The development and validation of a prognostic prediction modeling study in acute myocardial infarction patients after percutaneous coronary intervention: hemorrhea and major cardiovascular adverse events
Highlight box
Key findings
• When it comes to predicting multiple clinical outcomes, machine learning outperformed logistic regression, the best of which was eXtreme Gradient Boosting. SHapley Additive exPlanation provides the possibility for visual and personalized risk identification.
What is known and what is new?
• After years of development, machine learning can handle complex data better than traditional algorithms and has been applied in many fields.
• We tried to increase the application range of the model by adding different prediction outcomes, and make visual and personalized risk identification.
What is the implication, and what should change now?
• Through the model and online calculator (https://prediction-model-for-mace.streamlit.app/), doctors and patients can estimate the personalized prognosis risk anytime and anywhere, and make early adjustments. In the future, we need to further conduct external validation and gradually adjust the model parameters. If possible, case data from other regions can be used. Further researches to improve the interpretability of the model contribute to increase patients’ understanding of the disease and improves treatment compliance.
Introduction
Worldwide, it has been discovered that the cardiovascular disease (CVD) leads to the death of middle-aged and elderly people, and its incidence is steadily rising (1). A report (2) indicated that CVD ranks first among causes of disease death throughout urban and rural residents in China. Thus, CVD is responsible for 2 out of every 5 deaths. Acute myocardial infarction (AMI), a type of CVD, stands out as a frequently occurring condition characterized by sudden onset, an increased death and disability rate, and a high recurrence rate (3). Percutaneous coronary intervention (PCI) is one of the most important and effective diagnostic and treatment techniques for AMI. Also, complications after PCI have attracted wide interest, particularly the long-term prognosis after PCI (4). Although PCI can resolve coronary artery stenosis, it cannot affect atherosclerosis. Cardiovascular adverse events will still occur, and coronary artery stenosis will remain a possibility (5). Increasing incidences of major cerebrovascular and cardiovascular events can be discovered with prolongation of time after PCI (6). Further, patients who undergo PCI are at a higher risk of experiencing a cardiovascular adverse event such as rebleeding or reinfarction, which are unpredictable clinical difficulties (7). Therefore, it is very urgent to pay attention to the prediction of prognosis after PCI. Existing works have suggested the potential of machine learning to predict the major adverse events of CVD after PCI. Nonetheless, postoperative hemorrhea, cardiac death, and in-stent restenosis have not been appropriately predicted in CVD patients (8-11).
Traditional prediction models paid little attention on patients’ medication information while paying too much attention on the past history, laboratory data, ignoring the effects of medication on prognosis. In addition, traditional models developed on the basis of large-scale data may not be able to provide individualized risk assessment for specific patients.
As an important branch of artificial intelligence, a high-dimensional and complex mathematical model has been built by machine learning to perform fast and good classifications and regressions on clinical and imaging data. Compared to traditional models, machine learning can reduce overfitting through regularization techniques, cross-validation and ensemble learning, making the model more accurate in predicting large amounts of complex unknown data. Machine learning has shown great potential in healthcare for disease diagnosis, risk prediction, and identification (12-14). Motwani et al. studied 10,030 patients with suspected coronary artery disease (CAD) over a period of 5 years, all of whom underwent coronary computed tomography angiography (CCTA). The authors evaluated 25 clinical and 44 CCTA features, and both categories were positive for CAD. Through machine learning, the authors predicted the overall mortality rate with a higher area under the curve (AUC) (15). As of now, machine learning has gradually been applied to the prediction of adverse events after PCI. Previous studies researches paid little attention on patients’ medication information while paying too much attention on the past history, laboratory data, ignoring the effects of medication on prognosis. In addition, few research focused on individualized risk factor identification.
The purpose of this study was to build a prediction model with added information such as drug therapy through machine learning to accurately predict the prognosis of patients after PCI, control the risk of postoperative hemorrhea and major adverse cardiovascular events (MACEs), as well as provide more evidence for clinical treatment. The modeling study includes the development and validation process. We present this article in accordance with the TRIPOD reporting checklist (available at https://jtd.amegroups.com/article/view/10.21037/jtd-24-1362/rc).
Methods
Study population and criterion
The study was conducted in accordance with the Declaration of Helsinki (as revised in 2013). The study was approved by the Ethics Committee of The Affiliated Wuxi Second People’s Hospital (No. 2022-Y-174) and informed consent was taken from all the patients and their families. Jiangsu Provincial Hospital was informed and agreed with this study.
