Clinical characteristics and microbial signatures in the lower airways of diabetic and nondiabetic patients with pneumonia
Highlight box
Key findings
• We identified the microbial signatures in the lower airways and the association between clinical characteristics and severe pneumonia.
What is known and what is new?
• It is well known that diabetes mellitus (DM) affects the host immune response, and is strongly associated with systemic inflammation and oxidative stress, making individuals with DM more susceptible to lung disease including pneumonia.
• We compared the clinical and microbial characteristics of pneumonia patients with or without diabetes, and then performed a subgroup analysis of severe pneumonia in the two groups. Finally, we proposed a risk model of severe pneumonia using combined clinical and microbial markers, in which diabetes was also a key factor for early warning of severe pneumonia.
What is the implication, and what should change now?
• We proposed a risk model of severe pneumonia using combined clinical and microbial markers, in which diabetes was also a key factor for early warning of severe pneumonia.
Introduction
Diabetes mellitus (DM) is a metabolic disease characterized by chronic hyperglycemia. In recent years, the incidence and prevalence of DM have increased worldwide. Especially since early 2020, coronavirus disease 2019 (COVID-19) remains prevalent and carries metabolic sequelae increasing incident diabetes (1,2). About 537 million adults in the world suffer from diabetes (1 in 10 people). It is estimated that by 2045, the number will rise to 783 million (3).
Respiratory tract infection is a common infectious disease among diabetic patients, as pneumonia accounts for 26% of hospitalized patients with diabetes and 8% of the direct cause of death in the late stage of diabetes (4,5). There have been many studies on the etiology of diabetes complicated with pneumonia (6,7). But they routinely employed culture-based techniques to identify lung pathogens. The approach of using a selective medium for specific pathogens is bound to bias towards known, previously encountered pathogens whereas novel, slow-growing or rare microorganisms may be left out (8). Molecular detection methods mostly rely on known sequences of pathogen nucleic acids or antigen-antibody reactions (9,10). These traditional microbial detection methods have been unable to fully meet the needs of research. Therefore, the next-generation sequencing (NGS) technology with higher throughput, faster speed and lower cost has emerged and aroused our interest. The composition of pulmonary microbes is more closely related to the immunological state of the lungs (11). Pneumonia ecological models believe that the pulmonary microbiome rapidly changes from a homeostatic state to an ecological imbalance characterized by low microbial diversity, high microbial burden and host inflammation (12). It is well known that DM can affect the host immune response (13), and is strongly associated with systemic inflammation and oxidative stress, making individuals more susceptible to lung disease including pneumonia in DM patients (14,15). It is speculated that diabetes may also indirectly affect the pulmonary microbiome, so it is meaningful to study the changes of pulmonary microbiome composition in diabetes patients with pneumonia.
Some studies suggested that patients with diabetes had a higher risk of developing severe pneumonia (SP) (16-18). CURB-65 (confusion, urea, respiratory rate, blood pressure, age >65 years) and pneumonia severity index (PSI) are only suitable for assessing the general pneumonia population. The pneumonia prediction performance of diabetic patients is poor, and the area under the curve (AUC) is only 0.655–0.727 (19,20). Therefore, exploring the correlation between lower respiratory tract microbial characteristics, clinical characteristics and SP, and constructing an effective combined biomarker early risk model for predicting SP may be beneficial to improve the prognosis of pneumonia patients, especially those with diabetes.
In this study, we compared the clinical and microbial characteristics of pneumonia patients with or without diabetes, and then performed a subgroup analysis of SP in the two groups. Finally, we proposed a risk model of SP using combined clinical and microbial markers, in which diabetes was also a key factor for early warning of SP. We present this article in accordance with the STROBE reporting checklist (available at https://jtd.amegroups.com/article/view/10.21037/jtd-24-490/rc).
Methods
Study participants and data collection
From January 2018 to May 2021, 273 hospitalized adult patients with pneumonia at Ruijin hospital Affiliated to Shanghai Jiaotong University School of Medicine were consecutively recruited for this study. The study was conducted in accordance with the Declaration of Helsinki (as revised in 2013) and Good Clinical Practice Guidelines. This study was approved by the Ruijin Hospital Ethics Committee in Shanghai (No. 2017-205). All patients gave written informed consent. Patients were included according to the following inclusion criteria: (I) age ≥18 years; (II) clear diagnosis of pneumonia according to the Infectious Diseases Society of American (IDSA)/American Thoracic Society (ATS) guideline (21); and (III) complete baseline information available. Patients with a history of cancer or with an autoimmune disease, or those receiving intravenous steroids or immunosuppressant treatment or were excluded. According to past history or admission laboratory tests, pneumonia patients were divided into two groups: DM group and non-DM (NDM) group (Figure 1). Patients in the DM group included those with a clear history of type 2 diabetes mellitus (T2DM) and those newly diagnosed with T2DM based on laboratory tests at admission according to the American Diabetes Association (ADA) guidelines (22). The patients who met the diagnostic criteria for SP of the IDSA/ATS were divided into SP group (21), and the rest were mild pneumonia (MP) group. Figure 1 showed the flow chart of this study. The differences in pneumonia severities, pathogen profiles, and clinical characteristics between patients with and without DM were first compared. Further, the bronchoalveolar lavage fluid (BALF) samples from 177 patients which consisted of 137 patients without DM and 40 patients with DM, were sequenced using metagenomic NGS (mNGS), and then the distinction in BALF microbial diversity and copy number variations (CNVs) in human genomes were analyzed between patients with and without DM. The reasons why the patients underwent bronchoscopy were explained in Appendix 1. Moreover, the model based on different kinds of signatures such as diabetes, were constructed for predicting pneumonia severity. The clinical data were collected at the time of admission including demographic data, complications, main symptoms, vital signs and laboratory tests (hematological data, biochemical parameters, inflammatory markers, blood coagulation indicators, etc.).
