Prognostic model of lung adenocarcinoma based on immunoprognosis-related genes and related drug prediction
Highlight box
Key findings
• A new prognostic model was constructed to predict the prognosis of lung adenocarcinoma (LUAD), and several small molecule drugs targeting high-risk LUAD were predicted to provide new ideas for the treatment of LUAD.
What is known and what is new?
• Lung cancer is the most common malignant tumor in the world and immune microenvironment plays a critical role in cancer from onset to relapse.
• Based on immune-related prognostic genes, a new prognostic model for LUAD was constructed.
What is the implication, and what should change now?
• This study provides a new idea for the treatment of LUAD.
Introduction
Lung cancer (LC) remains the most common cause of human cancer death worldwide, and it accounts for greater than 25% (1). LC is divided into two major subtypes based on histology: small cell LC (SCLC) and non-small cell LC (NSCLC), which account for 15% and 85% of all cases, respectively (2). NSCLC is divided into squamous cell carcinoma, adenocarcinoma, and large cell carcinoma. Squamous cell carcinoma accounts for 25–30% of all LC cases, and lung adenocarcinoma (LUAD) is the most common type of LC and accounts for approximately 40% of all LC (3). The incidence of LUAD has increased recently due to air pollution, the increasing number of smokers, the aging population, and the popularization of medical checkups. The lack of obvious symptoms and rapid onset of LUAD in the early stage of its development has led to an overall five-year survival rate of less than 20% (4), and there are less than 15% of patients with advanced LUAD (5). With the development of precision medicine, gene-targeted therapeutic agents, such as gefitinib, erlotinib and crizotinib, are widely used in the clinical treatment of small cell carcinoma (6), and the survival rate of patients improves with targeted gene therapy.
The occurrence and development of tumor is closely related to the biological characteristics of tumor cells, immune system and immune microenvironment. Tumor microenvironment (TME) is a bidirectional, dynamic and complex network system, including various types of stromal cells (fibroblasts, lymphocytes and endothelial cells), immune cells (T lymphocytes, B lymphocytes and etc.) and extracellular components (cytokines, growth factors, hormones, extracellular matrix, etc.), which surrounds tumor cells and are nourished by the vascular system. It plays an important role in tumor progression, such as local drug resistance, cancer metastasis, immune escape and so on. NSCLC has always been regarded as a non-immunogenic tumor, but the latest research shows that NSCLC is one of the most sensitive cancers to monoclonal antibody immune checkpoint inhibitors (ICIs). For example, ICIs targeting the programmed death receptor 1 (PD-1)/programmed death receptor 1 (PD-L1) axis has changed the first-line treatment of patients with advanced NSCLC, and several PD-1/PD-L1 inhibitors have been approved to market, significantly improving the prognosis of patients. It can be seen that the study of immune system in LC is also of great significance.
Advances in genomic microarray and high-throughput sequencing technologies in combination with bioinformatics analysis has provided effective methods to study tumor development, and the widespread use of gene chips and RNA sequencing has also greatly enriched tumor-related data. The current study screened The Cancer Genome Atlas (TCGA) database for immune-related transcriptomic data in LUAD and normal lung tissues, constructed a prognostic model, and analyzed the TME and immune cell infiltration in tumor tissues via integration with the prognostic model. In addition, we analyzed the risk difference of transcriptome data in order to screen out the relevant risk factors to predict the relevant tumor therapeutic drugs. We present this article in accordance with the TRIPOD reporting checklist (available at https://jtd.amegroups.com/article/view/10.21037/jtd-24-569/rc).
Methods
Data collection
The transcriptional group data and clinical case data of patients with LUAD were obtained from TCGA database (http://portal.gdc.cancer.gov/). Immune-related genes were obtained from IMMPORT (The Immunology Database and Analysis Portal) database. The cancer/paracancerous tissues of LUAD patients involved in this study were resected by Department of Thoracic Surgery, and diagnosed as LUAD by the Department of Pathology, Affiliated Hospital of Nantong University. All tissue samples were quickly placed into the medium paraformaldehyde fixation solution after surgical resection. The study was approved by the Human Ethics Committee of the Affiliated Hospital of Nantong University (No. 2023-L017) and written informed consent was obtained from all patients. Human LUAD cell line (A549) was purchased from Shanghai Cell Bank of Chinese Academy of Sciences. The study was conducted in accordance with the Declaration of Helsinki (as revised in 2013).
Data processing
We used R software to extract messenger RNA (mRNA) and long non-coding RNA (LncRNA) data sets from transcriptome data, and then the immune related genes were screened out by using “limma” package in R software. The clinical data with clear sample information was analyzed through the Weighted Gene Co-expression Network analysis (WGCNA) to screen out and filter the modules related to survival. Table 1 lists the clinicopathological characteristics of the patients which with prognostic follow-up information. The survival data and gene expression data of the relevant samples in the selected module were combined, and a forest map of survival-related immune genes (P≤0.05) and survival-related immune LncRNAs (P≤0.01) was drawn using the “survival” package in R language.