In this study, we extracted medical records, treatment plans, and clinical outcome data from Jiangsu Provincial Hospital and The Affiliated Wuxi Second People’s Hospital. Finally, we obtained a total of 7,931 cases. All patients who met the criteria for PCI between January 2007 and January 2022 were included in this study. The AMI patients with PCI met all the following criteria: (I) AMI must meet the diagnostic criteria set out in “Guidelines for the Diagnosis and Treatment of Acute ST-High Myocardial Infarction (2015 Edition)”(16); (II) without malignant tumors; (III) PCI was performed in an emergency situation; (IV) a good level of stability was maintained by the hemodynamics; (V) the patients were in good condition to detect the radial artery pulse; and (VI) informed consent forms were signed by the patients and their families. AMI patients with therapy contraindications were excluded from the study.
Study outcomes
Primary outcomes included hemorrhea, cardiac death, and in-stent restenosis. Hemorrhea was defined as major or minor bleeding with Bleeding Academic Research Consortium (BARC) (17) score ≥2. Cardiac death was defined as an unexpected sudden death due to a heart condition occurring within 1 hour of symptom onset. In-stent restenosis was defined as an event with re-narrowing of ≥50% of the vessel diameter.
Training and validation data
Of note, we randomly divided the entire data set into a validation set (30%, n=2,380) and a training set (70%, n=5,551). Classifiers can be biased toward the majority class if some classes have significantly fewer samples than others. To oversample the minority class, the Synthetic Minority Oversampling Technique (SMOTE) was applied in this study to balance the training set. Models were adjusted by 5-fold cross-validation. Later on, the best model with maximum mean AUC was applied to the validation set (Figure 1).
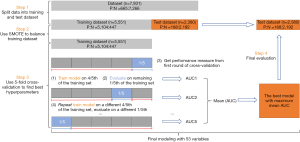
Feature selection
Vital signs, clinical manifestations, laboratory index, drug regimen, postoperative adverse events were recorded. After a hospital discharge, patient data were collected by trained researchers.
First, we selected 56 features and manually labeled each feature as a number or category. The features were as follows: sex, age (years), body mass index (BMI; kg/m2), weight (kg), anamnesis (history of hypertension, diabetes, coronary heart disease, myocardial infarction, cerebral infarction, smoking, and others), systolic and diastolic blood pressure (mmHg), heart rate (bpm), lab results [hemoglobin (g/L), platelet (PLT; ×109/L), and others], position of stents, application of drug-eluting stents (DESs) and drug-coated balloons (DCBs), left ventricular ejection fraction (%), treatment options [dual antiplatelet therapy (DAPT), statins, and others], and other detailed features.
Afterwards, the mean value, mode, and number of missing values were analyzed in detail. The number of missing values for each feature was counted and sorted (Figure 2). Features with more than 80% missing values were deleted, namely hemoglobin Alc (HbA1c), C-reactive protein (CRP), and cardiac troponin I (cTnI). Among features with less than 80% missing values, continuous features such as albumin (ALB) and white blood cells (WBCs), were filled with the mean, whereas discrete features (such as drug eluting stents) were filled with the mode.
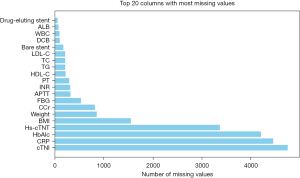
In order to understand the internal relationships between the features, we calculated and drew a correlation matrix of balanced data. Except that some features, for example smoking history significantly correlated with sex, had one-to-one correlation (Figure 3), we did not find that multiple features had complex correlations with each other. The selection of features was not affected. Ultimately, we included 53 features for the following study.
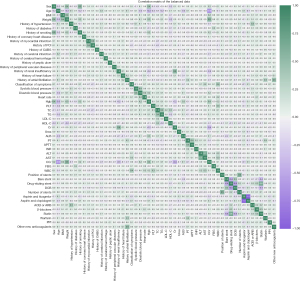
For each feature, we assessed the positive or negative effect on certain outcomes based on literature searches and clinical experience. For example, 24-hour blood pressure was strongly associated with all-cause mortality (18), whereas antiplatelet drugs had different effects on hemorrhea and in-stent restenosis. This helped feature preprocessing.
