Peripheral immunological characteristics of spontaneous pneumothorax: a Mendelian randomization study
Highlight box
Key findings
• Through two-sample Mendelian randomization analysis, seven peripheral immune cells were found to be significantly associated with the risk of spontaneous pneumothorax.
What is known and what is new?
• Evidence supporting a causal link between peripheral immune cells and spontaneous pneumothorax is inconclusive.
• This study confirmed the causal relationship between peripheral immune cells and spontaneous pneumothorax, revealing a positive correlation between eosinophils and disease incidence. Additionally, B cells, T cells, and natural killer T cell subsets were also related to disease occurrence, whereas natural killer cell subsets showed no correlation.
What is the implication, and what should change now?
• These findings are valuable for radiation-free early warning of spontaneous pneumothorax and the exploration of related immune mechanisms.
Introduction
Spontaneous pneumothorax (SP) is one of the most common pleural diseases affecting adolescents and adults (1,2). Recent epidemiological studies in European populations have reported that the incidence of SP is notably higher in males, with rates ranging from 20.2–21.4/100,000 individuals aged 15 and above, in contrast to females, ranging from 7.2–7.9/100,000 individuals (3). SP is characterized by the occurrence of pneumothorax without traumatic or iatrogenic causes, leading to air accumulation in the pleural cavity. Factors increasing the risk of primary spontaneous pneumothorax (PSP) include a lean, tall physique, exposure to air pollution, smoking, and marijuana use (4-7). Additionally, secondary spontaneous pneumothorax (SSP) results from underlying pulmonary conditions like pulmonary tuberculosis, malignancies, and chronic obstructive pulmonary disease (COPD) (3). Computed tomography (CT) scan is the key method for screening and diagnosis of bulla and pneumothorax (8,9). In a multinational long-term follow-up of 948,174 individuals by Bosch de Basea Gomez et al. (10), the risk of hematologic malignancies in adolescents and adults significantly increased with higher CT doses and frequencies. Given these risks, understanding the pathogenesis of SP and identifying effective biomarkers are crucial. This knowledge can facilitate convenient auxiliary screening methods for high-risk populations, potentially reducing SP incidence in its early stages.
The immune system plays a pivotal role in maintaining lung health and responding to injury. The pulmonary immune system constitutes a highly intricate network of various immune cells (11-14). These immune cells collaborate to shield the lungs from external threats such as bacteria, viruses, and allergens, with tissue-resident memory T cells (MTC) in the respiratory tract (15) being recognized as key players in localized immune responses against various viral and bacterial infections. Furthermore, the development of pulmonary diseases like asthma and COPD has been closely linked to the abnormal accumulation and activation of eosinophils (16-18). Eosinophils mediate lung inflammation, bronchoconstriction, and mucus production through immune responses. Additionally, derived cathepsin L exacerbates the degradation of the extracellular matrix, leading to emphysema in lung tissue (16,19), with COPD-associated emphysema and bullae rupture being the most common etiology in all hospitalized SP cases (3). However, for SP patients who exhibit lung parenchymal changes resembling emphysema without apparent clinical pulmonary diseases, the mechanisms underlying SP development in relation to immune cells and peripheral blood cells remain elusive.
Mendelian randomization (MR) offers an advantageous method for investigating causal relationships between exposures and outcomes. It employs genetic characteristics of the human genome as tools for causal inference in epidemiology (20,21). Unlike observational studies, MR leverages the random allocation of genes from parents to offspring during meiosis, akin to the randomization of treatments in randomized controlled trials (RCTs). This design effectively mitigates numerous potential biases, including environmental factors like air pollution (22) and weather conditions (4), which are often unaccounted for in conventional research studies (23,24).
In this study, we implemented a two-sample, bidirectional MR framework, utilizing summary statistics from large-scale genome-wide association studies (GWAS) of peripheral immune blood cells, including B cells, T cells, natural killer T (NKT) cells, and natural killer (NK) cell subtypes (25,26). Our aim is to investigate the characteristic phenotypes of these immune cells in individuals susceptible to SP, providing new insights into the immunological mechanisms of SP. Additionally, we hope to offer an auxiliary screening method for the early warning of high-risk SP patients. We present this article in accordance with the STROBE-MR reporting checklist (available at https://jtd.amegroups.com/article/view/10.21037/jtd-24-798/rc) (27,28).
Methods
Study design
A two-sample bidirectional MR investigation was carried out to systematically establish causal effects between peripheral immune cell counts, including B cells, T cells, NKT cells, NK cells, and susceptibility to SP. The MR study adhered to three core assumptions (29): (I) the selected genetic variants should be closely associated with the exposure; (II) they need to be independent of other confounding factors; (III) the instrumental variables (IVs) must exclusively affect the outcome through the exposure they represent, not via alternative pathways (Figure 1). The study was conducted in accordance with the Declaration of Helsinki (as revised in 2013).
