Branched-chain amino acids and risk of lung cancer: insights from mendelian randomization and NHANES III
Highlight box
Key findings
• Genetically predicted circulatory branched-chain amino acids (BCAAs) concentrations were associated with higher risks of lung cancer (LUCA), and body mass index might play a mediational role in this relationship.
What is known and what is new?
• Circulatory and dietary intake of BCAA were observed to be associated with long-term risk of certain cancers.
• Abnormal BCAA metabolism may serve as novel biomarkers and histology-specifically influences the carcinogenesis of LUCA.
What is the implication, and what should change now?
• The findings provide insights into the effect of abnormal metabolism on LUCA and might guide early diagnosis and intervention strategies of the disease.
Introduction
Lung cancer (LUCA), mainly composed of lung adenocarcinoma (LUAD) and squamous cell lung cancer (LUSC), is one of the most commonly diagnosed malignant tumors and the leading cause of cancer-related mortality worldwide (1,2). Despite tremendous progress in therapeutic strategies, only approximately 23% of all patients diagnosed with LUCA manage to survive for more than 5 years in the US population, partially attributed to over half of the cases being diagnosed at an advanced stage (2). Therefore, rectifying of modifiable risk factors and early tumor detection are of pivotal importance to prevent cancer and improve prognosis. LUCA is associated with a range of risk factors, such as smoking, air pollution, obesity, blood copper, socioeconomic status, and others (3-6). In recent years, emerging evidence has suggested that nutritional metabolites are essential for not only normal biological function of human bodies, but also the malignancy development (7,8). For instance, cancer cells exhibit the Warburg effect, wherein they prefer to carry out glycolysis in the cytosol, even with sufficient oxygen (9); and cancer cells have also experienced alterations in the utilization of amino acids, fatty acids and other nutritional metabolites (10).
Branched-chain amino acids (BCAAs), which consist of leucine, isoleucine, and valine, are considered a vital class of essential amino acids, and could be derived solely from diets. Based on accumulating evidence from basic studies, it appears that BCAA metabolism is associated with specific cancer phenotypes and may be involved in modulating pathways related to tumor development and progression (8,11). For example, elevated levels of circulating BCAA have been demonstrated to be an early predictive and prognostic indicator for human pancreatic ductal adenocarcinoma (PDAC), which could potentially share etiological pathways with LUCA (12-14). In a case-control study of 141 non-small cell lung cancer (NSCLC) patients and 423 matched controls, both plasma levels of leucine and isoleucine were found to be significantly higher in NSCLC patients and plasma amino acid profiling was established as a potential screening tool (15). For breast cancer (BC), elevated circulatory BCAA levels were found to be associated with postmenopausal BC risk (16); while dietary intakes of BCAA were not likely to be a risk factor for BC (17). Moreover, positive associations between elevated circulating BCAA and potential risk factors of carcinogenesis and tumor progression, such as obesity and diabetes, were reported in previous investigations (18,19). Overall, BCAA metabolism plays an essential role in tumorigenesis and tumor progression and may be associated with LUCA through mediated or unmediated pathways. However, most prior observational studies to investigating the correlation between circulating or dietary BCAA and cancer are in a case-control or cross-sectional design, which lacks reliability for the presence of potential confounders and reverse causation (20-22). Consequently, whether circulatory and dietary BCAA is associated with LUCA incidence, and whether elevated BCAA plays a causal role in the etiology of LUCA, remain unknown.
The application of Mendelian randomization (MR) analyses can circumvent potential biases mentioned above by utilizing genetic variants, usually single nucleotide polymorphisms (SNPs), as instrumental variables (IVs) to investigate the causal effect of an exposure on an outcome (23,24). Given that genetic variants are randomly allocated during meiosis and remain unaffected by environmental and other confounding factors, MR design imitates a randomization procedure and can provide reliable results when performing randomized controlled trials (RCTs) becomes challenging (25). To establish a reliable causal inference, IVs of MR analyses must satisfy the following assumptions (26): (I) the relevance assumption, the genetic variants are strongly associated with the exposure of interest; (II) the independence assumption, the genetic variants are not associated with any confounding factors; (III) the exclusion restriction assumption, the genetic variants are independent of the outcome.
In this study, we used data from genome-wide association studies (GWAS) summary statistics and the National Health and Nutrition Examination Survey (NHANES) III to investigate the relationship between circulatory levels and dietary intake of BCAA and risk of LUCA using two-sample MR analyses, weighted multivariate logistic regression (WMLR) and non-linearity tests. Moreover, we evaluated the associations between BCAA and several LUCA risk factors, such as smoking, alcohol consumption, body mass index (BMI) and diabetes, and utilized two-step MR to quantify the effect of potential mediators. We present this article in accordance with the STROBE-MR reporting checklist (available at https://jtd.amegroups.com/article/view/10.21037/jtd-24-420/rc) (27).
Methods
We conducted two-sample univariate MR (UVMR) analyses based on GWAS summary statistics to infer the causal effects of circulatory BCAA concentrations on overall LUCA and its pathological subtypes. Multivariate MR (MVMR) analyses were performed to explore estimated effect of three types of potentially related BCAA on LUCA by adjusting for the other two amino acids (28). To identify potential factors mediating the causality from circulatory BCAA to LUCA, we adopted UVMR to assess the causal relationship between BCAA and several common risk factors of LUCA, subsequently, performed two-step MR analyses to quantify the mediation effects. To investigate the relationship between dietary BCAA intake and LUCA and its risk factors, we obtained population level data from NHANES III to conduct observational epidemiological analyses. The study was conducted in accordance with the Declaration of Helsinki (as revised in 2013). A comprehensive flowchart illustrating the study design and associations examined is presented in Figure 1.
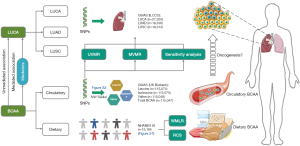
Data sources and study population
We identified IVs for BCAA from the largest GWAS summary data on circulatory levels of total BCAA, leucine, isoleucine and valine, which included a total of 115,047 to 115,075 cases of European population (29). These statistics were derived from an analysis in a half million blood samples within UK Biobank by Nightingale Health Company, and have been available to investigators since 2020. The GWAS data for LUCA were obtained from International Lung Cancer Consortium (ILCCO), with a total of 11,348 cases and 15,861 controls of European descent included in the analysis (30). For histological subtypes of LUCA, we further acquired data for LUAD (3,442 cases and 14,894 controls) and LUSC (3,275 cases and 15,038 controls) from ILCCO within the same population as the overall LUCA cohort. GWAS data for potential risk factors of LUCA, including smoking initiation, alcoholic drinks per week (DrnkWK), BMI and type 2 diabetes, were extracted from the GWAS and Sequencing Consortium of Alcohol and Nicotine use (GSCAN), the international Genetic Investigation of Anthropometric Traits (GIANT) and statistics of Xue et al. (31-33). Detailed information of GWAS involved in the MR analyses is presented in Table S1.