Statistical analysis
In this study, the versions of the tools and libraries we used were Python 3.10 (https://www.python.org/) and scikit-learn 1.3 (https://scikit-learn.org/) for the machine learning. In a respective manner, frequencies and percentages were used to describe discrete features (e.g., sex), whereas mean and standard deviation (SD) were employed to report continuous features (e.g., diastolic and systolic blood pressures). A P value <0.05 was set as the statistically significant threshold.
This study primarily applied various methods, including logistic regression (LR) and machine learning models, including eXtreme Gradient Boosting (XGBoost), random forest (RF), and deep learning neural network (DNN). Due to the large number of features involved, it was difficult to determine whether the relationship between features and outcomes was linear or non-linear. Therefore, LR was chosen in this study to explore the linear relationship, while other models could explore the nonlinear relationship. These were all supervised learning models that fitted the features in the training set based on labeled data. Through the training process, the model was able to capture the importance of each feature in prognosis prediction, generating a distribution of feature importance. On this basis, the model predicted the probabilities of different prognostic outcomes, providing an evaluation of the likelihood of each outcome, thus supporting subsequent decision-making analysis.
Model comparison
Herein, we drew receiver operating characteristic (ROC) curves of all the models before measuring their performance with sensitivity (Sens), specificity (Spec), accuracy (Acc), AUC, and net reclassification improvement (NRI). For imbalanced data sets, the binary classifiers’ performance was often evaluated using their AUCs. The model with higher Acc, Sens, Spec, AUC, and NRI has better predictive performance. Conversely, the closer the Acc, Sens, Spec, and NRI were to 0 (NRI was compared to LR) and the closer AUC was to 0.5, the worse the predictive performance was.
Model explanation
It was not convenient to directly interpret the model results, so the SHapley Additive exPlanation (SHAP) method was introduced to improve the interpretability of the results. Using the SHAP method, it was possible to understand the extent to which different features contribute to the prediction. In addition, the ranking of promoting and inhibiting features were visible when the prediction results were output for each unique patient.
Results
This study included a total of 7,931 independent cases, wherein the baseline characteristics were analyzed and the results are presented in Table 1. The patients had mean age of 65.8±11.3 years. Among all participants, men accounted for the majority (73.7%). With regards to the statistical analysis, 9 cardiovascular risk factors were selected. Most of the patients had hypertension (68.4%) or diabetes (70.5%), whereas nearly half of the patients were overweight (BMI ≥24 kg/m2, 46.1%), and more than half of the patients had a history of smoking (54.4%). In addition, only a small proportion had hyperlipidemia (39.4%). The baseline characteristics of the training and validation sets are shown in Table S1.
Table 1
Characteristics | Data |
---|---|
Age, years | 65.8±11.3 |
Sex | |
Male | 5,844 (73.7) |
Female | 2,087 (26.3) |
Cardiovascular risk factors | |
BMI ≥24 kg/m2 | 3,656 (46.1) |
Diabetes | 5,595 (70.5) |
Hyperlipidemia | 3,122 (39.4) |
Hypertension | 5,422 (68.4) |
Prior reperfusion surgery | 1,311 (16.5) |
Prior myocardial infarction | 418 (5.3) |
Prior cerebral infarction or hemorrhage | 991 (12.5) |
Family history of CVD | 418 (5.3) |
Smoking history | 4,313 (54.4) |
Data are presented as mean ± SD or n (%). BMI, body mass index; CVD, cardiovascular disease; SD, standard deviation.
In this study, we selected three machine learning models (RF, DNN, XGBoost) and LR. In the final comparison, overall NRIs of machine learning models were positive, whereas the prediction effect was improved compared with LR. Such results are in line with the advantages of machine learning in dealing with nonlinear, complex, and large data, among which XGBoost performed best in this study (Table 2).
