Anoikis-related subtype and prognosis analyses based on bioinformatics, and an expression verification of ANGPTL4 based on experiments of lung adenocarcinoma
Highlight box
Key findings
• We discovered novel molecular subtypes and constructed a novel prognostic model of Lung adenocarcinoma (LUAD). We also identified ANGPTL4 as a prognostic indicator of LUAD.
What is known and what is new?
• Anoikis is a form of programmed cell death, and Anoikis resistance promotes tumorigenesis and metastasis. The role of anoikis-related genes (ARGs) in molecular typing and prognosis assessment of LUAD remain unclear.
• ARGs could be used to divide LUADs into two novel molecular subtypes and predict the prognosis. Cluster B and low-risk group had longer overall survival (OS), more immune cells infiltration and higher tumor microenvironment scores. Tumor mutation burden and Tumor Immune Dysfunction and Exclusion analyses showed better immunotherapy response in high-risk group. ANGPTL4 expression was correlated with stage, tumor differentiation, T classification, N classification, and OS.
What is the implication, and what should change now?
• Our study shows the molecular typing and prognosis assessment of LUAD based on ARGs are of great significance.
Introduction
Lung cancer (LC) is one of the most aggressive and lethal malignancies. In 2020, there were approximately 2.2 million new LC cases and 1.8 million LC-related deaths worldwide, accounting for about 11.4% of new cancers and 18% of cancer-related death cases, respectively (1). Lung adenocarcinoma (LUAD) is the most important pathological histological type of LC, accounting for 40% of all LCs (2,3). In recent years, with the rapid development of molecular biology and tumor genetics, numerous new therapeutic methods have emerged. For example, inhibitors of the targets of immunotherapy [e.g., cytotoxic T lymphocyte associated protein 4, programmed cell death protein 1, and programmed death-ligand 1 (PD-L1)] and targeted therapy (e.g., anaplastic lymphoma kinase, ROS proto-oncogene 1 and neurotrophic tropomyosin receptor kinase) have been shown to effectively prolong the survival of LUAD patients (4-6). However, LUAD is a highly heterogeneous cancer with significant individual differences, and some patients do not respond well to existing treatments (7). Thus, conducting further research to identify new molecular subtypes is of great significance to the accurate diagnosis and prognostic evaluation of LUAD.
Anoikis is a form of programmed death that prevents cells from growing, attaching to inappropriate stroma, and colonizing distant organs when they detach from the primary extracellular matrix (8). The overactivation of carcinogenic signaling pathways, mutations of growth factor receptors and enhancements of oxidative stresses promote cancer cells to develop anoikis resistance (8). For instance, the transforming growth factor β1/SH2B adaptor protein 3 axis promotes the anoikis resistance of LC through the Janus kinase 2/signal transducer and activator of transcription 3 signaling pathway (9). The overexpression of epidermal growth factor Cripto-1 has been shown to enhance the anoikis resistance and aggressiveness of breast cancer cells (10). Meanwhile, a LC study has also found that anoikis inhibits the escape of tumor cells from the natural extracellular matrix to other organs, and is associated with tumor progression and treatment failure (11). In vivo and in vitro studies of LC have shown that several natural and synthetic products (such as artonin E, oroxylin A, sulforaphane, and carbenoxolone) can inhibit tumor progression by activating anoikis (11). Given the important roles of anoikis in cancer progression, numerous research studies have sought to explore the relationship between anoikis and tumor molecular typing and prognosis.
A classification and prognostic risk model based on five anoikis-related genes (ARGs) in hepatocellular carcinoma provided novel insights into tumor subtype and survival analyses (12). In an ovarian cancer study, the ARG signature could be used to evaluate the prognosis, immune cell infiltration, mutation, and therapeutic response (13). In pancreatic cancer and pancreatic neuroendocrine tumors, the ARGs also revealed the molecular, prognostic and microenvironmental characteristics of tumors (14). A recent study also revealed the relationship between ARGs and the prediction of prognosis, immune infiltration and treatment outcome of LUAD (15), but numerous bioanalyses and experiments are still needed for further confirmation.
In our research, we first identified 135 differentially expressed and 23 prognosis-related ARGs of LUAD in The Cancer Genome Atlas (TCGA) program. Subsequently, we performed an unsupervised consensus clustering analysis of the 23 prognosis-related ARGs, and divided the 494 LUAD samples into cluster A and cluster B. There were significant differences in the prognosis, clinicopathological characteristics, gene set variation analysis (GSVA) results, and immune cell infiltration between cluster A and cluster B. Next, we constructed a prognostic model using least absolute shrinkage and selection operator (LASSO) and Cox regression analyses based on 10 ARGs. We then validated the model with a Gene Expression Omnibus (GEO) data set and further investigated the differences between the risk groups in terms of the clinicopathologic characteristics, immune cell infiltration, mutation, and drug sensitivity. Finally, we conducted experiments to verify the relationship between ANGPTL4 expression and the clinicopathological characteristics and prognosis of LUAD patients. Our research provides novel insights into molecular subtype classification and the prognosis prediction of LUAD. We present this article in accordance with the TRIPOD reporting checklist (available at https://jtd.amegroups.com/article/view/10.21037/jtd-24-1123/rc).
Methods
Acquire and process LUAD data from TCGA and the GEO
We downloaded the gene expression matrix and clinicopathologic data of 535 LUAD and 59 matched normal lung samples from TCGA (https://portal.gdc.cancer.gov/) (16), and 226 LUAD samples from the GSE31210 GEO (https://www.ncbi.nlm.nih.gov/gds) data set (17). After removing the samples with incomplete clinical data, 494 TCGA and 226 GEO LUAD samples remained for the analysis. R software (version 4.1.3) and Perl (Strawberry version) software were applied to process and analyze the data.