Sample collection and DNA extraction
BALF sample was collected from each patient and a 5 mL BALF sample was placed in a sterile container and inactivated at 65 ℃ for 30 minutes. Then 500 µL sample and 1 g 0.5 mm glass beads were mixed in a 1.5 mL microcentrifuge tube on a vortex mixer and agitated vigorously at 2,800–3,200 rpm for 30 min. Then 300 µL of the supernatant was mixed with 0.2 ng of internal DNA control in another 1.5 mL microcentrifuge tube for DNA extraction. Extraction of DNA from the BALF sample was performed using the TIANamp Micro DNA Kit (DP316, Tiangen Biotech, Beijing, China) following the manufacturer’s instructions. Extracted DNA was applied for further DNA library construction.
Library preparation and sequencing
The DNA library was constructed through several steps including DNA fragmentation, end-repair, adapter-ligation and polymerase chain reaction (PCR) amplification. The quality of the constructed DNA library was evaluated by Agilent 2100 (Agilent Technologies, Santa Clara, CA, USA) and Qubit 2.0 (Invitrogen, Waltham, MA, USA). Based on the qualified double-strand DNA library, the single-stranded circular DNA library was further generated through DNA denaturation and circularization. Then DNA nanoballs (DNBs) were produced through rolling circle amplification (RCA). The qualities of DNBs were assessed by Qubit 2.0 (Invitrogen). Qualified DNBs were then sequenced on the MGISEQ-2000 platform (MGI, Shenzhen, China).
Analysis of microbiome and CNVs
DNA-seq raw data were first filtered by fastp, and then the filtered reads were aligned to the human genome (GRCh38) using HISAT2 (2.2.1 release) to remove human sequences. Based on the remained microbial DNA clean reads, the identifications of microbial species were conducted using Kraken2. Principal component analysis (PCA) was carried out with the ade4 R package. Microbial diversity Shannon index was analyzed by the vegan package in R v4.0.3. LDA effect size (LEfSe) analysis was performed to compare differences in microbial operational taxonomic units between different patient groups to explore microbial biomarkers. Figures (Figures 2-4 and Figures S1,S2) including the PCA plots, box plots and heatmap were created through the ggplot2 package in R v4.0.3. The filtered DNA clean reads were aligned to the human genome (GRCh38) by using BWA (version: 0.7.17-r1188) (23) and further marked duplications by sambamba (version: 0.7.1). CNV in the human genome were detected by CNVkit (version 0.9.6.dev0) (24). The statistical significance was assessed by Fisher’s test.
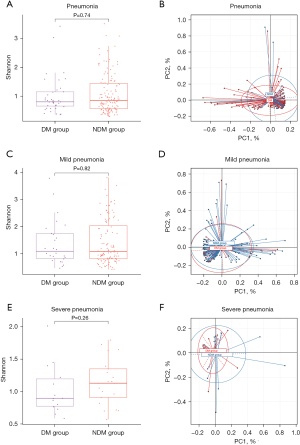
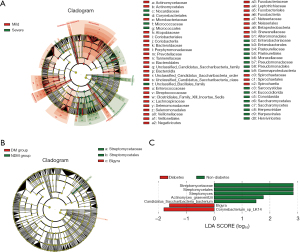
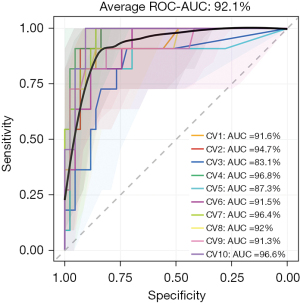
Model construction
Several random forest (RF) models were built to distinguish SP cases from MP. In total, 177 cases were used, and the dataset was split into two sets including a training set and a testing set according to 7:3 ratio to train or test the model respectively. Firstly, least absolute shrinkage and selection operator (LASSO) regression was applied to all the cases to identify the most important markers from the less significant ones to prevent model overfitting. Markers were considered significant if they had a LASSO importance value greater than zero. Then the selected markers were used to build RF models for predicting SP. To test the predictive power of the RF models built, the AUC of the receiver operating characteristic (ROC) curve was calculated. The model prediction was performed ten times, and at each time the dataset was randomly split according to the 8:2 ratio. Therefore, model parameters may be different at each round of model training, thus ROC-AUC values obtained from ten rounds were averaged. Proper sensitivity and specificity values were also decided from the plotted ROC curves. Codes were written in R, and both LASSO regression and RF models were built using R caret package.