Table 2
Outcome | Model | AUC (95% CI) | Accuracy | Sensitivity | Specificity | NRI |
---|---|---|---|---|---|---|
Hemorrhea | LR | 0.781 (0.733–0.829) | 0.715 | 0.671 | 0.759 | 0 |
RF | 0.852 (0.817–0.887) | 0.752 | 0.750 | 0.754 | 0.710 | |
DNN | 0.882 (0.810–0.954) | 0.803 | 0.773 | 0.833 | 0.101 | |
XGBoost | 0.921 (0.864–0.978) | 0.845 | 0.851 | 0.837 | 0.140 | |
Cardiac death | LR | 0.791 (0.722–0.860) | 0.806 | 0.878 | 0.538 | 0 |
RF | 0.884 (0.874–0.894) | 0.783 | 0.808 | 0.684 | 0.093 | |
DNN | 0.906 (0.855–0.957) | 0.913 | 0.945 | 0.789 | 0.115 | |
XGBoost | 0.939 (0.903–0.975) | 0.914 | 0.950 | 0.800 | 0.148 | |
In-stent restenosis | LR | 0.838 (0.792–0.884) | 0.750 | 0.699 | 0.814 | 0 |
RF | 0.863 (0.804–0.922) | 0.779 | 0.646 | 0.939 | 0.025 | |
DNN | 0.887 (0.829–0.945) | 0.801 | 0.778 | 0.829 | 0.049 | |
XGBoost | 0.915 (0.863–0.967) | 0.834 | 0.778 | 0.902 | 0.077 |
AUC, area under the curve; CI, confidence interval; NRI, net reclassification index; LR, logistic regression; RF, random forest, DNN, deep learning neural network; XGBoost, eXtreme Gradient Boost.
Hemorrhea
In the whole population, a total of 931 (3.9%) patients experienced bleeding. Among all of those with bleeding, hemorrhea with BARC score ≥2 accounted for 32.9% (306–931sts). This indicates that about 1/3 of patients with bleeding after PCI needed further treatment. The prediction effect of XGBoost on this event was impressive [AUC: 0.921, 95% confidence interval (CI): 0.864–0.978, Acc: 0.845, Sens: 0.851, Spec: 0.837, and NRI: 0.140] (Figure 4A), which was obviously better than other machine learning models and LR. The importance order was accordingly number of stents, position of stents, DCB, aspirin and clopidogrel, aspirin and ticagrelor, PLT, and other laboratory indexes (Figure 4B). The prediction of a unique individual suggested that the patient had a higher probability (0.94) of hemorrhea. Application of DCB, therapy of aspirin and ticagrelor, lack of proton pump inhibitor (PPI), β-blockers and angiotensin-converting enzyme inhibitor or angiotensin receptor blocker (ACEI or ARB), as well as weight (70 kg) had a promoting effect on hemorrhea, whereas the protective factor was the therapy of aspirin and clopidogrel (Figure 4C).
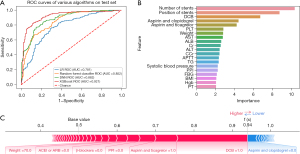
Cardiac death
A total of 107 (1.3%) patients died of heart diseases. Machine learning performed well in predicting cardiac death, with XGBoost still having the best predictive effect (AUC: 0.939, 95% CI: 0.903–0.975, Acc: 0.914, Sens: 0.950, Spec: 0.800 and NRI: 0.148) (Figure 5A). The features that affected the outcome in order of importance were age, number of stents, weight, DCB, urea and others (Figure 5B). During the model validation, a particular patient was shown to have had an extremely high chance (0.95) of experiencing cardiac death. For this patient, advanced age (80 years), urea (16.48 mmol/L), ALB (28 g/L), lack of PPI, creatinine (Cr; 200.3 µmol/L), history of smoking, and weight (70 kg) were the risk features, whereas the displayed protective feature was the therapy of ACEI or ARB (Figure 5C).
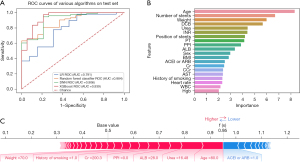
In-stent restenosis
Of all the patients undergoing PCI, 7,775 (98.0%) were implanted with stents and 218 (2.7%) had in-stent restenosis. The XGBoost far outperformed other models (AUC: 0.915, 95% CI: 0.863–0.967, Acc: 0.834, Sens: 0.778, Spec: 0.902, and NRI: 0.077) in terms of in-stent restenosis prediction (Figure 6A). The main predictors of this event were DBC, number of stents, position of stents, β-blockers, aspirin and ticagrelor, DES and others (Figure 6B). From a certain prediction outcome, the promoting features that affected in-stent restenosis were application of DCB, number of stents (3), history of smoking, alanine aminotransferase (ALT; 268 U/L), therapy of aspirin and ticagrelor, and diastolic blood pressure (56 mmHg). This patient exhibited a great probability (0.84) of in-stent restenosis (Figure 6C).
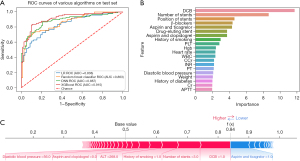
Discussion
The prognosis prediction is difficult yet of significant importance. Traditional LR is not always efficient (19-24). We have built a model to predict hemorrhea and MACE (cardiac death and in-stent restenosis) after PCI. Satisfactorily, the model showed good performance.