Identification of differentially expressed and prognosis-related ARGs
Initially, we obtained 640 ARGs from the GeneCards (https://www.genecards.org/) (18) and Harmonizome (https://maayanlab.cloud/Harmonizome/) portals (19). Subsequently, we used the “limma” R package to analyze the differentially expressed genes between the LUAD and normal lung tissues based on the following criteria: |log fold change| >1 and false discovery rate <0.05. A univariate Cox analysis was then conducted to identify the prognosis-related ARGs based on the following criterion: P<0.01.
Consensus clustering analysis based on ARGs
“ConsensusClusterPlus” R package (20) was used for the unsupervised clustering of the 494 TCGA-LUAD samples. The following criteria were set: a gradual and steady growth in the cumulative distribution function (CDF) curve, an increase in the intra-group correlation, and a decrease in the inter-group correlation. The clusters were verified by a principal component analysis (PCA) and t-distributed stochastic neighborhood embedding (t-SNE) using the “ggplot2” and “Rtsne” packages respectively. The “pheatmap” package was used to visualize the differences in the gene expression profiles and clinicopathological characteristics of the different clusters. A Kaplan-Meier (K-M) analysis was used to examine differences in OS between the different clusters.
Construction and validation of anoikis-related risk model
First, we used LASSO regression to reduce overfitting and screen out the best value for lambda (λ) (21). Subsequently, we constructed a Cox regression risk model comprising ten prognosis-related ARGs. We calculated the risk score of each patient using the following formula: risk score = esum(each gene expression × corresponding regression coefficient). The LUAD patients were segmented into low- and high-risk groups based on the median score. The K-M curve was used to analyze the differences in OS between the risk groups. Receiver operating characteristic (ROC) curves were used to validate the 1-, 3- and 5-year prognostic efficacy of the model and to compare the accuracy of the risk score with the clinicopathological characteristics. In addition, we analyzed the relationship between the risk score and clinicopathological characteristics, and further validated the model efficacy with the GSE31210external data set.
Functional enrichment analysis
The “GSVA” package was used to examine the differences in the enrichment pathways between the different clusters and risk groups (22). The hallmark gene sets “c2.cp.kegg.v7.2.symbols.gmt” from the MsigDB database (http://software.broadinstitute.org/gsea/msigdb/index.jsp) were employed. In addition, a gene set enrichment analysis (GSEA) annotated by the Kyoto Encyclopedia of Genes and Genomes (KEGG) database was used to further examine the differences in biological function between the two clusters (23).
Immune cell infiltration and immune microenvironment analyses
The CIBERSORT algorithm was used to estimate the infiltration abundance of 28 types of immune cells in the different clusters and risk groups (24). The single-sample GSEA (ssGSEA) algorithm was used to evaluate the proportion of 23 immune cells in the different clusters and risk groups (25). The results were visualized using the “ggplot2” package. The ESTIMATE algorithm was used to assess the ImmuneScore, MatrixScore, and ESTIMATEScore of each patient (26).
Mutation, immunotherapy, and drug sensitivity analyses
The “maftools” package was used to analyze the somatic mutation data of the different risk groups, which were visualized in waterfall plots. We also calculated and compared the tumor mutation burden (TMB) scores of the different risk groups. The Tumor Immune Dysfunction and Exclusion (TIDE) score of each patient was calculated to evaluate the efficacy of immune checkpoint blocking (ICB) therapy (27). The “pRRophetic” package was used to calculate the half-maximal inhibitory concentration of common chemotherapy drugs based on the Genomics of Drug Sensitivity in Cancer database (28).
Immunohistochemical staining analysis of tissue microarrays
We collected 150 LUAD samples and 35 matched normal lung tissue paraffin blocks from the People’s Hospital of Rugao from 2015 to 2017, and fabricated them into tissue microarrays. The clinical data, including age, sex, stage, tumor differentiation, tumor size, lymph node status, and survival time, of all the patients were collected. The microarrays were dewaxed with xylene and gradient ethanol, and then repaired with ethylenediaminetetraacetic acid antigen repair buffer (pH 9.0) under high pressure for 20 minutes. The microarrays were then incubated with 3% hydrogen peroxide and 10% goat serum solutions, respectively, for 20 minutes to block endogenous peroxidase and non-specific antibody binding sites. ANGPTL4 polyclonal antibody (Proteintech, Wuhan, China) was diluted at 1:100 and incubated on the microarrays overnight at 4 ℃. Next, we successively covered the microarrays with horseradish peroxidase-conjugated secondary antibody and 3,3’-diaminobenzidine. The staining intensities of no, weak, medium, and strong staining were allocated 0, 1, 2, and 3 scores, respectively. The staining range percentages of 0–10%, 11–30%, 31–50%, and ≥50% were evaluated as 1, 2, 3, and 4 scores respectively. The immunostaining score was equal to the staining intensity score multiplied by the range percentage score. Immunostaining scores ≥6 or <6 were considered positively or negatively expressed, respectively (29). Two pathologists independently scored the microarrays. The study was conducted in accordance with the Declaration of Helsinki (as revised in 2013). The study was approved by institutional ethics board of The People’s Hospital of Rugao (No. KY202308002) and informed consent was taken from all the patients.
Statistical analysis
The statistical analyses of TCGA and GEO data were conducted using Rsoftware (version 4.1.3). Kruskal and Wilcoxon tests were used to analyze the differences in the clinicopathological characteristics between the risk groups. A Spearman test was used to evaluate the correlation between the ARGs and the abundance of immune cell infiltration. The Chi-squared test was used to confirm the relationship between ANGPTL4 expression and the clinicopathological characteristics using SPSS software (version 20.0). The correlation between ANGPTL4 and OS was examined by a K-M analysis in GraphPad Prism 5. A P value <0.05 was considered statistically significant.
