Risk factors and prognostic modeling in bridging extracorporeal membrane oxygenation patients before lung transplantation
Highlight box
Key findings
• This study identified several risk factors affecting the prognosis of patients undergoing preoperative extracorporeal membrane oxygenation (ECMO) bridging, including being over 51 years old, having a high body mass index (BMI), a history of pre-transplant dialysis, donor hypertension, prolonged cold ischemia time, and high serum total bilirubin levels. Based on these factors, a survival prognosis model was established and its accuracy was validated.
What is known and what is new?
• An increasing number of lung transplant patients are opting to use preoperative bridging ECMO to extend their waiting time for a lung donation. As this technology matures, preoperative bridging ECMO is no longer considered a risk factor affecting patient prognosis.
• This study analyzed the prognostic risk factors of lung transplant patients bridged with ECMO, constructing a survival prognostic model to assist clinical doctors effectively. This model enables clinicians to more accurately assess the survival risk of lung transplant patients undergoing preoperative ECMO bridging, optimize treatment plans, and improve surgical success rates.
What is the implication, and what should change now?
• With an increasing number of patients opting for ECMO preoperatively to extend the waiting time for lung donor matching, this study provides valuable insights for patient selection and prognosis assessment. However, the variables used in this study are limited by the statistical data from the database, and their accuracy needs to be validated through further large-scale multicenter studies. Future research should focus on expanding the sample size, incorporating additional influencing factors, and improving the accuracy and generalizability of predictions.
Introduction
Lung transplantation is regarded as an effective intervention for terminal lung diseases when conventional medical treatments have proven ineffective. While there has been a continuous advancement in the associated surgical procedure, a tangible issue is the scarcity of available organs (1). This shortage results in postponed surgeries for those requiring immediate lung transplantation. The data from the Organ Procurement and Transplantation Network/Scientific Registry of Transplant Recipients (OPTN/SRTR) published in 2017 showed that about 10% to 20% of patients die while awaiting a lung donor (2). Among patients unable to sustain life via conventional mechanical ventilation, extracorporeal membrane oxygenation (ECMO) is occasionally employed to assist respiratory function and extend survival before lung transplantation. Short-term ECMO use can act as a feasible emergency measure before surgery. Over the past decade, the utilization of ECMO as a bridge to lung transplantation has progressively increase (3).
ECMO bridge technology is relatively new. Until a few years ago, ECMO was considered a contraindication for lung transplantation due to potential complications like hemolysis, bleeding, stroke, infection, and renal insufficiency from prolonged ECMO support (4). However, recent research indicates that advances in ECMO technology and management have provided increasing evidence supporting the safety and utility of bridging strategies in lung transplantation (5-7). Recent studies from high-volume centers have shown similar outcomes between ECMO-bridged and non-bridged lung transplant patients (7-9). Findings on the use of ECMO in lung transplantation patients were derived from a high-volume transplant center in Toronto. The researchers reported favorable short-term and long-term results from ECMO bridging therapy prior to the initial lung transplantation, despite a notable reduction in survival of patients undergoing re-transplantation (10).
Pre-transplantation bridging with ECMO has been increasingly favored as the primary auxiliary method to extend the lives of those awaiting lung transplantation. However, given the limitation of a small sample size, there is currently no reliable prognostic model to forecast the survival of patients on bridging ECMO. The precise survival prediction necessitates the development of a credible predictive model that employs clinical indicators and patient characteristics for evaluation of the survival likelihood among patients bridged with ECMO before lung transplantation. Such a model will help physicians to better assess patient conditions, optimize treatment strategies, and enhance patient care and support.