The XGBoost is a recently developed advanced machine learning algorithm, which integrates a series of decision trees into a more powerful classifier (25). Particularly, XGBoost integrates a sparse sensing algorithm for accurately handling missing values. All our case data came from the structured data of the medical system including the past history, vital signs, laboratory data, operation-related data, and postoperative treatment. The prediction can be carried out right after the postoperative treatment decision is completed, which provides evidence for subsequent intervention.
When dealing with medical problems using the SHAP method, it is important to understand that the explanations are not causal. For example, a large contribution of a feature does not mean that this feature is a risk factor of the outcome. This relationship can only indicate to what extent the performance of the model can be improved due to the contribution of the features.
Using the current features, we constructed an online calculator (https://prediction-model-for-mace.streamlit.app/). The calculator enables patients to make predictions of their own prognosis. Also, doctors are able to understand the weight of different features in the prediction result, then further evaluate and adjust the treatment strategy in combination with the clinical situation. There is no clear limit to how many features a well-performing model should involve, or the minimum number of features a model should contain for the sake of simplicity of the model and calculator. Therefore, in order to better predict multiple adverse events, we retained the features as much as possible to reduce the information loss. But even if we had bedside calculators, due to the large number of features our models contain, this may limit their practical application in clinical settings where simpler models may be preferred for ease of use.
The number and position of stents and DCB reflected the location and severity of lesions, and contributed the most to prediction. DAPT was also of high importance. Jeger et al. (26) found that the rate of major bleeding [Kaplan-Meier estimate 2% versus 4%, hazard ratio (HR) 0.43, 95% CI: 0.17–1.13; P=0.088] was lower in DCB versus DES. However, the different combined effects of number of stents, position of stents (or culprit vessels), and DCB need to be further evaluated. The choice of DAPT in patients also requires caution, as ticagrelor is associated with a higher risk of bleeding (27) and fatal bleeding unrelated to coronary artery bypass grafting (28).
Cardiac death is a serious adverse event, and the feature importance suggested that the most significant predictor was age. Older patients have an increased burden of cardiovascular risk factors and ischemic disease, which requires more individualized treatment or care decisions (29). The following important features were the reflection of hepatic, renal, and coagulation function. Body weight was not as direct a reflection of a patient’s obesity as BMI, but it may also affect the medicine dosage. The link between weight and cardiac death was not direct. Correlation matrix (Figure 3) showed the strongest correlation between weight and Cr clearance (Ccr), thus suggesting that body weight may be related to renal function. It has been shown that weight loss significantly improved renal function in overweight individuals (30).
Despite many improvements in stent design and polymer coatings over the past 2 decades, in-stent restenosis remains a common clinical problem. In the prediction of in-stent restenosis, the model suggested that DCB, the number and position of stents, DES, and DAPT therapy were important influencing factors. The selection of aspirin and ticagrelor had greater influence on in-stent restenosis than that of aspirin and clopidogrel. Ullrich found that DES, small target vessels, complex lesions, length of the lesion stenosis, and other implantation-related factors were predictors (31). The use of DCB is often associated with small CAD and stent dilation after implantation. The number and position of stents may also be related to the length of the lesion stenosis and the severity of overall coronary disease. This study lacks more direct data, and this information needs to be supplemented in the future.
The two centers we selected were two affiliated hospitals of Nanjing Medical University. The populations were all from Jiangsu Province, with similar diets and lifestyles, the same testing methods, diagnostic criteria, diagnostic, inclusion and exclusion criteria, so we merged the data of the two centers, and every data was independent and non-repetitive. We randomly allocated the merged data to the training set and validation set in proportion. Although the performance and generalization ability were weakened, the sample size during training and validation was guaranteed. Dong et al. also trained the data with a larger sample size by merging the data of the entire cohort in their study (32). It is worth noting that they applied the predictive model to clinical early warning rather than focusing on provisional prediction, strengthening the collaboration between nursing staffs and clinicians. This becomes another way in which machine learning may be applied in clinical settings.
With the development of computer technology, a single model can not always take into account different data types and usage scenarios, ensemble learning shows a new way to solve problems. Ensemble learning is used in machine learning and data science, especially for tasks such as classification and regression. It can use multiple models and take advantage of the diversity and complementarity of different models to make more robust and accurate predictions. Several studies have focused on the application of ensemble learning to medical prediction (33-35). For this study, the effect of ensemble learning was not evaluated, which will be further validated in the future.