Results
Identification of the differentially expressed and prognosis-related ARGs
First, we obtained 640 ARGs from the GeneCards website and Harmonizome portal (Table S1). We then screened 135 differentially expressed genes between the LUAD and normal lung tissues and presented them in a Venn diagram (Table S2) (Figure 1A). The univariate Cox analysis identified 23 prognosis-related ARGs (Figure 1B). Compared with the normal lung tissues, DAPK2 (P<0.001) and KL (P<0.001) were underexpressed in the LUAD tissues, while CDX2 (P<0.001), KIF18A (P<0.001), PBK (P<0.001), CDC25C (P<0.001), BIRC5 (P<0.001), CDKN3 (P<0.001), MAD2L1 (P<0.001), BUB1 (P<0.001), CDK1 (P<0.001), CENPF (P<0.001), PLK1 (P<0.001), UBE2C (P<0.001), HMGA1 (P<0.001), SLC2A1 (P<0.001), SLCO1B3 (P<0.001), ANGPTL4 (P<0.001), LDHA (P<0.001), CDCP1 (P<0.001), ITGB4 (P<0.001), KRT14 (P<0.001), and SERPINB5 (P<0.001) were overexpressed in the LUAD tissues (Figure 1C). The correlation among the expression of the 23 ARGs was also examined (Figure 1D).
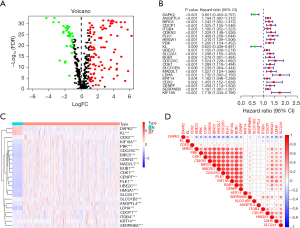
Consensus clustering of LUAD based on the ARGs
We performed an unsupervised consensus clustering analysis to explore the possible subtype clustering of LUAD based on the 23 ARGs. As Figure 2A-2C shows, when k=2, the data set could be well clustered into two groups. The PCA and t-SNE analyses revealed a clear boundary between cluster A and cluster B (Figure 2D,2E). The K-M curve showed that cluster B had better OS than cluster A (Figure 2F). The clinicopathological characteristics analysis revealed significant differences in N classification (P<0.01), T classification (P<0.01), stage (P<0.001), gender (P<0.01), age (P<0.01), and survival status (P<0.01) between cluster A and cluster B (Figure 2G). Further the boxplot analysis showed that the expression of the 23 ARGs differed significantly between clusters A and B, which also indicated the ARG clusters performed well (Figure 2H).
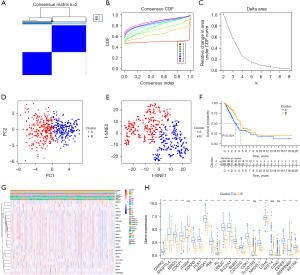
Functional enrichment and immune cell infiltration analyses of the clusters
To explore the differences in biological function between cluster A and cluster B, we conducted a GSVA. Cluster A was significantly more enriched in the p53 signaling pathway, cell cycle, one carbon pool by folate, glyoxylate and dicarboxylate metabolism, and other pathways. While cluster B was significantly more enriched in arachidonic acid metabolism, alpha linolenic acid metabolism, primary bile acid biosynthesis, and the taurine and hypotaurine metabolism pathways (Figure 3A). The GSEA showed that the cell cycle, DNA replication, and oocyte meiosis pathways were more active in cluster A, while the asthma and systemic lupus erythematosus pathways were more active in cluster B (Figure 3B,3C). In view of the significant differences in the metabolism-related pathways between the clusters, and the correlation between metabolism and the tumor immune microenvironment (30), we further analyzed the difference in immune cell infiltration between cluster A and cluster B. The CIBERSORT analysis revealed that activated B cells, activated dendritic cells, central memory CD4 T cells, effector memory CD8 T cells, eosinophils, immature B cells, immature dendritic cells, macrophages, mast cells, myeloid-derived suppressor cells, memory B cells, monocytes, natural killer cells, T follicular helper cells, and type 17 T helper cells were more infiltrated in cluster B. While activated CD4 T cells, natural killer T cells, and type 2 T helper cells were more infiltrated in cluster B (Figure 3D). The results of the ssGSEA also showed that the activated B cells, activated dendritic cells, eosinophils, immature B cells, immature dendritic cells, macrophages, mast cells, natural killer cells, T follicular helper cells, and type 17 T helper cells were more abundant in cluster B than cluster A. While the activated CD4 T cells, CD56 dim natural killer cells, natural killer T cells, and type 2 T helper cells were more abundant in cluster A than cluster B (Figure 3E). The tumor microenvironment (TME) analysis revealed that the StromalScore, ImmuneScore, and ESTIMATEScore were all higher in cluster B than cluster A (Figure 3F).
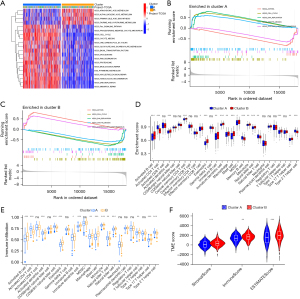
Construction of the anoikis-related prognostic model
To further examine the effect of anoikis on the LUAD prognosis, we performed a LASSO Cox regression analysis of the 23 prognosis-related ARGs from TCGA. We ultimately constructed a risk model comprising ten ARGs (i.e., ANGPTL4, CDCP1, ITGB4, KL, SLC2A1, CDC25C, SLCO1B3, LDHA, CDX2, and KIF18A) (Figure 4A,4B). The median value of the risk score was used to divide the patients into low- and high-risk groups. Compared to the high-risk group, the low-risk group had a better OS rate and a better survival status (P<0.001) (Figure 4C,4D). The expression profiles of the risk genes are shown in Figure 4E. The ROC analysis clarified the values of the areas under the curve (AUCs) of the model at 1, 3, and 5 years were 0.716, 0.715, and 0.624, respectively (Figure 4F).