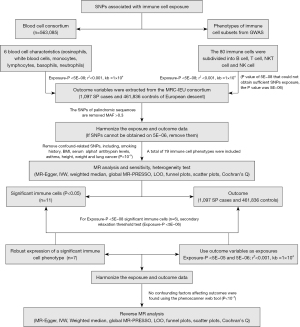
Data sources for exposure and outcome
For exposure instruments, the Blood Cell Consortium dataset in the IEU-OPEN-GWAS repository provided the most recent and extensive summary data on peripheral immune blood cell counts, encompassing data from 563,085 individuals of European ancestry (26). This dataset included genetic instruments related to eosinophil, white blood cells, monocytes, lymphocytes, basophils, and neutrophils. Furthermore, we subdivided lymphocytes into B cells, T cells, NKT cells, and NK cell subtypes for a more in-depth analysis of their causal associations with SP (25). For outcome data on SP, we obtained data from the large-scale case-control dataset of the Medical Research Council Integrative Epidemiology Unit (MRC-IEU) Consortium, which involved 1,097 cases and 461,836 controls of European descent. From the following website: https://gwas.mrcieu.ac.uk/, all relevant data mentioned above can be retrieved using the provided GWAS ID numbers.
IV selection
For each immune trait, a significance threshold of P<5E−08 was set as the primary criterion for selecting IVs to meet the assumption of relevance [linkage disequilibrium (LD) r2<0.001, kb =10,000]. The threshold for the maximum minor allele frequency (MAF) for aligned palindromic single nucleotide polymorphisms (SNPs) was established at 0.3. In instances where no SNPs associated with exposure were identified in the outcome dataset, we opted for proxy SNPs that exhibited a high correlation (r2>0.8) with the variants of interest. To ensure consistency in effect estimates across different allele frequencies, we used a conservative reference allele frequency to harmonize ambiguous SNPs with opposite effect directions. Subsequently, IVs were extracted from the outcome of SP, and SNPs that might violate the independence assumption were removed. Phenotypes related to SP risk factors such as smoking history, body mass index (BMI), serum alpha1 antitrypsin levels, asthma, height (30), weight and lung cancer were considered (4,9,31-33). Therefore, the network tool (http://www.phenoscanner.medschl.cam.ac.uk/) was used to test the correlation of SNPs within IVs with these risk factors at the threshold of P<1E−05 (34,35). SNPs related to risk factors were excluded from IVs to ensure a purified causal effect estimation between immune phenotypes and SP. However, in cases where the threshold for IV selection was relaxed to P<5E−06 (LD r2<0.001, kb =10,000) for the following three conditions: (I) immune traits for which no related SNPs were obtainable below the P<5E−08 threshold; (II) even if a sufficient number of SNPs was selected under the 5E−08 threshold, these SNPs were excluded as confounding SNPs in the process of controlling for confounding factors; (III) it was not possible to obtain enough SNPs due to LD under the P<5E−08 threshold.
Furthermore, due to the strict threshold of P<5E−08 for IV selection, some positive IVs for immune cell subtype analysis exhibited only a single SNP, leading to complete overlap of SNPs between individual IVs (25,36). This may result in spurious causal effects. To avoid this bias, we performed a secondary analysis with the threshold extended to P<5E−06 for immune phenotypes that exhibited statistical significance under the 5E−08 threshold.
Reverse MR analyses
This step involves reverse MR analyses based on positive immune traits identified in forward MR analyses to further examine whether there are reciprocal causal effects between SP and immune cell subtypes. We initially restricted the SP IV selection threshold to P<5E−06 (LD r2<0.001, kb =10,000) and subsequently relaxed it to P<5E−05 (LD r2<0.001, kb =10,000) for the secondary analysis to assess the stability of results.
Statistical analyses
Considering that different methods are fundamentally based on the level of pleiotropy, we employed multiple MR approaches, including inverse variance-weighted (IVW) (37), weighted median (38), MR-Egger methods (39) and Wald ratios for single SNP IVs. If the results in the IVW method were statistically significant (P<0.05) and no (40) anomalies were detected in subsequent sensitivity analyses performed, and the odds ratio (OR) directions reported by the other methods were consistent, the causal effect of exposure on SP was affirmed reliable and stable.
A range of sensitivity analyses were employed to detect the presence of pleiotropy or heterogeneity in MR causal analyses to ensure the reliability of the results. Cochran’s Q (40) quantified heterogeneity in individual causal effect estimates, with P>0.05 indicating no heterogeneity, thus justifying the use of a fixed-effects IVW analysis. Potential horizontal pleiotropy and directional pleiotropy were assessed based on the intercept of MR-Egger and the global test of MR-Pleiotropy RESidual Sum and Outlier (PRESSO), with P values >0.05 indicating the absence of pleiotropy (36). Leave-one-out (LOO) analysis (41), funnel plots, and scatter plots were used to detect outliers and examine the correlation trends between exposure and outcome.