Limitations
There are various studies that have clearly predicted the prognosis in different time periods within and outside the hospital, and obtained better prediction results (19,36). Due to the poor compliance of patients and the memory decline of elderly patients, the occurrence time of adverse events during the follow-up period was not recorded completely in this study. We could not draw time-to-event curves to effectively predict adverse events in different time periods. In the future, we need to strengthen in-hospital health education improve patients’ compliance. In addition, for time data, the phased analysis is also important.
Our approach to managing missing data, including the deletion of features with high levels of missingness and imputation for others, might have introduced biases or inaccuracies in the models. The extent to which these methods influenced model performance and predictions remains an area for further investigation.
The clinical data so far still contain a number of other questions: (I) the selection of 53 clinical features for model training was based on availability and presumed relevance to the outcomes of interest. However, this approach may have overlooked potentially influential predictors not included in our dataset. (II) Past history did not include current status assessment of patients, for example current smoking status was not assessed (such as frequency and quantity of cigarettes) or smoking cessation status (such as duration and method of smoking cessation). (III) Vital signs did not accurately contain dynamic information, only the specific value was identified at the time of admission. Also lacking in dynamic information was laboratory data. (IV) In the perioperative period, changes in vital signs and laboratory data were not recorded. Although the type and number of stents and balloons were included, lack of specific information such as the size of stents and balloons could not be ignored. (V) Treatment data did not involve other diseases. (VI) Human factors were not considered, such as the patient evaluation of the education experience, cognitive level, and treatment compliance, and an evaluation of the doctor’s professional title, years of practice, and experience. (VII) The predictive models were developed using data from two different centers, which may limit their applicability to different healthcare settings or populations with varied demographic characteristics. Future work should focus on validating and potentially adjusting these models with data from a broader range of settings. (VIII) The span of our dataset across fifteen years incorporates a period of significant evolution in medical practices and technologies related to PCI and AMI treatment. As such, the predictive performance of our models may vary when applied to more recent patient cohorts or future cases, necessitating periodic reevaluation and updating of the models to maintain their accuracy and relevance.
Conclusions
In this study, XGBoost in machine learning performed best in predicting post-PCI hemorrhea and MACE (including cardiac death and in-stent restenosis). Through the SHAP method, we increased the interpretability of the model so that clinicians can better understand the results. Clinicians can personalize patient management and adjust treatment decisions through bedside calculations. In future studies, we will conduct multi-center prospective cohort studies in conjunction with other centers to verify and adjust our model.
Acknowledgments
Funding: This work was supported by
Footnote
Reporting Checklist: The authors have completed the TRIPOD reporting checklist. Available at https://jtd.amegroups.com/article/view/10.21037/jtd-24-1362/rc
Data Sharing Statement: Available at https://jtd.amegroups.com/article/view/10.21037/jtd-24-1362/dss
Peer Review File: Available at https://jtd.amegroups.com/article/view/10.21037/jtd-24-1362/prf
Conflicts of Interest: All authors have completed the ICMJE uniform disclosure form (available at https://jtd.amegroups.com/article/view/10.21037/jtd-24-1362/coif). The authors have no conflicts of interest to declare.
Ethical Statement: The authors are accountable for all aspects of the work in ensuring that questions related to the accuracy or integrity of any part of the work are appropriately investigated and resolved. The study was conducted in accordance with the Declaration of Helsinki (as revised in 2013). Human experiments were approved by the Ethics Committee of The Affiliated Wuxi Second People’s Hospital (No. 2022-Y-174). Jiangsu Provincial Hospital was informed and agreed with this study. All participants and their families were fully informed about the study requirements, whereafter they read and signed an informed consent document.
Open Access Statement: This is an Open Access article distributed in accordance with the Creative Commons Attribution-NonCommercial-NoDerivs 4.0 International License (CC BY-NC-ND 4.0), which permits the non-commercial replication and distribution of the article with the strict proviso that no changes or edits are made and the original work is properly cited (including links to both the formal publication through the relevant DOI and the license). See: https://creativecommons.org/licenses/by-nc-nd/4.0/.