The NHANES III is the seventh in a periodic series of surveys conducted by the National Center for Health Statistics (NCHS) from 1988 to 1994 (34). This survey adopted a complex multistage sample design to provide insights into the health and nutritional status of non-institutionalized population aged 2 months and older in the USA. Detailed information of the survey design and components for analysis have been described previously (34). To obtain eligible participants, we identified those no less than 18 years old from the NHANES III dataset, and then excluded those with missing data on dietary BCAA, BMI, diabetes, cancer location, or other important covariates. The flowchart of the selection of eligible participants in the NHANES III population is presented in Figure S1.
Genetic instruments selection criteria
Genetic instruments for circulatory BCAA, BMI, type 2 diabetes, smoking initiation and DrnkWK were all derived from the corresponding study at genome-wide significant threshold (P value <5×10−8). We performed linkage disequilibrium (LD) pruning to remove SNPs in LD (LD threshold, r2<0.001 and distance >10,000 kb) using a 1,000 genomes European reference panel. Additionally, we imposed a minor allele frequency (MAF) cutoff of >0.01 and removed palindromic SNPs with TwoSampleMR package (default settings of the ‘harmonise_data’ command) in R software (35). To avoid violation of the exclusion restriction assumption, we excluded SNPs significantly associated with outcome phenotype (P value <5×10−8). To avoid disturb of confounding effect, the SNPs associated with potential risk factors of LUCA (smoking, alcohol, BMI, diabetes and C-reactive protein, etc.) were removed according to SNP-phenotype associations found at PhenoScanner v2 (http://www.phenoscanner.medschl.cam.ac.uk, P value <5×10−8) (36). Furthermore, we calculated F-statistics to avoid weak instrument bias, with F-statistics >10 as an often-cited rule-of-thumb for strong IVs. For IVs not extracted in the outcome dataset, we searched for proxies if available (European population, r2>0.8) with LDlinkR package (1.2.3) in R (37).
The Galbraith Radial plot represents a modification of scatter plot designed to display data and findings from an MR study, offering a more straightforward approach to identify potential outliers and influential data points (38). We utilized IVW (inverse-variance weighted) Radial plot to detect and exclude underlying outliers before performing MR analyses. Flowchart of the SNP selection process for MR analyses was shown in Figure S2.
Measurements and definitions
In the mobile examination center (MEC), a trained interviewer administered dietary interviews to all examinees and recorded foods and beverages in the 24-hour dietary recall. Dietary BCAA intake data were acquired from the total nutrient intake file based on 24 h dietary recall and the University of Minnesota Nutrition Coordinating Center (NCC) nutrient database (39). Containing data about more than 80 nutrients and food components, the NCC nutrient database includes fatty acids, amino acids, sugars, caffeine, Vitamin D, and various others. The outcomes were MEC-measured BMI and self-reported LUCA and diabetes. Individuals with LUCA were classified according to the tissue anatomical site of cancer, and no distinction was made among histological subtypes due to dearth of available data. To calculate BMI, weight in kilograms was divided by the square of height in meters. The resultant BMI values were then categorized as either ≥30 or <30 kg/m2, since it was considered the cutoff value for obesity. Diabetes was categorized with the self-reported information that whether they were told by a doctor that they had diabetes.
Information on other important covariates, including age, sex, race/ethnicity, education attainment, marital status, annual household income, smoking, physical activity, serum total cholesterol (TC) concentration, serum triglyceride (TAG) concentration and dietary calories, were collected in the study as well. Specifically, physical activity was computed by multiplying the frequency and intensity rating [a metabolic equivalent of task (MET)] of all physical activities undertaken in the preceding week, as defined in a prior study (40).
Statistical analysis
MR analyses
In the two-sample MR analyses, we conducted UVMR to estimate the causal effect of circulatory BCAA concentrations on LUCA and its pathological subtypes. After harmonizing the exposure and outcome data, the primary approach employed for two-sample MR was random-effects inverse variance weighting (IVW) (41), followed by MR-Egger, weighted median, simple mode and weighed mode. Multiple approaches were implemented to address variant heterogeneity and horizontal pleiotropy. Even if all SNPs display pleiotropic effects, MR-Egger could provide an unbiased estimate, however, this might lead to larger standard error for the estimated effects (42). Weighted median is robust to outliers and provides unbiased estimate even when up to 50% of the information comes from invalid IVs (43). Simple mode and weighed mode derive single causal effect estimates from multiple genetic instruments and offer robustness against pleiotropy (44). Since these approaches for MR have different underlying assumptions, the attainment of consistent results across various methods could potentially enhance the robustness against bias from horizontal pleiotropy. Since there were shared genetic variants among leucine, isoleucine and valine (45), IVW MVMR and MR-Egger MVMR were applied with summary level data to determine the causal effects of multiple, potentially related exposures (namely different BCAA) on LUCA (28).
To identify potential mediators for the causal effects of circulatory BCAA concentration on LUCA and its subtypes, we further investigated the relationship between BCAA and several LUCA risk factors, such as smoking initiation, DrnkWK, BMI and type 2 diabetes. Subsequently, two-step MR approach, which can overcome bias from confounders in traditional non-IV mediation analyses, was adopted to quantify proportion of the mediation effects (46).
Sensitivity analysis
A series of sensitivity analysis were performed to validate the reliability of MR estimates. Firstly, we applied five MR methods with different underlying assumptions, and consistent results would indicate the robustness of IVW estimates. Cochran’s Q test and I2 statistic derived from IVW method were applied to test between-SNP heterogeneity. MR-Egger regression intercept test was adopted to identify potential horizontal pleiotropy. MR-Pleiotropy Residual Sum and Outlier (MR-PRESSO) was performed to detect whether there were remaining outliers and provide corrected estimates (47). Steiger directionality test was performed to test the causal direction between the hypothesized exposure (circulatory BCAA concentrations) and outcomes (LUCA and its subtypes) (48). Multiple types of visual graphical approaches, including forest plot, leave-one-out (LOO) analysis, scatter plot and funnel plot, were additionally employed to offer an intuitive portrayal of the results.
Observational epidemiological analysis
The baseline characteristics were presented as means (SD, standard deviation) for continuous variables and as frequencies (%, percentages) for categorical variables. Statistical comparisons were performed by using the Wilcoxon rank-sum test for complex survey samples and chi-squared test with Rao & Scott’s second-order correction (49). For the low prevalence of LUCA in the cross-sectional population of NHANES III, we only evaluated the relationships between dietary BCAA intake and potential mediators as indicated by the MR analyses, that is, BMI and diabetes, in the subsequent observational analysis. We applied WMLR models to examine the cross-sectional associations of dietary BCAA with BMI and diabetes. Model 1 primarily adjusted for age, sex, race, education, marital status, annual household income, smoking, and physical activity; model 2 included model 1, diabetes, TC, TAG, and calories; model 3 included model 1, BMI, TC, TAG, and calories. After that, we performed subgroup analyses by stratifying the data based on gender. Furthermore, we evaluated non-linearity relationships of dietary BCAA intake with BMI and diabetes using restricted cubic spline (RCS).
