Correlation between handgrip strength and air trapping in patients with stable chronic obstructive pulmonary disease
Highlight box
Key findings
• Handgrip strength (HGS) shows a significant negative correlation with air trapping in stable chronic obstructive pulmonary disease (COPD). Additionally, HGS correlates with other lung functions, including forced vital capacity, forced expiratory volume in 1 second, and diffusing capacity of the lung for carbon monoxide.
What is known and what is new?
• COPD patients often experience difficulty breathing, which contributes to the development of air trapping in the lungs. The effects of air trapping in the lungs are associated with clinical outcomes in COPD patients, including fatigue, respiratory muscle function, limb muscle function, and risk of exacerbations. The correlation between HGS and air trapping has not been investigated.
• This study demonstrated that air trapping is common in COPD patients, and HGS is significantly correlated with air trapping.
What is the implication, and what should change now?
• HGS may serve as an alternative tool for assessing air trapping in patients with clinically stable COPD.
Introduction
Chronic obstructive pulmonary disease (COPD) is a common disease with the third-highest mortality rate globally (1). In Thailand, the prevalence of COPD is 7.1% (2), making it a significant health concern. It is progressive and irreversible, with a key feature being the inability to fully exhale, leading to trapped air in the lungs (3). This results from decreased elasticity of the lung tissues and obstruction of the airways during exhalation. Additionally, COPD patients often experience difficulty breathing, contributing to the development of air trapping in the lungs (4).
Studies of the effects of air trapping in the lungs on clinical outcomes in patients with COPD have found various impacts, including fatigue (5), respiratory muscle function (6), limb muscle function (7), and risk of exacerbations (8).
It is well-established that individuals with COPD commonly experience issues related to the depletion of muscular mass (9). Notably, there is a significant relationship between handgrip strength (HGS) and reduction in mortality rates among COPD patients (10). Consequently, it is reasonable to infer that the assessment of HGS is a valuable tool in monitoring COPD patients.
Air trapping can be assessed through techniques such as closed-circuit helium dilution and body plethysmography (3). Specifically, an abnormal value of the ratio of residual volume (RV) to total lung capacity (TLC) greater than 40% is indicative of air trapping (11). The implementation of these diagnostic methods necessitates specialized equipment and proficient individuals for accurate interpretation. Some alternative tools might be beneficial for assessing air trapping. The correlation between HGS and air trapping has not been investigated. Therefore, this study aimed to investigate the relationship between HGS and air trapping in stable COPD patients. We present this article in accordance with the STROBE reporting checklist (available at https://jtd.amegroups.com/article/view/10.21037/jtd-24-631/rc).
Methods
Study design and participants
A cross-sectional study was conducted at Thammasat University Hospital in Thailand from May 2022 to December 2023. COPD patients aged over 40 years, diagnosed by spirometry [postbronchodilator forced expiratory volume in 1 second (FEV1)/forced vital capacity (FVC) <0.7], and with a smoking history of 10 or more pack-years were included. Exclusion criteria included COPD exacerbation in the past 3 months, inability to perform HGS assessment, inability to measure lung volume, undergoing tracheostomy tube placement, and using mechanical ventilation.
Demographic data, respiratory symptoms, and functional capacity assessed by modified Medical Research Council (mMRC) (12), COPD Assessment Test (CAT) (13), 6-minute walking distance (6MWD), and spirometry data were collected.
The Global Initiative for Chronic Obstructive Lung Disease (GOLD) criteria for COPD severity were based on FEV1 value: grade 1 indicates mild (≥80% of predicted value), grade 2 indicates moderate (50–79% of predicted value), and grades 3 and 4 indicate severe and very severe (<50% and <30% of predicted values), respectively (3). GOLD symptoms and risk were classified into 3 groups: A, B, and E, depending on dyspnea score and history of exacerbation (3).
This study was conducted in accordance with the Declaration of Helsinki (as revised in 2013). The study protocol was approved by the Human Research Ethics Committee of Thammasat University (Medicine) (IRB No. MTU-EC-IM-0-209/65, COA No. 083/2023). All participants provided written informed consent. This study was registered with ClinicalTrials.gov with number NCT06220851.
