Predicting the invasiveness of ground-glass opacity predominant lung adenocarcinoma with clinical stage Ia: a CT-based semantic and radiomics analysis
Highlight box
Key findings
• Computed tomography (CT)-based radiomics and semantic features can accurately assess the quantitative risk associated with the invasiveness of ground-glass opacity (GGO) predominant lung adenocarcinoma in clinical stage Ia.
What is known and what is new?
• GGO predominant pulmonary nodules are commonly considered associated with several specific CT semantic features.
• Radiomics has the potential to enhance the predictive accuracy of models, as evidenced by a significant increase in the area under the curve of the combined model compared to that of the CT semantic model.
What is the implication, and what should change now?
• The results of our study underlined the potential importance of CT-based radiomics and semantic features in the management of GGO predominant pulmonary nodules.
Introduction
Globally, lung cancer is the leading cause of cancer-related deaths. Non-small cell lung cancer (NSCLC) makes up approximately 80–85% of all lung cancers, with lung adenocarcinoma (ADC) being the most common histological type (1,2). To achieve the highest likelihood of a cure, the established treatment approach for early-stage NSCLC involves lobectomy in conjunction with systematic lymph nodes (LNs) dissection (3). Advancements in early lung cancer screening and low-dose computed tomography (CT) technology have increased detection of early and small volume lung cancers, leading to a shift towards less invasive surgical procedures to preserve lung function. Numerous studies have demonstrated that the 5-year survival rates for preinvasive and minimally invasive adenocarcinoma (MIA) have approached nearly 100%, whereas that for invasive adenocarcinoma (IAC) has ranged from 38% to 74.6% (4,5). Hence, certain scholars have proposed a conservative approach for the follow-up of preinvasive ADC, while limited surgical resection may be considered for MIA instead of lobectomy (6,7). Unfortunately, accurately diagnosing the invasiveness of ADC before surgery remains difficult, despite using frozen sections or biopsy specimens (8,9). Precise preoperative classification is crucial for tailoring surgical strategies for individual patients.
Chest CT is the preferred non-invasive method for detecting lung diseases, including ground-glass nodules (GGNs) which appear as slightly denser shadows on scans, where the vascular and bronchial bundles are not obscured (10,11). Early NSCLC often presents as part-solid or pure GGN on thin slice CT scans, especially MIA (12,13). The assessment of subsolid nodules primarily relies on radiologists’ subjective evaluation of factors like nodule shape, size, and surroundings, which may lead to inaccurate diagnoses (14,15). Radiomics is the process of converting images into quantitative data through machine-learning methods (16,17), and has become a valuable tool for quantitatively analyzing the inherent heterogeneity of GGN (18). However, prior radiomics studies pertaining to the invasiveness assessment have primarily been conducted as single-center studies, frequently involving preinvasive lesions (19-21).
Consequently, the present study aimed to address this limitation by focusing on ground-glass opacity (GGO) predominant ADC with clinical stage Ia, and undertaking a retrospective, double-center clinical study. The objective was to preoperatively and non-invasively predict the invasiveness of ADC through radiomics analysis of CT examination, thereby assisting surgeons in selecting appropriate treatment strategies and surgical options. We present this article in accordance with the TRIPOD reporting checklist (available at https://jtd.amegroups.com/article/view/10.21037/jtd-24-775/rc).
