibitors exerts a substantial and promising impact on the treatment outcomes of advanced NSCLC and SCLC. However, the scope of research on immunotherapy in PLCNEC remains limited. Tsuruoka et al. reported a significant programmed death-ligand 1 (PD-L1) expression in 10.4% of 106 PLCNEC patients and longer survival in those with positive PD-L1 expression than in those with negative expression (19). A multicenter retrospective cohort study demonstrated that the addition of pembrolizumab to first-line chemotherapy not only enhanced disease control rate (DCR) but also improved progression-free survival (PFS) and OS outcomes in patients with PLCNEC (20). Collectively, these findings imply a favorable impact of immunotherapy in PLCNEC (21). However, this study was excluded due to the unavailability of immunotherapy data in the SEER database. Furthermore, ongoing clinical trials investigating immunotherapy hold promise for advancing our understanding and management of this disease in the foreseeable future.
Given the infrequency and unfavorable prognosis of PLCNEC, it is imperative to establish a reliable strategy for predicting the prognosis of patients with this malignancy to facilitate early identification of appropriate treatment options. In recent years, machine learning has gained increasing prominence in clinical oncology for its ability to facilitate cancer diagnosis, predict patient outcomes, and inform treatment plans. This approach can automatically optimize the weighting of factors based on available data. Inputting the aforementioned characteristic data into the model enables the prediction of patient survival status after 2 years (22). Currently, machine learning methods are widely employed in predicting the survival status, therapeutic efficacy, and prognosis of lung cancer patients by utilizing common clinical features, imaging omics, and pathological data (23,24). This novel approach offers an additional auxiliary tool for personalized treatment and prognosis prediction in patients with PLCNEC. However, distinct machine learning algorithms have both advantages and disadvantages, with no single algorithm prevailing over others in all scenarios. Each algorithm performs differently across various data sets. Therefore, the selection of a machine learning algorithm should be based on its suitability for the specific data set rather than seeking a universal “best” algorithm. Furthermore, feature selection is crucial for artificial intelligence machine learning algorithms, as different feature selections directly impact the accuracy and generalization of the established model (25).
GBDT is widely recognized as a highly efficient machine learning model in predictive analytics. Its primary advantage lies in its ability to automatically uncover nonlinear interactions with minimality error through decision tree learning. Moreover, GBDT is often regarded as one of the top-performing out-of-the-box classifiers due to its exceptional generalization capabilities, enabling the combination of weak learners into a robust learner (26). The excellent performance in different data sets was demonstrated by ROC curves, calibration curves, and DCA, which may improve the current situation of risk assessment. Based on our statistics, surgery, AJCC-TNM classification, and chemotherapy were regarded as the most relevant variables to explain the 2-year survival status using the GBDT model. As previously stated, the prognosis of PLCNEC is significantly influenced by surgical intervention and chemotherapy. Besides, AJCC-developed and periodically reviewed lung cancer TNM classification is the internationally accepted standard for cancer staging. The T category defines the size and/or extent of the primary tumor, the N category defines the involvement of regional lymph nodes, and the M category indicates the presence of distant metastases, of which the grade reflects their severity (27). This classification informs management decisions and prognostication, facilitating consistent communication among clinicians, researchers, and patients.
However, this study has several limitations. Firstly, given that the vast majority of the patients included in the study were white (82.6%) and the existence of geographic variation in data collection, these findings cannot be generalized to other populations and geographic regions; however, follow-up analyses found no significant differences in disease outcomes. Secondly, the study was not comprehensive enough due to the lack of variables such as tumor-related test indicators, immunotherapy status, tumor mutational status, and chemotherapy regimens in the SEER database. Finally, the study lacked sufficient external validation data, necessitating the inclusion of data from patients diagnosed with PLCNEC at other institutions to enhance the reliability and robustness of the trained GBDT model.