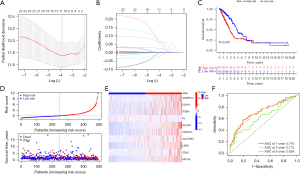
Evaluation and validation of the anoikis-related prognostic model
The multivariate ROC curve showed that the 1-year AUC value of the model was higher than that of age (0.550), gender (0.593), stage (0.711), T classification (0.648), and N classification (0.625) (Figure 5A). The univariate Cox and multivariate Cox analyses revealed that the risk score could be considered an independent survival prognostic indicator of LUAD (hazard ratio: 1.065, P<0.001) (Figure 5B,5C). To better predict patient survival, we constructed a nomogram incorporating the risk score and other clinicopathological characteristics, and visualized the performance of the nomogram with calibration plots at 1, 3, and 5 years (Figure 5D,5E). We used the GSE31210 data set to verify the accuracy of the model. Similarly, we calculated the risk score of each sample separately using the above-mentioned model formula and divided the samples into low- and high-risk groups. Consistent with TCGA analysis, the patients in the high-risk group had higher risk scores, worse OS, and a higher mortality rate than those in the low-risk group (Figure 5F,5G). The expression of the modeling genes in the risk groups were similar to that in TCGA (Figure 5H). The alluvial diagram showed the change in patient distribution between the clusters and risk groups (Figure 5I).
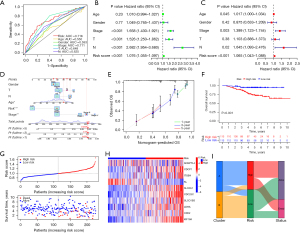
Clinicopathological characteristics and functional enrichment analyses of the risk groups
The PCA and t-SNE analyses showed that the risk groups were well distinguished in the model (Figure 6A,6B). The Kruskal test indicated the risk scores of T2 (P<0.001), T3 (P<0.001), and T4 (P=0.04) were higher than that of T1 (Figure 6C), and the risk scores of N1 (P=0.004) and N2 (P<0.001) were significantly higher than that of N0 (Figure 6D). Similarly, patients in stage II (P=0.002), stage III (P<0.001), and stage IV (P=0.009) all had higher risk scores than those in stage I (Figure 6E). The Wilcox test revealed that the dead patients had higher risk scores than the alive patients (P<0.001) (Figure 6F). In addition, the Chi-squared test revealed that the risk score was correlated with N classification (P<0.01), T classification (P<0.01), stage (P<0.001), gender (P<0.05), age (P<0.05), and survival status (P<0.001) (Figure 6G). Subsequently, to understand the differences in the biological function enrichment of the risk groups, we performed a differential GSVA. The results showed that the low-risk group was more enriched in taurine and hypotaurine metabolism, alpha linolenic acid metabolism, and arachidonic acid metabolism, while the high-risk group was more enriched in pentose phosphate pathway, glycolysis gluconeogenesis, cell cycle, oocyte meiosis, and the p53 signaling pathway (Figure 6H).
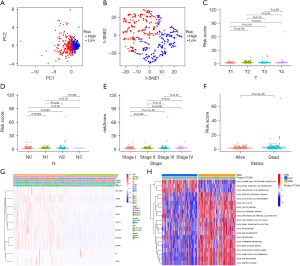
Immune cell infiltration and TME analyses of the risk groups
The CIBERSORT analysis showed that the activated B cells, eosinophils, immature B cells, immature dendritic cells, mast cells, T follicular helper cells, and type 17 T helper cells were more infiltrated in the low-risk group, and activated CD4 T cells, gamma delta T cells, memory B cells, natural killer T cells, regulatory T cells, and type 2 T helper cells were more infiltrated in the high-risk group (Figure 7A). The ssGSEA analysis showed that the activated B cells, eosinophils, immature B cells, immature dendritic cells, mast cells, monocytes, and type 17 T helper cells were more infiltrated in the low-risk group, and activated CD4 T cells, CD56 bright natural killer cells, gamma delta T cells, natural killer T cells, regulatory T cells, and type 2 T helper cells were more infiltrated in the high-risk group (Figure 7B). In addition, the immune checkpoint gene PD-L1 was overexpressed in the high-risk group (Figure 7C). The StromalScore, ImmuneScore, and ESTIMATEScore were all higher in the low-risk group than the high-risk group (P<0.05) (Figure 7D).
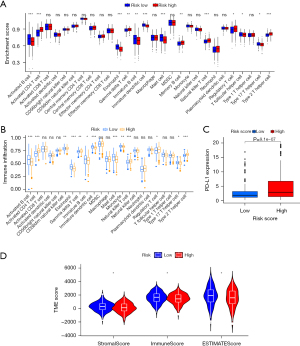
Mutation, immunotherapy, and chemotherapy sensitivity analyses
The rate of somatic mutation is closely related to the TME (31). The “maftools” package was used to analyze the difference in the somatic mutation rates between the two risk groups. The five genes with the highest mutation rates were TP53, TTN, MUC16, CSMD3, and RYR2, and their mutation rates were significantly higher in the high-risk group than the low-risk group (Figure 8A,8B). In addition, we discovered that the TMB score was higher in the high-risk group than the low-risk group (P<0.001) (Figure 8C), and the TMB score was positively associated with OS both in the entire cohort and the risk groups (P=0.01 and P<0.001, respectively) (Figure 8D,8E). The high-risk group had a lower TIDE score than the low-risk group, which indicated that ICB therapy was more effective in the high-risk group than the low-risk group (Figure 8F). The chemotherapy drug sensitivity analysis showed that the high-risk group had lower sensitivity to bleomycin (P<0.001), camptothecin (P<0.001), cisplatin (P<0.001), cytarabine (P<0.001), gemcitabine (P<0.001), methotrexate (P<0.001), paclitaxel (P<0.001), and vinorelbine (P<0.001) than the low-risk group (Figure 8G-8N).