Procedures
The measurements of lung volume and diffusing capacity of the lung for carbon monoxide (DLCO) were conducted using a body plethysmograph (Vyntus™ BODY by CareFusion Germany 234 GmbH, Germany). These assessments adhered to the standardized protocols established by both the American Thoracic Society and the European Respiratory Society (14-16). Air trapping was defined as RV/TLC >40% (17).
HGS was measured by a Jamar® hand dynamometer (Asimow Engineering Co., CA, USA) and was reported in kilograms. Patients performed the test at rest in sitting position with the dominant hand unsupported, with the wrist in a neutral position, with the elbow at a 90° flexion, and the shoulder adducted. All patients were instructed to squeeze the hand dynamometer as hard as possible for 3–5 seconds. The test was performed with three attempts, with one-minute breaks between attempts. The maximal value of three efforts was recorded for the final analysis.
Outcomes
The primary outcome was the correlation between HGS and air trapping in clinically stable COPD patients. The secondary outcome was the best cutoff value of HGS to predict occurrence of air trapping.
Statistical analysis
Due to the lack of prior studies investigating the relationship between HGS and air trapping in patients with COPD, we hypothesized that there is a moderate-level correlation between HGS and air trapping, with a correlation coefficient of 0.4. The sample size was calculated for a two-sample means test using 90% power and 5% type I error. Thus, the calculated sample size would be 72.
Baseline characteristics were shown in number (%) and mean ± standard deviation (SD). Pearson correlation was used to determine the correlation between HGS and spirometry or lung volume data. The relationship between two variables was reported as correlation coefficient (r). To determine the set of variables associated with air trapping, we used the linear regression model with RV/TLC set as the dependent variable. All independent variables—age, body mass index, CAT scores, and DLCO level—were entered into the regression model. Regression coefficients (95% confidence interval) were reported.
The receiver operator characteristic (ROC) curve was used to determine the best HGS cutoff value to predict air trapping. A two-sided P value <0.05 was considered statistically significant. Statistical analyses were performed using SPSS version 26.0 software (IBM Corp., Armonk, NY, USA).
Results
Participants
A total of 90 patients were screened, and 18 were excluded (Figure 1). Seventy-two patients (90.3% male) were included, with an average age of 72.4±9.7 years. The body mass index was 23.5±4.3 kg/m2, and the smoking history was 23.2±14.8 pack-years. Common comorbidities included hypertension (36.1%) and diabetes (22.2%). Most patients were classified as COPD grade 2 and group E. The mean postbronchodilator FEV1 was 72.1%±21.2%, and RV/TLC was 44.2%±10.3%. The prevalence of air trapping was 55.6%. Mean HGS was 30.5±8.1 kg (Table 1). Air trapping in COPD group A, B, and E was 1.4%, 27.8%, and 26.4%, respectively (P=0.39).
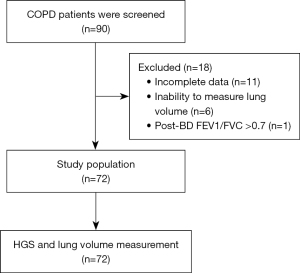
Table 1
Characteristics | Data (n=72) |
---|---|
Age, years | 72.4±9.7 |
Male | 65 (90.3) |
BMI, kg/m2 | 23.5±4.3 |
Smoking, pack-years | 23.2±14.8 |
Dominant right hand | 64 (88.9) |
Comorbidity | |
Hypertension | 26 (36.1) |
Diabetes | 16 (22.2) |
Clinical history | |
COPD grade | |
1 | 24 (33.3) |
2 | 34 (47.2) |
3 | 13 (18.1) |
4 | 1 (1.4) |
COPD group | |