Statistical analyses
The difference in the ratio of patients with SP in diabetic and nondiabetic patients, the positive detection rate of pathogens, the preference in pathogen categories detected, and the dependences of pneumonia severity on pathogen categories, between diabetic and nondiabetic patients were examined by Fisher’s exact test. The pathogen profiles between DM and NDM groups were tested by the analysis of variance (ANOVA). Differences between groups in PCA plot were examined by pairwise permutational multivariate ANOVA (PERMANOVA). Comparisons of microbial diversity and abundance between two groups were assessed by Wilcoxon rank sum test (25). Correlations between microbial taxa and clinical characteristics or pneumonia severity were tested using Spearman correlation analysis. The spearman correlation coefficient (rho) >0.6 was regarded as a strong correlation between the independent variable and dependent variable.
Results
The baseline information and clinical characteristics of pneumonia patients with or without DM
Demographics, clinical characteristics and laboratory examinations of 273 patients with pneumonia were analyzed and summarized (Table 1). Patients in DM group were older than those in NDM group (P<0.001). DM group had a higher proportion of men and longer smoking history than the NDM group (P=0.03; P=0.03), with obvious differences. The body mass index (BMI) of pneumonia patients with DM was higher than that of patients without diabetes (P=0.001). The DM group had a higher proportion of patients admitted to intensive care unit (ICU) and receiving mechanical ventilation (P=0.01); 145 (53.11%) patients had complications. There were 20 SP patients (32.79%) in DM group and 31 SP patients (14.62%) in NDM group. The rate of SP in DM group was higher than NDM group (P=0.003). Compared with NDM group, DM group had a higher prevalence of hypertension (P<0.001), coronary heart disease (CHD) (P=0.07) and cerebral infarction (P=0.07). C-reactive protein (CRP) and urine protein were higher and albumin was lower in DM group compared with NDM group (P=0.008; P=0.003; P=0.002). There was no significant difference in the other 15 lab features between the two groups. However, we revealed fifteen clinical indicators [lymphocyte count, albumin, eosinophil count, calcium, D-dimer, CRP, CD3, CD4, CD8, complement 3 (C3), tumor necrosis factor (TNF), urinary protein, lactate dehydrogenase (LDH), myoglobin, procalcitonin (PCT)] correlated to SP in diabetic patients (Table S1).
Table 1
Characteristics | DM (n=61) | NDM (n=212) | P value |
---|---|---|---|
Socio-demographic details | |||
Age, years | 63.90±11.81 | 54.17±16.31 | <0.001* |
≥60 | 70.59±6.65 | 68.99±6.66 | 0.20 |
Male sex | 41 (67.21) | 110 (51.89) | 0.03* |
Smoking | 23 (37.70) | 49 (23.11) | 0.03* |
BMI, kg/m2 | 23.23±3.28 | 21.47±3.50 | 0.001* |
Severe cases | 20 (32.79) | 31 (14.62) | 0.003* |
Admission to ICU | 8 (13.11) | 8 (3.77) | 0.01* |
Mechanical ventilation | 8 (13.11) | 8 (3.77) | 0.01* |
Comorbidity | |||
Hypertension | 33 (54.10) | 45 (21.23) | <0.001* |
Asthma | 0 | 7 (3.30) | 0.36 |
COPD | 1 (1.64) | 5 (2.36) | >0.99 |
Coronary heart disease | 7 (11.48) | 9 (4.25) | 0.07 |
Cerebral infarction | 7 (11.48) | 9 (4.25) | 0.07 |
Clinical characteristics | |||
CRP, mg/L | 78.08±85.75 | 43.68±69.19 | 0.008* |
≥10 | 38 | 85 | 0.005* |
Lymphocyte count, ×109/L |
1.28±0.72 | 1.46±0.64 | 0.06 |
>4 | 16 | 31 | 0.05 |
Eosinophil count, ×109/L | 0.36±1.15 | 0.24±0.67 | 0.30 |
>0.5 | 21 | 41 | 0.03* |
Urine protein | 0.51±0.77 | 0.16±0.47 | 0.003* |
++++~ | 19 | 19 | <0.001* |
Albumin, g/L | 33.70±7.74 | 37.32±7.85 | 0.002* |
>55 | 32 | 75 | 0.03* |
Calcium, mmol/L | 2.20±0.19 | 2.25±0.24 | 0.10 |
>2.75 | 9 | 18 | 0.22 |
LDH, U/L | 241.45±161.90 | 207.46±115.23 | 0.16 |
>192 | 25 | 47 | 0.04* |
Myoglobin, ng/mL | 48.13±78.06 | 66.10±321.33 | 0.69 |
≥70 | 7 | 13 | 0.42 |
PCT, μg/L | 1.33±4.77 | 0.34±1.07 | 0.14 |
≥0.5 | 111 | 15 | 0.10 |
D-dimer, mg/L | 2.77±6.47 | 1.53±4.02 | 0.07 |
≥0.55 | 37 | 77 | 0.001* |
CD3+ T cell, /μL | 887.56±636.85 | 986.96±538.95 | 0.43 |
≥2,368 | 13 | 30 | 0.36 |
CD4+ T cell, /μL | 516.37±423.62 | 602.32±361.08 | 0.31 |
≥1,346 | 13 | 30 | 0.36 |
CD8+ T cell, /μL | 339.70±232.40 | 346.22±215.34 | 0.89 |
≥1,110 | 8 | 25 | >0.99 |
C3, pg/dL | 101.40±23.94 | 106.71±25.59 | 0.41 |
>152 | 4 | 8 | 0.46 |
TNF, pg/mL | 22.51±15.86 | 20.72±43.67 | 0.88 |
≥8.1 | 14 | 20 | 0.03* |