Conclusions
In summary, the present study demonstrated that males, aged ≥65 years, with distant metastasis (such as bone, liver, and brain) exhibit a poorer prognosis of PLCNEC, while surgery and chemotherapy play a beneficial role in improving the prognosis. Additionally, the GBDT artificial intelligence algorithm was successfully employed to develop a predictive model for 2-year survival in patients with PLCNEC. This model can serve as a valuable reference for clinical diagnosis and treatment and it is anticipated to increasingly contribute to disease diagnosis, treatment, and prognosis assessment.
Acknowledgments
The authors would like to thank all the reviewers who participated in the review, as well as MJEditor (www.mjeditor.com) for providing English editing services during the preparation of this manuscript.
Funding: This work was supported by
Footnote
Reporting Checklist: The authors have completed the TRIPOD reporting checklist. Available at https://jtd.amegroups.com/article/view/10.21037/jtd-23-1927/rc
Data Sharing Statement: Available at https://jtd.amegroups.com/article/view/10.21037/jtd-23-1927/dss
Peer Review File: Available at https://jtd.amegroups.com/article/view/10.21037/jtd-23-1927/prf
Conflicts of Interest: All authors have completed the ICMJE uniform disclosure form (available at https://jtd.amegroups.com/article/view/10.21037/jtd-23-1927/coif). J.G. reports that this study was supported by the Science and Technology Program of Guangzhou (No. 202201010951). Y.Z. reports that this study was supported by the Natural Science Foundation of Guangdong Province (Nos. 2021A1515010480 and 2024A1515013043). The other authors have no conflicts of interest to declare.
Ethical Statement:
Open Access Statement: This is an Open Access article distributed in accordance with the Creative Commons Attribution-NonCommercial-NoDerivs 4.0 International License (CC BY-NC-ND 4.0), which permits the non-commercial replication and distribution of the article with the strict proviso that no changes or edits are made and the original work is properly cited (including links to both the formal publication through the relevant DOI and the license). See: https://creativecommons.org/licenses/by-nc-nd/4.0/.
References
- Li M, Yang L, Lu H. Pulmonary Combined Large Cell Neuroendocrine Carcinoma. Pathol Oncol Res 2022;28:1610747. [Crossref] [PubMed]
- Mengoli MC, Longo FR, Fraggetta F, et al. The 2015 World Health Organization Classification of lung tumors: new entities since the 2004 Classification. Pathologica 2018;110:39-67. [PubMed]
- Kinslow CJ, May MS, Saqi A, et al. Large-Cell Neuroendocrine Carcinoma of the Lung: A Population-Based Study. Clin Lung Cancer 2020;21:e99-e113. [Crossref] [PubMed]
- Rieber J, Schmitt J, Warth A, et al. Outcome and prognostic factors of multimodal therapy for pulmonary large-cell neuroendocrine carcinomas. Eur J Med Res 2015;20:64. [Crossref] [PubMed]
- Ferrara MG, Stefani A, Simbolo M, et al. Large Cell Neuro-Endocrine Carcinoma of the Lung: Current Treatment Options and Potential Future Opportunities. Front Oncol 2021;11:650293. [Crossref] [PubMed]
- Rekhtman N. Lung neuroendocrine neoplasms: recent progress and persistent challenges. Mod Pathol 2022;35:36-50. [Crossref] [PubMed]
- Atieh T, Huang CH. Treatment of Advanced-Stage Large Cell Neuroendocrine Cancer (LCNEC) of the Lung: A Tale of Two Diseases. Front Oncol 2021;11:667468. [Crossref] [PubMed]
- Greener JG, Kandathil SM, Moffat L, et al. A guide to machine learning for biologists. Nat Rev Mol Cell Biol 2022;23:40-55. [Crossref] [PubMed]