A | 4 (5.6) |
B | 33 (45.8) |
E | 35 (48.6) |
AECOPD in the past year | 22 (30.6) |
AECOPD with hospitalization in the past year | 10 (13.9) |
Oxygen saturation level, % | 96.6±1.6 |
Medication | |
SABA/SAMA | 36 (50.0) |
ICS/LABA/LAMA | 27 (37.5) |
PDE4i | 25 (34.7) |
LABA/LAMA | 21 (29.2) |
ICS/LABA | 18 (25.0) |
LAMA | 15 (20.8) |
SABA | 9 (12.5) |
Macrolide | 4 (5.6) |
Spirometry data | |
Post-BD FVC, L | 2.78±0.82 |
Post-BD FVC, % predicted | 94.2±20.9 |
FVC change after BD test, % | 4.0±7.1 |
Post-BD FEV1, L | 1.62±0.59 |
Post-BD FEV1, %predicted | 72.1±21.2 |
FEV1 change after BD test, % | 7.6±7.6 |
Post-BD FEV1/FVC, % | 58.7±13.2 |
Lung volume and DLCO data | |
TLC, L | 5.19±1.05 |
TLC, % predicted | 90.4±14.6 |
RV, L | 2.44±0.66 |
RV, % predicted | 100.6±25.5 |
RV/TLC, % | 44.2±10.3 |
DLCO, mL/mmHg/min | 13.12±4.44 |
DLCO, % | 63.7±22.8 |
KCO, mL/mmHg/min/L | 3.43±2.36 |
KCO, % | 86.6±26.6 |
Air trapping in all groups | 40 (55.6) |
Air trapping in COPD group A* | 1 (1.4) |
Air trapping in COPD group B* | 20 (27.8) |
Air trapping in COPD group E* | 19 (26.4) |
Functional performance | |
mMRC, points | 2.1±0.8 |
CAT scores | 14.9±6.9 |
6MWD, m | 358.0±121.9 |
Hand grip strength, kg | 30.5±8.1 |
Data shown as mean ± SD or n (%). *, P=0.391 for the comparison between the 3 groups. AECOPD, acute exacerbation of chronic obstructive pulmonary disease; BD, bronchodilator; BMI, body mass index; CAT, COPD Assessment Test; COPD, chronic obstructive pulmonary disease; DLCO, diffusing capacity of the lung for carbon monoxide; FEV1, forced expiratory volume in 1 second; FVC, forced vital capacity; ICS, inhaled corticosteroid; KCO, carbon monoxide transfer coefficient; LABA, long-acting beta2 agonist; LAMA, long-acting muscarinic receptor; mMRC, modified Medical Research Council; 6MWD, 6-minute walking distance; PDE4i, phosphodiesterase-4 inhibitor; RV, residual volume; SABA, short-acting beta2 agonist; SAMA, short-acting muscarinic receptor; TLC, total lung capacity.
Association between HGS and air trapping
There was a negative correlation between HGS and RV/TLC (r=−0.399, P=0.001) (Table 2 and Figure 2). The equation for predicting air trapping is as follows: predicted RV/TLC (%) =59.63 − 0.51 × HGS. Additionally, HGS had a negative correlation with RV (r=−0.272, P=0.02). Moreover, positive correlation was observed between HGS and DLCO (r=0.393, P=0.001), FVC (r=0.320, P=0.007), and FEV1 (r=0.260, P=0.02) (Table 2). HGS was also positively correlated with 6WMD (r=0.378, P=0.03).
Table 2
Variable | Correlation coefficient | P value |
---|---|---|
Spirometry data | ||
Post-BD FVC, L | 0.320 | 0.007 |
Post-BD FVC, % predicted | 0.070 | 0.55 |
Post-BD FEV1, L | 0.260 | 0.02 |
Post-BD FEV1, % predicted | 0.032 | 0.78 |
Post-BD FEV1/FVC, % | −0.026 | 0.82 |
FEV1/FVC, % predicted | 0.121 | 0.31 |
FVC change after BD, % | 0.192 | 0.10 |
FEV1 change after BD, % | −0.050 | 0.67 |
Lung volume and DLCO data | ||
TLC, L | 0.115 | 0.33 |
TLC, % predicted | −0.055 | 0.64 |
RV, L | −0.272 | 0.02 |
RV, % predicted | −0.155 | 0.19 |
RV/TLC, % | −0.399 | 0.001 |
DLCO, mL/mmHg/min | 0.393 | 0.001 |
DLCO, % predicted | 0.218 | 0.06 |
KCO, mL/mmHg/min/L | 0.169 | 0.15 |
KCO, % | 0.126 | 0.29 |
6-minute walking distance, m | 0.378 | 0.03 |
BD, bronchodilator; DLCO, diffusing capacity of the lung for carbon monoxide; FEV1, forced expiratory volume in 1 second; FVC, forced vital capacity; KCO, carbon monoxide transfer coefficient; RV, residual volume; TLC, total lung capacity.
