Strategies for the acquisition of transcriptional and epigenetic information in single cells
Introduction
The fundamental unit in a multicellular organism is the single cell. To understand how the tissues develop and function, it is very important to understand the molecular and functional variation in each cell. Single cell transcription reflects single cell protein expression and single cell function (1-3), but this information has been inaccessible thus far due to the lack of approaches to consistently capture single cells and efficiently amplify their RNA transcripts. The development of microfluidic- (4-6) and droplet-based (7-9) isolation methods has greatly improved our ability to study single cells. Along this line, the creation of research tools such as CEL-seq, STRT-seq and SMART-seq2 (10,11) has significantly improved the transcriptional amplification efficiency genome-wide. These advances have now resulted in the acquisition of single cell transcriptional expression data in multiple different species and tissues for various purposes.
Epigenetic modifications directly contribute to gene transcription, they mainly consist of histone modification, DNA methylation, and chromatin accessibility (12). Compared with single cell transcriptional quantification, it is much more difficult to analyze epigenetic modifications in single cells given the presence of only two copies of genomic DNA in mononuclear cells. The recent progresses in single cell genomic DNA amplification have significantly enhanced our ability to analyze epigenetic modifications in single cells. Several different approaches such as whole genome amplification (WGA), multiple annealing and looping based amplification cycles (MALBAC), and multiple displacement amplification (MDA) had been developed to amplify genomic DNA in single cells (13,14). The profiling of DNA methylation and nucleosome position, as a measure of chromatin accessibility, in single cells have revealed novel mechanisms of gene transcription that were previously unable to be appreciated when cells were analyzed as populations. While the determination of histone modifications in single cells remain challenging thus far, an approach to indirectly measure this has been developed (15).
In parallel with the development of single cell transcriptional and epigenetic technology, there have been great progresses in single cell genomics, proteomics, and metabolomics measurements as well. These developments are outside of the scope of this review and we encourage interested readers to seek additional information on these topics in other publications (16-18).
Single cell gene expression analysis
Single cell gene expression analysis has been applied to studies in different tissues to reveal their cellular heterogeneity, expression of novel markers, presence of rare cell populations, and anatomical locations (19-22) (Figure 1). Here, we review systematically the recent progresses in this area of research for each of these purposes.
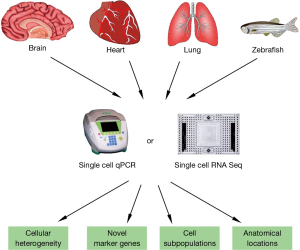
Cellular heterogeneity
The quantification of gene expression in single cells has significantly improved our understanding of cellular heterogeneity in different organ systems. For example, a malignant tumor is well known to be composed of multiple different cell types that interact in a dynamic fashion. Using single cell qPCR, a list of lineage genes expressed in epithelial cells of normal human colon vs. colon cancer can be determined (22). Furthermore, this study also showed that in colon cancer tissues, the other non-epithelial cell types were transcriptionally similar to their counterpart in normal colon tissue. Using single cell RNA sequencing, another study analyzed five types of primary glioblastomas and found significant cellular heterogeneity and diverse transcriptional regulatory programs. These new regulatory programs were shown to be important in glioblastoma biology and help to improve patient prognosis and treatment (25). The cellular heterogeneity of induced pluripotent stem cell (iPSC) and embryonic stem cell (ESC) populations have been systematically compared using single cell qPCR recently (26). The study found that iPSCs are remarkably more heterogeneous than ESCs, and iPSC displayed slower growth kinetics and impaired differentiation compared with ESCs, implicating iPSC occupy a less stable pluripotent state (26).
Novel markers and rare cell populations
By profiling genome-wide transcription in single cells, investigators have identified novel markers and rare cell populations that were previously unappreciated. For example, Treutlein et al. described single alveolar cells at four continuous differentiation stages of lung development using single cell RNA-sequencing. These authors found a wealth of cell type-specific genes including transcriptional factors (21). The support from sophisticated bioinformatic methods in analyzing single cell RNA-seq data have also greatly promoted the identification of novel marker genes and cell subpopulations. One example of such method is single-cell latent variable model (scLVM) which was used to analyze RNA-seq data of single immune cells. By considering cell cycle as a co-variate, these authors uncovered hidden subpopulations of cells during the differentiation of naive T cells into T helper 2 cells (27). Another algorithm named RaceID was developed to analyze the gene expression profiles of single intestinal cells (20). These authors identified successfully a novel gene called Reg4 to label a rare population of hormone-producing intestinal cells. Furthermore, through combining a divisive biclustering method BackSPIN with single neuron RNA-seq data, Linnarsson and colleagues identified 47 subclasses of cell types in mouse cerebral cortex as well as a novel layer I interneuron labeled by marker gene Pax6 and a new subclass of post-mitotic oligodendrocyte that specifically express Itpr2 (24).