To this end, we began a retrospective review of the United Network for Organ Sharing (UNOS) database spanning the past 20 years concerning ECMO bridging. A reliable forecast of preoperative ECMO-bridged patients is vital to aid the treating physician in decision making related to adjunctive therapies and follow-up methods and frequencies as well as to provide valuable information about treatment options and short-term and long-term outcomes to patients and their families. Therefore, this study aims to identify the demographic and clinical data associated with post-lung transplantation survival and recurrence risk in a large, multicenter cohort of preoperative ECMO-bridged patients. Such a long-term survival prediction model will aid in clinical decision-making and offer more accurate prognostic assessments. In particular, we seek to create and internally validate a nomogram that predicts the individual risk of post-lung transplantation survival time for patients. We present this article in accordance with the TRIPOD reporting checklist (available at https://jtd.amegroups.com/article/view/10.21037/jtd-24-430/rc).
Methods
Patient cohorts
Data for this study were gathered from a retrospective multicenter database. Our retrospective cohort study utilized standardized transplantation analysis and research files procured from UNOS that encompassed data from all solid organ transplant patients in the United States up August 2001 to April 2022. We obtained permission to use the database by requesting access via email. The analysis was conducted in July 2023 and included all adult patients (18 years and older) who underwent lung transplantation with preoperative ECMO. Patients lacking relevant predictive indicators or follow-up data were not considered for the study. The final analysis cohort comprised 1,202 patients. Because this research is the study of a public database, it does not involve ethical issues. The original data were partitioned into five equal subsets, with each subset serving as the test set in rotation and the remaining four subsets formed the training set. The study was conducted in accordance with the Declaration of Helsinki (as revised in 2013).
Inclusion criteria for the study were as follows: (I) all patients received ECMO treatment before undergoing lung transplant surgery; (II) all patients were at least 18 years old; (III) patients had no history of other transplant surgeries. Exclusion criteria included: (I) presence of other malignant tumors before surgery; (II) missing relevant data in the database [includes recipient age, gender, body mass index (BMI), history of diabetes, history of tumors, acute rejection reactions, infection status in the 2 weeks before surgery, history of cardiac surgery, pre-transplant dialysis history, lung donor category, recipient functional status, waiting time, use of mechanical ventilation, smoking history, donor age, donor smoking history, cold ischemia time, and serum total bilirubin]; (III) loss to follow-up during the study period. Ultimately, 1,202 patients who underwent ECMO as a bridge to lung transplantation were included in the analysis.
Data collection
We gathered baseline and clinical information from patients, including their age, gender, body BMI, diabetes status, previous malignancy, acute rejection incidents, pre-transplant dialysis, infection 2 weeks before transplantation, prior cardiac surgery, recipient function status, total days in waiting list, ventilatory support since listing, history of cigarette use, donor’s history of hypertension, donor’s age, donor-cardiac arrest following brain death, history of diabetes in the donor, cold ischemia time and serum total bilirubin levels. In this study, institutional review board approval was not required because the cohort data were anonymous and publicly available. A total of 1,202 adult patients (age >18 years) who underwent preoperative ECMO and the subsequent lung transplantation were analyzed in this study. All baseline characteristics are presented in Table 1. We determined cut-off values for age, BMI, waiting duration, ischemic time, and serum total bilirubin, and then converted metrics into categorical variables. All variables, with the exception of age, were incorporated into the regression model as continuous variables for analysis. Continuous variables were transformed into binary categories with the intent to enhance the visual representation of each variable’s impact on patient prognosis through the Kaplan-Meier curve.