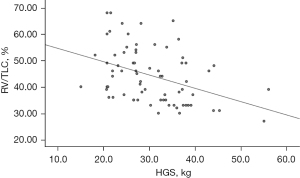
HGS cutoff value for predicting air trapping
The optimal cutoff value for HGS to predict air trapping was 28.3 kg, with a sensitivity of 71.9% and specificity of 65.0%. The area under the ROC curve for identifying air trapping was 0.681 (95% CI: 0.554 to 0.808, P=0.009) (Table 3 and Figure 3).
Table 3
Parameters | Values |
---|---|
Cutoff value of HGS (kg) | 28.3 |
AUC | 0.681 |
95% CI | 0.554–0.808 |
Sensitivity (%) | 71.9 |
Specificity (%) | 65.0 |
PPV (%) | 72.0 |
NPV (%) | 64.9 |
P value | 0.009 |
AUC, area under the curve; CI, confidence interval; HGS, hand grip strength; NPV, negative predictive value; PPV, positive predictive value.
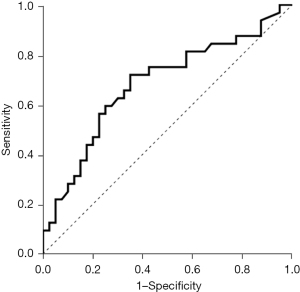
The linear regression analysis revealed that HGS was the only variable associated with air trapping in the model (regression coefficients of −0.334, P=0.04) (Table 4).
Table 4
Variables | Regression coefficients | 95% CI of coefficients | P value |
---|---|---|---|
Intercept | 46.579 | 16.634 to 76.523 | 0.003 |
Handgrip strength, kg | −0.334 | −0.657 to −0.010 | 0.04 |
Age, years | 0.124 | −0.138 to 0.387 | 0.34 |
Body mass index, kg/m2 | 0.136 | −0.451 to 0.723 | 0.64 |
CAT scores, points | 0.100 | −0.317 to 0.517 | 0.31 |
DLCO, mL/mmHg/min | −0.447 | −1.153 to 0.259 | 0.21 |
CAT, COPD Assessment Test; COPD, chronic obstructive pulmonary disease; DLCO, diffusing capacity of the lung for carbon monoxide; CI, confidence interval.
Discussion
To our knowledge, this is the first study to explore the correlation between HGS and air trapping, as well as to identify an HGS cutoff value for predicting air trapping in patients with clinically stable COPD. Based on the results of our study, a significant negative correlation between HGS and air trapping suggests that HGS may be a valuable test in the assessment of air trapping in COPD patients.
The prevalence of air trapping in our study (55.6%) aligns with previous findings reported by Kim et al. (51.1%) (8) and Lim et al. (60.9%) (18). HGS is correlated with various factors in COPD patients, including age, health-related quality of life, CAT score, pulmonary functions, hospitalization rate, and mortality rate (19-22). A decline in HGS consequently reflects aspects such as CAT scores and hospitalization rates, which is consistent with findings from a systematic literature review by Martinez et al. (10). This review revealed that COPD patients with increased HGS were associated with decreased hospitalization rates and lower CAT scores. Furthermore, a meta-analysis conducted by Mgbemena et al. demonstrated a significant correlation between HGS and FEV1 as well as between HGS and FVC (21). Similarly, a study by Jeong et al. found significant associations between HGS and FEV1 (r=0.69) and FVC (r=0.76) (19), consistent with our results. Additionally, Suriyakul et al. demonstrated in their study that HGS correlated with peak inspiratory flow rate, which measures the inspiratory effort needed for drug inhalation (23).