Methods
Patients
The study was conducted in accordance with the Declaration of Helsinki (as revised in 2013). The study was approved by the Medical Research Ethics Committee and the Institutional Review Board of Tianjin Medical University General Hospital (No. IRB2024-KY-240) and Tianjin Medical University Cancer Institute and Hospital (No. Ek2021067). Individual consent for this retrospective analysis was waived. Initial enrollment included 1,326 patients with surgery dates from January 2016 to May 2023 in accordance with the following inclusion criteria: (I) patients with lung ADC who underwent surgical resection; (II) available chest thin-layer CT plain images in the picture archive and communication system 1 month prior to surgery; and (III) identification of patients in clinical stage Ia and availability of pathological information. We excluded patients who had received preoperative treatment (n=7), lesions with a GGO component of less than 50% (n=673), images of poor quality or inability to identify lesions (n=16), as well as cases of multiple primary lung cancers and carcinomas in situ confirmed by pathology (n=58) (Figure 1). Finally, a total of 572 patients were enrolled, of which 437 patients from the Tianjin Medical University Cancer Institute and Hospital were divided into the primary cohort, and other 135 patients from the Tianjin Medical University General Hospital were classified as the validation cohort. Clinical information, including age, sex, cigarette smoking status, and driving gene mutation status were obtained from the clinical database. ADCs in the lung were classified according to the 2015 World Health Organization (WHO) classification system (22). The tumor staging and tumor-node-metastasis (TNM) classification were conducted based on the 8th edition guidelines released by the Union for International Cancer Control and the American Joint Committee on Cancer (23).
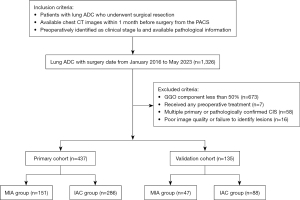
Definition of clinical stage Ia and ADC invasiveness
All patients who were enrolled in the study underwent preoperative chest CT scanning to evaluate the size of tumor and the status of LN. Additional examinations, such as whole abdomen CT, magnetic resonance imaging of the brain, bone scintigraphy, or positron emission tomography, were conducted to verify the absence of distant metastasis. The criteria for determining cN0 on CT scans was based on the short-axis diameter of all LNs being less than 10 mm. Pathological results were obtained from the pathological database, and the frequency of each histological subtype was recorded on the form by frequency when the pattern was >5%. Based on the histopathological findings, the cases were categorized into the MIA group and the IAC group.
CT scanning protocol
Three multidetector CT systems were used to perform chest CT: Lightspeed16 by GE Healthcare (Milwaukee, WI, USA), Somatom Sensation 64 by Siemens (Erlangen, Germany) and Discovery CT750 HD by GE Healthcare. For the 64-detector scanner, 120 kVp with the automatic regulation of the tube current were used, as well as 1.5 mm reconstruction thickness and intervals. The other two scanner types operated at 120 kVp, 150–200 mA, and 1.25 mm reconstruction thickness intervals.
CT image interpretation
Two clinical radiologists, possessing 6 and 9 years of experience in CT imaging of thoracic malignancies respectively, independently interpreted the CT images following specialized training without the awareness of clinicopathological information, as well as the confirmation of lesions with clinical stage Ia and GGO predominant. To resolve any discrepancies in image interpretation and achieve consensus, a senior radiologist with 40 years of experience provided assistance. The CT semantic features of the primary tumor and peritumoral lung tissue and pleura include location, maximum diameter, consolidation diameter, tumor shadow disappearance rate (TDR), contour, lobulation, spiculation, texture, calcification, air bronchogram, bubble-like lucency, cavity, pleural attachment, pleural retraction, bronchovascular bundle thickening, obstructive change, peripheral emphysema, and peripheral fibrosis. The CT descriptors were evaluated on multi-planar reconstruction images with lung window width 1,500 HU, window level −600 HU, and mediastinal window width 350 HU at level of 40 HU.
Tumor segmentation and features extraction
The thickness of CT images was resampled to 1 mm using a linear interpolation algorithm for image preprocessing. Additionally, Gaussian filtering was employed for further preprocessing of the CT images. Tumor segmentation was carried out independently by two radiologist utilizing ITK-snap 3.6.0, using the manual method of drawing regions of interest (ROIs) on the processed CT images at the lung window. The radiologists were aware of the tumor’s location but remained blinded to other relevant information. PyRadiomics 3.0 program, an open-source software (http://www.radiomics.io/pyradiomics.html) (24) was used to automatically extract radiomics features. Three-dimensional ROI CT images were used to extract a total of 1,316 radiomics features, including first-order (n=108), shape (n=14), gray level cooccurrence matrix (GLCM, n=144), gray level dependence matrix (GLDM, n=84), gray level run-length matrix (GLRLM, n=96), gray level size zone matrix (GLSZM, n=96), neighboring gray tone difference matrix (NGTDM, n=30) and higher-order wavelet features (n=744).