Table 1
Characteristic | ECMO (n=1,202) | Train set (n=961) | Test set (n=241) |
---|---|---|---|
Age (years) | 46.02±14.323 | 46.06±15.673 | 45.86±14.472 |
≥51 | 550 (45.8) | 436 (45.4) | 114 (47.3) |
<51 | 652 (54.2) | 525 (54.6) | 127 (52.7) |
Gender | |||
Male | 803 (66.8) | 635 (66.1) | 168 (69.7) |
Female | 399 (33.2) | 326 (33.9) | 73 (30.3) |
BMI (kg/m2) | 26.55±5.357 | 26.59±5.423 | 26.40±5.324 |
≥28.15 | 441 (36.7) | 351 (36.5) | 90 (37.3) |
<28.15 | 761 (63.3) | 610 (63.5) | 151 (62.7) |
Recipient diabetes | |||
Yes | 317 (26.4) | 253 (26.3) | 64 (26.6) |
No | 885 (73.6) | 708 (73.7) | 177 (73.4) |
Previous malignancy | |||
Yes | 77 (6.4) | 61 (6.3) | 16 (6.6) |
No | 1,125 (93.6) | 900 (93.7) | 225 (93.4) |
Acute rejection | |||
Yes | 171 (14.2) | 144 (15.0) | 27 (11.2) |
No | 1,031 (85.8) | 817 (85.0) | 214 (88.8) |
Infection 2 weeks before transplantation | |||
Yes | 493 (41.0) | 397 (41.3) | 96 (39.8) |
No | 709 (59.0) | 564 (58.7) | 145 (60.2) |
Prior cardiac surgery | |||
Yes | 216 (18.0) | 176 (18.3) | 40 (16.6) |
No | 986 (82.0) | 785 (81.7) | 201 (83.4) |
Pre-transplant dialysis | |||
Yes | 297 (24.7) | 230 (24.0) | 67 (27.8) |
No | 905 (75.3) | 731 (76.0) | 174 (72.2) |
Donor-cardiac arrest post brain death | |||
Yes | 84 (7.0) | 67 (7.0) | 17 (7.0) |
No | 1,118 (93.0) | 894 (93.0) | 224 (93.0) |
Recipient function status | |||
Very sick | 404 (33.6) | 319 (33.2) | 85 (35.3) |
Relatively better | 798 (66.4) | 642 (66.8) | 156 (64.7) |
Total days in waiting list | 34.678±114.361 | 35.36±114.723 | 31.95±113.274 |
≥26 | 227 (18.9) | 186 (19.4) | 41 (17.0) |
<26 | 975 (81.1) | 775 (80.6) | 200 (83.0) |
Ventilatory support since listing | |||
Yes | 853 (71.0) | 690 (71.8) | 163 (67.6) |
No | 349 (29.0) | 271 (28.2) | 78 (32.4) |
History of cigarette use | |||
Yes | 360 (30.0) | 287 (29.9) | 73 (30.3) |
No | 842 (70.0) | 674 (70.1) | 168 (69.7) |
History of donor hypertension | |||
Yes | 233 (19.4) | 191 (19.9) | 42 (17.4) |
No | 969 (80.6) | 770 (80.1) | 199 (82.6) |
Donor age (years) | 33.52±12.393 | 33.42±13.172 | 33.92±12.271 |
≥43 | 303 (25.2) | 242 (25.2) | 61 (25.3) |
<43 | 899 (74.8) | 719 (74.8) | 180 (74.7) |
History of diabetes in the donor | |||
Yes | 54 (4.5) | 41 (4.3) | 13 (5.4) |
No | 1,148 (95.5) | 920 (95.7) | 228 (94.6) |
Cold ischemic time (hours) | 4.743±1.996 | 4.733±2.034 | 4.784±1.762 |
≥4.34 | 592 (49.3) | 465 (48.4) | 127 (52.7) |
<4.34 | 610 (50.7) | 496 (51.6) | 114 (47.3) |
Serum total bilirubin (mg/dL) | 1.512±2.214 | 1.490±2.032 | 1.963±2.334 |
≥1.35 | 366 (30.4) | 285 (29.7) | 81 (33.6) |
<1.35 | 836 (69.6) | 676 (70.3) | 160 (66.4) |
Data are presented as n (%) or mean ± SD. BMI, body mass index; ECMO, extracorporeal membrane oxygenation; SD, standardized difference.
Statistical analysis
An initial evaluation was performed on clinical variables associated with survival, as guided by clinical relevance, scientific understanding, and prior research. A Cox proportional hazard regression model was used to assess the prognostic significance of each factor discerned in the univariate analysis. In addition, a multivariate survival analysis was performed using the same model to independently account for potential confounders related to the link between clinical factors and survival duration. A multivariable model was developed based on the factors that demonstrated significance in the univariate analysis. A nomogram was subsequently generated to design a statistical prognostic model capable of anticipating the 1-year survival probability. Internal validation was performed using N-fold cross-validation. To mitigate the impact of data segmentation, current k-fold cross-validation was typically executed multiple times (200 instances), i.e., 200 rounds of 5-fold cross-validations were conducted.