HGS serves as a primary diagnostic tool for sarcopenia and is useful in assessing various chronic conditions (24). For instance, a study by Strandkvist et al. demonstrated that COPD patients with GOLD grade 3–4 exhibited lower HGS compared to those without COPD, and HGS was associated with FEV1 % (25). Similarly, lower HGS was also correlated with reduced FVC and FEV1 in research conducted by Shah et al. (26). Moreover, Martinez et al. illustrated that HGS was associated with body composition, airway thickness, body mass index, emphysema, and exacerbation rate (10). Beyond chronic conditions, HGS can also be used to assess critically ill patients (27,28). For instance, a study conducted in Thailand by Saiphoklang et al. revealed that HGS correlated with the rapid shallow breathing index (RSBI), a weaning parameter, in mechanically ventilated patients (29).
Our study revealed a significant negative correlation between HGS and the RV/TLC ratio, supporting the hypothesis that COPD patients may undergo muscle mass loss. This phenomenon could stem from various factors, including chronic oxygen deficiency, acidosis, insulin resistance, and the administration of corticosteroids in treatment (9). Moreover, increased muscle utilization within the respiratory system may trigger changes in the autonomic nervous system, responsible for regulating blood volume. This alteration can lead to heightened blood flow to respiratory muscles and diminished blood flow to extremity muscles, potentially explaining the observed skeletal muscle weakness in COPD patients (7). Furthermore, we observed a negative correlation between HGS and RV, although its association was weaker compared to the RV/TLC ratio. This finding aligns with research by de Weger et al., who reported that the RV/TLC ratio exhibited a stronger correlation with patient-related outcomes than other parameters in COPD patients (30). However, the mean RV and RV/TLC% in our group are borderline high but not severe. This could be one of the reasons for the lower sensitivity and specificity of the HGS in our study.
In addition, our results indicated a significantly positive correlation between HGS and DLCO levels. In COPD patients, abnormalities in the airways, lung parenchyma, and pulmonary vasculature often lead to decreased DLCO (31). A meta-analysis conducted by Ni et al. revealed that reductions in DLCO were more common in emphysema-dominant COPD compared to those with non-emphysema COPD (32). The identification of the relationship between HGS and DLCO suggests that HGS may reflect the overall lung gas exchange capabilities.
Our study unveiled that the optimal HGS cutoff values for predicting air trapping demonstrated an acceptably high area under the ROC curve (0.681) with sensitivity and specificity. Additionally, HGS was the only parameter associated with air trapping, as demonstrated by linear regression analysis in our study.
This study has certain limitations. The main limitation is its generalizability. The mean age was 72 years, and the subjects were predominantly male (90%). The results of this study may not be generalized to female COPD. The higher age in the study group could have an exaggerated effect on the findings since sarcopenia itself is more common in this group. There are other limitations in the study. Firstly, it was conducted in a single research center in Thailand, which may limit the generalizability of the results to other ethnicities or countries. Secondly, the study employed a small sample size, potentially limiting the representativeness of the findings for the broader population. Thirdly, the participants consisted of clinically stable COPD patients without acute exacerbation, raising questions about the applicability of the HGS cutoff value to more unstable COPD patients. Fourthly, this was a cross-sectional study; the observed HGS and lung function may vary over time depending on the patient’s condition. Finally, the study did not collect data on participants’ medication compliance. Since medication compliance is known to play a crucial role in symptom control, it could act as a confounding factor in the study.
Conclusions
Air trapping in stable COPD is a common condition. HGS shows a significant negative correlation with air trapping in stable COPD. Additionally, HGS correlates with other lung functions such as FVC, FEV1, and DLCO. Hence, HGS may function as an assessment tool for air trapping in stable COPD patients.
Acknowledgments
The authors thank Michael Jan Everts, Faculty of Medicine, Thammasat University, for proofreading this manuscript.
Funding: This work was supported by
Footnote
Reporting Checklist: The authors have completed the STROBE reporting checklist. Available at https://jtd.amegroups.com/article/view/10.21037/jtd-24-631/rc
Data Sharing Statement: Available at https://jtd.amegroups.com/article/view/10.21037/jtd-24-631/dss
Peer Review File: Available at https://jtd.amegroups.com/article/view/10.21037/jtd-24-631/prf
Conflicts of Interest: Both authors have completed the ICMJE uniform disclosure form (available at https://jtd.amegroups.com/article/view/10.21037/jtd-24-631/coif). The authors have no conflicts of interest to declare.