Feature selection and establishment of radiomics signature
The radiomics parameters extracted by two radiologists were averaged after standardizing using the Z-score method. Feature correlations were determined by Spearman pairwise correlation analysis, which eliminated features with absolute correlation values exceeding 0.9. By applying the minimum redundancy maximum relevance method, we selected the top 100 features. A least absolute shrinkage and selection operator (LASSO) model was then used to identify the optimal subsets for assessing the invasiveness of ADC. The identified optimal subsets were subsequently utilized to construct a logistic regression model to eliminate variables that were not statistically significant. The radiomics score (Rad-score) formula was derived by combining the selected features in a linear manner, with their coefficients serving as weights. By applying this formula, the Rad-score for each patient was calculated to assess the disparity between the MIA group and the IAC group.
Statistical analysis
Statistical analyses were conducted using R 4.3.0 and SPSS 26.0. Continuous variables were expressed using means and standard deviations, and categorical variables as frequency. An analysis of the agreement between two readers was carried out using the ĸ index and Kendall coefficient of concordance. Univariate analysis used non-parametric Wilcoxon tests for ranked and continuous variables, and Chi-squared or Fisher’s tests for categorical variables. Using the bootstrap methodology, models were assessed for predictive accuracy through multivariate logistic regression to determine the ability to identify IAC in different models. A nomogram was created for visualizing the final prediction model, followed by a Hosmer-Lemeshow test and calibration curve. An analysis of decision curve analysis (DCA) was performed to assess whether the nomogram model could be used to measure clinical diagnostic effects at different threshold probabilities. In order to assess the predictive efficacy, the area under the receiver operating characteristic (ROC) curve (AUC) was calculated, and Delong’s test would compare the AUC of different models. P values <0.05 were regarded as statistically significant.
Results
Demographics of all patients
An overview of the clinical characteristics and histological subtypes of each patient is presented in Table 1; 359, 156 and 57 patients were diagnosed with clinical stage T1a, T1b and T1c, respectively. Of all patients, women (403/572, 70.5%) and non-smokers (418/572, 73.1%) accounted for the vast majority; 198 (34.6%) and 374 (65.4%) cases were identified as MIA and IAC based on postoperative histopathology, respectively. The primary and validation cohorts didn’t differ significantly in either feature.
Table 1
Variables | Primary cohort | Validation cohort | P value |
---|---|---|---|
Number | 437 | 135 | |
Age (years) | 57.28±9.02 | 59.34±8.62 | 0.57 |
Sex | 0.50 | ||
Male | 126 | 43 | |
Female | 311 | 92 | |
Smoking history | 0.06 | ||
Yes | 126 | 28 | |
No | 311 | 107 | |
Histologic subtype | 0.96 | ||
MIA | 151 | 47 | |
IAC | 286 | 88 | |
EGFR | 0.77 | ||
Mutation | 120 | 53 | |
Wild | 64 | 26 | |
KRAS | 0.67 | ||
Mutation | 10 | 3 | |
Wild | 162 | 79 | |
ALK | >0.99 | ||
Positive | 7 | 3 | |
Negative | 144 | 58 | |
Clinical T stage | 0.56 | ||
T1a | 272 | 87 | |
T1b | 119 | 37 | |
T1c | 46 | 11 |
Data for age are mean ± standard deviation. MIA, microinvasive adenocarcinoma; IAC, invasive adenocarcinoma; EGFR, epidermal growth factor receptor; KRAS, Kirsten rat sarcoma viral oncogene; ALK, anaplastic lymphoma kinase.
Correlation of ADC invasiveness with clinicoradiological features in primary cohort
Table S1 shows that the two readers had a good level of agreement. In terms of maximum diameter, consolidation diameter, and TDR, the intraclass correlation coefficient was 0.93 (range, 0.91–0.94), 0.88 (range, 0.85–0.90), and 0.90 (range, 0.87–0.92), respectively.