All analyses were performed using R (version 4.2.1) and implemented through the Two Sample MR (version 0.5.7) (42) and MR-PRESSO (version 1.0) packages. Forest plots were generated using the Forest plot (Package Forestploter version 1.1.1). The heat map was mapped by using ChiPlot (https://www.chiplot.online/). There were no missing data for the data used.
Results
Eosinophilic absolute count (AC) had a robust positive causal relationship to SP susceptibility
The causal relationship between peripheral blood cell counts and susceptibility on SP has been explored, utilizing IVs derived from peripheral blood cells (Figure 2). The proportion of variance explained (PVE) was as follows: basophil cell count 0.83% (n=58), white blood cell count 0.11% (n=156), monocyte cell count 5.44% (n=131), lymphocyte cell count 3.36% (n=191), eosinophil cell count 3.03% (n=119), and neutrophil cell count 2.62% (n=137) (tables available at https://cdn.amegroups.cn/static/public/10.21037jtd-24-798-1.xlsx).
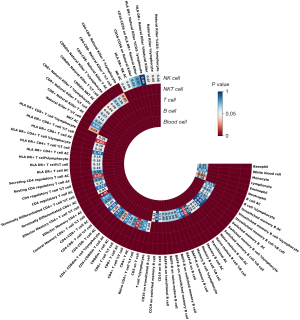
Our results revealed a robust and statistically significant causal relationship between increased susceptibility to SP and elevated eosinophil cell counts, following the rigorous elimination of confounding SNPs. IVW analysis showed an OR of 1.0008 [95% confidence interval (CI): 1.0001–1.0014, P=0.02]. Conversely, no significant associations were found between susceptibility to SP and counts of basophil cells (P=0.40), white blood cells (P=0.51), monocytes (P=0.71), lymphocytes (P=0.27), or neutrophils (P=0.28).
Cochran’s Q test (P>0.05) indicated an absence of noteworthy heterogeneity among the peripheral blood cell IVs. MR-Egger intercept and global MR-PRESSO test effectively excluded horizontal pleiotropy. The directional consistency between MR-Egger, Weighted median, and IVW results was confirmed by LOO analysis and scatter plot (Figure S1).
An increase in memory B cell (MBC) %B cell ratio increased the risk of susceptibility to SP
After rigorous screening, a total of 23 B lymphocyte subsets were included in the follow-up analysis (Figure 2).
The IVW method indicated that an increase in MBC %B cell ratio was associated with an increased SP risk, with a PVE of 4.5% and an F-statistic of 167.05 (OR =1.0008, 95% CI: 1.0002–1.0015, P=0.01). The other two methods also observed a similar positive correlation: MR-Egger (OR =1.0024, 95% CI: 0.9898–1.0150, P=0.74) and weighted median (OR =1.0009, 95% CI: 1.0000–1.0018, P=0.04). The results for the remaining B cell subgroups can be found in the online table (available at https://cdn.amegroups.cn/static/public/10.21037jtd-24-798-2.xlsx).
The global test of MR-PRESSO and MR-Egger intercept (P>0.05) also ruled out the possibility of horizontal pleiotropy. LOO analysis did not reveal any individual SNP that strongly violated the overall impact of MBC %B cell ratio on SP (Figure S2A). Subsequent scatterplots further supported the consistency of the three methods in the direction of the association with SP, confirming its reliability (Figure S2B-S2D).
Positive and negative associations between three T cell subsets and the risk of SP
In the subsequent analysis of 32 T-cell subgroups (Figure 2), a significant positive correlation between increases in CD4+ T cell AC (OR =1.0014, 95% CI: 1.0003–1.0025, P=0.009), effector memory CD4+ T cell %T cell proportion (OR =1.0028, 95% CI: 1.0010–1.0046, P=0.003), and HLA-DR+CD8+ T cell %T cell proportion (OR =1.0019, 95% CI: 1.0004–1.0035, P=0.01) with susceptibility to SP were observed. Conversely, three T-cell subgroups with immunophenotypes, CD8dim T cell AC (OR =0.9983, 95% CI: 0.9967–0.9999, P=0.03), CD4−CD8− T cell AC (OR =0.9986, 95% CI: 0.9972–0.9999, P=0.04), and resting CD4 regulatory T cell AC (OR =0.9988, 95% CI: 0.9979–0.9998, P=0.01), showed protective effects on SP.
Since each of the aforementioned IVs had fewer than two associated SNPs, the P values were represented using the Wald ratio. Further details regarding PVE, F values, and results for the remaining T-cell subgroups can be found in the online table (available at https://cdn.amegroups.cn/static/public/10.21037jtd-24-798-3.xlsx).