All the MR and complex survey sample analyses in the study were performed using R software (4.3.1, R Development Core Team, Austria) with TwoSamlpleMR package (0.5.6), MendelianRandomization package (0.7.0), and survey package (4.2-1). Significance was determined using a threshold of P<0.05 (two-sided).
Results
IVs and characteristics of the study population
In total, 13 index SNPs were selected to genetically predict total circulatory BCAA concentration, and 14, 8, 19 SNPs were employed to genetically predict circulatory leucine, isoleucine, and valine, respectively. F-statistics for IVs in all UVMR analyses were >10, indicating less likelihood of weak instruments. Details of SNPs that were used as genetic instruments in the main analysis were presented in Table S2. Characteristics of pleiotropic SNPs found in PhenoScanner database for estimating the casual relationship of circulatory BCAA with LUCA and its subtypes were presented in Table S3. The IVW Radial plots for identification of outliers, which were removed from analyses, were shown in Figure S3.
Among the NHANES III participants, a sum of 15,185 fulfilled the inclusion criteria for the analysis (Figure S1). The mean age was 43.29 years and 48.11% were men. The crude prevalence of LUCA was 0.11% (weighed prevalence was 0.10%), and the median total dietary BCAA intake was 12.80 g/d. Baseline characteristics of study participants categorized by BMI, diabetes, and LUCA, were shown in Table 1, and participants with LUCA tended to be older (P<0.001) and have lower annual household income (P=0.02) and higher serum TC (P=0.007).
Table 1
Characteristic | Overall (N=15,185)† | BMI group | Diabetes group | Lung cancer group | ||||||||
---|---|---|---|---|---|---|---|---|---|---|---|---|
<30 kg/m2 (N=11,349)† | ≥30 kg/m2 (N=3,836)† | P value‡ | Yes (N=6,879)† | No (N=8,306)† | P value‡ | Yes (N=17)† | No (N=15,168)† | P value‡ | ||||
Age (years) | 43.29±17.13 | 42.47±17.41 | 46.23±15.74 | <0.001 | 42.30±16.10 | 44.10±17.89 | 0.02 | 66.47±10.57 | 43.27±17.12 | <0.001 | ||
Sex | <0.001 | <0.001 | 0.20 | |||||||||
Male | 7,115 (48.11) | 5,667 (49.67) | 1,448 (42.54) | 2,816 (41.97) | 4,299 (53.12) | 12 (72.36) | 7,103 (48.08) | |||||
Female | 8,070 (51.89) | 5,682 (50.33) | 2,388 (57.46) | 4,063 (58.03) | 4,007 (46.88) | 5 (27.64) | 8,065 (51.92) | |||||
Race/ethnicity | <0.001 | 0.041 | 0.50 | |||||||||
Non-Hispanic White | 6,237 (76.64) | 4,913 (77.54) | 1,324 (73.43) | 2,700 (77.28) | 3,537 (76.12) | 10 (82.82) | 6,227 (76.63) | |||||
Non-Hispanic Black | 4,127 (10.52) | 2,877 (9.59) | 1,250 (13.83) | 1,959 (10.96) | 2,168 (10.16) | 5 (13.32) | 4,122 (10.52) | |||||
Mexican-American | 4,230 (5.27) | 3,088 (5.02) | 1,142 (6.18) | 1,994 (5.56) | 2,236 (5.04) | 2 (3.86) | 4,228 (5.28) | |||||
Other | 591 (7.56) | 471 (7.84) | 120 (6.56) | 226 (6.19) | 365 (8.68) | 0 | 591 (7.57) | |||||
Education | <0.001 | 0.08 | 0.20 | |||||||||
Less than high school | 6,162 (24.70) | 4,494 (23.50) | 1,668 (28.96) | 2,584 (23.87) | 3,578 (25.37) | 8 (42.79) | 6,154 (24.68) | |||||
High school graduate | 4,750 (34.27) | 3,497 (33.32) | 1,253 (37.66) | 2,288 (35.88) | 2,462 (32.96) | 5 (32.95) | 4,745 (34.28) | |||||
Greater than high school | 4,273 (41.03) | 3,358 (43.18) | 915 (33.39) | 2,007 (40.26) | 2,266 (41.66) | 4 (24.26) | 4,269 (41.05) | |||||
Marital status | <0.001 | 0.11 | 0.30 | |||||||||
Married | 9,165 (65.10) | 6,753 (64.08) | 2,412 (68.70) | 4,186 (66.13) | 4,979 (64.25) | 13 (74.26) | 9,152 (65.09) | |||||
Widowed/divorced | 3,140 (16.48) | 2,253 (15.67) | 887 (19.36) | 1,406 (16.52) | 1,734 (16.44) | 4 (25.74) | 3,136 (16.47) | |||||
Never married | 2,880 (18.42) | 2,343 (20.25) | 537 (11.95) | 1,287 (17.34) | 1,593 (19.31) | 0 (0.00) | 2,880 (18.44) | |||||
Annual household income ($) | 0.009 | 0.20 | 0.02 | |||||||||
<$20,000 | 7,335 (32.64) | 5,388 (31.67) | 1,947 (36.08) | 3,192 (31.68) | 4,143 (33.43) | 9 (59.82) | 7,326 (32.61) | |||||
≥$20,000 | 7,850 (67.36) | 5,961 (68.33) | 1,889 (63.92) | 3,687 (68.32) | 4,163 (66.57) | 8 (40.18) | 7,842 (67.39) | |||||
Smoking | <0.001 | 0.40 | 0.20 | |||||||||
Never | 7,703 (46.76) | 5,644 (46.70) | 2,059 (47.00) | 3,499 (45.81) | 4,204 (47.55) | 1 (15.86) | 7,702 (46.80) | |||||
Former | 3,623 (24.95) | 2,608 (23.48) | 1,015 (30.17) | 1,625 (25.43) | 1,998 (24.56) | 13 (53.03) | 3,610 (24.92) | |||||
Current | 3,859 (28.28) | 3,097 (29.82) | 762 (22.83) | 1,755 (28.76) | 2,104 (27.89) | 3 (31.12) | 3,856 (28.28) | |||||
Physical activity (MET/week) | 26.03±30.97 | 27.63±31.76 | 20.32±27.24 | <0.001 | 24.24±29.34 | 27.49±32.17 | <0.001 | 28.08±41.03 | 26.03±30.96 | 0.60 | ||