- Shen Y, Hu F, Li C, et al. Clinical Features and Outcomes Analysis of Surgical Resected Pulmonary Large-Cell Neuroendocrine Carcinoma With Adjuvant Chemotherapy. Front Oncol 2020;10:556194. [Crossref] [PubMed]
- Zhang JT, Li Y, Yan LX, et al. Disparity in clinical outcomes between pure and combined pulmonary large-cell neuroendocrine carcinoma: A multi-center retrospective study. Lung Cancer 2020;139:118-23. [Crossref] [PubMed]
- Peng K, Cao H, You Y, et al. Optimal Surgery Type and Adjuvant Therapy for T1N0M0 Lung Large Cell Neuroendocrine Carcinoma. Front Oncol 2021;11:591823. [Crossref] [PubMed]
- Kujtan L, Muthukumar V, Kennedy KF, et al. The Role of Systemic Therapy in the Management of Stage I Large Cell Neuroendocrine Carcinoma of the Lung. J Thorac Oncol 2018;13:707-14. [Crossref] [PubMed]
- Girelli L, Casiraghi M, Sandri A, et al. Results of Surgical Resection of Locally Advanced Pulmonary Neuroendocrine Tumors. Ann Thorac Surg 2021;112:405-14. [Crossref] [PubMed]
- Altieri B, La Salvia A, Modica R, et al. Recurrence-Free Survival in Early and Locally Advanced Large Cell Neuroendocrine Carcinoma of the Lung after Complete Tumor Resection. J Pers Med 2023;13:330. [Crossref] [PubMed]
- Raman V, Jawitz OK, Yang CJ, et al. Adjuvant Therapy for Patients With Early Large Cell Lung Neuroendocrine Cancer: A National Analysis. Ann Thorac Surg 2019;108:377-83. [Crossref] [PubMed]
- Chen H, Ishihara M, Horita N, et al. Effect of Adjuvant and Palliative Chemotherapy in Large Cell Neuroendocrine Carcinoma of the Lung: A Systematic Review and Meta-Analysis. Cancers (Basel) 2021;13:5948. [Crossref] [PubMed]
- Sun JM, Ahn MJ, Ahn JS, et al. Chemotherapy for pulmonary large cell neuroendocrine carcinoma: similar to that for small cell lung cancer or non-small cell lung cancer? Lung Cancer 2012;77:365-70. [Crossref] [PubMed]
- Derks JL, van Suylen RJ, Thunnissen E, et al. Chemotherapy for pulmonary large cell neuroendocrine carcinomas: does the regimen matter? Eur Respir J 2017;49:1601838. [Crossref] [PubMed]
- Tsuruoka K, Horinouchi H, Goto Y, et al. PD-L1 expression in neuroendocrine tumors of the lung. Lung Cancer 2017;108:115-20. [Crossref] [PubMed]
- Song L, Zhou F, Xu T, et al. Clinical activity of pembrolizumab with or without chemotherapy in advanced pulmonary large-cell and large-cell neuroendocrine carcinomas: a multicenter retrospective cohort study. BMC Cancer 2023;23:443. [Crossref] [PubMed]
- Komiya T, Ravindra N, Powell E. Role of Immunotherapy in Stage IV Large Cell Neuroendocrine Carcinoma of the Lung. Asian Pac J Cancer Prev 2021;22:365-70. [Crossref] [PubMed]
- Swanson K, Wu E, Zhang A, et al. From patterns to patients: Advances in clinical machine learning for cancer diagnosis, prognosis, and treatment. Cell 2023;186:1772-91. [Crossref] [PubMed]
- Gao Q, Yang L, Lu M, et al. The artificial intelligence and machine learning in lung cancer immunotherapy. J Hematol Oncol 2023;16:55. [Crossref] [PubMed]
- Chen NB, Xiong M, Zhou R, et al. CT radiomics-based long-term survival prediction for locally advanced non-small cell lung cancer patients treated with concurrent chemoradiotherapy using features from tumor and tumor organismal environment. Radiat Oncol 2022;17:184. [Crossref] [PubMed]
- Williamson BD, Gilbert PB, Carone M, et al. Nonparametric variable importance assessment using machine learning techniques. Biometrics 2021;77:9-22. [Crossref] [PubMed]
- Liao Z, Huang Y, Yue X, et al. In Silico Prediction of Gamma-Aminobutyric Acid Type-A Receptors Using Novel Machine-Learning-Based SVM and GBDT Approaches. Biomed Res Int 2016;2016:2375268. [Crossref] [PubMed]
- Kutob L, Schneider F. Lung Cancer Staging. Surg Pathol Clin 2020;13:57-71. [Crossref] [PubMed]