NKT AC, CD8dim NKT %T cell, and CD4−CD8− NKT %lymphocyte exhibited protective effects on SP
In the analysis of 11 representative NKT cell subgroups (Figure 2), the causal effects of SP were assessed. The Wald ratio method revealed intriguing insights, with three NKT cell subgroups demonstrating a distinct protective effect against SP. Specifically, NKT AC displayed a significant negative correlation with SP susceptibility (OR =0.9984, 95% CI: 0.9970–0.9999, P=0.03). Moreover, the CD8dim NKT %T cell subgroup exhibited a similar protective role (OR =0.9982, 95% CI: 0.9965–0.9999, P=0.04). CD4−CD8− NKT %Lymphocyte ratio showed a negative correlation with SP risk (OR =0.9983, 95% CI: 0.9968–0.9999, P=0.03) (table available at https://cdn.amegroups.cn/static/public/10.21037jtd-24-798-4.xlsx).
NK cells did not exhibit a causal relationship with SP
In MR analysis of eight distinct NK cell subgroups regarding SP, for the various phenotypes, the P values were as follows: NK AC (P=0.08), HLA-DR+ NK AC (P=0.09), CD16-CD56 on NK (P=0.43), CD16-CD56 on HLA-DR+ NK (P=0.57), HLA-DR+ NK %CD3− lymphocyte (P>0.99), HLA-DR+ NK %NK (P=0.07), NK %lymphocyte (P=0.08), and NK %CD3− lymphocyte (P=0.08) (table available at https://cdn.amegroups.cn/static/public/10.21037jtd-24-798-5.xlsx).
The summary information for all the aforementioned immune cells, along with the confounding SNP information, can be found in the online tables (available at https://cdn.amegroups.cn/static/public/10.21037jtd-24-798-6.xlsx).
The robustness of positive results was evaluated by secondary validation
The relaxation threshold of peripheral immune cells with statistical difference was verified twice to ensure the reliability of the results.
The IVW method revealed that, following the relaxation of the threshold, CD4−CD8− NKT %lymphocyte proportion, CD4−CD8− T cell AC, NKT AC, and resting CD4 regulatory T cell AC lost their statistical significance (Figure 3, Figure S3). However, CD4+ T cell AC (OR =1.0014, 95% CI: 1.0003–1.0025, P=0.009), CD8dim T cell AC (OR =0.9983, 95% CI: 0.9967–0.9999, P=0.03), and HLA-DR+ CD8+ T cell %T cell (OR =1.0011, 95% CI: 1.0002–1.0021, P=0.01) retained their statistical significance, with consistent directions as observed at the 5E−08 threshold. Additionally, all F>10, and no horizontal pleiotropy or heterogeneity was detected among the reported positive findings. No evident outlier SNPs were identified through scatterplots and funnel plots (tables available at https://cdn.amegroups.cn/static/public/10.21037jtd-24-798-7.xlsx).
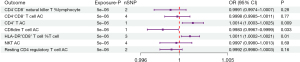
Reverse causal association of SP on immune cells for positive results was not detected
To explore the possibility of a reverse causal relationship between SP and immune cell phenotypes, SP was designated as the exposure variable and identified seven stable immune cell phenotypes as outcomes for further analysis (Figures S4,S5). No heterogeneity was detected according to Cochran’s Q test, and the absence of horizontal pleiotropy was corroborated by the intercept of MR-Egger and the global test of MR-PRESSO, F<10. No evidence of confounding exposure SNPs was found, and the IVW method failed to yield statistically significant results (tables available at https://cdn.amegroups.cn/static/public/10.21037jtd-24-798-8.xlsx).
Discussion
The study identified eosinophils AC, MBC percentage among B cells, CD4+ T cell AC, effector memory CD4+ T cells percentage among T cells, and HLA-DR+CD8+ T cells percentage among T cells as risk factors that increased susceptibility to SP. On the other hand, CD8dim T cell AC and CD8dim NKT cell percentage among T cells demonstrated protective effects against SP. No significant findings were observed in the NK cell subgroups and reverse MR analysis.
Existing etiological investigations into SP primarily emphasize the interplay between lung-related irregularities and environmental factors like smoking (4,22,43). However, the involvement of the immune system in its pathogenesis remains enigmatic. This lack of understanding stems from the confounding biases associated with SP risk factors that traditional research methodologies fail to effectively control, including environmental factors (22) and personal emotional factors in daily life (44). Conversely, the MR approach utilizes genetic variations as exposure indicators, thereby rectifying the shortcomings of conventional study designs (24,37).
Leveraging extensive datasets from public gene repositories (25,26), we scrutinized potential causal relationships between peripheral immune blood cells, lymphocyte subsets, and SP. Notably, seven immune cell phenotypes displayed a significant positive causal effect in SP development without any reverse causal effect.