An analysis of Table 2 illustrates the relationship between clinical features and invasiveness of ADC. Significantly, patients in MIA group were younger than that with IAC (P<0.001), and the proportion of women was higher (P<0.001), consequently more non-smokers (P=0.01). Patients with clinical stage T1b and T1c [105/119 (88.2%), 42/46 (91.3%) vs. 139/272 (51.1%)] developed IAC more frequently than T1a patients [odds ratio (OR) =7.81, 95% confidence interval (CI): 4.54, 13.46 for T1b and OR =6.33, 95% CI: 2.22, 18.00 for T1c; P<0.001]. No significant association was noted for other clinical features.
Table 2
Variables | MIA | IAC | P value | Univariate OR (95% CI) |
---|---|---|---|---|
Number | 151 | 286 | ||
Age (years) | 55.21±10.26 | 58.37±8.04 | <0.001 | |
Sex | <0.001 | |||
Male | 26 | 100 | Reference | |
Female | 125 | 186 | 0.39 (0.24, 0.63) | |
Smoking history | 0.01 | |||
Yes | 32 | 94 | Reference | |
No | 119 | 192 | 0.55 (0.35, 0.87) | |
EGFR | 0.11 | |||
Mutation | 22 | 98 | ||
Wild | 19 | 45 | ||
KRAS | >0.99 | |||
Mutation | 2 | 8 | ||
Wild | 37 | 125 | ||
ALK | >0.99 | |||
Positive | 2 | 5 | ||
Negative | 35 | 109 | ||
Clinical T stage | <0.001 | |||
T1a | 133 | 139 | Reference | |
T1b | 14 | 105 | 7.81 (4.54, 13.46) | |
T1c | 4 | 42 | 6.33 (2.22, 18.00) |
Data for age are mean ± standard deviation. ADC, adenocarcinoma; MIA, microinvasive adenocarcinoma; IAC, invasive adenocarcinoma; OR, odds ratio; CI, confidence interval; EGFR, epidermal growth factor receptor; KRAS, Kirsten rat sarcoma viral oncogene; ALK, anaplastic lymphoma kinase.
Based on univariate analysis, tumors with larger overall size (P<0.001) and solid component size (P<0.001), lower TDR (P<0.001), bronchovascular bundle thickening (OR =12.50, 95% CI: 1.67, 93.67; P=0.004), lobulation (OR =5.31, 95% CI: 2.06, 13.69; P<0.001), irregular contour (OR =10.15, 95% CI: 3.39, 30.42 for score 2; OR =9.87, 95% CI: 6.02, 16.20 for score 3; OR =5.19, 95% CI: 3.15, 8.56 for score 4; P<0.001), air bronchogram (OR =4.86, 95% CI: 3.02, 7.82; P<0.001), part-solid texture (OR =3.06, 95% CI: 2.03, 4.61; P<0.001) and pleural retraction (OR =5.00, 95% CI: 3.20, 7.81; P<0.001) were more likely to be IAC (Table 3). The rest of the radiological features (Table S2) were not statistically significant.
Table 3
Variables | MIA | IAC | P value | Univariate OR (95% CI) |
---|---|---|---|---|
Number | 151 | 286 | ||
Maximum diameter (cm) | 1.42±0.60 | 2.25±0.85 | <0.001 | |
Consolidation diameter (cm) | 0.33±0.51 | 1.03±0.86 | <0.001 | |
TDR | 0.78±0.32 | 0.56±0.33 | <0.001 | |
Contour | <0.001 | |||
Round | 19 | 4 | Reference | |
Oval | 62 | 26 | 10.15 (3.39, 30.42) | |
Somewhat irregular | 47 | 118 | 9.87 (6.02, 16.20) | |
Irregular | 23 | 138 | 5.19 (3.15, 8.56) | |
Bronchovascular bundle thickening | 0.004 | |||
Absence | 150 | 264 | Reference | |
Presence | 1 | 22 | 12.50 (1.67, 93.67) | |
Lobulation | <0.001 | |||
Absence | 146 | 242 | Reference | |
Presence | 5 | 44 | 5.31 (2.06, 13.69) | |
Air bronchogram | <0.001 | |||
Absence | 124 | 139 | Reference | |
Presence | 27 | 147 | 4.86 (3.02, 7.82) | |
Texture | <0.001 | |||
Pure GGO | 95 | 102 | Reference | |
GGO component ≥50% | 56 | 184 | 3.06 (2.03, 4.61) | |
Pleural retraction | <0.001 | |||
Absence | 116 | 114 | Reference | |
Presence | 35 | 172 | 5.00 (3.20, 7.81) |
Data for maximum diameter, consolidation diameter and TDR are mean ± standard deviation. CT, computed tomography; ADC, adenocarcinoma; MIA, microinvasive adenocarcinoma; IAC, invasive adenocarcinoma; OR, odds ratio; CI, confidence interval; TDR, tumor shadow disappear rate; GGO, ground-glass opacity.