Spatial location analysis
With single cell RNA sequencing data, investigators have been able to determine the anatomical location of single cells from various different contexts. By profiling 96 otic lineage and signal pathway genes from the otocyst of the inner ear, Heller and colleagues examined the use of these data to reconstruct the physical location of each single cell. Given the simple spherical structure of the otocyst these investigators applied a PCA-based model of this sphere in 3D using the qPCR data. In this model, the spherical structure was divided into eight quadrants along the three major axes that generate six defined hemispheres. Each of the single cells was projected onto the surface of the sphere based on its pattern of gene expression (28). In another study, the anatomical location of each embryonic zebrafish cell was determined by integrating single cell RNA-seq data with previously known in situ hybridization data. A bioinformatics algorithm named Seurat was developed in this study to identify the most differentially expressed genes among single cells and infer the spatial position of each cell. The authors further validated the anatomical mapping accuracy using different approaches to independently confirm that the Seurat method can accurately map the location of a small collection of cells as well as different rare cell populations (19). Furthermore, the anatomical location of single cells in the brain of a marine annelid Platynereis dumerilii was recently mapped by combining single cell RNA-seq data with positional gene expression profiles. These authors reported a mapping accuracy as high as 81% (29).
Single cell epigenetic analysis
Epigenetic modifications directly contributed to gene transcriptional regulation and play important roles in tissue development and disease progression. Epigenetic modifications include but are not limited to histone modification, DNA methylation, and nucleosome positioning (12) (Figure 2). Considering the large heterogeneity of transcriptional variation in cell populations, the analysis of epigenetic modifications in single cells has helped to better inform the detailed mechanism of gene expression regulation.
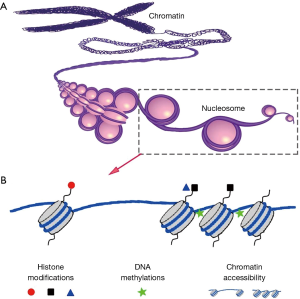
Single cell histone modifications
Histone modification is one of the most important epigenetic mechanisms, and is reported to play critical roles in multiple cell lineage specification processes. So far, two approaches have been developed to analyze histone modifications in single cells. One approach is to visualize histone modifications by combining in situ hybridization and proximity ligation assays (PLA) (30). The PLA was used to detect proximity between a biotin labeled probe targeting the MYH11 promoter and histone modification H3K4me2 at the same locus. These authors confirmed that H3K4me2 at the MYH11 locus is restricted to smooth muscle cells in both human and mouse tissue sections (30). While this method can only profile one or a few genes at a time, it has the advantage of histological localization to help verify the cell lineage specificity. To-date, there has been no reported success with chromatin immunoprecipitation (ChIP) to directly analyze histone modifications in single cells due to the very high experimental noise to detect two genomic DNA copies. However, an indirect approach called Drop-ChIP has been reported to successfully generate the histone modification profiles in single cells (15). With this approach, ChIP was used to analyze single cell chromatin in which the chromatin of each single cell was indexed and mixed as a pool. After ChIP has been performed, the ChIP products were de-multiplexed back into single cell data (Figure 3). This approach was able to successfully overcome the noise inherent in low input samples to demonstrate histone modification in single cells (15). Drop-ChIP enabled the identification of a spectrum of subpopulations of cells within ESCs marked by different histone modification signatures that have been described for pluripotency and lineage differentiation priming (15).
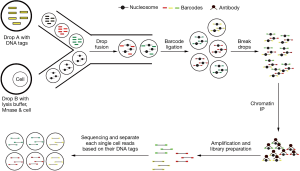
The workflow of single cell Drop-ChIP. Single cells were lysed and their chromatin was fragmented with micrococcal nuclease (MNase) in each drops. Drops with fragmented chromatin were then merged with the drops carrying different DNA barcode, and these DNA barcodes were ligated to the chromatin fragments from each drop to index them to the original cells. The merged drops were combined and the pool of bar-coded chromatin was then used for immunoprecipitation by various histone modification-specific antibodies. The purified DNA fragments were then further amplified and used for library preparation and sequencing. Finally, based on the barcode information, sequencing reads were partitioned to generate each single cell chromatin profiles.