IL-1β, pg/mL | 8.65±11.65 | 9.49±12.84 | 0.82 |
C4, g/L | 28.05±8.64 | 27.96±9.94 | 0.97 |
Pro-BNP, pg/mL | 679.1±1,409.75 | 344.0±1,334.03 | 0.16 |
Data are presented as mean ± SD or n (%) by applying Fisher’s exact test or Wilcoxon rank sum test. Continuous variables were analyzed using Student’s t-test or Mann-Whitney U test as appropriate. “++++~” means that the qualitative value of urine protein is greater than or equal to ++++. *, statistical significance (P<0.05). DM, diabetes mellitus; NDM, non-diabetes mellitus; BMI, body mass index; ICU, intensive care unit; COPD, chronic obstructive pulmonary disease; CRP, C-reactive protein; LDH, lactate dehydrogenase; PCT, procalcitonin; C3, complement 3; TNF, tumor necrosis factor; IL-1β, interleukin-1β; C4, complement 4; Pro-BNP, pro-brain natriuretic peptide.
Pathogens of lung infection in DM and NDM patients with pneumonia
We first analyzed the pneumonia pathogens identified based on traditional culture, PCR methods, and serological detection. In this study, the pathogens were mainly divided into five categories: bacteria, fungi, Mycobacterium tuberculosis, mixed infection and undetermined pathogens. Viral infection was excluded from our pathogen classifications since only one sample was positive for influenza virus A. There was no significant difference between DM and NDM in the positive rate of pathogens detected in pulmonary (P=0.19). However, compared with the NDM group, DM group had a higher rate of mixed infection (P=0.02) (Table 2). There were significant differences in the categories of pathogens detected between the two groups (P<0.001) (Figure S1). A greater variety of pathogens were detected in the NDM group compared with DM group. The top 3 common pathogens detected in pneumonia patients with DM were Mycobacterium tuberculosis (18.0%), Klebsiella pneumoniae (11.5%), Candida albicans (8.2%), and Candida tropicalis (8.2%). The top 5 common pathogens detected in NDM group were Mycobacterium tuberculosis (13.2%), Candida albicans (6.1%), Klebsiella pneumoniae (4.2%), Acinetobacter baumannii (4.2%), Stenotrophomonas maltophilia (4.2%). Enterococcus faecium, Viridans streptococci, Cryptococcus, etc., were detected in NDM group, but not in DM group.
Table 2
Pathogenic types | DM (n=61) | NDM (n=212) | P value |
---|---|---|---|
Bacteria | 10 | 29 | 0.68 |
Fungi | 5 | 17 | >0.99 |
Mycobacterium tuberculosis | 7 | 28 | 0.83 |
Mixed | 9 | 11 | 0.02* |
Undetermined | 30 | 127 | 0.21 |
Data are presented by applying Fisher’s exact test or Pearson Chi-squared test. *, statistical significance (P<0.05). DM, diabetes mellitus; NDM, non-diabetes mellitus.
The relation between BALF microbiome and pneumonia severity in DM patients
Further, to explore associations of BALF microbiome and pneumonia severity in diabetic patients, the BALF samples from 177 patients which consisted of 137 patients without DM and 40 patients with DM, were sequenced using mNGS, and then BALF microbial diversity was analyzed between patients with and without DM and between patients with severe and MP. The clinical characteristics of 177 patients whose BALF samples were used for further analysis were showed in Table S2. BALF microbial alpha- and beta-diversity were compared between DM group and NDM group. The results showed that there was no significant difference in the both groups (Figure 2A,2B). However, the NDM group had a richer variety of microorganisms than the diabetic patients. There were 178 significantly differentially abundant microbial species between DM and NDM (Figure S2A). Then we performed a subgroup analysis based on the severity of pneumonia. There was no significant difference in BALF microbial alpha- and beta-diversity between SP and MP in diabetic and non-diabetic patients (Figure 2C-2F). There were 66 and 232 significantly differentially abundant microbial species between SP and MP in diabetic patients, and between SP and MP in non-diabetic patients, respectively (Figure S2B,S2C). We found that 57 microbial species were common differential microbes by comparing the differentially abundant microbes characterized in the two comparisons, no matter in diabetic patients or non-diabetic patients (Figure S3 and Table S3). Interestingly, nine microbial species were found to be differently abundant (P<0.05) in diabetic patients with SP compared to those with MP, while not between SP and MP in patients without diabetes.