Table 1
Clinical | Group | Data, n (%) |
---|---|---|
Age (years) | ≤65 | 241 (46.2) |
>65 | 262 (50.2) | |
Gender | Female | 280 (53.6) |
Male | 242 (46.4) | |
Stage | I–II | 403 (77.2) |
III–IV | 111 (21.3) | |
T | T1–2 | 453 (86.8) |
T3–4 | 66 (12.6) | |
M | M0 | 353 (67.6) |
M1 | 25 (4.8) | |
N | N0 | 335 (64.2) |
N1–3 | 175 (33.5) |
We included patients with prognostic follow-up information in the TCGA database into this table. However, the individual clinical characteristics of some patients may be missing, but we ensured that their prognostic information was complete. TCGA, The Cancer Genome Atlas; T, tumor; N, node; M, metastasis.
Construction of prognostic model
The samples obtained from the TCGA database were randomly divided into training and validation sets, and the core genes involved in model construction were screened using least absolute shrinkage and selection operator (LASSO) Cox and ten rounds of cross-validation using the formula, (Coefi: coefficient; Expi: expression of prognostic characteristic genes), to obtain the risk score. The risk score of each sample was calculated based on the expression levels of immune-related genes in the prognostic model, and all samples were divided into high-risk and low-risk groups by the median value of the risk score. Differential analysis was performed for the genes involved in model construction, and Kaplan-Meier survival analysis was used to assess the differences in overall survival (OS) between the high-risk and low-risk score groups. The “survival” and “time ROC” packages in R software were used to plot the receiver operating characteristic curve (ROC) for five-year OS. The ability of the prognostic model to predict five-year survival was evaluated by plotting the area under the curve (AUC) of the ROC. Univariate and multifactor Cox regression analyses were performed to verify whether the risk assessment calculated by the model was an independent predictor.
Clinical correlation analysis
The clinical data obtained from TCGA database were correlated and sorted out, and the “ComplexHeatmap” package of R software was used to draw the heat map of the difference of clinical traits between high-risk group and low-risk group.
Risk differential gene analysis
The samples were divided into high-risk and low-risk according to the constructed prediction model, and the genes differentially expressed in the high-and low-risk groups (|Log2 fold change (logFC)| >1 and false discovery rate (FDR) <0.05 were statistically significant) were screened by using the “limma” package in R software, The differentially expressed genes (DEGs) in the high-and low-risk groups were analyzed using GO enrichment, and KEGG enrichment analysis was performed. Enrichment analysis of DEGs was performed using GSEA software (7).
TME score and immune cell infiltration
Based on TCGA transcriptome data, the immune score, stromal score, and Estimation of STromal and Immune cells in MAlignant Tumor tissues using Expression data (ESTIMATE) score of the samples were calculated based on the immune and stromal cells in the tumor tissues using the “ESTIMATE” package in R software. The samples were divided into high-and low-score groups according to the scores, and prognostic analysis was performed on both groups. The relative expression of different immune cells between the high- and low-risk groups was analyzed using the “preprocessCore” package in R software, and the differentially expressed immune cells were screened for prognostic correlation analysis.
Connectivity Map (CMAP) analysis
CMAP (https://clue.io/) provides a wealth of information on small molecule drugs, gene expression, and closely related diseases at the genomic level (8). Researchers can link gene expression data to disease-related drugs, and a connectivity score from −1 to 1 is used to reflect the proximity between expression profiles. Drugs with negative scoring indicate potential therapeutic molecules. We uploaded high-risk genes associated with survival to CMAP and screened small molecule drugs with potential antitumor effects as new target candidates for high-risk LUAD patients (9).
In situ hybridization experiment
The paraffin slices were baked at 62 ℃ for two hours. The paraffin slices were dewaxed in the environmental dewaxing solution I and rehydrated with graded alcohol detergent. The paraffin sections were digested with protease K (20 µg/mL) at 37 ℃ and circled with a gene pen, then the pen ring was rinsed three times in PBS. The paraffin slices were then incubated with 3% methanol-H2O2 for 15 minutes and washed in PBS for 3 times. The slices were pre-hybridized in 37 ℃ hybridization buffer for 1 hour, and then hybridized overnight in the hybridization buffer containing probes in the thermostat. The following day, the slides were washed at 37 ℃ for 30 minutes, and then hybridized with corresponding branching probes. The slides were hybridized horizontally for 45 min in a wet box at 40 ℃, and the slides were washed at 37 ℃ for 20 minutes, diluted with a hybrid solution containing signal probes, diluted at 42 ℃ for 3 h. After taking the normal rabbit serum, the 30 min was sealed at room temperature, the HRP-mouse anti-DIG IgG was dropped, and the 50 min was incubated at 37 ℃. Finally, the five minutes was washed with PBS for four times. Adding newly prepared 3,3N-Diaminobenzidine tetrahydrochloride (DAB) chromogenic solution, and the positive was brown. After re-staining, the nucleus was dehydrated and sealed with graded alcohol.
Cell lines and culture
Human LUAD cell line (A549 and H1299) was from Nantong College, which were cultured in a cell incubator with 5% CO2 at 37 ℃ in complete culture medium composed of RMPI-1640 basic culture medium and 10% fetal bovine serum.