Single cell DNA methylation
To analyze DNA methylation in single cells, a single-base resolution DNA methylation analysis method using reduced representation bisulfite sequencing (scRRBS) was firstly developed (31). This method was used to characterize the DNA methylation changes of maternal and paternal genomes after fertilization within individual pronuclei of mouse zygotes. Subsequently, a whole-genome single-cell bisulfite sequencing (scBS-seq) method was reported to be able to measure DNA methylation at approximately 50% of all CpG sites. It was also applied successfully to identify the DNA methylation heterogeneity in ESCs cultured in the presence of serum or 2i medium (32). Furthermore, another whole genome bisulfite sequencing method was developed for single cells (scWGBS) and was optimized to profile many samples at low coverage and reduce the per cell sequencing cost (33). While these assays were developed to analyze a snapshot of single cell DNA methylation status, a recent study demonstrated the ability to monitor the DNA methylation dynamics using a genomic methylation reporter. This reporter was inserted into promoter-associated CpG islands and non-coding regulatory elements to report the loci specific DNA methylation changes during cell state transitions, and they found dynamic de novo DNA methylation during mouse ES cell differentiation and significant DNA demethylation during mouse fibroblast reprogramming into iPSCs (34).
Single cell nucleosome positioning
The binding of transcription factors and epigenetic modifiers to genomic DNA requires the movement of nucleosome to increase chromatin accessibility. Hence, nucleosome positioning plays a critical role in regulating the availability of transcription factors and RNA polymerase to their target gene loci and genes expression. To analyze the position of nucleosomes in single cells, Small et al. developed a novel technique that takes advantage of the ability of nucleosome to protect DNA from GpC methylation to identify the nucleosome position at the promoter region of the PHO5 locus (35). These investigators found significant cell-to-cell variation in nucleosome positions and nucleosome positioning shifts correlates with gene expression changes. Furthermore, through combining a microfluidics platform with assay for transposase-accessible chromatin using sequencing (ATAC-seq), Greenleaf, Chang, and colleagues reported the development of single cell ATAC-seq (scATAC-seq) and used it to analyze the genome-wide chromatin accessibility in multiple types of single cells. Using scATAC-seq, significant cell-to-cell accessibility variance was observed and the variation was found to associate with specific cis-elements and trans-factors (36). Additionally, they found some trans-factors such as CTCF can buffer variability, but some other trans-factors such as P300 can amplify variability (36). Meanwhile, Shendure and colleagues used a similar approach to measure the chromatin accessibility in thousands of single cells, and they successfully identified functionally relevant differences in accessibility between cell types and between subtypes within an apparently homogeneous cell population (37). Recently, a single cell DNase sequencing (scDNase-seq) method was reported to detect genome-wide DNase hypersensitive sites (DHSs) (38). DHSs generally correlate with the chromatin states of important transcriptional regulatory elements. With scDNASE-seq, the chromatin regions with multiple active histone modifications were found to display constitutive DHSs but the ones with fewer histone modifications exhibit high variation of DHSs among single cells (38). The cell-to-cell variation in DHSs can also be used to predict gene expression levels.
Clinical applications of single cell techniques
Clinical diagnosis and therapies have also greatly benefited from the advance of single cell techniques. Rare circulating tumor cells (CTCs) in patients’ blood can be detected by single cell genomic sequencing analysis to provide cancer prognosis, monitor disease progression, and evaluate therapy efficiency (39). Given that cancer tissue is quite complex and cancer cells respond heterogeneously to drug treatment (40), single cell RNA-seq has been applied to investigate the cellular response of metastatic human breast cancer cells to paclitaxel treatment. This study showed that drug-tolerant cells expressed specific RNA variants involved in microtubule organization and cell surface signaling (41). Single cell epigenetics can also provide valuable insights in understanding disease mechanisms and providing clues for clinical therapies. For example, ATAC-seq has been optimized to assay the T cells and B cells of patients on a clinical timescale, and the generated information can be used to assemble a personalized regulatory network for disease prognosis (42). Additionally, scDNase-seq has been applied to normal and thyroid cancer samples to successfully identify single mutation within a DHS site that disrupted p53 protein binding resulting in down-regulated target gene TXNL1 expression (38).
Summary and future perspectives
Single cell transcriptional and epigenetic profiling is a powerful method to dissect tissue complexity and elucidate novel markers and cell populations. It has also shown promise in analyzing clinical samples with rare cell number. Single cell genome-wide transcriptional profiling is becoming a well-established method for determination of the anatomical location of single cells from various developmental and disease contexts. In the future, we envision an increased ability to combine genome-wide single cell gene expression profiling with histological information to determine the influence of cell-cell interaction on transcription. Furthermore, we predict that single cell epigenetics profiling will become more sophisticated and sensitive to help further delineate the mechanisms that regulate gene expression at a single cell level. Ultimately, the combination of single cell expression and epigenetic profiling will prove to be extremely valuable for further understanding of the natural variation of gene expression and their regulation.