In addition, we found more stringent biomarkers for pneumonia severity and diabetes. Bacteroidetes (mainly including Bacteroides, Flavobacteria), Betaproteobacteria and Fusobacteria were enriched in MP, while Peploviricota (Herpesviridae), Ascomycota (Yeasts), Apicomplexa (Sarcocystidae), Gamaproteo bacteria, (mainly including Enterobacteriaceae, Pasteuriaceae, Moraceae, Pseudomonas), Micrococcales and Corynebacilli (Nocardia) in SP (Figure 3A). Streptomycetales were more abundant in NDM compared to DM, while Bigyra was just the opposite (Figure 3B,3C).
The association of BALF microbiome and CNVs in DM patients with SP
Moreover, we analyzed the correlation between laboratory examinations related to SP and BALF respiratory microbiome. The results indicated that some microbes were closely related to urine protein, C3 and TNF screened out from the indicators relating to SP in DM patients. Among them, Porphyromonas endodontalis was negatively correlated with urine protein and positively correlated with C3. In addition, 16 microbes were found to be related to TNF (Table S4).
Subsequently, we explored the human genome of different groups using CNVs and found that CNVs had no relevance to the pneumonia severity in DM group. The CNVs in DM group mainly occurred on chromosomes 8 and 15. According to the gene locus, the main function of the location of the CNV gene is to regulate miRNAs and β-defensins relating to immune response, no CNV is significantly related to SP among patients with DM (available online: https://cdn.amegroups.cn/static/public/jtd-24-490-1.xlsx).
Prediction model of SP with combined markers
We found that the rate of SP in DM group was higher than NDM group and adding diabetes as an important marker to predict SP can improve the accuracy. Based on the previous research results, we attempt to establish a model using RF to predict pneumonia severity to identify patients early and improve prognosis. The selected model parameters included microbial biomarkers (Staphylococcus haemolyticus and Candida tropicalis), clinical characteristics [N-terminal pro-brain natriuretic peptide (BNP), PCT, myoglobin, D-dimer, isocitrate dehydrogenase, calcium ion] and whether patients suffered with diabetes or not. In total, 177 cases were used, and the dataset was split into two sets including a training set and a testing set according to the 7:3 ratio to train or test the model respectively. The model prediction was performed 10 times, and the averaged ROC-AUC value, specificity and sensitivity of this model were 92.1%, 82% and 91%, respectively (Figure 4).
Discussion
Our study is the first one to compare the lower respiratory microbes of pneumonia patients with or without diabetes in a relatively large cohort using NGS technology. Besides, we innovatively put forward a model to predict SP in patients by combining the microbial signatures with clinical characteristics and found the key microbial characteristics of the lower airways in pneumonia patients with diabetes, especially those with SP, which is of great significance to the treatment and prognosis of patients.
This study demonstrates that diabetic patients had a higher proportion of SP compared to NDM group. A previous study showed the hospitalization rate due to infections in diabetic patients was twice of those without diabetes, and the former were at increased risk of developing serious infections (26). Patients without known DM but their admission HbA1c ≥48 mmol/mol would have a greater rate (19.8% vs. 6.3%, P<0.001) of moderate to SP compared to patients without DM (27). These reports are in accordance with our findings and there is an association between DM and the development of more SP or poor outcomes in patients.
Patients with diabetes usually have a higher rate of respiratory infections by non-classic pathogens, such as gram-negative bacilli, Mycobacterium tuberculosis and Nocardia, which are associated with poor prognosis (28). In our cohort, the pathogenic diversities were significantly different between DM and NDM. The common pathogens detected in diabetic patients were Mycobacterium tuberculosis, Klebsiella pneumoniae, Candida albicans. Several studies suggested that categories of infectious pathogens are associated with adverse outcomes in diabetic patients (29-32). Pneumonia due to gram-negative bacilli was significantly more common in diabetic patients who died compared with those who survived (29). S. aureus infections were more common in dead patients than in survivors regardless of whether the patients had diabetes or not, but the incidence of S. aureus infection was relatively low in both groups (32). It may be that unique pathogens have different pathogenicity and virulence under the state of decreased anti-infection ability in diabetes.
Our results showed that 15 clinical features were related to SP in diabetic patients and some microbes were associated with the clinical characteristics of patients. Among them, lymphocyte count, CRP, urinary protein and myoglobin were significantly different between DM and NDM (P<0.05). The results are consistent with the findings of some previous studies (33-35). It indicates that some microbes are likely related to SP or poor prognosis in patients with diabetes. For example, Porphyromonas endodontalis was a differential microbe between MP and SP in DM group, with a higher abundance in MP. Besides, it was negatively correlated with urinary protein level and positively correlated with C3. C3 was sensitive to the limited proteolysis of arginine-specific cysteine protease isolated from Porphyromonas gingivalis (36), indicating that the bacteria may affect pneumonia in diabetic patients by involving the activation of C3. Furthermore, some researchers reported that the expression of TNF was related to SP (37), which suggested 16 microbes might affect the expression of TNF and indirectly affect pneumonia.