Continuous variables were expressed as mean ± standard deviation, categorical variables as percentages, and ordinal variables as median (interquartile range). Survival estimates were derived based on the Kaplan-Meier product limit method. Cox regression analysis was employed to evaluate factors related to ECMO support, and the findings were presented as hazard ratios and 95% confidence intervals. A P value <0.05 was considered as statistically significant. Statistical analysis was carried out using R (version 3.5.1, R Development Core Team).
Results
Survival analysis in the training set
We performed univariate Cox analysis using the selected variables, and found that the factors such as patient age, BMI, diabetes, infection 2 weeks before transplantation, acute rejection reaction, pre-transplant dialysis, total days in waiting list, ventilatory support since listing, donor hypertension history, ischemic duration, and serum total bilirubin were significantly linked to post-transplant survival duration. The outcomes of the univariate analysis are presented in Table 2. The variables with a P value <0.05 were subsequently subjected to multivariate Cox analysis. Multivariable analysis (Table 3) identified age, BMI, pre-transplant dialysis, donor hypertension history, ischemic duration, and serum bilirubin as independent prognostic factors for ECMO-supported lung transplant patients. Survival rates among patients were assessed using Kaplan-Meier curves.
Table 2
Characteristic factor | HR | 95% CI | P value |
---|---|---|---|
Age | 1.529 | 1.202–1.944 | <0.001 |
Gender | 0.921 | 0.7178–1.181 | 0.52 |
BMI | 1.028 | 1.006–1.051 | 0.01 |
Recipient diabetes | 1.485 | 1.152–1.915 | 0.002 |
Previous malignancy | 1.298 | 0.823–2.047 | 0.26 |
Acute rejection | 0.613 | 0.421–0.892 | 0.01 |
Infection 2 weeks before transplantation | 1.395 | 1.097–1.774 | 0.007 |
Prior cardiac surgery | 1.147 | 0.852–1.545 | 0.36 |
Pre-transplant dialysis | 3.884 | 3.045–4.956 | <0.001 |
Donor-cardiac arrest post brain death | 1.340 | 0.881–2.039 | 0.17 |
Recipient function status | 1.240 | 0.969–1.587 | 0.09 |
Total days in waiting list | 0.535 | 0.375–0.763 | <0.001 |
Ventilatory support since listing | 1.420 | 1.070–1.884 | 0.02 |
History of cigarette use | 1.283 | 0.996–1.653 | 0.054 |
History of donor hypertension | 1.527 | 1.158–2.015 | 0.003 |
Donor age | 1.263 | 0.975–1.638 | 0.08 |
History of diabetes in the donor | 1.156 | 0.648–2.063 | 0.62 |
Ischemic time | 1.109 | 1.049–1.173 | <0.001 |
Serum total bilirubin | 1.080 | 1.041–1.120 | <0.001 |
BMI, body mass index; HR, hazard ratio; CI, confidence interval.
Table 3
Characteristic factor | HR | 95% CI | P value |
---|---|---|---|
Age | 1.0041 | 1.1097–1.835 | 0.006 |
BMI | 1.0288408 | 1.0048–1.053 | 0.02 |
Recipient diabetes | 1.2140146 | 0.9337–1.578 | 0.15 |
Acute rejection | 0.8011818 | 0.5471–1.173 | 0.26 |
Infection 2 weeks before transplantation | 1.1608768 | 0.9004–1.497 | 0.25 |
Pre-transplant dialysis | 3.2949081 | 2.5518–4.254 | <0.001 |
Total days in waiting list | 0.9991596 | 0.9974–1.001 | 0.34 |
Ventilatory support since listing | 1.3455167 | 0.9801–1.847 | 0.07 |
History of donor hypertension | 1.3321192 | 1.0013–1.772 | 0.049 |
Ischemic time | 1.1018129 | 1.0375–1.170 | 0.002 |
Serum total bilirubin | 1.0578941 | 1.0157–1.102 | 0.007 |
BMI, body mass index; HR, hazard ratio; CI, confidence interval.