Eosinophils represent a common element involved in mediating lower respiratory tract inflammation and lung parenchymal injury. Experimental research highlights the role of human blood eosinophils in inducing airway inflammation through extensive degranulation (17). Collagenase contained within eosinophilic granules specifically targets the two primary connective tissue components of human lung parenchyma—type I and III collagen. Furthermore, the cytotoxic impact of toxic oxygen free radicals contributes to lung epithelial cell injury and interstitial lung disease alveolitis (18). Previous observations by De Smedt et al. (45) indicated a substantial increase in eosinophilic AC in pleural lavage fluid and blood in PSP patients, correlating with severe pleural inflammatory response. Rigorous MR Analysis in this study corroborates the association between eosinophilic AC and SP, signifying that an increase in peripheral blood eosinophilic AC heightens susceptibility to SP. Unlike prior studies, this investigation explicitly proposes a positive causal relationship, augmenting our understanding of eosinophilic effects in SP and serving as a reference for subsequent SP-related research into its mechanisms.
Transcriptomic analysis of the lungs revealed a correlation between B cell recruitment, immunoglobulin (Ig) transcription genes, and the severity of emphysema (46,47). B-cell memory is a hallmark of adaptive immunity, where naive B cells expressing IgM and IgD irreversibly switch within the germinal center, transforming into B-cell receptor (BCR) primarily expressing IgG. These IgG-expressing BCR undergo antigen-driven selections, ultimately emerging from the germinal center as co-expressed MBC or other forms (46,48). MBC serves as a pivotal reservoir for plasma cell production in secondary immune responses, collaborating with MTC to sustain durable antibody titers (49). Recent evidence from Habener et al. (50) implies that an increased frequency of IgA-type MBC significantly relates to asthma development and small airway dysfunction, leading to impaired lung function. MBC deficiency has also been associated with adverse respiratory outcomes and chronic lung diseases in common variant immunodeficiency disease (CVID) (51). In tandem with this study’s results, the increased MBC %B cell ratio may be attributed to augmented airway pressure and lung compliance due to small airway dysfunction, potentially heightening the risk of SP.
The coordinated efforts between lung T cells and B cells stand as an indispensable component of lung immunity, as indicated by pertinent animal experiments demonstrating the protective role of T lymphocytes in pulmonary immunity (52). The main immunological framework of CD4 T cells encompasses two subpopulations: helper T-1 (Th1) cells and helper T-2 (Th2) cells, secreting interferon-gamma (IFN-γ) and interleukin-4 (IL-4) respectively (53,54). During T cell activation, non-apoptotic T cells develop into long-lived MTC, fractionated into central memory, efficient memory (TEM), and stem cell MTC based on phenotype (55). In addition, experimental findings in mice have illuminated how IFN-γ, induced by alveoli, potentially leads to the destruction of alveolar septa causing emphysema (56). TEM exhibits active cytokine production, such as IFN-γ and IL-4 (57), explaining why an increase in CD4+ T cell %T cell ratio, effector memory CD4+ T cell %T cell, significantly elevates SP risk, thereby maintaining a positive causal effect. Moreover, the augmented CD8+HLA-DR+ T lymphocytes in CVID patients with impaired MBC differentiation (51,58) elucidate the collaborative impact of T and B cells in the process of SP-related immune damage, aligning with our findings. In addition, the study by Barceló et al. revealing that circulating CD8+ T cell expression of cytokines such as IFN-γ was unaffected supports MR results independent of SP occurrence (59). However, the physiological role of CD8dim T cell AC remains unknown, and its observed protective effect in SP might result from inhibiting direct or indirect immune-inflammatory responses (60-62).
The results showed that NKT cells and their subpopulations had a protective relationship with SP development. This protective link may stem from the varying stimulative capabilities of NKT cells and their subsets (CD4, CD8, double-negative NKT) on lymphocyte subsets. A study by Urbanowicz et al. (13) observed a marked decrease in peripheral NKT cells in COPD patients compared to non-smoking healthy individuals and smokers. They further found that circulating NKT cells in COPD patients solely expressed granzyme B and exhibited significantly reduced cytotoxic effects compared to the other groups. In the MR analysis of NKT cell subsets against SP, statistically significant subset cells exhibited protective causal effects. However, the NK cell subpopulation showed no significance in this study.
Despite this study’s innovative identification of the causal role of immune mechanisms in SP occurrence through genetic methods, certain limitations remain. Although differences between various SP subtypes were noted, this study could not further analyze the peripheral immune cell characteristics of SP subgroups due to the lack of GWAS data for PSP and SSP. The limited sample size in publicly available datasets may have impacted the reverse causal analysis of SP, with some F<10, potentially leading to correlation bias. While this study extensively analyzed the majority of representative lymphocyte subgroups, there is scope for a more comprehensive analysis of additional immune cell populations in future research.
Conclusions
The study highlights the significance of increased peripheral eosinophils and certain lymphocyte subpopulations in significantly raising the susceptibility to SP. Additionally, the increase in specific NKT cell subpopulations indicates a protective and causal relationship with SP. This could potentially serve as an auxiliary method for screening susceptible populations for SP in the future. Importantly, this study provides a theoretical foundation for subsequent research into the immune mechanisms of SP.