We did not find the different CNVs between SP and MP for diabetic patients but some researchers found that 7 of 26 intragenic tandem repeat sequences were “polymorphic” in terms of repeat copy number between a large number of L. pneumophila serogroup 1 strains in 2008 (38). There are few studies on the association between CNVs and SP.
CURB-65 and PSI are only applicable to the general population. Several researchers proposed the APUA (age, pulse, urea and albumin) model to predict in-hospital mortality of community-acquired pneumonia (CAP), adapted for patients with type 2 diabetes and Cheng et al. constructed a prediction score based on nine independent predictors of in-hospital mortality in diabetic patients (33,39). Pneumonia in diabetic patients predictive index was the first predictive tool specifically aiming at the prediction of the risk of pneumonia among diabetic patients (40). But there is still a lack of clinical prediction scores for mild and SP in diabetic patients (41,42). We constructed a risk model for predicting SP combining clinical characteristics, microbial biomarkers and diabetes. Some of these factors have been confirmed to be associated with worsening pneumonia and the occurrence of critical diseases (43-45). The combination of BNP and PSI significantly improved the efficiency of prediction and treatment failure in CAP than PSI alone (AUC 0.78 vs. 0.71; P=0.02) (43). The levels of inflammation such as D-dimer in diabetic patients were higher compared with nondiabetic patients (P<0.01), indicating the former was more prone to the inflammatory storm, resulting in the exacerbation of pneumonia (44).
We compared the lower respiratory microbes of pneumonia patients with or without diabetes in a relatively large cohort using NGS technology. Certainly, this study also has limitations. Firstly, the sample size of patients with diabetes is limited. Secondly, patients are not undergoing tracheoscopy continuously in our hospital, our study does not track the disease progress. Thirdly, our model lacks a larger validation set due to the limitation of sample size. Fourthly, most of the patients analyzed in our study were complex patients with poor response to empirical antibiotic treatment and those who need to identify the pathogen by NGS. They were not CAP patients evenly distributed in society, so there was a certain degree of admission bias.
Conclusions
In conclusion, we identified the microbial signatures in the lower airways and the association with clinical characteristics and SP. The risk model was more accurate in predicting SP, which might be beneficial to the early identification and management of patients with SP.
Acknowledgments
Funding: This work was supported by a Grant from
Footnote
Reporting Checklist: The authors have completed the STROBE reporting checklist. Available at https://jtd.amegroups.com/article/view/10.21037/jtd-24-490/rc
Data Sharing Statement: Available at https://jtd.amegroups.com/article/view/10.21037/jtd-24-490/dss
Peer Review File: Available at https://jtd.amegroups.com/article/view/10.21037/jtd-24-490/prf
Conflicts of Interest: All authors have completed the ICMJE uniform disclosure form (available at https://jtd.amegroups.com/article/view/10.21037/jtd-24-490/coif). X.H., Jiumeng Min, R.S.Y.Y., Z.C., and Jinmin Ma are current employees of BGI PathoGenesis Pharmaceutical Technology company. The other authors have no conflicts of interest to declare.
Ethical Statement: The authors are accountable for all aspects of the work in ensuring that questions related to the accuracy or integrity of any part of the work are appropriately investigated and resolved. The study was conducted in accordance with the Declaration of Helsinki (as revised in 2013) and the Good Clinical Practice Guidelines. This study was approved by the Ruijin Hospital Ethics Committee in Shanghai (No. 2017-205). All patients gave written informed consent.
Open Access Statement: This is an Open Access article distributed in accordance with the Creative Commons Attribution-NonCommercial-NoDerivs 4.0 International License (CC BY-NC-ND 4.0), which permits the non-commercial replication and distribution of the article with the strict proviso that no changes or edits are made and the original work is properly cited (including links to both the formal publication through the relevant DOI and the license). See: https://creativecommons.org/licenses/by-nc-nd/4.0/.