Screening and integration of radiomics features
The three-dimensional ROI in each CT image yielded a comprehensive set of 1,316 radiomics features. Among the top 100 features, 11 were identified using the LASSO algorithm to mitigate the risk of overfitting (Table 4; Figure 2). Additionally, seven features were excluded through logistic regression with backward step-wise selection to eliminate non-significant variables. This process resulted in the identification of four radiomics features, in order to generate the Rad-score formula weighted by their coefficients (Table 4). Using a waterfall plot, the Rad-scores for each patient were displayed (Figure 3). Notably, the Rad-score exhibited a significant difference between the two groups (P<0.001), the mean value in MIA group was significantly higher (2.23±1.85) than that in IAC group (−1.12±2.17).
Table 4
Radiomics features | Regression coefficients | Significant predictors | |
---|---|---|---|
P value | Odds ratio (95% CI) | ||
original_gldm_Dependence Entropy | 0.096024564 | NA | |
square_glrlm_LongRunEmphasis | 0.032557823 | <0.001 | 2.76 (1.75, 4.66) |
squareroot_gldm_Dependence Entropy | 0.030621369 | NA | |
squareroot_firstorder_Skewness | 0.037556212 | NA | |
wavelet-LLH_gldm_Dependence Entropy | 0.029765043 | <0.001 | 2.91 (1.74, 4.85) |
wavelet-LHH_glcm_MCC | 0.005081872 | NA | |
wavelet-HLH_glcm_MCC | −0.003845782 | NA | |
wavelet-HHH_glcm_MCC | −0.006079658 | 0.02 | 0.52 (0.35, 0.88) |
wavelet-LLL_glcm_Joint Entropy | −0.010321374 | NA | |
wavelet-LLL_gldm_Large Dependence Low Gray Level Emphasis | −0.016845576 | NA | |
wavelet-LLL_ngtdm_Strength | −0.047721135 | 0.003 | 0.40 (0.23, 0.69) |
*, Rad-score = 0.982 + 1.043 × square_glrlm_LongRunEmphasis + 1.059 × wavelet-LLH_gldm_Dependence Entropy − 0.542 × wavelet-HHH_glcm_MCC − 0.926 × wavelet-LLL_ngtdm_Strength. Rad-score, radiomics score; CI, confidence interval; NA, not applicable.
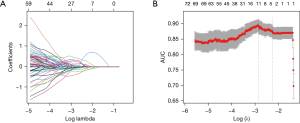
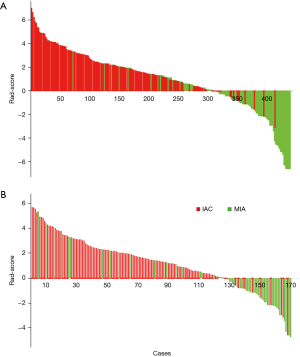
Predictive model and validation test
Larger consolidation diameter (OR =2.41, 95% CI: 1.37, 3.82, P=0.02), part-solid with GGO ≥50% (OR =1.56, 95% CI: 0.98, 2.61, P=0.042), and Rad-score (OR =2.88, 95% CI: 2.13, 3.84, P<0.001) were identified as the significant independent predictors in a multivariable logistic regression model (Table 5). A nomogram that incorporated these predictors was developed and presented in Figure 4. The Hosmer-Lemeshow goodness-of-fit test indicated a high degree of concordance between the predicted and observed probabilities (P=0.79). DCA and Calibration curve are shown in Figure 5. The DCA showed that the use of nomogram could provide an added net benefit compared to the “treat-all” or “treat-none” strategy with a threshold probability ranging from 15% to 95%, which indicated the nomogram was clinically useful.