Ethical Statement: The authors are accountable for all aspects of the work in ensuring that questions related to the accuracy or integrity of any part of the work are appropriately investigated and resolved. This study was conducted in accordance with the Declaration of Helsinki (as revised in 2013). The study protocol was approved by the Human Research Ethics Committee of Thammasat University (Medicine) (IRB No. MTU-EC-IM-0-209/65, COA No. 083/2023). All participants provided written informed consent.
Open Access Statement: This is an Open Access article distributed in accordance with the Creative Commons Attribution-NonCommercial-NoDerivs 4.0 International License (CC BY-NC-ND 4.0), which permits the non-commercial replication and distribution of the article with the strict proviso that no changes or edits are made and the original work is properly cited (including links to both the formal publication through the relevant DOI and the license). See: https://creativecommons.org/licenses/by-nc-nd/4.0/.
References
- World Health Organization. Chronic obstructive pulmonary disease (COPD) 2021. Available online: https://www.who.int/news-room/fact-sheets/detail/chronic-obstructive-pulmonary-disease-(copd). Accessed April 1 2024.
- Maranetra KN, Chuaychoo B, Dejsomritrutai W, et al. The prevalence and incidence of COPD among urban older persons of Bangkok Metropolis. J Med Assoc Thai 2002;85:1147-55. [PubMed]
- Global Initiative for Chronic Obstructive Lung Disease. Global strategy for the diagnosis, management and prevention of chronic obstructive pulmonary disease 2024 report. Available online: https://goldcopd.org/2024-gold-report/. Accessed April 1 2024.
- Gagnon P, Guenette JA, Langer D, et al. Pathogenesis of hyperinflation in chronic obstructive pulmonary disease. Int J Chron Obstruct Pulmon Dis 2014;9:187-201. [PubMed]
- O'Donnell DE, Webb KA. Exertional breathlessness in patients with chronic airflow limitation. The role of lung hyperinflation. Am Rev Respir Dis 1993;148:1351-7. [Crossref] [PubMed]
- Mador MJ, Kufel TJ, Pineda LA, et al. Diaphragmatic fatigue and high-intensity exercise in patients with chronic obstructive pulmonary disease. Am J Respir Crit Care Med 2000;161:118-23. [Crossref] [PubMed]
- Sheel AW, Derchak PA, Morgan BJ, et al. Fatiguing inspiratory muscle work causes reflex reduction in resting leg blood flow in humans. J Physiol 2001;537:277-89. [Crossref] [PubMed]
- Kim Y, Kim SH, Rhee CK, et al. Air Trapping and the Risk of COPD Exacerbation: Analysis From Prospective KOCOSS Cohort. Front Med (Lausanne) 2022;9:835069. [Crossref] [PubMed]
- Jagoe RT, Engelen MP. Muscle wasting and changes in muscle protein metabolism in chronic obstructive pulmonary disease. Eur Respir J Suppl 2003;46:52s-63s. [Crossref] [PubMed]
- Martinez CH, Diaz AA, Meldrum CA, et al. Handgrip Strength in Chronic Obstructive Pulmonary Disease. Associations with Acute Exacerbations and Body Composition. Ann Am Thorac Soc 2017;14:1638-45. [Crossref] [PubMed]
- Diez Herranz A. RV/TLC% ratio: alternative criteria of normality. Eur Respir J 1995;8:1812-3. [Crossref] [PubMed]
- Mahler DA, Wells CK. Evaluation of clinical methods for rating dyspnea. Chest 1988;93:580-6. [Crossref] [PubMed]
- Jones PW, Harding G, Berry P, et al. Development and first validation of the COPD Assessment Test. Eur Respir J 2009;34:648-54. [Crossref] [PubMed]