References
- Conte C, Cipponeri E, Roden M. Diabetes Mellitus, Energy Metabolism, and COVID-19. Endocr Rev 2024;45:281-308. [Crossref] [PubMed]
- Schlesinger S, Lang A, Christodoulou N, et al. Risk phenotypes of diabetes and association with COVID-19 severity and death: an update of a living systematic review and meta-analysis. Diabetologia 2023;66:1395-412. [Crossref] [PubMed]
- Sun H, Saeedi P, Karuranga S, et al. IDF Diabetes Atlas: Global, regional and country-level diabetes prevalence estimates for 2021 and projections for 2045. Diabetes Res Clin Pract 2022;183:109119. [Crossref] [PubMed]
- Koskela HO, Salonen PH, Romppanen J, et al. Long-term mortality after community-acquired pneumonia--impacts of diabetes and newly discovered hyperglycaemia: a prospective, observational cohort study. BMJ Open 2014;4:e005715. [Crossref] [PubMed]
- Lin W, Chen C, Guan H, et al. Hospitalization of elderly diabetic patients: characteristics, reasons for admission, and gender differences. BMC Geriatr 2016;16:160. [Crossref] [PubMed]
- Akbar DH. Bacterial pneumonia: comparison between diabetics and non-diabetics. Acta Diabetol 2001;38:77-82. [Crossref] [PubMed]
- Saibal MA, Rahman SH, Nishat L, et al. Community acquired pneumonia in diabetic and non-diabetic hospitalized patients: presentation, causative pathogens and outcome. Bangladesh Med Res Counc Bull 2012;38:98-103. [Crossref] [PubMed]
- Feigelman R, Kahlert CR, Baty F, et al. Sputum DNA sequencing in cystic fibrosis: non-invasive access to the lung microbiome and to pathogen details. Microbiome 2017;5:20. [Crossref] [PubMed]
- Burillo A, Bouza E. Use of rapid diagnostic techniques in ICU patients with infections. BMC Infect Dis 2014;14:593. [Crossref] [PubMed]
- Kostrzewa M, Sparbier K, Maier T, et al. MALDI-TOF MS: an upcoming tool for rapid detection of antibiotic resistance in microorganisms. Proteomics Clin Appl 2013;7:767-78. [Crossref] [PubMed]
- Dickson RP, Erb-Downward JR, Falkowski NR, et al. The Lung Microbiota of Healthy Mice Are Highly Variable, Cluster by Environment, and Reflect Variation in Baseline Lung Innate Immunity. Am J Respir Crit Care Med 2018;198:497-508. [Crossref] [PubMed]
- Dickson RP, Erb-Downward JR, Freeman CM, et al. Bacterial Topography of the Healthy Human Lower Respiratory Tract. mBio 2017;8:e02287-16. [Crossref] [PubMed]
- Bobadilla-Del-Valle M, Leal-Vega F, Torres-Gonzalez P, et al. Mycobacterial Growth Inhibition Assay (MGIA) as a Host Directed Diagnostic Tool for the Evaluation of the Immune Response in Subjects Living With Type 2 Diabetes Mellitus. Front Cell Infect Microbiol 2021;11:640707. [Crossref] [PubMed]
- Ehrlich SF, Quesenberry CP Jr, Van Den Eeden SK, et al. Patients diagnosed with diabetes are at increased risk for asthma, chronic obstructive pulmonary disease, pulmonary fibrosis, and pneumonia but not lung cancer. Diabetes Care 2010;33:55-60. [Crossref] [PubMed]
- Jagadapillai R, Rane MJ, Lin X, et al. Diabetic Microvascular Disease and Pulmonary Fibrosis: The Contribution of Platelets and Systemic Inflammation. Int J Mol Sci 2016;17:1853. [Crossref] [PubMed]
- Cao P, Song Y, Zhuang Z, et al. Obesity and COVID-19 in Adult Patients With Diabetes. Diabetes 2021;70:1061-9. [Crossref] [PubMed]
- Li S, Wang J, Zhang B, et al. Diabetes Mellitus and Cause-Specific Mortality: A Population-Based Study. Diabetes Metab J 2019;43:319-41. [Crossref] [PubMed]
- Zúñiga J, Buendía-Roldán I, Zhao Y, et al. Genetic variants associated with severe pneumonia in A/H1N1 influenza infection. Eur Respir J 2012;39:604-10. [Crossref] [PubMed]
- Chen PC, Liao WI, Wang YC, et al. An Elevated Glycemic Gap is Associated With Adverse Outcomes in Diabetic Patients With Community-Acquired Pneumonia. Medicine (Baltimore) 2015;94:e1456. [Crossref] [PubMed]
- Ma CM, Wang N, Su QW, et al. Age, Pulse, Urea and Albumin (APUA) Model: A Tool for Predicting in-Hospital Mortality of Community-Acquired Pneumonia Adapted for Patients with Type 2 Diabetes. Diabetes Metab Syndr Obes 2020;13:3617-26. [Crossref] [PubMed]
- Mandell LA, Wunderink RG, Anzueto A, et al. Infectious Diseases Society of America/American Thoracic Society consensus guidelines on the management of community-acquired pneumonia in adults. Clin Infect Dis 2007;44:S27-72. [Crossref] [PubMed]
- American Diabetes Association. 2. Classification and Diagnosis of Diabetes: Standards of Medical Care in Diabetes-2019. Diabetes Care 2019;42:S13-S28. [Crossref] [PubMed]
- Li H, Durbin R. Fast and accurate long-read alignment with Burrows-Wheeler transform. Bioinformatics 2010;26:589-95. [Crossref] [PubMed]