TC (mg/dL) | 203.03±43.13 | 199.98±42.53 | 213.85±43.49 | <0.001 | 203.69±43.24 | 202.49±43.02 | 0.40 | 224.84±41.28 | 203.01±43.12 | 0.007 | ||
TAG (mg/dL) | 142.21±121.80 | 129.53±99.41 | 187.24±172.72 | <0.001 | 148.53±142.42 | 137.04±101.60 | 0.02 | 174.97±86.07 | 142.18±121.83 | 0.06 | ||
Calories (kcal/d) | 2,218.82±1,077.71 | 2,253.80±1,091.61 | 2,094.61±1,017.29 | <0.001 | 2,172.98±1,043.86 | 2,256.32±1,103.28 | 0.01 | 2,295.64±895.12 | 2,218.74±1,077.91 | 0.50 | ||
Leucine (g/d) | 6.42±3.53 | 6.47±3.58 | 6.25±3.33 | 0.12 | 6.28±3.44 | 6.53±3.59 | 0.03 | 7.62±4.31 | 6.42±3.53 | 0.30 | ||
Isoleucine (g/d) | 3.74±2.08 | 3.77±2.11 | 3.65±1.96 | 0.20 | 3.65±2.02 | 3.81±2.12 | 0.03 | 4.53±2.60 | 3.74±2.08 | 0.30 | ||
Valine (g/d) | 4.19±2.30 | 4.23±2.33 | 4.08±2.17 | 0.10 | 4.10±2.24 | 4.27±2.34 | 0.03 | 4.95±2.68 | 4.19±2.30 | 0.30 | ||
Total BCAA (g/d) | 14.35±7.89 | 14.46±8.01 | 13.97±7.45 | 0.13 | 14.03±7.68 | 14.62±8.05 | 0.03 | 17.11±9.58 | 14.35±7.89 | 0.30 | ||
Total BCAA (quartile) | 0.30 | 0.03 | 0.50 | |||||||||
Q1 (6.46±1.87) | 4,369 (25.29) | 3,173 (24.93) | 1,196 (26.57) | 2,008 (26.32) | 2,361 (24.44) | 2 (8.35) | 4,367 (25.30) | |||||
Q2 (10.94±1.09) | 3,840 (25.24) | 2,886 (25.35) | 954 (24.86) | 1,723 (25.54) | 2,117 (25.00) | 7 (36.60) | 3,833 (25.23) | |||||
Q3 (15.21±1.45) | 3,586 (24.78) | 2,678 (24.56) | 908 (25.59) | 1,651 (25.45) | 1,935 (24.24) | 4 (24.37) | 3,582 (24.78) | |||||
Q4 (25.07±7.36) | 3,390 (24.68) | 2,612 (25.16) | 778 (22.98) | 1,497 (22.69) | 1,893 (26.32) | 4 (30.68) | 3,386 (24.68) |
†, mean (SD) for continuous; n (%) for categorical, unweighted frequency counts and weighted percentages are shown. ‡, Wilcoxon rank-sum test for complex survey samples; chi-squared test with Rao & Scott’s second-order correction. BMI, body mass index; NHANES III, the National Health and Nutrition Examination Survey III; MET, metabolic equivalent of task; TC, total cholesterol; TAG, triglyceride; BCAA, branched-chain amino acids; SD, standard deviation.
Effects of circulatory BCAA on LUCA and subtypes
We carried out a comprehensive MR study, employing genetically predicted circulatory concentrations of leucine, isoleucine, valine, and total BCAA, to examine their causal relationships with LUCA and its pathological subtypes. We observed that higher circulatory valine concentration was significantly associated with increased risk of overall LUCA [odds ratio (OR) =1.324, 95% confidence interval (CI): 1.058–1.658, P=0.01], while no significant associations between leucine, isoleucine, or total BCAA and LUCA were found (Figure 2). In the analysis of pathological subtypes, we did not observe positive causal relationships between circulatory BCAA concentrations and LUAD (Figure 3); however, UVMR estimates showed that a genetic predisposition to high circulatory BCAA potentially increases the risk of LUSC (Figure 4). Genetically predicted elevated levels of leucine (OR =1.924, 95% CI: 1.274–2.906, P=0.002), isoleucine (OR =1.902, 95% CI: 1.171–3.088, P=0.009), valine (OR =1.940, 95% CI: 1.395–2.700, P<0.001), and total BCAA (OR =1.866, 95% CI: 1.267–2.747, P=0.002) were founded to be significantly associated with a higher risk of LUSC. In the IVW MVMR analysis, after adjusting for the other two types of BCAA, the causal impact of valine on LUSC remained significant with a wider range of CI (OR =3.165, 95% CI: 1.013–9.882, P=0.048). Additionally, a reduction in significance was observed for the effect of leucine (P=0.664) and isoleucine (P=0.64) on LUSC (Table S4).
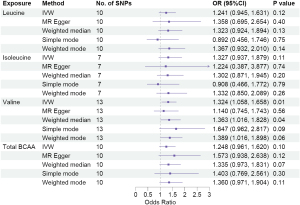
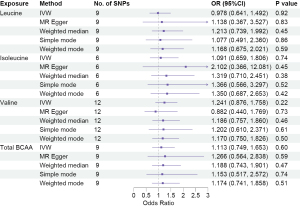
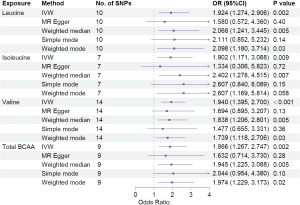
To identify whether the effects of circulatory BCAA on LUCA and its subtypes were partially explained through mediating pathways, we investigated the relationships between BCAA and several LUCA risk factors. We found positive associations between higher circulatory levels of leucine and elevated BMI (beta =0.060, 95% CI: 0.022–0.099, P=0.002), leucine and risk of type 2 diabetes (OR =1.270, 95% CI: 1.040–1.552, P=0.02), and between valine and type 2 diabetes (OR =1.260, 95% CI: 1.039–1.528, P=0.02) (Tables S5,S6). No causal effects were observed from circulatory BCAA on smoking initiation or DrnkWK (Tables S5,S6). For two-step MR analysis, the potential role of BMI in mediating the effect of circulatory BCAA on LUSC was identified (Tables S5,S6), and approximately mediated 3.91% (95% CI: 1.22–7.18%) of the total effects.