Acknowledgements
Funding: This work was supported by a post-doctoral fellowship from the Child Health Research Institute at Stanford (to G Li), by the German Heart Foundation (to E Dzilic), and by the NIH Progenitor Cell Biology Consortium (U01 HL099776), the NIH Director’s Pioneer Award (DP1 LM012179-01), and the Endowed Faculty Scholar Award of Lucile Packard Foundation for Children and Child Health Research Institute at Stanford (to SM Wu).
Footnote
Conflicts of Interest: The authors have no conflicts of interest to declare.
References
- Albayrak C, Jordi CA, Zechner C, et al. Digital Quantification of Proteins and mRNA in Single Mammalian Cells. Mol Cell 2016;61:914-24. [Crossref] [PubMed]
- Tian Q, Stepaniants SB, Mao M, et al. Integrated genomic and proteomic analyses of gene expression in Mammalian cells. Mol Cell Proteomics 2004;3:960-9. [Crossref] [PubMed]
- Schwanhäusser B, Busse D, Li N, et al. Global quantification of mammalian gene expression control. Nature 2011;473:337-42. [Crossref] [PubMed]
- Kalisky T, Quake SR. Single-cell genomics. Nat Methods 2011;8:311-4. [Crossref] [PubMed]
- Marcus JS, Anderson WF, Quake SR. Microfluidic single-cell mRNA isolation and analysis. Anal Chem 2006;78:3084-9. [Crossref] [PubMed]
- Sanchez-Freire V, Ebert AD, Kalisky T, et al. Microfluidic single-cell real-time PCR for comparative analysis of gene expression patterns. Nat Protoc 2012;7:829-38. [Crossref] [PubMed]
- Klein AM, Mazutis L, Akartuna I, et al. Droplet barcoding for single-cell transcriptomics applied to embryonic stem cells. Cell 2015;161:1187-201. [Crossref] [PubMed]
- Macosko EZ, Basu A, Satija R, et al. Highly Parallel Genome-wide Expression Profiling of Individual Cells Using Nanoliter Droplets. Cell 2015;161:1202-14. [Crossref] [PubMed]
- Mazutis L, Gilbert J, Ung WL, et al. Single-cell analysis and sorting using droplet-based microfluidics. Nat Protoc 2013;8:870-91. [Crossref] [PubMed]
- Grün D, van Oudenaarden A. Design and Analysis of Single-Cell Sequencing Experiments. Cell 2015;163:799-810. [Crossref] [PubMed]
- Picelli S, Björklund ÅK, Faridani OR, et al. Smart-seq2 for sensitive full-length transcriptome profiling in single cells. Nat Methods 2013;10:1096-8. [Crossref] [PubMed]
- Li E. Chromatin modification and epigenetic reprogramming in mammalian development. Nat Rev Genet 2002;3:662-73. [Crossref] [PubMed]
- Zong C, Lu S, Chapman AR, et al. Genome-wide detection of single-nucleotide and copy-number variations of a single human cell. Science 2012;338:1622-6. [Crossref] [PubMed]
- de Bourcy CF, De Vlaminck I, Kanbar JN, et al. A quantitative comparison of single-cell whole genome amplification methods. PLoS One 2014;9:e105585. [Crossref] [PubMed]
- Rotem A, Ram O, Shoresh N, et al. Single-cell ChIP-seq reveals cell subpopulations defined by chromatin state. Nat Biotechnol 2015;33:1165-72. [Crossref] [PubMed]
- Spitzer MH, Nolan GP. Mass Cytometry: Single Cells, Many Features. Cell 2016;165:780-91. [Crossref] [PubMed]
- Gawad C, Koh W, Quake SR. Single-cell genome sequencing: current state of the science. Nat Rev Genet 2016;17:175-88. [Crossref] [PubMed]
- Zenobi R. Single-cell metabolomics: analytical and biological perspectives. Science 2013;342:1243259. [Crossref] [PubMed]
- Satija R, Farrell JA, Gennert D, et al. Spatial reconstruction of single-cell gene expression data. Nat Biotechnol 2015;33:495-502. [Crossref] [PubMed]
- Grün D, Lyubimova A, Kester L, et al. Single-cell messenger RNA sequencing reveals rare intestinal cell types. Nature 2015;525:251-5. [Crossref] [PubMed]
- Treutlein B, Brownfield DG, Wu AR. Reconstructing lineage hierarchies of the distal lung epithelium using single-cell RNA-seq. Nature 2014;509:371-5. [Crossref] [PubMed]