Table 5
Variables | Significant predictors | ||
---|---|---|---|
P value | Odds ratio (95% CI) | Value in the formula* | |
Consolidation diameter | 0.02 | 2.41 (1.37, 3.82) | Numeric value |
Texture | 0.042 | ||
Pure GGO | Reference | 0 | |
GGO component ≥50% | 1.56 (0.98, 2.61) | 1 | |
Rad-score | <0.001 | 2.88 (2.13, 3.84) | Numeric value |
Maximum diameter | NA | NA | |
TDR | NA | NA | |
Contour | NA | NA | |
Bronchovascular bundle thickening | NA | NA | |
Lobulation | NA | NA | |
Air bronchogram | NA | NA | |
Pleural retraction | NA | NA | |
Age | NA | NA | |
Sex | NA | NA | |
Smoking history | NA | NA | |
Clinical T stage | NA | NA |
*, formula: ex/(1 + ex), x = −7.138 + 3.102 × consolidation diameter + 2.106 × texture +0.935 × Rad-score. Rad-score, radiomics score; ADC, adenocarcinoma; CI, confidence interval; GGO, ground-glass opacity; TDR, tumor shadow disappear rate; NA, not applicable.
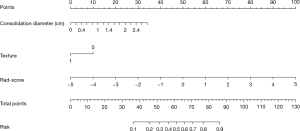
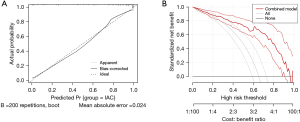
The AUC of the integrated model rose to 0.911 (95% CI: 0.872, 0.951), in contrast to 0.883 (95% CI: 0.849, 0.932; DeLong’s test P=0.16) and 0.842 (95% CI: 0.801, 0.896; DeLong’s test P<0.001) when only radiomics or CT semantic features were utilized separately (Figure 6A). Based on the maximum Youden index as the cutoff, the combined model exhibited 85.7% sensitivity, 83.9% specificity, and 82.6% accuracy. AUC of 0.865 (95% CI: 0.816, 0.908) indicated a reasonable accuracy of combined model for the validation cohort with a sensitivity of 81.6%, specificity of 79.5%, and accuracy of 82.7% (Figure 6B).
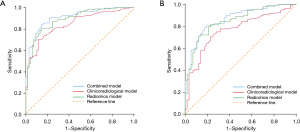
Discussion
The 2011 International Association for the Study of Lung Cancer/American Thoracic Society/European Respiratory Society International Multidisciplinary Lung Adenocarcinoma Classification classified lung ADC into three categories: preinvasive lesions, MIA, and IAC (25). Preinvasive lesions include atypical adenomatous hyperplasia and adenocarcinoma in situ, the later was excluded from the 2021 WHO ADC classification (9). The therapeutic efficacy of limited surgery has been demonstrated to be substantial, particularly in patients with MIA, as it allows for the preservation of a greater amount of functional lung tissue and results in lower perioperative mortality rates compared to standard lobectomy (26,27). However, it is important to note that limited surgery in patients with IAC may carry a certain risk of distant metastasis and local recurrence, which can ultimately decrease the overall survival rate. It is recommended to consider sublobar resection for peripheral nodules measuring ≤2 cm with ≥50% GGO appearance on CT or a long doubling time (400 days) during the resection process by the National Comprehensive Cancer Network guidelines (28). Therefore, our study aimed to assess the invasiveness of GGO predominant ADC with clinical stage Ia in order to identify patients with MIA, who would benefit from limited surgery.