Sensitivity analyses
No significant heterogeneity was observed for the analyses of circulatory BCAA and risk of LUCA and its subtypes using Cochran’s Q and I2 statistics (Table S7). For the application of random-effects IVW model, heterogeneity in certain analyses for LUCA risk factors was acceptable. P values for MR-Egger intercept tests were >0.05, except for the relationship between leucine and DrnkWK (Table S8). No outliers were detected with MR-PRESSO in the primary UVMR analyses. Evidence from Steiger directionality test supported the causal direction from hypothesized exposure (circulatory BCAA) to LUCA and subtypes (Table S9). Multiple visual graphics, such as forest plot (Figure S4), LOO analysis (Figure S5), scatter plot (Figure S6) and funnel plot (Figure S7), further ensured the robustness of unbiased results.
Observational epidemiological analyses
Based on the NHANES III, we investigated the relationships of dietary BCAA intake with excessive BMI (≥30 kg/m2) and prevalence of diabetes. For only 17 participants with LUCA (crude prevalence 0.11%) were identified in the population, the associations between dietary BCAA and LUCA were not further evaluated. Without adjusting for any covariate, participants with higher dietary intake of leucine (P=0.03), isoleucine (P=0.03), valine (P=0.03), total BCAA (P=0.03), and total BCAA quartiles (P=0.03), demonstrated increased prevalence of diabetes (Table 1). The multivariate-adjusted associations of dietary BCAA intake with BMI and diabetes were shown in Table 2. After adjusting for all covariates with model 2, we observed that dietary intake of leucine (OR =1.04, 95% CI: 1.01–1.07, P=0.004), isoleucine (OR =1.07, 95% CI: 1.02–1.12, P=0.002), valine (OR =1.07, 95% CI: 1.02–1.11, P=0.004), total BCAA (OR =1.02, 95% CI: 1.01–1.03, P=0.003), and total BCAA quartiles (P=0.03) were independently associated with excessive BMI (≥30 kg/m2). Similar results were observed in stratified analyses by gender (Tables S10,S11). For tests of non-linearity, multivariate-adjusted RCS demonstrated negative non-linear dose-response relationships of dietary total BCAA intake with BMI (Pnon-linearity=0.10) and diabetes (Pnon-linearity=0.89), and the same is true for dietary intake of leucine, isoleucine, and valine (Figures S8,S9).
Table 2
Characteristic | BMI ≥30 or <30 kg/m2 | Diabetes | |||||||||||||
---|---|---|---|---|---|---|---|---|---|---|---|---|---|---|---|
Model 1 | Model 2 | Model 1 | Model 3 | ||||||||||||
OR | 95% CI | P value | OR | 95% CI | P value | OR | 95% CI | P value | OR | 95% CI | P value | ||||
Leucine (g/d) | 1.01 | 1.00–1.03 | 0.13 | 1.04 | 1.01–1.07 | 0.004 | 1.00 | 0.97–1.02 | 0.809 | 1.00 | 0.96–1.04 | 0.99 | |||
Isoleucine (g/d) | 1.02 | 0.99–1.06 | 0.11 | 1.07 | 1.02–1.12 | 0.002 | 0.99 | 0.95–1.03 | 0.742 | 1.00 | 0.93–1.06 | 0.89 | |||
Valine (g/d) | 1.02 | 0.99–1.05 | 0.15 | 1.07 | 1.02–1.11 | 0.004 | 1.00 | 0.96–1.03 | 0.753 | 1.00 | 0.94–1.06 | 0.92 | |||
Total BCAA (g/d) | 1.01 | 1.00–1.01 | 0.13 | 1.02 | 1.01–1.03 | 0.003 | 1.00 | 0.99–1.01 | 0.774 | 1.00 | 0.98–1.02 | 0.94 | |||
Total BCAA (quartile) | 0.13 | 0.03 | 0.132 | 0.57 | |||||||||||
Q1 (6.46±1.87) | – | – | – | – | – | – | – | – | |||||||
Q2 (10.94±1.09) | 0.99 | 0.85–1.16 | 1.06 | 0.91–1.24 | 0.99 | 0.85–1.16 | 1.01 | 0.88–1.17 | |||||||
Q3 (15.21±1.45) | 1.16 | 0.97–1.38 | 1.26 | 1.04–1.53 | 1.16 | 0.97–1.38 | 1.06 | 0.88–1.28 | |||||||
Q4 (25.07±7.36) | 1.15 | 0.95–1.40 | 1.40 | 1.07–1.82 | 1.15 | 0.95–1.40 | 0.96 | 0.72–1.27 |
Model 1: adjusted for age, sex, race, education, marital status, annual household income, smoking, and physical activity; Model 2: including model 1, diabetes, TC, TAG, and calories; Model 3: including model 1, BMI, TC, TAG, and calories. BCAA, branched-chain amino acids; BMI, body mass index; NHANES III, the National Health and Nutrition Examination Survey III; OR, odds ratio; CI, confidence interval; TC, total cholesterol; TAG, triglyceride.
Discussion
In the current study, we conducted a combination of MR and observational analyses to probe the relationships of circulatory levels and dietary intake of BCAA with LUCA and its pathological subtypes, as well as potential mediators. Our findings demonstrated that genetically predicted circulatory levels of BCAA were associated with increased risk of LUSC, while they did not significantly affect LUAD. For potential mediation factors, we provided causal evidence that BMI mediated a minor proportion of circulatory leucine level on LUSC risk. Furthermore, positive associations between dietary intake of BCAA and both of BMI and diabetes were observed in the NHANES III population. Future studies are needed to reveal other mediated or unmediated associations from circulatory and dietary levels of BCAA on LUCA and its subtypes.
Most recently, the emerging role of altered BCAA metabolism in tumor development and progression has attracted much concern (11,50). As essential amino acids with various metabolites in human bodies, BCAA is involved in complex metabolic pathways and plays a pivotal role in the regulation of normal physiological functions, such as protein synthesis, energy production, signaling transduction and so on. Previous studies have demonstrated the alteration of BCAA metabolism in specific cancer phenotypes, highlighting its potential as an indicative factor for tumor diagnosis and prognosis prediction (8,12). For instance, based on prospectively collected pre-diagnostic blood samples, Mayers et al. reported a correlation that linked elevated plasma BCAA levels with a twofold higher risk of future pancreatic cancer (PC) diagnosis (12). Furthermore, they observed elevated plasma BCAA, which was a product of tissue protein breakdown, in mice with Kras-driven early-stage PC (12). Previous prospective studies also suggested that plasma leucine and valine were associated with long-term BC risk and could contribute to screening of early BC (51,52). However, few prior studies evaluated the association between circulatory BCAA and risk of LUCA, especially its pathological subtypes.