Cell growth assay
Cell growth was detected using a Cell Counting Kit-8 assay (CCK-8) Kit (China Seville Corporation, Wuhan, China) according to the manufacturer’s protocol. The cell suspension was inoculated in a 96-well plate (1,000 cells/well) and the same sample was repeated for three times. It was pre-cultured for a period of time (37 ℃, 5% CO2), then 0.25 and 1.00 µL drugs were added to each hole of the culture plate. CCK-8 (10 µL/well) was added at six, twelve, 24 and 48 hours, respectively. After an additional incubation of two hours, optical density (OD) was measured at the wavelength of 450 nm (OD450) using a microplate reader.
Statistical analysis
All analyses were performed using R language (version 4.2.1) and its appropriate packages. Data were analyzed using standard statistical tests as appropriate. P≤0.05 indicated a significant difference between or among the indicated groups. Multiple testing consideration was adjusted using the FDR method.
Results
Immune genes and immune LncRNAs related to prognosis
Transcriptomic and clinical data of LUAD samples were obtained from TCGA, and the mRNA and LncRNA datasets were extracted from the transcriptomic data using Perl software. The mRNA dataset was screened for genes associated with immunity. The clinical data were analyzed to remove samples with unknown data, and the remaining 346 samples were analyzed using the WCGNA module (Figure 1A). The red, brown and yellow modules correlate with survival status. The green module correlates with survival time. Genes in the red, brown, yellow and green modules were selected for subsequent analyses. Figure 1B,1C show the forest plots of mRNAs and LncRNAs associated with immune prognosis, respectively.
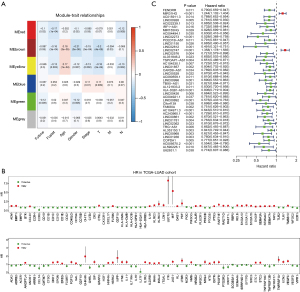
Construction and evaluation of prediction model
A total of 126 immune genes related to prognosis and 43 immune LncRNA related to prognosis were selected by WGCNA module analysis. The samples were randomly divided into training set and validation set, and the prognostic genes were identified by LASSO Cox regression algorithm (Figure 2A). Four core genes (LINC02747, DKK1, INSL4, VEGFC) were screened out through ten rounds of cross-validation (Figure 2B). Figure 2C makes a differential analysis of the genes involved in the construction of the model, and finds that the genes are differentially expressed between the high-risk group and the low-risk group.
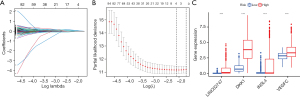
Then Kaplan-Meier survival analysis showed that the survival rate in the low-risk group (blue) was significantly better than that in the high-risk group (red) (Figure 3A). The same results were obtained in both the training set and the validation set (Figure 3B,3C).
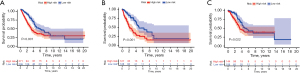
The risk score distribution (Figure 4A,4B) and the corresponding survival status (Figure 4C,4D) of patients in the training group and validation group showed that the OS time of the high-risk group was shorter, and the Figure 4E,4F show the heat map of gene expression in the prognostic model.
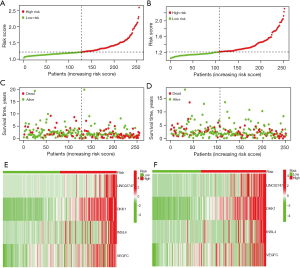
As Chen et al. (10-12) reported, the establishment of a prognostic model requires univariate and multifactorial independent prognostic analysis to verify the predictive efficacy and independence of the model. Univariate and multivariate independent prognostic analyses showed that the prognostic model could be used as an independent prognostic factor, and had nothing to do with age, sex, pathological stage and other clinical traits. In the univariate Cox regression analysis, the risk score of the training set and the corresponding risk ratio of hazard ratio (HR) [95% confidence interval (CI)] were 5.388 (3.153–9.207) (P<0.001) respectively, and the risk score of the validation set and the corresponding risk ratio of HR (95% CI) were 6.381 (2.969–13.715) (P<0.001) respectively (Figure 5A,5B). In multivariate Cox regression analysis, the risk score of the training set and the corresponding risk ratio of HR (95% CI) were 4.635 (2.643–8.127) (P<0.001), and the risk score of the validation set and the corresponding risk ratio of HR (95% CI) were 6.644 (3.058–14.434) (P<0.001) (Figure 5C,5D).
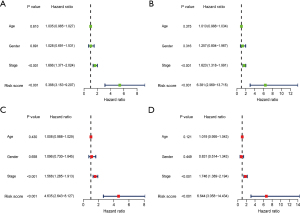
The AUC of the risk score of the combined set, the training set and the validation set were 0.667, 0.724 and 0.609 respectively by working characteristics of time dependent receptors (ROC) analysis (Figure 6A-6C), suggesting that the prognostic characteristics were reliable. In order to further evaluate whether the 4 core genes are involved in the occurrence and development of LUAD, we explored the relationship between risk score and clinicopathological factors in the combined set and the training set (Figure 6D,6E). The results showed that patients with high stage and lymph node metastasis had a higher risk than those with low stage and no lymph node metastasis (P<0.05). The risk score was also related to the clinical characteristics of age in the validation set (Figure 6F).