References
- Zhang Y, Cao H, Jiang P, et al. Cardiac rehabilitation in acute myocardial infarction patients after percutaneous coronary intervention: A community-based study. Medicine (Baltimore) 2018;97:e9785. [Crossref] [PubMed]
- Xiao M, Li Y, Guan X. Community-Based Physical Rehabilitation After Percutaneous Coronary Intervention for Acute Myocardial Infarction. Tex Heart Inst J 2021;48:e197103. [Crossref] [PubMed]
- Tehrani BN, Basir MB, Kapur NK. Acute myocardial infarction and cardiogenic shock: Should we unload the ventricle before percutaneous coronary intervention? Prog Cardiovasc Dis 2020;63:607-22. [Crossref] [PubMed]
- Miyachi H, Yamamoto T, Takayama M, et al. 10-Year Temporal Trends of In-Hospital Mortality and Emergency Percutaneous Coronary Intervention for Acute Myocardial Infarction. JACC Asia 2022;2:677-88. [Crossref] [PubMed]
- Nishihira K, Kuriyama N, Kadooka K, et al. Outcomes of Elderly Patients With Acute Myocardial Infarction and Heart Failure Who Undergo Percutaneous Coronary Intervention. Circ Rep 2022;4:474-81. [Crossref] [PubMed]
- Spinler SA, Hilleman DE, Cheng JW, et al. New recommendations from the 1999 American College of Cardiology/American Heart Association acute myocardial infarction guidelines. Ann Pharmacother 2001;35:589-617. [Crossref] [PubMed]
- Rigattieri S, Cristiano E, Tempestini F, et al. Lipoprotein(a) and the risk of recurrent events in patients with acute myocardial infarction treated by percutaneous coronary intervention. Minerva Cardiol Angiol 2023;71:406-413. [Crossref] [PubMed]
- Liu J, Zhang Q, Liu Z, et al. Microvascular reperfusion of fibrinolysis followed by percutaneous coronary intervention versus primary percutaneous coronary intervention for ST-segment-elevation acute myocardial infarction. Quant Imaging Med Surg 2024;14:765-776. [Crossref] [PubMed]
- Du M, Ye X, Li D, et al. Development of a prediction model for exercise tolerance decline in the exercise assessment of patients with acute myocardial infarction undergoing percutaneous coronary intervention revascularization in the acute phase. J Thorac Dis 2023;15:4486-4496. [Crossref] [PubMed]
- Xiao Z, Riletu A, Yan X, et al. Association of serum cystatin C level and major adverse cardiovascular events in patients with percutaneous coronary intervention. Cardiovasc Diagn Ther 2024;14:621-629. [Crossref] [PubMed]
- Ranasinghe S, Tjoe B, Shufelt C, et al. Association of abnormal electrocardiography response on dobutamine stress echocardiogram with longer-term major adverse cardiovascular events in women with symptoms of ischemic heart disease. Cardiovasc Diagn Ther 2023;13:948-955. [Crossref] [PubMed]
- Jiang X, Zhang H, Ni J, et al. Identifying tumor antigens and immune subtypes of gastrointestinal MALT lymphoma for immunotherapy development. Front Oncol 2022;12:1060496. [Crossref] [PubMed]
- Jiang X, Zhang H, Wang X, et al. Comprehensive Analysis of the Association between Human Diseases and Water Pollutants. Int J Environ Res Public Health 2022;19:16475. [Crossref] [PubMed]
- Ye S, Liu Q, Huang K, et al. The comprehensive analysis based study of perfluorinated compounds-Environmental explanation of bladder cancer progression. Ecotoxicol Environ Saf 2022;229:113059. [Crossref] [PubMed]
- Motwani M, Dey D, Berman DS, et al. Machine learning for prediction of all-cause mortality in patients with suspected coronary artery disease: a 5-year multicentre prospective registry analysis. Eur Heart J 2017;38:500-7. [PubMed]
- 2015 Chinese Society of Cardiology (CSC) guidelines for the diagnosis and management of patients with ST-segment elevation myocardial infarction. Chinese Journal of Cardiology 2015;43:380-93.