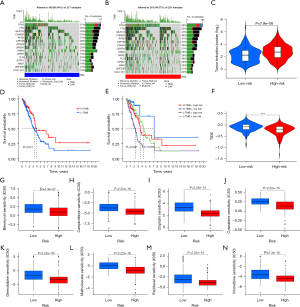
Verification of the expression and prognostic significance of ANGPTL4 in LUAD
ANGPTL4 was a key gene in our prognostic model, and previous studies have shown it plays important roles in regulating apoptosis, amino acid and fatty acid metabolism, and drug sensitivity in LUAD cells (32,33). And the significance of ANGPTL4 expression in LUAD remains unclear. Therefore, we further analyzed the protein expression of ANGPTL4 in LUAD and its correlation with patient prognosis. The immunohistochemical staining of the tissue microarrays showed that ANGPTL4 was highly expressed in the LUAD tissues compared with the normal lung tissues (Figure 9A,9B). The Chi-squared test revealed ANGPTL4 expression was correlated with stage (P=0.004), differentiation degree (P=0.004), T classification (P=0.01), and N classification (P=0.01), but was not correlated with age (P=0.55) and gender (P=0.98) (Table 1). The K-M analysis showed that ANGPTL4 expression was correlated with OS in LUAD patients (P=0.04) (Figure 9C).
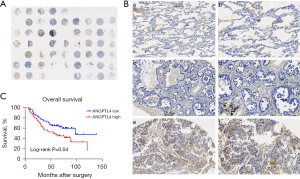
Table 1
Clinicopathological characteristics | All cases | ANGPTL4 expression | P | |
---|---|---|---|---|
Low | High | |||
Age | 0.55 | |||
≤60 years | 37 | 22 | 15 | |
>60 years | 83 | 43 | 40 | |
Gender | 0.98 | |||
Male | 59 | 32 | 27 | |
Female | 61 | 33 | 28 | |
Stage | 0.004 | |||
I + II | 97 | 59 | 38 | |
III + IV | 23 | 6 | 17 | |
Differentiation degree | 0.004 | |||
I | 33 | 25 | 8 | |
II + III | 87 | 40 | 47 | |
T classification | 0.01 | |||
1 | 88 | 54 | 34 | |
2+3+4 | 32 | 11 | 21 | |
N classification | 0.01 | |||
N0 | 78 | 49 | 29 | |
N1+2+3 | 42 | 16 | 26 |
LUAD, lung adenocarcinoma.
Discussion
In recent years, tumor marker research in the field of molecular biology has achieved positive results in LUAD prognosis and therapy (21,34). Despite this, the 5-year OS rate of LUAD patients remains <20% (6). Therefore, novel LUAD molecular subtypes, novel therapies, and early prognostic indicators urgently need to be explored.
Anoikis is involved in the occurrence and progression of many diseases, such as diabetes, infectious diseases, and tumors (11). Hyperglycemia promotes anoikis and impairs angiogenesis by inducing the dicarbonyl metabolism of vascular endothelial cells (35). Aspergillus toxin induces lung epithelial cell anoikis and promotes aspergillus invasion by inhibiting the binding of integrins with extracellular matrix components (36). Tumor cells have strong abilities to escape anoikis, which protects them in the lymphatic and circulatory systems and allows them to metastasize and grow uncontrollably elsewhere (37). A previous study has found the composition of the extracellular matrix, cytoskeletal regulatory factors, cell adhesion associated membrane proteins, and epithelial-mesenchymal transition (EMT) are involved in anoikis resistance (11). For example, highly expressed laminin 5 induces weak resistance and promotes the proliferation and migration of LUAD by activating integrin focal adhesion kinase signal transduction (38). Phosphorylation of the membrane attached molecule Src kinase attenuates anoikis and promotes the growth of LUAD cells in the lymph nodes (39). High levels of βIII-tubulin promote tumorigenesis and anoikis resistance in LUAD via the phosphatase and tensin homolog/protein kinase signaling axis (40). In addition, the dysregulation of EMT-related proteins (E-cadherin and N-cadherin) promotes LUAD progression by inhibiting anoikis (11). In view of their important roles and extensive regulatory mechanisms, the identification of anoikis-related LUAD subtypes and prognostic evaluation is highly valuable.
In this study, we first identified 23 prognosis-related ARGs in TCGA data set, and then divided the LUAD samples into cluster A and cluster B according to the expression of the 23 ARGs. The PCA, t-NSE, and clinicopathological characteristic analyses showed that cluster A and cluster B differed significantly. The prognostic analysis showed that the OS time of cluster B was longer than that of cluster A. The GSVA showed that cluster A was significantly more enriched in the cell cycle and DNA replication signaling pathways, and cluster B was significantly more enriched in the fatty acid-related pathways. Cell cycle pathway activation enables the cells to deviate from the normal cycle, and enables continuous division and uncontrolled proliferation (41). DNA replication is a major driver of sustained proliferation for many cancers, and targeting DNA replication is an effective therapeutic target (42). Linolenic acid inhibits the hypoxia-induced proliferation and invasion of non-small cell LC cells by inhibiting hypoxia-inducible factor 1 alpha (43). Taurine significantly inhibits cell proliferation, metastasis, and colony formation, and induces apoptosis in colorectal cancer cells (44). Thus, we speculated that the better OS of cluster B might be related to its different enrichment pathways.