We observed a higher proportion of female patients compared to male patients (403/169), particularly in the MIA group. Additionally, the proportion of smokers was lower in the MIA group compared to the IAC group. This discrepancy may be attributed to the higher likelihood of GGO predominant lung ADC occurring in women, while solid lesions on CT scans, such as squamous cell carcinoma, is more common in men. In recent years, the prevalence of ADC in women has been steadily increasing, attributed to factors such as indoor air pollution and exposure to second-hand smoke. With a higher participation rate among women, the implementation of low-dose CT screening has facilitated the early detection and surgical removal of lung cancer, particularly MIA (29). However, these clinical characteristics were not identified as independent predictors in the multivariate regression analysis.
Based on previous reports, CT characteristics including the primary tumor size and proportion of solid component, were found to be autonomous indicators of ADC invasiveness (30,31). Our findings revealed that the maximum consolidation diameter, rather than the overall tumor size, was an independent prognostic factor. The Union for International Cancer Control recommends measuring the diameter of the invasive component as a criterion for T staging, as it demonstrates greater predictive value for prognosis (32,33). It is commonly held that GGO predominant pulmonary nodules in CT scans are associated with a lower likelihood of invasive pathological outcomes (34). The well-established correlation between solid components in ADC and invasive pathological components is widely recognized (35). As expected, the proportion of IAC in pure GGN of our study was significantly lower compared to part-solid nodule (51.8%/84.5%). Despite 48.2% (95 out of 197 cases) of pure GGN being confirmed as MIA through postoperative pathology, the existence of IAC should not be disregarded.
Our univariate analysis indicated that pleural retraction was associated with IAC, which is consistent with the results of Fan et al. (36). However, there is ongoing debate regarding whether this peritumoral feature is indicative of malignancy. Pleural retraction is thought to be caused by elastosis, inflammation, and fibrous proliferation, suggesting that it may simply reflect pleural fiber tension (37). Additionally, our study revealed that lobulation, air bronchogram, and irregular contour were strongly correlated with ADC invasiveness, although not identified as independent predictors, similar with previous studies (31,38,39). We observed that nine CT morphological characteristics were linked to the invasiveness of ADC. Nevertheless, the amalgamation of clinical and semantic CT features exhibited only moderate effectiveness (AUC =0.842). This finding suggested a noteworthy overlap in the CT morphological features between MIA and IAC, as previously reported by Sakurai et al. (40). Consequently, we endeavored to investigate the potential of radiomics features in order to construct a more proficient model.
Radiomics is the process of converting radiographic images into quantifiable information, which helps facilitating the development of predictive models and potentially enhancing diagnostic precision (41). Recently, a number of studies have been undertaken to employ radiomics for the purpose of characterizing lung nodules, and these studies have yielded promising results in terms of distinguishing between IAC and preinvasive lesions (19,31). In this study, we identified four radiomics features that were deemed optimal for our study. The “square_glrlm_LongRunEmphasis” feature quantifies the distribution of long run lengths, where higher values signify longer run lengths and coarser structural textures. The “dependence entropy” feature, derived from the GLDM, signifies the correlation between the gray-level intensity of CT voxels. A higher value of this feature indicates greater heterogeneity in the texture patterns, aligning with previous research findings (42). Heterogeneity, a well-established malignant characteristic of tumors, encompasses localized variations in tumor proliferation, metabolic activity, cell apoptosis, and blood supply (43). The “glcm_MCC” serves as a feature that captures the velocity and magnitude of alterations in pixel grayscale levels, thereby elucidating the internal attributes and spatial heterogeneity of tumors (44). An increasing body of research has demonstrated the potential significance of this feature in delineating pathological invasiveness and lesion composition (45). The “ngtdm_Strength” feature pertains to second-order features, primarily characterizing the interrelationships among various voxels and elucidating the heterogeneity of signal intensity within the lesion. In a previous study (46), a combined prediction model incorporating lesion shape and radiomics features was developed to differentiate MIA from IAC, achieving an AUC of 0.888. In this study, we developed a composite nomogram model by integrating radiomics quantitative characteristics and CT semantic features. The model demonstrated a favorable AUC value of 0.911, indicating its efficacy in recognition and calibration.