- Dalerba P, Kalisky T, Sahoo D, et al. Single-cell dissection of transcriptional heterogeneity in human colon tumors. Nat Biotechnol 2011;29:1120-7. [Crossref] [PubMed]
- Li G, Plonowska K, Kuppusamy R, et al. Identification of cardiovascular lineage descendants at single-cell resolution. Development 2015;142:846-57. [Crossref] [PubMed]
- Zeisel A, Muñoz-Manchado AB, Codeluppi S, et al. Brain structure. Cell types in the mouse cortex and hippocampus revealed by single-cell RNA-seq. Science 2015;347:1138-42. [Crossref] [PubMed]
- Patel AP, Tirosh I, Trombetta JJ, et al. Single-cell RNA-seq highlights intratumoral heterogeneity in primary glioblastoma. Science 2014;344:1396-401. [Crossref] [PubMed]
- Narsinh KH, Sun N, Sanchez-Freire V, et al. Single cell transcriptional profiling reveals heterogeneity of human induced pluripotent stem cells. J Clin Invest 2011;121:1217-21. [Crossref] [PubMed]
- Buettner F, Natarajan KN, Casale FP, et al. Computational analysis of cell-to-cell heterogeneity in single-cell RNA-sequencing data reveals hidden subpopulations of cells. Nat Biotechnol 2015;33:155-60. [Crossref] [PubMed]
- Durruthy-Durruthy R, Gottlieb A, Hartman BH, et al. Reconstruction of the mouse otocyst and early neuroblast lineage at single-cell resolution. Cell 2014;157:964-78. [Crossref] [PubMed]
- Achim K, Pettit JB, Saraiva LR, et al. High-throughput spatial mapping of single-cell RNA-seq data to tissue of origin. Nat Biotechnol 2015;33:503-9. [Crossref] [PubMed]
- Gomez D, Shankman LS, Nguyen AT, et al. Detection of histone modifications at specific gene loci in single cells in histological sections. Nat Methods 2013;10:171-7. [Crossref] [PubMed]
- Guo H, Zhu P, Wu X, et al. Single-cell methylome landscapes of mouse embryonic stem cells and early embryos analyzed using reduced representation bisulfite sequencing. Genome Res 2013;23:2126-35. [Crossref] [PubMed]
- Smallwood SA, Lee HJ, Angermueller C, et al. Single-cell genome-wide bisulfite sequencing for assessing epigenetic heterogeneity. Nat Methods 2014;11:817-20. [Crossref] [PubMed]
- Farlik M, Sheffield NC, Nuzzo A, et al. Single-cell DNA methylome sequencing and bioinformatic inference of epigenomic cell-state dynamics. Cell Rep 2015;10:1386-97. [Crossref] [PubMed]
- Stelzer Y, Shivalila CS, Soldner F, et al. Tracing dynamic changes of DNA methylation at single-cell resolution. Cell 2015;163:218-29. [Crossref] [PubMed]
- Small EC, Xi L, Wang JP, et al. Single-cell nucleosome mapping reveals the molecular basis of gene expression heterogeneity. Proc Natl Acad Sci U S A 2014;111:E2462-71. [Crossref] [PubMed]
- Buenrostro JD, Wu B, Litzenburger UM, et al. Single-cell chromatin accessibility reveals principles of regulatory variation. Nature 2015;523:486-90. [Crossref] [PubMed]
- Cusanovich DA, Daza R, Adey A, et al. Multiplex single cell profiling of chromatin accessibility by combinatorial cellular indexing. Science 2015;348:910-4. [Crossref] [PubMed]
- Jin W, Tang Q, Wan M, et al. Genome-wide detection of DNase I hypersensitive sites in single cells and FFPE tissue samples. Nature 2015;528:142-6. [PubMed]
- Navin NE. The first five years of single-cell cancer genomics and beyond. Genome Res 2015;25:1499-507. [Crossref] [PubMed]
- Dean M, Fojo T, Bates S. Tumour stem cells and drug resistance. Nat Rev Cancer 2005;5:275-84. [Crossref] [PubMed]
- Ting DT, Wittner BS, Ligorio M, et al. Single-cell RNA sequencing identifies extracellular matrix gene expression by pancreatic circulating tumor cells. Cell Rep 2014;8:1905-18. [Crossref] [PubMed]
- Buenrostro JD, Giresi PG, Zaba LC, et al. Transposition of native chromatin for fast and sensitive epigenomic profiling of open chromatin, DNA-binding proteins and nucleosome position. Nat Methods 2013;10:1213-8. [Crossref] [PubMed]