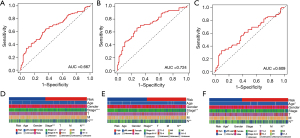
TME analysis and immune cell infiltration
Stromal and immune cells in tumor tissues were estimated from the expression data, and the stromal score, immune score, and ESTIMATE score of each sample were obtained using the ESTIMATE algorithm. The TME scores were analyzed in combination with the risk scores of the samples. Figure 7A-7C show that the immune scores were different in the high- and low-risk groups. The immune cells were higher in the low-risk group compared to the high-risk group, but the stromal scores and ESTIMATE scores were not significantly different. The samples were divided into high- and low-risk groups according to their scores, and Kaplan-Meier survival analysis shows that the high-risk group (red) had a better survival rate than the low-risk group (blue) based on stromal score, immune score and composite score (Figure 7D-7F).
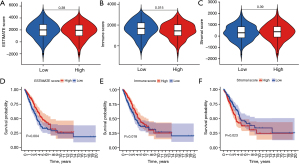
Taken together, these results suggested a correlation between immune cell content in LUAD tissue and patient survival prognosis. The relative contents of different immune cells in each sample were obtained by analyzing the expression of genes in the samples and the expression of genes in immune cells. The tumor tissue samples with high accuracy were screened according to the P value, and the relative contents of different immune cells in high-and low-risk samples were obtained by combining the risk scores of the samples. Figure 8A,8B show the histogram and heatmap of the expression content of different immune cells in the high- and low-risk groups. Figure 8C shows the difference in the expression of different immune cells between the high- and low-risk groups, based on P value and suggested that plasma cells, resting memory CD4+ T cells, follicular helper cells, resting natural killer (NK) cells, M0 macrophages, M2 macrophages, mast cells, eosinophils and neutrophils had differential expression. Compared to the low- risk group, plasma cells, resting NK cells, and eosinophils were downregulated in the high-risk group, and resting memory CD4+ T cells, follicular helper cells, macrophage M0, macrophage M2, mast cells, and neutrophils were upregulated in the high-risk group. The samples were divided into high and low expression groups according to the median immune cell values, and Kaplan-Meier survival analysis showed that the expression of M0 macrophages correlated with survival time and survival status. The survival rate of the M0 macrophage low expression group (blue) was better than the macrophage M0 high expression group (red) (Figure 8D).
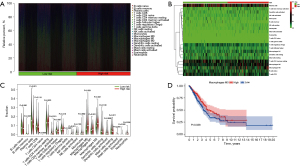
Risk differential gene analysis
Differential analysis of genes between the high- and low-risk groups was performed, and 201 low-risk genes and 351 high-risk genes were screened (Figure 9A). GO enrichment analysis (Figure 9B) and KEGG enrichment analysis (Figure 9C) were performed for DEGs. The most important GO enrichment analyses were epidermal development, collagen-containing extracellular matrix and receptor ligand activity. The KEGG enrichment analysis showed that the DEGs were primarily enriched in pathways associated with cytokine receptor interactions, the PIK3/ATK signaling pathway and human papillomavirus infection.
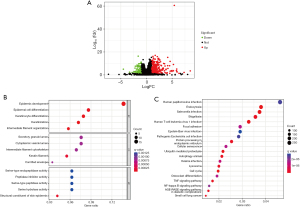
To identify significant changes in biological pathways between the high- and low-risk groups, further GSEA enrichment analysis was performed. Using FDR<0.05 and P<0.05 as filtering criteria, genes in the high-risk group were primarily enriched in seventeen significantly altered pathways including “cell regulation”, “P53 signaling pathway” and “cell cycle” (Figure 10A-10C); Three significantly altered pathways in the low-risk group were filtered according to P<0.05, including “linoleic acid metabolism”, “α-linolenic acid metabolism” and “arachidonic acid metabolism” pathways (Figure 10D-10F).
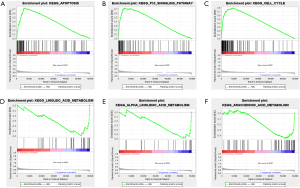
Screening of five LUAD candidate small molecule drugs
To identify new drugs for patients with LUAD, we performed CMAP analysis of high-risk genes and screened the five most relevant small molecule drugs online using the CMAP database (Table 2).
Table 2
Name | Score | Description |
---|---|---|
Tyrphostin-AG-1295 | 98.42 | PDGFR receptor inhibitor |
ABT-751 | 96.27 | Tubulin inhibitor |
Oxibendazole | 95.81 | Tubulin inhibitor |
Mirin | 95.24 | MRE11A exonuclease inhibitor |
Ingenol | 94.23 | PKC activator |
PDGFR, platelet-derived growth factor receptor; PKC, protein kinase C.
Verification of in situ hybridization and expression of LINC02747 in LUAD
DKK1, INSL4 and VEGFC have been reported to be highly expressed in LUAD, so in situ hybridization was used to detect the expression of LINC02747 in paracancerous tissues and LUAD tissues. By DAB Horseradish Peroxidase Color Development Kit, we found that the expression of LINC02747 in LUAD tissue was higher than that in normal lung epithelial tissue (Figure 11).