The research of Mayers et al. showed that NSCLC tumors actively utilize plasma BCAA and the loss of key metabolic enzymes, such as BCAT1 and BCAT2, impairs NSCLC tumors formation (14). Another preliminary research reported increased plasma levels of BCAA in preoperative NSCLC patients, compared with healthy controls and postoperative status (53). Plasma BCAA combined with other amino acids have also been used for development of LUCA screening tools (21). Overall, prior studies indicated a crucial role of plasma BCAA in LUCA occurrence and progression, but the causality from circulatory BCAA to LUCA and its pathological subtypes remains unknown due to interference of confounders. Compared with previous research, our study provided novel genetic evidence for the causal impact of circulatory BCAA concentrations on overall LUCA and LUSC. In our results, there was no significant causal relationships between circulatory BCAA and LUAD. Given the significant molecular and pathological distinctions between LUAD and LUSC (54), the examination of the histology-specific impact of BCAA on lung oncogenesis was well-founded. Moreover, results of IVW MVMR analysis suggested a causal effect of plasma valine on LUSC independent of leucine and isoleucine, which should be interpreted with caution. Future investigations are required to unveil the foundational mechanisms contributing to the histology-specific disparities.
It has been observed that elevated circulatory BCAA is independently associated with multiple tumor-related risk factors, such as obesity and diabetes (55). Genetic evidence for the causal effects of plasma BCAA on obesity have been provided in a previous MR study (OR =0.022, 95% CI: 1.35–4.28, P=0.004), with consistent results to our findings (56). Here we revealed the causal associations between circulatory BCAA and potential risk factors of LUCA, including BMI and type 2 diabetes. In the subsequent two-step MR analyses, we observed the causal relationships of circulatory leucine with BMI, and BMI with LUSC, respectively, which indicated the mediation role of BMI. Following this, we further quantified the effect mediated by BMI and found that only a minimal proportion can be elucidated through this pathway. In other words, our analyses uncovered a minor proportion mediated by BMI, leaving the majority of the associations between BCAA and LUCA, either mediated or unmediated, yet to be clarified. First, there may be other omitted potential risk factors mediating the effect of circulatory BCAA on lung carcinogenesis. Second, considering the crucial functional role of BCAA in human bodies, BCAA may induce lung tumor development through underlying unmediated pathways.
Elevated circulatory BCAA can affect both cell intrinsic characteristics and reflect systemic metabolic status of individuals with specific cancers (8). Although there are probably diverse pathways underlying the impact of circulatory BCAA on lung carcinogenesis, particular interest lies in the function of BCAA in modulating mTOR activity, through which BCAA primarily regulates protein metabolism (57). The impacts of BCAA metabolism on oncogenesis and tumor progression are far from being well-established yet, therefore, further studies are needed to understand the underlying mechanisms.
As the most direct approach to manipulating BCAA metabolism in vivo, dietary BCAA intake has been studied over the past years for its effects on multiple cancers, such as PDAC (58), BC (59), colorectal cancer (CRC) (60), and so on. In our study, for low prevalence of LUCA in the NHANES III population, we indirectly investigated the correlations of dietary BCAA intake with BMI and diabetes and obtained consistent results with our MR estimates. Moreover, recent research indicated a limited connection between dietary BCAA intake and circulatory BCAA levels (61). Future studies are needed to provide evidence from large-scale population and better understanding of the correlation between dietary BCAA intake and risk of LUCA.
The main highlight of this study is the combined application of genetic-level MR analyses and population-level observational analyses. The use of MR design reduced biases from confounders and reverse causality; and the additional observational analyses provided more insights into dietary BCAA intake, subgroup characteristics and non-linearity relationships. Results from genetic and population level of different perspectives provided a more comprehensive and robust evidence for the correlation of circulatory and dietary BCAA with LUCA risk. Second, we conducted all the MR analyses in European population, thereby reducing bias caused by population stratification. Third, we performed a series of sensitivity analyses, including visualized graphics, to ensure the credibility of MR estimates. Moreover, we validated the causal direction between the hypothesized exposure and outcomes.
There are also limitations that need to be considered in this study. First, we used BCAA GWAS from the UK Biobank and LUCA GWAS from ILCCO to control bias caused by sample overlap, nevertheless, potential overlap might still exist due to the large sample size. Second, our analysis was restricted to European population, whether our findings fit other ancestries remains to be validated. Third, in the sensitivity analyses, heterogeneity was observed in the analyses for circulatory BCAA on several risk factors of LUCA. However, no heterogeneity was found for the effects of BCAA on LUCA and its subtypes, and the application of random-effects IVW model provided undisturbed estimates. Fourth, in the observational epidemiological analyses, we did not further investigate the associations between dietary BCAA intake and LUCA in WMLR and RCS models due to low prevalence of LUCA in the NHANES III population. Another limitation could be the cross-sectional design of the observational study to investigate associations of dietary BCAA with BMI and diabetes, in which reverse causation is possible. Finally, since information of dietary BCAA intake was collected only once during the interview, the fluctuations in BCAA intake over time may lead to biased findings.
Conclusions
In conclusion, the present study provided a combination of genetic and population-based evidence for the relationships between BCAA and risk of LUCA. Genetically predicted circulatory levels of leucine, isoleucine, valine, and total BCAA were causally associated with an increased risk of LUSC, while they did not significantly affect LUAD. BMI mediated a minor proportion of the effect of circulatory BCAA concentrations on LUSC risk, however, the majority of BCAA’s effects remained unexplained. Population-based observational analyses added insights into the positive relationships between dietary intake of BCAA and potential risk factors of LUCA. Further prospective and basic studies are required to confirm the inference and uncover the underlying mediated or unmediated associations.
Acknowledgments
We are extremely grateful to all the participants and researchers of the UK Biobank, ILCCO, NHANES III, GIANT, and the GSCAN consortium. The creation of specific figures in this article involved the utilization of Servier Medical Art, which was provided by Servier and licensed under a Creative Commons Attribution 3.0 Unported License (https://creativecommons.org/licenses/by/3.0/).
Funding: This work was supported by
Footnote
Reporting Checklist: The authors have completed the STROBE-MR reporting checklist. Available at https://jtd.amegroups.com/article/view/10.21037/jtd-24-420/rc
Peer Review File: Available at https://jtd.amegroups.com/article/view/10.21037/jtd-24-420/prf
Conflicts of Interest: All authors have completed the ICMJE uniform disclosure form (available at https://jtd.amegroups.com/article/view/10.21037/jtd-24-420/coif). The authors have no conflicts of interest to declare.
Ethical Statement: The authors are accountable for all aspects of the work in ensuring that questions related to the accuracy or integrity of any part of the work are appropriately investigated and resolved. The study was conducted in accordance with the Declaration of Helsinki (as revised in 2013).
Open Access Statement: This is an Open Access article distributed in accordance with the Creative Commons Attribution-NonCommercial-NoDerivs 4.0 International License (CC BY-NC-ND 4.0), which permits the non-commercial replication and distribution of the article with the strict proviso that no changes or edits are made and the original work is properly cited (including links to both the formal publication through the relevant DOI and the license). See: https://creativecommons.org/licenses/by-nc-nd/4.0/.