Acknowledgments
We express our sincere gratitude to the MRC-IEU Consortium, Blood Cell Consortium, and the authors and participants involved in the relevant immune cell phenotyping studies for publicly sharing their data in the summary statistics of GWAS.
Funding: This work was supported by
Footnote
Reporting Checklist: The authors have completed the STROBE-MR reporting checklist. Available at https://jtd.amegroups.com/article/view/10.21037/jtd-24-798/rc
Peer Review File: Available at https://jtd.amegroups.com/article/view/10.21037/jtd-24-798/prf
Conflicts of Interest: All authors have completed the ICMJE uniform disclosure form (available at https://jtd.amegroups.com/article/view/10.21037/jtd-24-798/coif). The authors have no conflicts of interest to declare.
Ethical Statement: The authors are accountable for all aspects of the work in ensuring that questions related to the accuracy or integrity of any part of the work are appropriately investigated and resolved. The study was conducted in accordance with the Declaration of Helsinki (as revised in 2013).
Open Access Statement: This is an Open Access article distributed in accordance with the Creative Commons Attribution-NonCommercial-NoDerivs 4.0 International License (CC BY-NC-ND 4.0), which permits the non-commercial replication and distribution of the article with the strict proviso that no changes or edits are made and the original work is properly cited (including links to both the formal publication through the relevant DOI and the license). See: https://creativecommons.org/licenses/by-nc-nd/4.0/.
References
- Mummadi SR, Stoller JK, Lopez R, et al. Epidemiology of Adult Pleural Disease in the United States. Chest 2021;160:1534-51. [Crossref] [PubMed]
- Bobbio A, Dechartres A, Bouam S, et al. Epidemiology of spontaneous pneumothorax: gender-related differences. Thorax 2015;70:653-8. [Crossref] [PubMed]
- Hallifax RJ, Goldacre R, Landray MJ, et al. Trends in the Incidence and Recurrence of Inpatient-Treated Spontaneous Pneumothorax, 1968-2016. JAMA 2018;320:1471-80. [Crossref] [PubMed]
- Kim D, Eom SY, Shin CS, et al. The clinical effect of smoking and environmental factors in spontaneous pneumothorax: a case-crossover study in an Inland province. Ther Adv Respir Dis 2020;14:1753466620977408. [Crossref] [PubMed]
- Marx T, Bernard N, Kepka S, et al. Pneumothorax and the environment: A systematic review of the impact of air pollution and meteorology, and a meta-analysis on meteorology factors. Environ Pollut 2021;283:117089. [Crossref] [PubMed]
- Gracie K, Hancox RJ. Cannabis use disorder and the lungs. Addiction 2021;116:182-90. [Crossref] [PubMed]
- Vakil E, Taghizadeh N, Tremblay A. The Global Burden of Pleural Diseases. Semin Respir Crit Care Med 2023;44:417-25. [Crossref] [PubMed]
- Huan NC, Sidhu C, Thomas R. Pneumothorax: Classification and Etiology. Clin Chest Med 2021;42:711-27. [Crossref] [PubMed]
- Paskaradevan J, Sayad E, Sockrider M. What Is a Spontaneous Pneumothorax? Am J Respir Crit Care Med 2020;202:33-4. [Crossref] [PubMed]
- Bosch de Basea Gomez M, Thierry-Chef I, Harbron R, et al. Risk of hematological malignancies from CT radiation exposure in children, adolescents and young adults. Nat Med 2023;29:3111-9. [Crossref] [PubMed]
- Barnes JL, Yoshida M, He P, et al. Early human lung immune cell development and its role in epithelial cell fate. Sci Immunol 2023;8:eadf9988. [Crossref] [PubMed]
- Beppu AK, Zhao J, Yao C, et al. Epithelial plasticity and innate immune activation promote lung tissue remodeling following respiratory viral infection. Nat Commun 2023;14:5814. [Crossref] [PubMed]
- Urbanowicz RA, Lamb JR, Todd I, et al. Altered effector function of peripheral cytotoxic cells in COPD. Respir Res 2009;10:53. [Crossref] [PubMed]
- Semenzato G, Bortolin M, Facco M, et al. Lung lymphocytes: origin, biological functions, and laboratory techniques for their study in immune-mediated pulmonary disorders. Crit Rev Clin Lab Sci 1996;33:423-55. [Crossref] [PubMed]
- Zheng MZM, Wakim LM. Tissue resident memory T cells in the respiratory tract. Mucosal Immunol 2022;15:379-88. [Crossref] [PubMed]