- Talevich E, Shain AH, Botton T, et al. CNVkit: Genome-Wide Copy Number Detection and Visualization from Targeted DNA Sequencing. PLoS Comput Biol 2016;12:e1004873. [Crossref] [PubMed]
- Dutta S, Datta S. A rank-sum test for clustered data when the number of subjects in a group within a cluster is informative. Biometrics 2016;72:432-40. [Crossref] [PubMed]
- Hamilton EJ, Martin N, Makepeace A, et al. Incidence and predictors of hospitalization for bacterial infection in community-based patients with type 2 diabetes: the fremantle diabetes study. PLoS One 2013;8:e60502. [Crossref] [PubMed]
- Jensen AV, Faurholt-Jepsen D, Egelund GB, et al. Undiagnosed Diabetes Mellitus in Community-Acquired Pneumonia: A Prospective Cohort Study. Clin Infect Dis 2017;65:2091-8. [Crossref] [PubMed]
- Pannu AK, Saroch A, Singla V, et al. Clinical spectrum, etiology and outcome of infectious disease emergencies in adult diabetic patients in northern India. Diabetes Metab Syndr 2020;14:921-5. [Crossref] [PubMed]
- Di Yacovo S, Garcia-Vidal C, Viasus D, et al. Clinical features, etiology, and outcomes of community-acquired pneumonia in patients with diabetes mellitus. Medicine (Baltimore) 2013;92:42-50. [Crossref] [PubMed]
- Kornum JB, Thomsen RW, Riis A, et al. Type 2 diabetes and pneumonia outcomes: a population-based cohort study. Diabetes Care 2007;30:2251-7. [Crossref] [PubMed]
- Liu YX, Cao QM, Ma BC. Pathogens distribution and drug resistance in patients with acute cerebral infarction complicated with diabetes and nosocomial pulmonary infection. BMC Infect Dis 2019;19:603. [Crossref] [PubMed]
- López-de-Andrés A, de Miguel-Díez J, Jiménez-Trujillo I, et al. Hospitalisation with community-acquired pneumonia among patients with type 2 diabetes: an observational population-based study in Spain from 2004 to 2013. BMJ Open 2017;7:e013097. [Crossref] [PubMed]
- Cheng S, Hou G, Liu Z, et al. Risk prediction of in-hospital mortality among patients with type 2 diabetes mellitus and concomitant community-acquired pneumonia. Ann Palliat Med 2020;9:3313-25. [Crossref] [PubMed]
- Huang CH, Tsai JS, Chen IW, et al. Risk factors for in-hospital mortality in patients with type 2 diabetes complicated by community-acquired Klebsiella pneumoniae bacteremia. J Formos Med Assoc 2015;114:916-22. [Crossref] [PubMed]
- Huang D, He D, Gong L, et al. Clinical characteristics and risk factors associated with mortality in patients with severe community-acquired pneumonia and type 2 diabetes mellitus. Crit Care 2021;25:419. [Crossref] [PubMed]
- Wingrove JA, DiScipio RG, Chen Z, et al. Activation of complement components C3 and C5 by a cysteine proteinase (gingipain-1) from Porphyromonas (Bacteroides) gingivalis. J Biol Chem 1992;267:18902-7. [Crossref] [PubMed]
- Jia F, Wang G, Xu J, et al. Role of tumor necrosis factor-α in the mortality of hospitalized patients with severe and critical COVID-19 pneumonia. Aging (Albany NY) 2021;13:23895-912. [Crossref] [PubMed]
- Coil DA, Vandersmissen L, Ginevra C, et al. Intragenic tandem repeat variation between Legionella pneumophila strains. BMC Microbiol 2008;8:218. [Crossref] [PubMed]
- Ma CM, Wang N, Su QW, et al. Age, Pulse, Urea, and Albumin Score: A Tool for Predicting the Short-Term and Long-Term Outcomes of Community-Acquired Pneumonia Patients With Diabetes. Front Endocrinol (Lausanne) 2022;13:882977. [Crossref] [PubMed]
- Guo L, Song Y, Li N, et al. A New Prognostic Index PDPI for the Risk of Pneumonia Among Patients With Diabetes. Front Cell Infect Microbiol 2021;11:723666. [Crossref] [PubMed]
- Guo L, Wei D, Zhang X, et al. Clinical Features Predicting Mortality Risk in Patients With Viral Pneumonia: The MuLBSTA Score. Front Microbiol 2019;10:2752. [Crossref] [PubMed]
- Tashiro M, Fushimi K, Takazono T, et al. A mortality prediction rule for non-elderly patients with community-acquired pneumonia. BMC Pulm Med 2016;16:39. [Crossref] [PubMed]
- Christ-Crain M, Breidthardt T, Stolz D, et al. Use of B-type natriuretic peptide in the risk stratification of community-acquired pneumonia. J Intern Med 2008;264:166-76. [Crossref] [PubMed]
- Guo W, Li M, Dong Y, et al. Diabetes is a risk factor for the progression and prognosis of COVID-19. Diabetes Metab Res Rev 2020;36:e3319. [Crossref] [PubMed]
- Torres A, Ramirez P, Montull B, et al. Biomarkers and community-acquired pneumonia: tailoring management with biological data. Semin Respir Crit Care Med 2012;33:266-71. [Crossref] [PubMed]