References
- Sung H, Ferlay J, Siegel RL, et al. Global Cancer Statistics 2020: GLOBOCAN Estimates of Incidence and Mortality Worldwide for 36 Cancers in 185 Countries. CA Cancer J Clin 2021;71:209-49. [Crossref] [PubMed]
- Siegel RL, Miller KD, Wagle NS, et al. Cancer statistics, 2023. CA Cancer J Clin 2023;73:17-48. [Crossref] [PubMed]
- Li C, Li J, Xiong S, et al. Risk mapping of lung cancer: a comprehensive appraisal of published meta-analyses incorporating Mendelian randomization studies. J Cancer Res Clin Oncol 2023;149:6857-73. [Crossref] [PubMed]
- Shen J, Zhou H, Liu J, et al. A modifiable risk factors atlas of lung cancer: A Mendelian randomization study. Cancer Med 2021;10:4587-603. [Crossref] [PubMed]
- Hill W, Lim EL, Weeden CE, et al. Lung adenocarcinoma promotion by air pollutants. Nature 2023;616:159-67. [Crossref] [PubMed]
- Doll R, Peto R, Wheatley K, et al. Mortality in relation to smoking: 40 years' observations on male British doctors. BMJ 1994;309:901-11. [Crossref] [PubMed]
- Ananieva E. Targeting amino acid metabolism in cancer growth and anti-tumor immune response. World J Biol Chem 2015;6:281-9. [Crossref] [PubMed]
- Sivanand S, Vander Heiden MG. Emerging Roles for Branched-Chain Amino Acid Metabolism in Cancer. Cancer Cell 2020;37:147-56. [Crossref] [PubMed]
- Nava GM, Madrigal Perez LA. Metabolic profile of the Warburg effect as a tool for molecular prognosis and diagnosis of cancer. Expert Rev Mol Diagn 2022;22:439-47. [Crossref] [PubMed]
- Li Z, Zhang H. Reprogramming of glucose, fatty acid and amino acid metabolism for cancer progression. Cell Mol Life Sci 2016;73:377-92. [Crossref] [PubMed]
- Wetzel TJ, Erfan SC, Ananieva EA. The emerging role of the branched chain aminotransferases, BCATc and BCATm, for anti-tumor T-cell immunity. Immunometabolism (Cobham) 2023;5:e00014. [Crossref] [PubMed]
- Mayers JR, Wu C, Clish CB, et al. Elevation of circulating branched-chain amino acids is an early event in human pancreatic adenocarcinoma development. Nat Med 2014;20:1193-8. [Crossref] [PubMed]
- Li JT, Yin M, Wang D, et al. BCAT2-mediated BCAA catabolism is critical for development of pancreatic ductal adenocarcinoma. Nat Cell Biol 2020;22:167-74. [Crossref] [PubMed]
- Mayers JR, Torrence ME, Danai LV, et al. Tissue of origin dictates branched-chain amino acid metabolism in mutant Kras-driven cancers. Science 2016;353:1161-5. [Crossref] [PubMed]
- Maeda J, Higashiyama M, Imaizumi A, et al. Possibility of multivariate function composed of plasma amino acid profiles as a novel screening index for non-small cell lung cancer: a case control study. BMC Cancer 2010;10:690. [Crossref] [PubMed]
- Zeleznik OA, Balasubramanian R, Ren Y, et al. Branched-Chain Amino Acids and Risk of Breast Cancer. JNCI Cancer Spectr 2021;5:pkab059. [Crossref] [PubMed]
- Tobias DK, Chai B, Tamimi RM, et al. Dietary Intake of Branched Chain Amino Acids and Breast Cancer Risk in the NHS and NHS II Prospective Cohorts. JNCI Cancer Spectr 2021;5:pkab032. [Crossref] [PubMed]
- Guasch-Ferré M, Hruby A, Toledo E, et al. Metabolomics in Prediabetes and Diabetes: A Systematic Review and Meta-analysis. Diabetes Care 2016;39:833-46. [Crossref] [PubMed]
- Yuan S, Kar S, Carter P, et al. Is Type 2 Diabetes Causally Associated With Cancer Risk? Evidence From a Two-Sample Mendelian Randomization Study. Diabetes 2020;69:1588-96. [Crossref] [PubMed]
- Miyagi Y, Higashiyama M, Gochi A, et al. Plasma free amino acid profiling of five types of cancer patients and its application for early detection. PLoS One 2011;6:e24143. [Crossref] [PubMed]
- Kim HJ, Jang SH, Ryu JS, et al. The performance of a novel amino acid multivariate index for detecting lung cancer: A case control study in Korea. Lung Cancer 2015;90:522-7. [Crossref] [PubMed]
- Tobias DK, Hazra A, Lawler PR, et al. Circulating branched-chain amino acids and long-term risk of obesity-related cancers in women. Sci Rep 2020;10:16534. [Crossref] [PubMed]
- Tin A, Köttgen A. Mendelian Randomization Analysis as a Tool to Gain Insights into Causes of Diseases: A Primer. J Am Soc Nephrol 2021;32:2400-7. [Crossref] [PubMed]
- Burgess S, Davey Smith G, Davies NM, et al. Guidelines for performing Mendelian randomization investigations: update for summer 2023. Wellcome Open Res 2023;4:186. [Crossref] [PubMed]
- Sekula P, Del Greco M F, Pattaro C, et al. Mendelian Randomization as an Approach to Assess Causality Using Observational Data. J Am Soc Nephrol 2016;27:3253-65. [Crossref] [PubMed]
- de Leeuw C, Savage J, Bucur IG, et al. Understanding the assumptions underlying Mendelian randomization. Eur J Hum Genet 2022;30:653-60. [Crossref] [PubMed]
- Skrivankova VW, Richmond RC, Woolf BAR, et al. Strengthening the Reporting of Observational Studies in Epidemiology Using Mendelian Randomization: The STROBE-MR Statement. JAMA 2021;326:1614-21. [Crossref] [PubMed]
- Sanderson E, Spiller W, Bowden J. Testing and correcting for weak and pleiotropic instruments in two-sample multivariable Mendelian randomization. Stat Med 2021;40:5434-52. [Crossref] [PubMed]
- Xu H, Wang X, Geng G, et al. Association of Circulating Branched-Chain Amino Acids with Cardiovascular Diseases: A Mendelian Randomization Study. Nutrients 2023;15:1580. [Crossref] [PubMed]
- Wang Y, McKay JD, Rafnar T, et al. Rare variants of large effect in BRCA2 and CHEK2 affect risk of lung cancer. Nat Genet 2014;46:736-41. [Crossref] [PubMed]
- Liu M, Jiang Y, Wedow R, et al. Association studies of up to 1.2 million individuals yield new insights into the genetic etiology of tobacco and alcohol use. Nat Genet 2019;51:237-44. [Crossref] [PubMed]
- Yengo L, Sidorenko J, Kemper KE, et al. Meta-analysis of genome-wide association studies for height and body mass index in ∼700000 individuals of European ancestry. Hum Mol Genet 2018;27:3641-9. [Crossref] [PubMed]
- Xue A, Wu Y, Zhu Z, et al. Genome-wide association analyses identify 143 risk variants and putative regulatory mechanisms for type 2 diabetes. Nat Commun 2018;9:2941. [Crossref] [PubMed]
- Plan and operation of the Third National Health and Nutrition Examination Survey, 1988-94. Series 1: programs and collection procedures. Vital Health Stat 1 1994;1-407. [PubMed]
- Hemani G, Zheng J, Elsworth B, et al. The MR-Base platform supports systematic causal inference across the human phenome. Elife 2018;7:e34408. [Crossref] [PubMed]
- Kamat MA, Blackshaw JA, Young R, et al. PhenoScanner V2: an expanded tool for searching human genotype-phenotype associations. Bioinformatics 2019;35:4851-3. [Crossref] [PubMed]
- Myers TA, Chanock SJ, Machiela MJ. LDlinkR: An R Package for Rapidly Calculating Linkage Disequilibrium Statistics in Diverse Populations. Front Genet 2020;11:157. [Crossref] [PubMed]
- Bowden J, Spiller W, Del Greco M F, et al. Improving the visualization, interpretation and analysis of two-sample summary data Mendelian randomization via the Radial plot and Radial regression. Int J Epidemiol 2018;47:1264-78. [Crossref] [PubMed]
- Buzzard I, Feskanich D. Maintaining a food composition data base for multiple research studies: the NCC food table. In: Rand W, Windham C, Wyse B, et al. editors. Food composition data: A user’s perspective. Tokyo: The United Nations University; 1987:115-122.