- Cabrera López C, Sánchez Santos A, Lemes Castellano A, et al. Eosinophil Subtypes in Adults with Asthma and Adults with Chronic Obstructive Pulmonary Disease. Am J Respir Crit Care Med 2023;208:155-62. [Crossref] [PubMed]
- Malm-Erjefält M, Persson CG, Erjefält JS. Degranulation status of airway tissue eosinophils in mouse models of allergic airway inflammation. Am J Respir Cell Mol Biol 2001;24:352-9. [Crossref] [PubMed]
- Davis WB, Fells GA, Sun XH, et al. Eosinophil-mediated injury to lung parenchymal cells and interstitial matrix. A possible role for eosinophils in chronic inflammatory disorders of the lower respiratory tract. J Clin Invest 1984;74:269-78. [Crossref] [PubMed]
- Xu X, Yu T, Dong L, et al. Eosinophils promote pulmonary matrix destruction and emphysema via Cathepsin L. Signal Transduct Target Ther 2023;8:390. [Crossref] [PubMed]
- Carter AR, Anderson EL. Correct illustration of assumptions in Mendelian randomization. Int J Epidemiol 2024;53:dyae050. [Crossref] [PubMed]
- Lawlor DA, Harbord RM, Sterne JA, et al. Mendelian randomization: using genes as instruments for making causal inferences in epidemiology. Stat Med 2008;27:1133-63. [Crossref] [PubMed]
- Han C, Lim YH, Jung K, et al. Association Between Ambient Air Pollution Exposure and Spontaneous Pneumothorax Occurrence. Epidemiology 2019;30:S48-56. [Crossref] [PubMed]
- Li W, Wang W. Causal effects of exposure to ambient air pollution on cancer risk: Insights from genetic evidence. Sci Total Environ 2024;912:168843. [Crossref] [PubMed]
- Smith GD, Ebrahim S. 'Mendelian randomization': can genetic epidemiology contribute to understanding environmental determinants of disease? Int J Epidemiol 2003;32:1-22. [Crossref] [PubMed]
- Orrù V, Steri M, Sidore C, et al. Complex genetic signatures in immune cells underlie autoimmunity and inform therapy. Nat Genet 2020;52:1036-45. [Crossref] [PubMed]
- Vuckovic D, Bao EL, Akbari P, et al. The Polygenic and Monogenic Basis of Blood Traits and Diseases. Cell 2020;182:1214-1231.e11. [Crossref] [PubMed]
- Skrivankova VW, Richmond RC, Woolf BAR, et al. Strengthening the Reporting of Observational Studies in Epidemiology Using Mendelian Randomization: The STROBE-MR Statement. JAMA 2021;326:1614-21. [Crossref] [PubMed]
- Skrivankova VW, Richmond RC, Woolf BAR, et al. Strengthening the reporting of observational studies in epidemiology using mendelian randomisation (STROBE-MR): explanation and elaboration. BMJ 2021;375: [Crossref] [PubMed]
- Emdin CA, Khera AV, Kathiresan S. Mendelian Randomization. JAMA 2017;318:1925-6. [Crossref] [PubMed]
- Mitani A, Hakamata Y, Hosoi M, et al. The incidence and risk factors of asymptomatic primary spontaneous pneumothorax detected during health check-ups. BMC Pulm Med 2017;17:177. [Crossref] [PubMed]
- Nakanishi T, Forgetta V, Handa T, et al. The undiagnosed disease burden associated with alpha-1 antitrypsin deficiency genotypes. Eur Respir J 2020;56:2001441. [Crossref] [PubMed]
- Boone PM, Scott RM, Marciniak SJ, et al. The Genetics of Pneumothorax. Am J Respir Crit Care Med 2019;199:1344-57. [Crossref] [PubMed]
- Bense L, Eklund G, Wiman LG. Smoking and the increased risk of contracting spontaneous pneumothorax. Chest 1987;92:1009-12. [Crossref] [PubMed]
- Staley JR, Blackshaw J, Kamat MA, et al. PhenoScanner: a database of human genotype-phenotype associations. Bioinformatics 2016;32:3207-9. [Crossref] [PubMed]
- Kamat MA, Blackshaw JA, Young R, et al. PhenoScanner V2: an expanded tool for searching human genotype-phenotype associations. Bioinformatics 2019;35:4851-3. [Crossref] [PubMed]
- Bowden J, Holmes MV. Meta-analysis and Mendelian randomization: A review. Res Synth Methods 2019;10:486-96. [Crossref] [PubMed]
- Burgess S, Small DS, Thompson SG. A review of instrumental variable estimators for Mendelian randomization. Stat Methods Med Res 2017;26:2333-55. [Crossref] [PubMed]
- Bowden J, Davey Smith G, Haycock PC, et al. Consistent Estimation in Mendelian Randomization with Some Invalid Instruments Using a Weighted Median Estimator. Genet Epidemiol 2016;40:304-14. [Crossref] [PubMed]