In addition, the GSEA showed that the asthma and systemic lupus erythematosus pathways were more active in cluster B than cluster B, which suggested that cluster B had stronger immune activity. Thus, we further analyzed the differences in the tumor-infiltrating immune cells and TME between cluster A and cluster B. The results showed that activated B cells, activated dendritic cells, eosinophils, immature B cells, immature dendritic cells, macrophages, mast cells, natural killer cells, T follicular helper cells, and type 17 T helper cells were more infiltrated in cluster B than cluster A. While activated CD4 T cells, CD56 dim natural killer cells, natural killer T cells, and type 2 T helper cells were more infiltrated in cluster A than cluster B. Previous studies have shown that activated B cells play an anti-tumor role through the direct killing of tumor cells or the production of tumor-specific antibodies. The infiltrating abundance of B cells is closely related to the good prognosis of patients (45). Dendritic cells present tumor cell-associated antigens to naive T cells, which helps to produce specific T cell-mediated anti-tumor effects (46). Classically activated M1 macrophages directly mediate cytotoxicity and antibody-dependent cell-mediated cytotoxicity to kill tumor cells (47). Tumor-infiltrating follicular helper T cells located in the tertiary lymphoid structure around the tumor assist T cells to achieve anti-tumor immunity by secreting the cell detoxifying factor (48). Natural killer cells are the main effector cells in cancer innate immunity, which not only directly damage tumor cells, but also produce a large number of cytokines, such as interferon-γ, and participate in the immune effects of other related pathways (49). The difference in immune cell infiltration also explains the difference in prognosis between cluster A and cluster B to some extent.
To better predict of LUAD survival, we constructed a LASSO Cox prognostic model with the ARGs. The ROC curve showed the model had high prediction efficiency. The nomogram and external validation results showed that the model had high reliability and stability. The risk score of the model could be used as an independent prognostic factor of LUAD. The immune cell infiltration and TME analyses revealed greater immune cell infiltration and a higher TME score in the low-risk group than the high-risk group. The higher expression of PD-L1 suggested greater suppression of the immune response in the high-risk group, and was consistent with the lower infiltration of the immune cells. In addition, PD-L1 is also an important immunotherapeutic target, and its inhibitors act as tumor suppressors by modulating immune cell and tumor cell interactions (50). We also found that the high-risk group had a higher somatic mutation rate, TMB score, and lower TIDE score than the low-risk group. A previous study has shown patients with a high TMB are more sensitive to ICB therapy (51). Conversely, the TIDE score was negatively correlated with the patients’ response to ICB therapy (27). Thus, we hypothesized that the high-risk group patients would benefit more from immunotherapy than the low-risk group patients. The drug sensitivity analysis showed that the risk score was negatively correlated with common chemotherapy drugs sensitivity. Our research provides important references for the clinical selection of treatments. Finally, we showed that the expression of ANGPTL4 was significantly correlated with the clinicopathological characteristics of the LUAD patients. LUAD patients with higher ANGPTL4 expression had a worse prognosis than those with lower ANGPTL4 expression.
Conclusions
In conclusion, our research identified two novel molecular subtypes of LUAD and elucidated the differences in the clinicopathological characteristics, prognosis, functional enrichment, and immune infiltration of the two subtypes. We also constructed a new LUAD prognostic model and evaluated the prognostic efficacy of that model. The differences in prognosis, mutation, immunotherapy, and chemotherapy of the risk groups were also investigated. We examined the relationship between ANGPTL4 and the clinicopathological characteristics and prognosis of the LUAD patients. Our research provides important references for the accurate diagnosis, prognosis, and treatment of LUAD patients. However, it did not verify the expression of other genes or the mechanism by which ANGPTL4 promotes the progression of LUAD, which we will address in our future work.
Acknowledgments
Funding: This study was funded by grants from
Footnote
Reporting Checklist: The authors have completed the TRIPOD reporting checklist. Available at https://jtd.amegroups.com/article/view/10.21037/jtd-24-1123/rc
Data Sharing Statement: Available at https://jtd.amegroups.com/article/view/10.21037/jtd-24-1123/dss
Peer Review File: Available at https://jtd.amegroups.com/article/view/10.21037/jtd-24-1123/prf
Conflicts of Interest: All authors have completed the ICMJE uniform disclosure form (available at https://jtd.amegroups.com/article/view/10.21037/jtd-24-1123/coif).The authors have no conflicts of interest to declare.
Ethical Statement: The authors are accountable for all aspects of the work in ensuring that questions related to the accuracy or integrity of any part of the work are appropriately investigated and resolved. The study was conducted in accordance with the Declaration of Helsinki (as revised in 2013). The study was approved by the institutional ethics board of The People’s Hospital of Rugao (No. KY202308002) and informed consent was taken from all the patients.
Open Access Statement: This is an Open Access article distributed in accordance with the Creative Commons Attribution-NonCommercial-NoDerivs 4.0 International License (CC BY-NC-ND 4.0), which permits the non-commercial replication and distribution of the article with the strict proviso that no changes or edits are made and the original work is properly cited (including links to both the formal publication through the relevant DOI and the license). See: https://creativecommons.org/licenses/by-nc-nd/4.0/.