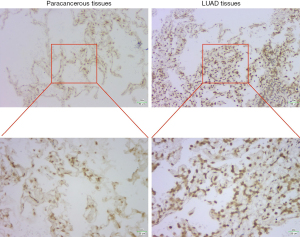
CCK-8 verifies that oxibendazole inhibits the growth of LUAD cells
After the A549 cells were plated, the CCK-8 was detected at 6, 12, 24 and 48 h after administration of 0.25 and 1.00 µm oxibendazole in the experimental group. Compared with the NC group, we found that oxibendazole could inhibit the growth of A549 cells and inhibit the activity of A549 cells in a time-dependent manner (Figure 12). Similarly, we further verified in the H1299 cell line that the growth and activity of H1299 cells were also inhibited by oxibendazole (Figure 13). These results indicate that oxibendazole had different inhibitory effects on LUAD cells cultured in vitro.
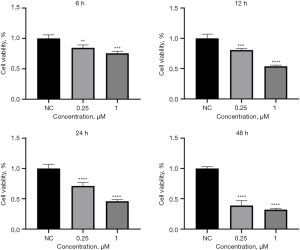
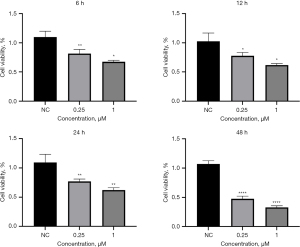
Discussion
LC is the main cause of cancer death in the world (13). LUAD is the most common histological subtype of primary LC. Due to the unobvious early symptoms of LC, patients are often at a late stage at initial diagnosis. Meanwhile, the prognosis is relatively poor, and the five-year survival rate is only about 19%. This low survival rate is related to many factors, including the patient’s age, with or without underlying disease, tumor size, tumor stage and postoperative treatment. In recent years, many studies have been carried out on LUAD, and a lot of progress has been made, but the molecular mechanism of carcinogenesis and progression of LUAD is still insufficient (14). In this study, we constructed a prognostic model consisting of four immune prognosis-related genes, which can be used to classify patients with LUAD into high and low risk groups. The characteristic genes come from the expression data of LUAD in TCGA, and the model is randomly divided into groups for verification, which proves the role of the model in the prognosis of LUAD.
These four immune prognosis-related genes included one LncRNA and three mRNA. Among them, a previous study has found that LINC02747 up-regulates the expression of TFE3 by adsorbing miR-608, and finally promotes the proliferation of clear cell renal cell carcinoma (15). However, it has not been found in the study of LUAD. In this study, we found that LINC02747 was also highly expressed in LUAD, so it is of great value to study whether LINC02747 plays a carcinogenic role in LUAD and its potential mechanism.
The imbalance of Dickkopf-associated protein 1 (Dickkopf-1, Dkk1) is related to the pathogenesis of various cancers. It is a member of the Dkk protein family, including Dickkopf-associated protein 2 (Dickkopf-2, Dkk2), Dickkopf-associated protein 3 (Dickkopf-3, Dkk3) and Dickkopf-associated protein 4 (Dickkopf-4, Dkk4). This secreted protein family shares a similar conserved cysteine domain and inhibits the Wnt/-catenin pathway by inducing proteasome B-catechin degradation, inducing apoptosis and preventing cell proliferation (16). One study has found that DKK1 can be used as an inhibitor of Wnt signal pathway to down-regulate the expression of Wnt-7a and promote the development of NSCLC (17). INSL4 was found for the first time in placental tissue and belongs to the relaxin/insulin-like peptide family. For many years, the peptide family has played a functional role in cancer (18). The functional consequences of relaxin receptor activation in cancer cells include cell movement ability increasing tumor growth and angiogenesis (19), all of these contribute to tumor expansion, tissue invasion and metastasis. INSL4 can be used as a prognostic marker for proliferation and invasiveness of NSCLC (20). Vascular endothelial growth factor C (VEGFC) can be promoted by amplified LncRNA PVT1 (plasma cell tumor variant translocation 1), thus promoting the proliferation and metastasis of LUAD (21). The above studies suggest that these may be new markers for the diagnosis, prognosis and treatment of LUAD. The risk score composed of these four genes divided the training set and validation set into high-risk group and low risk group, and the survival rate of the high-risk group was significantly lower than that of the low-risk group. This prognostic feature had been proved to be independent of other clinical traits as an independent prognostic factor and has a strong potential for clinical application.