- Burgess S, Thompson SG. Interpreting findings from Mendelian randomization using the MR-Egger method. Eur J Epidemiol 2017;32:377-89. [Crossref] [PubMed]
- Greco M FD, Minelli C, Sheehan NA, et al. Detecting pleiotropy in Mendelian randomisation studies with summary data and a continuous outcome. Stat Med 2015;34:2926-40. [Crossref] [PubMed]
- Burgess S, Bowden J, Fall T, et al. Sensitivity Analyses for Robust Causal Inference from Mendelian Randomization Analyses with Multiple Genetic Variants. Epidemiology 2017;28:30-42. [Crossref] [PubMed]
- Yavorska OO, Burgess S. MendelianRandomization: an R package for performing Mendelian randomization analyses using summarized data. Int J Epidemiol 2017;46:1734-9. [Crossref] [PubMed]
- Hedevang Olesen W, Katballe N, Sindby JE, et al. Cannabis increased the risk of primary spontaneous pneumothorax in tobacco smokers: a case-control study. Eur J Cardiothorac Surg 2017;52:679-85. [Crossref] [PubMed]
- Lee SH, Choi H, Kim S, et al. Association between anger and first-onset primary spontaneous pneumothorax. Gen Hosp Psychiatry 2008;30:331-6. [Crossref] [PubMed]
- De Smedt A, Vanderlinden E, Demanet C, et al. Characterisation of pleural inflammation occurring after primary spontaneous pneumothorax. Eur Respir J 2004;23:896-900. [Crossref] [PubMed]
- Rojas-Quintero J, Ochsner SA, New F, et al. Spatial Transcriptomics Resolve an Emphysema-Specific Lymphoid Follicle B Cell Signature in Chronic Obstructive Pulmonary Disease. Am J Respir Crit Care Med 2024;209:48-58. [Crossref] [PubMed]
- Faner R, Cruz T, Casserras T, et al. Network Analysis of Lung Transcriptomics Reveals a Distinct B-Cell Signature in Emphysema. Am J Respir Crit Care Med 2016;193:1242-53. [Crossref] [PubMed]
- Pierce SK, Liu W. Encoding immunological memory in the initiation of B-cell receptor signaling. Cold Spring Harb Symp Quant Biol 2013;78:231-7. [Crossref] [PubMed]
- Cancro MP, Tomayko MM. Memory B cells and plasma cells: The differentiative continuum of humoral immunity. Immunol Rev 2021;303:72-82. [Crossref] [PubMed]
- Habener A, Grychtol R, Gaedcke S, et al. IgA(+) memory B-cells are significantly increased in patients with asthma and small airway dysfunction. Eur Respir J 2022;60:2102130. [Crossref] [PubMed]
- Detková D, de Gracia J, Lopes-da-Silva S, et al. Common variable immunodeficiency: association between memory B cells and lung diseases. Chest 2007;131:1883-9. [Crossref] [PubMed]
- Shellito J, Suzara VV, Blumenfeld W, et al. A new model of Pneumocystis carinii infection in mice selectively depleted of helper T lymphocytes. J Clin Invest 1990;85:1686-93. [Crossref] [PubMed]
- Kolls JK. Helper T-cell type 17 cytokines and immunity in the lung. Ann Am Thorac Soc 2014;11:S284-6. [Crossref] [PubMed]
- Kolls JK. CD4(+) T-cell subsets and host defense in the lung. Immunol Rev 2013;252:156-63. [Crossref] [PubMed]
- Gattinoni L, Lugli E, Ji Y, et al. A human memory T cell subset with stem cell-like properties. Nat Med 2011;17:1290-7. [Crossref] [PubMed]
- Wang Z, Zheng T, Zhu Z, et al. Interferon gamma induction of pulmonary emphysema in the adult murine lung. J Exp Med 2000;192:1587-600. [Crossref] [PubMed]
- Raphael I, Joern RR, Forsthuber TG. Memory CD4(+) T Cells in Immunity and Autoimmune Diseases. Cells 2020;9:531. [Crossref] [PubMed]
- Viallard JF, Blanco P, André M, et al. CD8+HLA-DR+ T lymphocytes are increased in common variable immunodeficiency patients with impaired memory B-cell differentiation. Clin Immunol 2006;119:51-8. [Crossref] [PubMed]
- Barceló B, Pons J, Fuster A, et al. Intracellular cytokine profile of T lymphocytes in patients with chronic obstructive pulmonary disease. Clin Exp Immunol 2006;145:474-9. [Crossref] [PubMed]
- Koh CH, Lee S, Kwak M, et al. CD8 T-cell subsets: heterogeneity, functions, and therapeutic potential. Exp Mol Med 2023;55:2287-99. [Crossref] [PubMed]
- Arpaia N, Green JA, Moltedo B, et al. A Distinct Function of Regulatory T Cells in Tissue Protection. Cell 2015;162:1078-89. [Crossref] [PubMed]
- Kumar BV, Connors TJ, Farber DL, Human T. Cell Development, Localization, and Function throughout Life. Immunity 2018;48:202-13. [Crossref] [PubMed]