The Kaplan-Meier curve showed that the 1-year survival rate post-lung transplantation was significantly lower for patients older than 51 years compared to those younger than 51 years (Figure 1). Additionally, the 1-year survival post-surgery survival rate was significantly lower for patients who required dialysis prior to the procedure compared to those who did not (Figure 1). Patients with a BMI exceeding 28.15 kg/m2, ischemic durations over 4.34 hours, and serum total bilirubin levels above 13.5 mg/dL had a worse prognosis compared to their peers (Figure 1).
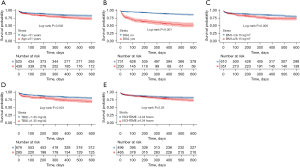
Nomograms of the prognostic model
A predictive model was developed through multivariate analysis. Considering the real-world circumstances, we integrated six statistically significant variables into this model based on the multivariate analysis. The model achieved a C-index of 0.731 and an area under the curve (AUC) of 0.780 for predicting the 1-year survival rate (Figure 2). Internal validation using AUC indicated the model’s precision in predicting the 1-year survival rate, with an AUC of 0.712 (Figure 2). After 200 rounds of 5-fold cross-validation, the average AUC was 0.760. The constructed nomogram is shown in Figure 3.
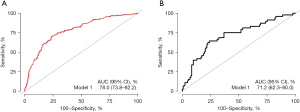
In the training set, calibration curves showed strong alignment between nomogram-estimated risk and actual observed risk for predicting 1-year survival (Figure 4).
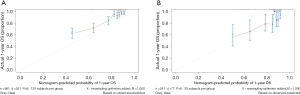
Discussion
In our study, we analyzed the risk factors influencing the prognosis of patients undergoing preoperative ECMO bridging. By examining the clinical data of 1,202 patients, we identified that age over 51, high BMI, a history of dialysis before transplantation, donor hypertension, prolonged cold ischemia time, and high serum total bilirubin are adverse prognostic factors for the survival of ECMO-bridged lung transplant patients. Additionally, we developed a nomogram to predict patient survival.
Advanced age has traditionally been a contraindication for the use of extracorporeal life support in lung transplants. Recent studies on the risk factors of bridging ECMO have consistently mentioned the impact of age on prognosis (3). Articles report that being over 35 years old is an independent risk factor for bridging treatment. Our research also indicates that patients aged 51 and older have poorer outcomes, likely due to decreased physical fitness and comorbidities.
Our research indicates that lung transplant patients with high BMI have poorer outcomes. Previous studies have similarly reported increased mortality rates in recipients with abnormal BMI before transplantation. A recent report by the International Society for Heart and Lung Transplantation (ISHLT) highlighted a significant association between pre-transplant BMI >30 kg/m2 and 1-year mortality rates (11). The mechanisms underlying the increased mortality due to severe obesity remain unclear but are suspected to relate to early postoperative complications.
Systemic hemodynamic instability during lung transplantation, requirement for ECMO support, and administration of postoperative immunosuppressive medications are the factors that contribute to the development of acute kidney injury (AKI) (12). Gan et al. noted a rising trend in the need for early hemodialysis following lung transplantation, as both 1- and 5-year survival rates significantly declined among patients requiring dialysis (13). This highlights the crucial relationship between renal function and postoperative survival. Studies have demonstrated that the use of ECMO is one of the risk factors for AKI. These results are consistent with our study, suggesting that the outlook for lung transplant recipients who underwent preoperative dialysis—indicative of compromised renal function—is less favorable compared to those without prior dialysis. This study identified a significant correlation between serum total bilirubin level and 1-year survival among lung transplant patients supported by ECMO. This finding is in line with previous studies that have demonstrated a significant link between serum total bilirubin concentration and early mortality in lung transplant recipients (14). Furthermore, the comparison between patients supported by ECMO and those without such support revealed significantly higher bilirubin levels in the former group (4). Elevated hyperbilirubinemia after ECMO initiation is one of the main factors leading to decreased survival rates (15). Prolonged hypertension and ischemia time have been identified in related studies to be significant adverse factors for the prognosis of lung transplant patients (16,17).