- Kim H, Hu EA, Rebholz CM. Ultra-processed food intake and mortality in the USA: results from the Third National Health and Nutrition Examination Survey (NHANES III, 1988-1994). Public Health Nutr 2019;22:1777-85. [Crossref] [PubMed]
- Burgess S, Butterworth A, Thompson SG. Mendelian randomization analysis with multiple genetic variants using summarized data. Genet Epidemiol 2013;37:658-65. [Crossref] [PubMed]
- Bowden J, Davey Smith G, Burgess S. Mendelian randomization with invalid instruments: effect estimation and bias detection through Egger regression. Int J Epidemiol 2015;44:512-25. [Crossref] [PubMed]
- Bowden J, Davey Smith G, Haycock PC, et al. Consistent Estimation in Mendelian Randomization with Some Invalid Instruments Using a Weighted Median Estimator. Genet Epidemiol 2016;40:304-14. [Crossref] [PubMed]
- Hartwig FP, Davey Smith G, Bowden J. Robust inference in summary data Mendelian randomization via the zero modal pleiotropy assumption. Int J Epidemiol 2017;46:1985-98. [Crossref] [PubMed]
- Mu L, Ye Z, Hu J, et al. PPM1K-regulated impaired catabolism of branched-chain amino acids orchestrates polycystic ovary syndrome. EBioMedicine 2023;89:104492. [Crossref] [PubMed]
- Carter AR, Sanderson E, Hammerton G, et al. Mendelian randomisation for mediation analysis: current methods and challenges for implementation. Eur J Epidemiol 2021;36:465-78. [Crossref] [PubMed]
- Verbanck M, Chen CY, Neale B, et al. Detection of widespread horizontal pleiotropy in causal relationships inferred from Mendelian randomization between complex traits and diseases. Nat Genet 2018;50:693-8. [Crossref] [PubMed]
- Hemani G, Tilling K, Davey Smith G. Orienting the causal relationship between imprecisely measured traits using GWAS summary data. PLoS Genet 2017;13:e1007081. [Crossref] [PubMed]
- Sjoberg DD, Whiting K, Curry M, et al. Reproducible summary tables with the gtsummary package. The R Journal 2021;13:570-80. [Crossref]
- Wang J, Wang W, Zhu F, et al. The role of branched chain amino acids metabolic disorders in tumorigenesis and progression. Biomed Pharmacother 2022;153:113390. [Crossref] [PubMed]
- Lécuyer L, Dalle C, Lyan B, et al. Plasma Metabolomic Signatures Associated with Long-term Breast Cancer Risk in the SU.VI.MAX Prospective Cohort. Cancer Epidemiol Biomarkers Prev 2019;28:1300-7. [Crossref] [PubMed]
- Yoo HJ, Kim M, Kim M, et al. Analysis of metabolites and metabolic pathways in breast cancer in a Korean prospective cohort: the Korean Cancer Prevention Study-II. Metabolomics 2018;14:85. [Crossref] [PubMed]
- Wang Y, Xiao J, Jiang W, et al. BCKDK alters the metabolism of non-small cell lung cancer. Transl Lung Cancer Res 2021;10:4459-76. [Crossref] [PubMed]
- Relli V, Trerotola M, Guerra E, et al. Abandoning the Notion of Non-Small Cell Lung Cancer. Trends Mol Med 2019;25:585-94. [Crossref] [PubMed]
- Vanweert F, Schrauwen P, Phielix E. Role of branched-chain amino acid metabolism in the pathogenesis of obesity and type 2 diabetes-related metabolic disturbances BCAA metabolism in type 2 diabetes. Nutr Diabetes 2022;12:35. [Crossref] [PubMed]
- Liu L, Wang X, Liu Y, et al. Association of plasma branched-chain amino acids with overweight: A Mendelian randomization analysis. Obesity (Silver Spring) 2021;29:1708-18. [Crossref] [PubMed]
- Jung MK, Okekunle AP, Lee JE, et al. Role of Branched-chain Amino Acid Metabolism in Tumor Development and Progression. J Cancer Prev 2021;26:237-43. [Crossref] [PubMed]
- Rossi M, Turati F, Strikoudi P, et al. Dietary intake of branched-chain amino acids and pancreatic cancer risk in a case-control study from Italy. Br J Nutr 2022; Epub ahead of print. [Crossref] [PubMed]
- Nouri-Majd S, Salari-Moghaddam A, Benisi-Kohansal S, et al. Dietary intake of branched-chain amino acids in relation to the risk of breast cancer. Breast Cancer 2022;29:993-1000. [Crossref] [PubMed]
- Rossi M, Mascaretti F, Parpinel M, et al. Dietary intake of branched-chain amino acids and colorectal cancer risk. Br J Nutr 2021;126:22-7. [Crossref] [PubMed]
- Cavallaro NL, Garry J, Shi X, et al. A pilot, short-term dietary manipulation of branched chain amino acids has modest influence on fasting levels of branched chain amino acids. Food Nutr Res 2016;60:28592. [Crossref] [PubMed]