TME plays an important role in tumor progression and metastasis, and there is a close relationship between inflammation and TME (22). Inflammation in TME is a characteristic of cancer, which is the interaction between inflammatory cells and tumor cells, thus affecting tumor progression (23). In this study, the TME score between the two risk groups showed that the high-risk group had lower immune score, resting memory CD4+T cells, follicular helper cells, macrophage M0, macrophage M2, mast cells and neutrophils were higher in the high-risk group. Macrophage M0 was abundant in the high-risk group and was related to the prognosis of patients. Tumor associated macrophages (TAM) are abundant in TME and participate in tumorigenesis, development, angiogenesis and metastasis (24). Preclinical and clinical data show that in many types of tumors such as pancreatic ductal adenocarcinoma (PDAC), glioblastoma and bladder cancer, the high infiltration of TAM is closely related to poor prognosis. At the same time, high TAM infiltration reduces the sensitivity of cancer patients to radiotherapy, chemotherapy and targeted therapy (25). TAM can be induced to polarize into typical activated “M1” macrophages and alternately activated “M2” macrophages (M0 macrophages are unpolarized immature macrophages). Different levels of immune cell infiltration have different effects on the prognosis of patients.
By comparing the genes expressed in high-risk group and low risk group, 405 DEGs were identified, including 119 low risk genes and 286 high risk genes. GO enrichment analysis showed that “epidermal development”, “keratin filament” and the structural components of “skin epidermis” were the most important biological processes, molecular function and cellular localization, respectively. KEGG enrichment analysis showed that the DEGs were mainly concentrated in “human papillomavirus infection”, “intracellular phagocytosis” and “salmonella infection”. GSEA enrichment analysis showed that the up-regulated genes in the high-risk group were mainly enriched in biological pathways such as “fine cell apoptosis”, “p53 signal pathway” and “cell cycle”. One study has found that 50% of invasive tumors have p53 gene mutations, which are often used as targets for anticancer therapy (26). Different from normal cells, tumor cells have an uncontrolled cell cycle, cells continue to divide and proliferate, and apoptosis-related signaling pathways in tumor cells are damaged (27). The imbalance of cell cycle is the main reason for the development of cancer, so the main mechanism of screening drugs is also related to the inhibition of cell cycle of cancer cells.
With the development of medical treatment and biotechnology, the treatment of LC has made a lot of progress, such as targeted therapy and immunotherapy, but only some patients can benefit from it. Therefore, new drugs for the treatment of LC still need to be developed. CMAP is a database of transcriptional expression of human cancer cells treated with compounds or drugs, which can be used to predict drugs based on changes in gene expression in diseases. Research shows that gliclazide could play an anticancer role in LUAD cells by CMAP analysis. We identified five small molecular drugs through CMAP analysis of high-risk genes. ABT-751 is a new oral antimitotic agent that inhibits microtubule polymerization (28). It has cytotoxicity in preclinical studies and this related toxicity is acceptable. Research found that ABT-751 combined with carboplatin inhibited the growth of LC fine cell lines, and it also shows moderate clinical anti-tumor activity in advanced NSCLC treated with carboplatin (29). Oxibendazole is a tubulin inhibitor and as a benzimidazole drug, it also shows anticancer activities in addition to antiparasitic drugs, such as disrupting microtubule polymerization, inducing apoptosis, stopping fine cell cycle (G2amp M), anti-angiogenesis and blocking glucose transport (30). Another study has shown that oxibendazole can induce apoptosis through mitochondrial-mediated calcium destruction and mitochondrial membrane potential destruction (31), which is related to cancer pathway. Ingenol, as a protein kinase C activator, has been found that ingenol 3,20 dibenzoate (IDB) is a tumor inhibitory protein kinase C (PKC) isozyme activator, which can increase the production of IFN-γ and degranulation of NK cells. Especially when NK cells are stimulated by NSCLC cells, IDB shows a strong anticancer effect by promoting NK cell-mediated killing of NSCLC cells (32). Mirin is a MRE11A exonuclease inhibitor. It has been found that mirin can inhibit events related to homologous recombination in a variety of cellular and biochemical contexts (33). Adel Alblihy et al. reported that mirin reverses platinum resistance in ovarian cancer cells and 3D sphere models through gene knockout or blocked Mre11 depletion (34). Kasey Jividen et al. found that inhibition of DNA repair enzyme MRE11 by small molecule mirin can inhibit androgen-dependent transcription and growth of prostate cancer cells (35), indicating that mirin has the potential to inhibit tumor cells. Tyrphostin-AG-1295 (casein AG1295) is an inhibitor of PDGFR tyrosine kinase. This study only has shown that it can inhibit the growth and invasion of Toxoplasma gondii (36), but its effect on tumor cells needs to be further studied. To sum up, ABT-751, oxibendazole and ingenol have potential therapeutic effects on LUAD, but the inhibitory effects of Mirin and tyrphostin-AG-1295 on LUAD need to be further studied. In this study, we successfully verified that oxibendazole can inhibit the growth and proliferation of LUAD cells in vitro. Other small molecular drugs are only data screening based on the database, and more studies are needed to verify the practicability of these molecules and carry out in vitro and in vivo experiments to clarify their molecular mechanisms.