- Mehran R, Rao SV, Bhatt DL, et al. Standardized bleeding definitions for cardiovascular clinical trials: a consensus report from the Bleeding Academic Research Consortium. Circulation 2011;123:2736-47. [Crossref] [PubMed]
- Staplin N, de la Sierra A, Ruilope LM, et al. Relationship between clinic and ambulatory blood pressure and mortality: an observational cohort study in 59 124 patients. Lancet 2023;401:2041-50. [Crossref] [PubMed]
- Zack CJ, Senecal C, Kinar Y, et al. Leveraging Machine Learning Techniques to Forecast Patient Prognosis After Percutaneous Coronary Intervention. JACC Cardiovasc Interv 2019;12:1304-11. [Crossref] [PubMed]
- Nakachi T, Yamane M, Kishi K, et al. Machine Learning for Prediction of Technical Results of Percutaneous Coronary Intervention for Chronic Total Occlusion. J Clin Med 2023;12:3354. [Crossref] [PubMed]
- Chen P, Wang B, Zhao L, et al. Machine learning for predicting intrahospital mortality in ST-elevation myocardial infarction patients with type 2 diabetes mellitus. BMC Cardiovasc Disord 2023;23:585. [Crossref] [PubMed]
- Mortazavi BJ, Bucholz EM, Desai NR, et al. Comparison of Machine Learning Methods With National Cardiovascular Data Registry Models for Prediction of Risk of Bleeding After Percutaneous Coronary Intervention. JAMA Netw Open 2019;2:e196835. [Crossref] [PubMed]
- Ding LP, Li P, Yang LR, et al. A novel machine learning model to predict high on-treatment platelet reactivity on clopidogrel in Asian patients after percutaneous coronary intervention. Int J Clin Pharm 2024;46:90-100. [Crossref] [PubMed]
- Galimzhanov A, Matetic A, Tenekecioglu E, et al. Prediction of clinical outcomes after percutaneous coronary intervention: Machine-learning analysis of the National Inpatient Sample. Int J Cardiol 2023;392:131339. [Crossref] [PubMed]
- Chen T, Guestrin C. Xgboost: A scalable tree boosting system. In Proceedings of the 22nd ACM SIGKDD International Conference on Knowledge Discovery and Data Mining, Association for Computing Machinery 2016;10:785-94.
- Jeger RV, Farah A, Ohlow MA, et al. Long-term efficacy and safety of drug-coated balloons versus drug-eluting stents for small coronary artery disease (BASKET-SMALL 2): 3-year follow-up of a randomised, non-inferiority trial. Lancet 2020;396:1504-10. [Crossref] [PubMed]
- Tjerkaski J, Jernberg T, Alfredsson J, et al. Comparison between ticagrelor and clopidogrel in myocardial infarction patients with high bleeding risk. Eur Heart J Cardiovasc Pharmacother 2023;9:627-35. [Crossref] [PubMed]
- Wallentin L, Becker RC, Budaj A, et al. Ticagrelor versus clopidogrel in patients with acute coronary syndromes. N Engl J Med 2009;361:1045-57. [Crossref] [PubMed]
- Choi KH, Park YH, Song YB, et al. Long-term Effects of P2Y12 Inhibitor Monotherapy After Percutaneous Coronary Intervention: 3-Year Follow-up of the SMART-CHOICE Randomized Clinical Trial. JAMA Cardiol 2022;7:1100-8. [Crossref] [PubMed]
- Sandhu K, Nadar SK. Percutaneous coronary intervention in the elderly. Int J Cardiol 2015;199:342-55. [Crossref] [PubMed]
- Ullrich H, Olschewski M, Münzel T, et al. Coronary In-Stent Restenosis: Predictors and Treatment. Dtsch Arztebl Int 2021;118:637-44. [PubMed]
- Dong J, Feng T. Thapa-Chhetry Bet al. Machine learning model for early prediction of acute kidney injury (AKI) in pediatric critical care. Crit Care. 2021;25:288. [Crossref] [PubMed]
- Pliakos K, Vens C. Drug-target interaction prediction with tree-ensemble learning and output space reconstruction. BMC Bioinformatics. 2020;21:49. [Crossref] [PubMed]
- Wang L, Liu H, Pan Z, Fan D, Zhou C, Wang Z. Long Short-Term Memory Neural Network with Transfer Learning and Ensemble Learning for Remaining Useful Life Prediction. Sensors (Basel) 2022;22:5744. [Crossref] [PubMed]
- Lekkas D, Price GD, Jacobson NC. Using Smartphone App Use and Lagged-Ensemble Machine Learning for the Prediction of Work Fatigue and Boredom. Comput Human Behav. 2022;127:107029. [Crossref] [PubMed]
- Doll JA, O’Donnell CI, Plomondon ME, et al. Contemporary Clinical and Coronary Anatomic Risk Model for 30-Day Mortality After Percutaneous Coronary Intervention. Circ Cardiovasc Interv 2021;14:e010863. [Crossref] [PubMed]