- Bhakta NR, McGowan A, Ramsey KA, et al. European Respiratory Society/American Thoracic Society technical statement: standardisation of the measurement of lung volumes, 2023 update. Eur Respir J 2023;62:2201519. [Crossref] [PubMed]
- Wanger J, Clausen JL, Coates A, et al. Standardisation of the measurement of lung volumes. Eur Respir J 2005;26:511-22. [Crossref] [PubMed]
- Graham BL, Brusasco V, Burgos F, et al. 2017 ERS/ATS standards for single-breath carbon monoxide uptake in the lung. Eur Respir J 2017;49:1600016. [Crossref] [PubMed]
- Albuquerque AL, Nery LE, Villaça DS, et al. Inspiratory fraction and exercise impairment in COPD patients GOLD stages II-III. Eur Respir J 2006;28:939-44. [Crossref] [PubMed]
- Lim JU, Lee JS, Lee JH, et al. Clinical impact of long-term change in air trapping on pulmonary function and computed tomography parameters in chronic obstructive pulmonary disease. Korean J Intern Med 2021;36:636-46. [Crossref] [PubMed]
- Jeong M, Kang HK, Song P, et al. Hand grip strength in patients with chronic obstructive pulmonary disease. Int J Chron Obstruct Pulmon Dis 2017;12:2385-90. [Crossref] [PubMed]
- Jung HI, Gu KM, Park SY, et al. Correlation of handgrip strength with quality of life-adjusted pulmonary function in adults. PLoS One 2024;19:e0300295. [Crossref] [PubMed]
- Mgbemena N, Jones A, Leicht AS. Relationship between handgrip strength and lung function in adults: a systematic review. Physiother Theory Pract 2022;38:1908-27. [Crossref] [PubMed]
- Holden M, Fyfe M, Poulin C, et al. Handgrip Strength in People With Chronic Obstructive Pulmonary Disease: A Systematic Review and Meta-Analysis. Phys Ther 2021;101:pzab057. [Crossref] [PubMed]
- Suriyakul A, Saiphoklang N, Barjaktarevic I, et al. Correlation between Hand Grip Strength and Peak Inspiratory Flow Rate in Patients with Stable Chronic Obstructive Pulmonary Disease. Diagnostics (Basel) 2022;12:3050. [Crossref] [PubMed]
- Cruz-Jentoft AJ, Bahat G, Bauer J, et al. Sarcopenia: revised European consensus on definition and diagnosis. Age Ageing 2019;48:16-31. [Crossref] [PubMed]
- Strandkvist VJ, Backman H, Röding J, et al. Hand grip strength is associated with forced expiratory volume in 1 second among subjects with COPD: report from a population-based cohort study. Int J Chron Obstruct Pulmon Dis 2016;11:2527-34. [Crossref] [PubMed]
- Shah S, Nahar P, Vaidya S, et al. Upper limb muscle strength & endurance in chronic obstructive pulmonary disease. Indian J Med Res 2013;138:492-6. [PubMed]
- Saiphoklang N, Tepwimonpetkun C. Interest of hand grip strength to predict outcome in mechanically ventilated patients. Heart Lung 2020;49:637-40. [Crossref] [PubMed]
- Saiphoklang N, Mokkongphai N. Handgrip strength cutoff value predicting successful extubation in mechanically ventilated patients. PLoS One 2021;16:e0258971. [Crossref] [PubMed]
- Saiphoklang N, Keawon T. Correlation between Handgrip Strength and Rapid Shallow Breathing Index for Assessment of Weaning from Mechanical Ventilation. Crit Care Res Pract 2021;2021:4637528. [Crossref] [PubMed]
- de Weger WW, Klooster K, Ten Hacken NH, et al. Determining Static Hyperinflation in Patients with Severe Emphysema: Relation Between Lung Function Parameters and Patient-Related Outcomes. Lung 2020;198:629-36. [Crossref] [PubMed]
- Hogg JC, Timens W. The pathology of chronic obstructive pulmonary disease. Annu Rev Pathol 2009;4:435-59. [Crossref] [PubMed]
- Ni Y, Yu Y, Dai R, et al. Diffusing capacity in chronic obstructive pulmonary disease assessment: A meta-analysis. Chron Respir Dis 2021;18:14799731211056340. [Crossref] [PubMed]