In this study, the prognostic model was constructed by screening the immune genes related to the prognosis of LUAD in the database, and the accuracy of the model was verified. The samples were grouped by the model, and the DEGs in the high-risk group were analyzed. Meanwhile, the potential anticancer drugs for LUAD were screened by CMAP analysis, which provided an important reference for the treatment of LUAD. However, due to the fact that the transcriptome data and clinical information studied in this paper came from the TCGA database, the stability of the validation model using other databases could not be estimated. It is necessary to further expand the data sample size and validate the model with external data sets. Secondly, among the selected small molecular drugs, three drugs have been studied in LC cells, and the potential mechanism of other drugs in LC cells needs to be further studied. In addition, more research evidence is needed to prove the effectiveness and safety of the predicted molecular drugs. At the same time, the drug resistance of tumor cells to anticancer drugs is often the main reason for the failure of chemotherapy. Thus, on the basis of routine chemotherapy drugs, the combination with other targeted drugs to improve the efficacy is also a new treatment strategy.
Conclusions
Based on immune-related prognostic genes, we constructed a new prognostic model to predict the prognosis of LUAD, and several small molecule drugs targeting high-risk LUAD were predicted to provide new ideas for the treatment of LUAD.
Acknowledgments
Funding: This project was supported by a grant from
Footnote
Reporting Checklist: The authors have completed the TRIPOD reporting checklist. Available at https://jtd.amegroups.com/article/view/10.21037/jtd-24-569/rc
Data Sharing Statement: Available at https://jtd.amegroups.com/article/view/10.21037/jtd-24-569/dss
Peer Review File: Available at https://jtd.amegroups.com/article/view/10.21037/jtd-24-569/prf
Conflicts of Interest: All authors have completed the ICMJE uniform disclosure form (available at https://jtd.amegroups.com/article/view/10.21037/jtd-24-569/coif). Y.J. reports that this project was supported by National Natural Science Foundation of China, Youth Science Foundation Project (No. 82300412). The other authors have no conflicts of interest to declare.
Ethical Statement: The authors are accountable for all aspects of the work in ensuring that questions related to the accuracy or integrity of any part of the work are appropriately investigated and resolved. The study was conducted in accordance with the Declaration of Helsinki (as revised in 2013). The study was approved by the Human Ethics Committee of the Affiliated Hospital of Nantong University (No. 2023-L017) and informed consent was obtained from all patients.
Open Access Statement: This is an Open Access article distributed in accordance with the Creative Commons Attribution-NonCommercial-NoDerivs 4.0 International License (CC BY-NC-ND 4.0), which permits the non-commercial replication and distribution of the article with the strict proviso that no changes or edits are made and the original work is properly cited (including links to both the formal publication through the relevant DOI and the license). See: https://creativecommons.org/licenses/by-nc-nd/4.0/.
References
- Thai AA, Solomon BJ, Sequist LV, et al. Lung cancer. Lancet 2021;398:535-54. [Crossref] [PubMed]
- Nicholson AG, Tsao MS, Beasley MB, et al. The 2021 WHO Classification of Lung Tumors: Impact of Advances Since 2015. J Thorac Oncol 2022;17:362-87. [Crossref] [PubMed]
- Cheng TY, Cramb SM, Baade PD, et al. The International Epidemiology of Lung Cancer: Latest Trends, Disparities, and Tumor Characteristics. J Thorac Oncol 2016;11:1653-71. [Crossref] [PubMed]
- Hayashi T, Takamochi K, Kohsaka S, et al. Transformation from EGFR/PTEN co-mutated lung adenocarcinoma to small cell carcinoma in lymph node metastasis. Pathol Int 2020;70:295-9. [Crossref] [PubMed]
- Herbst RS, Morgensztern D, Boshoff C. The biology and management of non-small cell lung cancer. Nature 2018;553:446-54. [Crossref] [PubMed]
- Frese KK, Simpson KL, Dive C. Small cell lung cancer enters the era of precision medicine. Cancer Cell 2021;39:297-9. [Crossref] [PubMed]
- Jiline M, Matwin S, Turcotte M. Annotation concept synthesis and enrichment analysis: a logic-based approach to the interpretation of high-throughput experiments. Bioinformatics 2011;27:2391-8. [Crossref] [PubMed]
- Lamb J, Crawford ED, Peck D, et al. The Connectivity Map: using gene-expression signatures to connect small molecules, genes, and disease. Science 2006;313:1929-35. [Crossref] [PubMed]
- Wang Y, Xiao J, Suzek TO, et al. PubChem's BioAssay Database. Nucleic Acids Res 2012;40:D400-12. [Crossref] [PubMed]