ECMO, a salvage therapy, is increasingly applied in clinical settings and has been reported to have a success rate of 50–83% (18). Despite higher perioperative risks and worse long-term survival rates, ECMO has been effective for patients awaiting lung transplants (19). While it also poses specific risks such as bleeding, neurological injury, and sepsis, recent developments in intensive care unit (ICU) and ECMO management have minimized these risks (20). ECMO serves as a critical intervention for those with rapidly worsening end-stage lung disease by providing optimized gas exchange and end-organ perfusion. Studies show that patients bridged to their first transplant had slightly worse outcomes than non-bridged recipients (10). Despite longer surgery time, ICU stay, and post-transplant hospital stay, ECMO-bridged patients showed no significant differences in postoperative complications, functional status at discharge, or long-term survival (21). Preoperative ECMO bridging is widely recognized in the industry as a safe means to prolong the wait for lung transplantation in patients. ECMO not only serves as a supportive therapy in the surgical setting but also allows the lungs to rest, enabling transplanted organs time to recover from ischemia-reperfusion injury (22). For patients with pulmonary arterial hypertension, ECMO bridging can improve left ventricular chronic underfilling and acute right ventricular remodeling, thus facilitating perioperative management (23).
The development of this prognosis model provides valuable decision support for clinicians, empowering them to more accurately evaluate the survival risks of patients undergoing lung transplantation with preoperative ECMO bridging, optimize treatment plans, and enhance surgical success rates. Furthermore, this model introduces novel ideas and approaches for future disease prediction and personalized treatment research. As more patients opt for ECMO before surgery to extend the waiting time for lung donor matching, this study will provide some reference value in patient selection and prognosis assessment.
Nonetheless, the variables employed in the present study were constrained by the statistical data derived from the database, thereby making it unfeasible to incorporate additional variables potentially associated with survival. The implementation of postoperative dialysis, despite having specific criteria, is subject to a degree of subjectivity owing to elements such as medical equipment, patient financial status, and family consent. This might pose a potential challenge to accurately portray the patients’ actual conditions. Furthermore, there have been studies indicating that individuals who overcome severe illness with ECMO support exhibit subpar health-related quality of life (HRQL) post-lung transplantation and generally display poorer physical functioning than healthy individuals. In addition, they have higher incidences of depressive symptoms (24,25) and restrictions in physical and emotional health (26-28). Therefore, given the database’s constraints, there are numerous factors we could not incorporate into the study. Hence, it is crucial to consider the relevant factors of ECMO treatment in the predictive model to obtain a precise evaluation of patients’ long-term survival.
This predictive model developed herein provides decision support for ECMO patients undergoing lung transplantation, but its accuracy needs validation through further large-scale multicenter studies. The model should also offer personalized predictions adaptable to different clinical environments and patient characteristics. Despite potential clinical applications, the model needs further optimization and validation in different practices before actual clinical applicability. Future studies should be conducted by expanding the sample size, incorporating additional influencing factors, and improving predictive accuracy and generalizability.
Conclusions
In conclusion, our research results suggest that age, BMI, preoperative dialysis history, donor hypertension history, ischemia time, and total serum bilirubin are the main factors affecting patient survival rate. Additionally, this study successfully constructed a nomogram to predict the prognosis of patients bridged to ECMO before surgery based on clinical and pathological characteristics. This provides a valuable reference for predicting the survival period of each patient undergoing preoperative ECMO bridging.
Acknowledgments
Funding: None.
Footnote
Reporting Checklist: The authors have completed the TRIPOD reporting checklist. Available at https://jtd.amegroups.com/article/view/10.21037/jtd-24-430/rc
Peer Review File: Available at https://jtd.amegroups.com/article/view/10.21037/jtd-24-430/prf
Conflicts of Interest: All authors have completed the ICMJE uniform disclosure form (available at https://jtd.amegroups.com/article/view/10.21037/jtd-24-430/coif). The authors have no conflicts of interest to declare.