References
- Sung H, Ferlay J, Siegel RL, et al. Global Cancer Statistics 2020: GLOBOCAN Estimates of Incidence and Mortality Worldwide for 36 Cancers in 185 Countries. CA Cancer J Clin 2021;71:209-49. [Crossref] [PubMed]
- Goldstraw P, Ball D, Jett JR, et al. Non-small-cell lung cancer. Lancet 2011;378:1727-40. [Crossref] [PubMed]
- Comprehensive molecular profiling of lung adenocarcinoma. Nature 2014;511:543-50. [Crossref] [PubMed]
- Santarpia M, Aguilar A, Chaib I, et al. Non-Small-Cell Lung Cancer Signaling Pathways, Metabolism, and PD-1/PD-L1 Antibodies. Cancers (Basel) 2020;12:1475. [Crossref] [PubMed]
- Marinelli D, Siringo M, Metro G, et al. Non-small-cell lung cancer: how to manage ALK-, ROS1- and NTRK-rearranged disease. Drugs Context 2022;11:2022-3-1. [Crossref] [PubMed]
- Zappa C, Mousa SA. Non-small cell lung cancer: current treatment and future advances. Transl Lung Cancer Res 2016;5:288-300. [Crossref] [PubMed]
- Xu Y, Tao T, Li S, et al. Prognostic model and immunotherapy prediction based on molecular chaperone-related lncRNAs in lung adenocarcinoma. Front Genet 2022;13:975905. [Crossref] [PubMed]
- Paoli P, Giannoni E, Chiarugi P. Anoikis molecular pathways and its role in cancer progression. Biochim Biophys Acta 2013;1833:3481-98. [Crossref] [PubMed]
- Wang LN, Zhang ZT, Wang L, et al. TGF-β1/SH2B3 axis regulates anoikis resistance and EMT of lung cancer cells by modulating JAK2/STAT3 and SHP2/Grb2 signaling pathways. Cell Death Dis 2022;13:472. [Crossref] [PubMed]
- Normanno N, De Luca A, Bianco C, et al. Cripto-1 overexpression leads to enhanced invasiveness and resistance to anoikis in human MCF-7 breast cancer cells. J Cell Physiol 2004;198:31-9. [Crossref] [PubMed]
- Wang J, Luo Z, Lin L, et al. Anoikis-Associated Lung Cancer Metastasis: Mechanisms and Therapies. Cancers (Basel) 2022;14:4791. [Crossref] [PubMed]
- Chen Y, Huang W, Ouyang J, et al. Identification of Anoikis-Related Subgroups and Prognosis Model in Liver Hepatocellular Carcinoma. Int J Mol Sci 2023;24:2862. [Crossref] [PubMed]
- Duan Y, Xu X. A signature based on anoikis-related genes for the evaluation of prognosis, immunoinfiltration, mutation, and therapeutic response in ovarian cancer. Front Endocrinol (Lausanne) 2023;14:1193622. [Crossref] [PubMed]
- Li N, Jia X, Wang Z, et al. Characterization of anoikis-based molecular heterogeneity in pancreatic cancer and pancreatic neuroendocrine tumor and its association with tumor immune microenvironment and metabolic remodeling. Front Endocrinol (Lausanne) 2023;14:1153909. [Crossref] [PubMed]
- Wang Y, Xie C, Su Y. A novel anoikis-related gene signature to predict the prognosis, immune infiltration, and therapeutic outcome of lung adenocarcinoma. J Thorac Dis 2023;15:1335-52. [Crossref] [PubMed]
- Wang Z, Jensen MA, Zenklusen JC. A Practical Guide to The Cancer Genome Atlas (TCGA). Methods Mol Biol 2016;1418:111-41. [Crossref] [PubMed]
- Clough E, Barrett T. The Gene Expression Omnibus Database. Methods Mol Biol 2016;1418:93-110. [Crossref] [PubMed]
- Rebhan M, Chalifa-Caspi V, Prilusky J, et al. GeneCards: integrating information about genes, proteins and diseases. Trends Genet 1997;13:163. [Crossref] [PubMed]
- Rouillard AD, Gundersen GW, Fernandez NF, et al. The harmonizome: a collection of processed datasets gathered to serve and mine knowledge about genes and proteins. Database (Oxford) 2016;2016:baw100. [Crossref] [PubMed]
- Wilkerson MD, Hayes DN. ConsensusClusterPlus: a class discovery tool with confidence assessments and item tracking. Bioinformatics 2010;26:1572-3. [Crossref] [PubMed]
- Tibshirani R. The lasso method for variable selection in the Cox model. Stat Med 1997;16:385-95. [Crossref] [PubMed]
- Hänzelmann S, Castelo R, Guinney J. GSVA: gene set variation analysis for microarray and RNA-seq data. BMC Bioinformatics 2013;14:7. [Crossref] [PubMed]
- Subramanian A, Tamayo P, Mootha VK, et al. Gene set enrichment analysis: a knowledge-based approach for interpreting genome-wide expression profiles. Proc Natl Acad Sci U S A 2005;102:15545-50. [Crossref] [PubMed]
- Newman AM, Liu CL, Green MR, et al. Robust enumeration of cell subsets from tissue expression profiles. Nat Methods 2015;12:453-7. [Crossref] [PubMed]
- Liang L, Yu J, Li J, et al. Integration of scRNA-Seq and Bulk RNA-Seq to Analyse the Heterogeneity of Ovarian Cancer Immune Cells and Establish a Molecular Risk Model. Front Oncol 2021;11:711020. [Crossref] [PubMed]