- Chen H, Lin R, Lin W, et al. An immune gene signature to predict prognosis and immunotherapeutic response in lung adenocarcinoma. Sci Rep 2022;12:8230. [Crossref] [PubMed]
- Wang Z, Zhang J, Shi S, et al. Predicting lung adenocarcinoma prognosis, immune escape, and pharmacomic profile from arginine and proline-related genes. Sci Rep 2023;13:15198. [Crossref] [PubMed]
- Deng L, Long F, Wang T, et al. Identification of an Immune Classification and Prognostic Genes for Lung Adenocarcinoma Based on Immune Cell Signatures. Front Med (Lausanne) 2022;9:855387. [Crossref] [PubMed]
- Hutchinson BD, Shroff GS, Truong MT, et al. Spectrum of Lung Adenocarcinoma. Semin Ultrasound CT MR 2019;40:255-64. [Crossref] [PubMed]
- Anichini A, Perotti VE, Sgambelluri F, et al. Immune Escape Mechanisms in Non Small Cell Lung Cancer. Cancers (Basel) 2020;12:3605. [Crossref] [PubMed]
- Ju X, Sun Y, Zhang F, et al. Long Non-Coding RNA LINC02747 Promotes the Proliferation of Clear Cell Renal Cell Carcinoma by Inhibiting miR-608 and Activating TFE3. Front Oncol 2020;10:573789. [Crossref] [PubMed]
- Igbinigie E, Guo F, Jiang SW, et al. Dkk1 involvement and its potential as a biomarker in pancreatic ductal adenocarcinoma. Clin Chim Acta 2019;488:226-34. [Crossref] [PubMed]
- Stewart DJ. Wnt signaling pathway in non-small cell lung cancer. J Natl Cancer Inst 2014;106:djt356. [Crossref] [PubMed]
- Lok S, Johnston DS, Conklin D, et al. Identification of INSL6, a new member of the insulin family that is expressed in the testis of the human and rat. Biol Reprod 2000;62:1593-9. [Crossref] [PubMed]
- Silvertown JD, Symes JC, Neschadim A, et al. Analog of H2 relaxin exhibits antagonistic properties and impairs prostate tumor growth. FASEB J 2007;21:754-65. [Crossref] [PubMed]
- Scopetti D, Piobbico D, Brunacci C, et al. INSL4 as prognostic marker for proliferation and invasiveness in Non-Small-Cell Lung Cancer. J Cancer 2021;12:3781-95. [Crossref] [PubMed]
- Pan Y, Liu L, Cheng Y, et al. Amplified LncRNA PVT1 promotes lung cancer proliferation and metastasis by facilitating VEGFC expression. Biochem Cell Biol 2020;98:676-82. [Crossref] [PubMed]
- Balkwill F, Mantovani A. Inflammation and cancer: back to Virchow? Lancet 2001;357:539-45. [Crossref] [PubMed]
- Mantovani A, Sica A. Macrophages, innate immunity and cancer: balance, tolerance, and diversity. Curr Opin Immunol 2010;22:231-7. [Crossref] [PubMed]
- Xiang X, Wang J, Lu D, et al. Targeting tumor-associated macrophages to synergize tumor immunotherapy. Signal Transduct Target Ther 2021;6:75. [Crossref] [PubMed]
- Wu K, Lin K, Li X, et al. Redefining Tumor-Associated Macrophage Subpopulations and Functions in the Tumor Microenvironment. Front Immunol 2020;11:1731. [Crossref] [PubMed]
- Zhu J, Wang J, Wang T, et al. Identification of molecular subtypes, risk signature, and immune landscape mediated by necroptosis-related genes in non-small cell lung cancer. Front Oncol 2022;12:955186. [Crossref] [PubMed]
- Evan GI, Vousden KH. Proliferation, cell cycle and apoptosis in cancer. Nature 2001;411:342-8. [Crossref] [PubMed]
- Mauer AM, Cohen EE, Ma PC, et al. A phase II study of ABT-751 in patients with advanced non-small cell lung cancer. J Thorac Oncol 2008;3:631-6. [Crossref] [PubMed]
- Ma T, Fuld AD, Rigas JR, et al. A phase I trial and in vitro studies combining ABT-751 with carboplatin in previously treated non-small cell lung cancer patients. Chemotherapy 2012;58:321-9. [Crossref] [PubMed]
- Florio R, Carradori S, Veschi S, et al. Screening of Benzimidazole-Based Anthelmintics and Their Enantiomers as Repurposed Drug Candidates in Cancer Therapy. Pharmaceuticals (Basel) 2021;14:372. [Crossref] [PubMed]
- Park H, Lim W, You S, et al. Oxibendazole induces apoptotic cell death in proliferating porcine trophectoderm and uterine luminal epithelial cells via mitochondria-mediated calcium disruption and breakdown of mitochondrial membrane potential. Comp Biochem Physiol C Toxicol Pharmacol 2019;220:9-19. [Crossref] [PubMed]
- Gong C, Yao C, Xu Z, et al. Enhancement of NK cell-mediated lysis of non-small lung cancer cells by nPKC activator, ingenol 3,20 dibenzoate. Mol Immunol 2017;83:23-32. [Crossref] [PubMed]
- Shibata A, Moiani D, Arvai AS, et al. DNA double-strand break repair pathway choice is directed by distinct MRE11 nuclease activities. Mol Cell 2014;53:7-18. [Crossref] [PubMed]
- Alblihy A, Ali R, Algethami M, et al. Targeting Mre11 overcomes platinum resistance and induces synthetic lethality in XRCC1 deficient epithelial ovarian cancers. NPJ Precis Oncol 2022;6:51. [Crossref] [PubMed]
- Jividen K, Kedzierska KZ, Yang CS, et al. Genomic analysis of DNA repair genes and androgen signaling in prostate cancer. BMC Cancer 2018;18:960. [Crossref] [PubMed]
- Han Y, Adeyemi OS, Kabir MHB, et al. Screening of compound libraries for inhibitors of Toxoplasma growth and invasion. Parasitol Res 2020;119:1675-81. [Crossref] [PubMed]