Ethical Statement: The authors are accountable for all aspects of the work in ensuring that questions related to the accuracy or integrity of any part of the work are appropriately investigated and resolved. The study was conducted in accordance with the Declaration of Helsinki (as revised in 2013).
Open Access Statement: This is an Open Access article distributed in accordance with the Creative Commons Attribution-NonCommercial-NoDerivs 4.0 International License (CC BY-NC-ND 4.0), which permits the non-commercial replication and distribution of the article with the strict proviso that no changes or edits are made and the original work is properly cited (including links to both the formal publication through the relevant DOI and the license). See: https://creativecommons.org/licenses/by-nc-nd/4.0/.
References
- Perch M, Hayes D Jr, Cherikh WS, et al. The International Thoracic Organ Transplant Registry of the International Society for Heart and Lung Transplantation: Thirty-ninth adult lung transplantation report-2022; focus on lung transplant recipients with chronic obstructive pulmonary disease. J Heart Lung Transplant 2022;41:1335-47. [Crossref] [PubMed]
- Valapour M, Lehr CJ, Schladt DP, et al. OPTN/SRTR 2022 Annual Data Report: Lung. Am J Transplant 2024;24:S394-456. [Crossref] [PubMed]
- Tsiouris A, Budev MM, Yun JJ. Extracorporeal Membrane Oxygenation as a Bridge to Lung Transplantation in the United States: A Multicenter Survey. ASAIO J 2018;64:689-93. [Crossref] [PubMed]
- Leard LE, Holm AM, Valapour M, et al. Consensus document for the selection of lung transplant candidates: An update from the International Society for Heart and Lung Transplantation. J Heart Lung Transplant 2021;40:1349-79. [Crossref] [PubMed]
- Mulhall A, Cole A, Patel S. Efficacy and Safety of Nintedanib in Idiopathic Pulmonary Fibrosis, Cytisine versus Nicotine for Smoking Cessation, and FACED Score for Non-Cystic Fibrosis Bronchiectasis. Am J Respir Crit Care Med 2015;192:249-51. [Crossref] [PubMed]
- Benazzo A, Schwarz S, Frommlet F, et al. Twenty-year experience with extracorporeal life support as bridge to lung transplantation. J Thorac Cardiovasc Surg 2019;157:2515-2525.e10. [Crossref] [PubMed]
- Lang G, Taghavi S, Aigner C, et al. Primary lung transplantation after bridge with extracorporeal membrane oxygenation: a plea for a shift in our paradigms for indications. Transplantation 2012;93:729-36. [Crossref] [PubMed]
- George TJ, Beaty CA, Kilic A, et al. Outcomes and temporal trends among high-risk patients after lung transplantation in the United States. J Heart Lung Transplant 2012;31:1182-91. [Crossref] [PubMed]
- Schechter MA, Ganapathi AM, Englum BR, et al. Spontaneously Breathing Extracorporeal Membrane Oxygenation Support Provides the Optimal Bridge to Lung Transplantation. Transplantation 2016;100:2699-704. [Crossref] [PubMed]
- Hoetzenecker K, Donahoe L, Yeung JC, et al. Extracorporeal life support as a bridge to lung transplantation-experience of a high-volume transplant center. J Thorac Cardiovasc Surg 2018;155:1316-1328.e1. [Crossref] [PubMed]
- Chambers DC, Perch M, Zuckermann A, et al. The International Thoracic Organ Transplant Registry of the International Society for Heart and Lung Transplantation: Thirty-eighth adult lung transplantation report - 2021; Focus on recipient characteristics. J Heart Lung Transplant 2021;40:1060-72. [Crossref] [PubMed]
- Jing L, Chen W, Zhao L, et al. Acute kidney injury following adult lung transplantation. Chin Med J (Engl) 2021;135:172-80. [Crossref] [PubMed]