- Yoshihara K, Shahmoradgoli M, Martínez E, et al. Inferring tumour purity and stromal and immune cell admixture from expression data. Nat Commun 2013;4:2612. [Crossref] [PubMed]
- Jiang P, Gu S, Pan D, et al. Signatures of T cell dysfunction and exclusion predict cancer immunotherapy response. Nat Med 2018;24:1550-8. [Crossref] [PubMed]
- Yang W, Soares J, Greninger P, et al. Genomics of Drug Sensitivity in Cancer (GDSC): a resource for therapeutic biomarker discovery in cancer cells. Nucleic Acids Res 2013;41:D955-61. [Crossref] [PubMed]
- Zhao J, Liu J, Wu N, et al. ANGPTL4 overexpression is associated with progression and poor prognosis in breast cancer. Oncol Lett 2020;20:2499-505. [Crossref] [PubMed]
- Xia L, Oyang L, Lin J, et al. The cancer metabolic reprogramming and immune response. Mol Cancer 2021;20:28. [Crossref] [PubMed]
- Wang JJ, Lei KF, Han F. Tumor microenvironment: recent advances in various cancer treatments. Eur Rev Med Pharmacol Sci 2018;22:3855-64. [Crossref] [PubMed]
- Fang Y, Li X, Cheng H, et al. ANGPTL4 Regulates Lung Adenocarcinoma Pyroptosis and Apoptosis via NLRP3\ASC\Caspase 8 Signaling Pathway to Promote Resistance to Gefitinib. J Oncol 2022;2022:3623570. [Crossref] [PubMed]
- Xiao S, Nai-Dong W, Jin-Xiang Y, et al. ANGPTL4 regulate glutamine metabolism and fatty acid oxidation in nonsmall cell lung cancer cells. J Cell Mol Med 2022;26:1876-85. [Crossref] [PubMed]
- Drosten M, Barbacid M. Targeting KRAS mutant lung cancer: light at the end of the tunnel. Mol Oncol 2022;16:1057-71. [Crossref] [PubMed]
- Dobler D, Ahmed N, Song L, et al. Increased dicarbonyl metabolism in endothelial cells in hyperglycemia induces anoikis and impairs angiogenesis by RGD and GFOGER motif modification. Diabetes 2006;55:1961-9. [Crossref] [PubMed]
- Haun F, Neumann S, Peintner L, et al. Identification of a novel anoikis signalling pathway using the fungal virulence factor gliotoxin. Nat Commun 2018;9:3524. [Crossref] [PubMed]
- Simpson CD, Anyiwe K, Schimmer AD. Anoikis resistance and tumor metastasis. Cancer Lett 2008;272:177-85. [Crossref] [PubMed]
- Kodama K, Ishii G, Miyamoto S, et al. Laminin 5 expression protects against anoikis at aerogenous spread and lepidic growth of human lung adenocarcinoma. Int J Cancer 2005;116:876-84. [Crossref] [PubMed]
- Sakuma Y, Takeuchi T, Nakamura Y, et al. Lung adenocarcinoma cells floating in lymphatic vessels resist anoikis by expressing phosphorylated Src. J Pathol 2010;220:574-85. [Crossref] [PubMed]
- McCarroll JA, Gan PP, Erlich RB, et al. TUBB3/βIII-tubulin acts through the PTEN/AKT signaling axis to promote tumorigenesis and anoikis resistance in non-small cell lung cancer. Cancer Res 2015;75:415-25. [Crossref] [PubMed]
- Matthews HK, Bertoli C, de Bruin RAM. Cell cycle control in cancer. Nat Rev Mol Cell Biol 2022;23:74-88. [Crossref] [PubMed]
- Taylor EM, Lindsay HD. DNA replication stress and cancer: cause or cure? Future Oncol 2016;12:221-37. [Crossref] [PubMed]
- Wang Y, Shi J, Gong L. Gamma linolenic acid suppresses hypoxia-induced proliferation and invasion of non-small cell lung cancer cells by inhibition of HIF1α. Genes Genomics 2020;42:927-35. [Crossref] [PubMed]
- Hou X, Hu J, Zhao X, et al. Taurine Attenuates the Hypotaurine-Induced Progression of CRC via ERK/RSK Signaling. Front Cell Dev Biol 2021;9:631163. [Crossref] [PubMed]
- Qin Y, Lu F, Lyu K, et al. Emerging concepts regarding pro- and anti tumor properties of B cells in tumor immunity. Front Immunol 2022;13:881427. [Crossref] [PubMed]
- Del Prete A, Salvi V, Soriani A, et al. Dendritic cell subsets in cancer immunity and tumor antigen sensing. Cell Mol Immunol 2023;20:432-47. [Crossref] [PubMed]
- Pan Y, Yu Y, Wang X, et al. Tumor-Associated Macrophages in Tumor Immunity. Front Immunol 2020;11:583084. [Crossref] [PubMed]
- Gu-Trantien C, Loi S, Garaud S, et al. CD4+ follicular helper T cell infiltration predicts breast cancer survival. J Clin Invest 2013;123:2873-92. [Crossref] [PubMed]
- Luetke-Eversloh M, Cicek BB, Siracusa F, et al. NK cells gain higher IFN-γ competence during terminal differentiation. Eur J Immunol 2014;44:2074-84. [Crossref] [PubMed]
- Alsaab HO, Sau S, Alzhrani R, et al. PD-1 and PD-L1 Checkpoint Signaling Inhibition for Cancer Immunotherapy: Mechanism, Combinations, and Clinical Outcome. Front Pharmacol 2017;8:561. [Crossref] [PubMed]
- Palmeri M, Mehnert J, Silk AW, et al. Real-world application of tumor mutational burden-high (TMB-high) and microsatellite instability (MSI) confirms their utility as immunotherapy biomarkers. ESMO Open 2022;7:100336. [Crossref] [PubMed]