- Gan CT, Hoek RAS, van der Bij W, et al. Long-term outcome and bridging success of patients evaluated and bridged to lung transplantation on the ICU. J Heart Lung Transplant 2022;41:589-98. [Crossref] [PubMed]
- Jawitz OK, Raman V, Becerra D, et al. Factors associated with short- versus long-term survival after lung transplant. J Thorac Cardiovasc Surg 2022;163:853-860.e2. [Crossref] [PubMed]
- Huang R, Shao M, Zhang C, et al. Serum Total Bilirubin With Hospital Survival in Adults During Extracorporeal Membrane Oxygenation. Front Med (Lausanne) 2022;9:914557. [Crossref] [PubMed]
- D'Alessandro D, Schroder J, Meyer DM, et al. Impact of controlled hypothermic preservation on outcomes following heart transplantation. J Heart Lung Transplant 2024;43:1153-61. [Crossref] [PubMed]
- Habertheuer A, Richards T, Sertic F, et al. Stratification Risk Analysis in Bridging Patients to Lung Transplant on ECMO: The STABLE Risk Score. Ann Thorac Surg 2020;110:1175-84. [Crossref] [PubMed]
- Diehl A, Burrell AJC, Udy AA, et al. Association Between Arterial Carbon Dioxide Tension and Clinical Outcomes in Venoarterial Extracorporeal Membrane Oxygenation. Crit Care Med 2020;48:977-84. [Crossref] [PubMed]
- Gupta P, McDonald R, Chipman CW, et al. 20-year experience of prolonged extracorporeal membrane oxygenation in critically ill children with cardiac or pulmonary failure. Ann Thorac Surg 2012;93:1584-90. [Crossref] [PubMed]
- Hileman BA, Martucci G, Subramanian H. Metabolic support for patients on extra-corporeal membrane oxygenation. Curr Opin Crit Care 2024;30:305-10. [Crossref] [PubMed]
- Ko RE, Lee JG, Kim SY, et al. Extracorporeal membrane oxygenation as a bridge to lung transplantation: analysis of Korean organ transplantation registry (KOTRY) data. Respir Res 2020;21:20. [Crossref] [PubMed]
- Fernando SM, Fan E, Rochwerg B, et al. Lung-Protective Ventilation and Associated Outcomes and Costs Among Patients Receiving Invasive Mechanical Ventilation in the ED. Chest 2021;159:606-18. [Crossref] [PubMed]
- Moser B, Jaksch P, Taghavi S, et al. Lung transplantation for idiopathic pulmonary arterial hypertension on intraoperative and postoperatively prolonged extracorporeal membrane oxygenation provides optimally controlled reperfusion and excellent outcome. Eur J Cardiothorac Surg 2018;53:178-85. [Crossref] [PubMed]
- McNelly AS, Rawal J, Shrikrishna D, et al. An Exploratory Study of Long-Term Outcome Measures in Critical Illness Survivors: Construct Validity of Physical Activity, Frailty, and Health-Related Quality of Life Measures. Crit Care Med 2016;44:e362-9. [Crossref] [PubMed]
- Wolters AE, Peelen LM, Welling MC, et al. Long-Term Mental Health Problems After Delirium in the ICU. Crit Care Med 2016;44:1808-13. [Crossref] [PubMed]
- Chandler HK, Teppa B, Johnson KA, et al. Determining comorbidities and quality of life among pediatric survivors of extracorporeal life support. J Crit Care 2015;30:1085-9. [Crossref] [PubMed]
- Fleck TP, Dangel G, Bächle F, et al. Long-Term Follow-Up on Health-Related Quality of Life After Mechanical Circulatory Support in Children. Pediatr Crit Care Med 2017;18:176-82. [Crossref] [PubMed]
- Schmidt M, Zogheib E, Rozé H, et al. The PRESERVE mortality risk score and analysis of long-term outcomes after extracorporeal membrane oxygenation for severe acute respiratory distress syndrome. Intensive Care Med 2013;39:1704-13. [Crossref] [PubMed]