However, there are certain limitations in this study. Firstly, it was a retrospective study that solely focused on GGO predominant ADC with clinical stage Ia, excluding atypical adenomatous hyperplasia and carcinoma in situ, which will undoubtedly limit its application scope. It is worth noting that the manual segmentation method employed in this study proved to be time-consuming, furthermore, the segmentation variability was not evaluated. The extraction of radiomics features is undeniably influenced by various factors, including inconsistent calculation software packages and CT scanning parameters. These factors inevitably reduce the robustness of the model and have an impact on the results of auxiliary diagnosis. Additionally, it is important to note that the data collection process is retrospective, and further confirmation of our nomogram model’s performance requires a larger prospective longitudinal queue.
Conclusions
In conclusion, this study has provided a non-invasive prediction tool based on radiomics and CT semantic characteristics that can accurately assess the quantitative risk associated with the invasiveness of GGO predominant ADC in clinical stage Ia.
Acknowledgments
Funding: This study was supported by
Footnote
Reporting Checklist: The authors have completed the TRIPOD reporting checklist. Available at https://jtd.amegroups.com/article/view/10.21037/jtd-24-775/rc
Data Sharing Statement: Available at https://jtd.amegroups.com/article/view/10.21037/jtd-24-775/dss
Peer Review File: Available at https://jtd.amegroups.com/article/view/10.21037/jtd-24-775/prf
Conflicts of Interest: All authors have completed the ICMJE uniform disclosure form (available at https://jtd.amegroups.com/article/view/10.21037/jtd-24-775/coif). The authors have no conflicts of interest to declare.
Ethical Statement: The authors are accountable for all aspects of the work in ensuring that questions related to the accuracy or integrity of any part of the work are appropriately investigated and resolved. The study was conducted in accordance with the Declaration of Helsinki (as revised in 2013). The study was approved by the Medical Research Ethics Committee and the Institutional Review Board of Tianjin Medical University General Hospital (No. IRB2024-KY-240) and Tianjin Medical University Cancer Institute and Hospital (No. Ek2021067). Individual consent for this retrospective analysis was waived.
Open Access Statement: This is an Open Access article distributed in accordance with the Creative Commons Attribution-NonCommercial-NoDerivs 4.0 International License (CC BY-NC-ND 4.0), which permits the non-commercial replication and distribution of the article with the strict proviso that no changes or edits are made and the original work is properly cited (including links to both the formal publication through the relevant DOI and the license). See: https://creativecommons.org/licenses/by-nc-nd/4.0/.
References
- Siegel RL, Giaquinto AN, Jemal A. Cancer statistics, 2024. CA Cancer J Clin 2024;74:12-49. [Crossref] [PubMed]
- Miller KD, Nogueira L, Devasia T, et al. Cancer treatment and survivorship statistics, 2022. CA Cancer J Clin 2022;72:409-36. [Crossref] [PubMed]
- Tang Z, Ge W, Zhou D, et al. Impact of the Size and Depth of Pulmonary Nodules on the Surgical Approach for Lung Resection in the Treatment of Early-stage Lung Cancer ≤2 cm. Zhongguo Fei Ai Za Zhi 2024;27:170-8. [Crossref] [PubMed]
- Casal-Mouriño A, Valdés L, Barros-Dios JM, et al. Lung cancer survival among never smokers. Cancer Lett 2019;451:142-9. [Crossref] [PubMed]
- Yanagawa N, Shiono S, Abiko M, et al. New IASLC/ATS/ERS classification and invasive tumor size are predictive of disease recurrence in stage I lung adenocarcinoma. J Thorac Oncol 2013;8:612-8. [Crossref] [PubMed]
- Eguchi T, Kondo R, Kawakami S, et al. Computed tomography attenuation predicts the growth of pure ground-glass nodules. Lung Cancer 2014;84:242-7. [Crossref] [PubMed]
- Woo W, Kang DY, Cha YJ, et al. Histopathologic fate of resected pulmonary pure ground glass nodule: a systematic review and meta-analysis. J Thorac Dis 2024;16:924-34. [Crossref] [PubMed]
- Amin MB, Greene FL, Edge SB, et al. The Eighth Edition AJCC Cancer Staging Manual: Continuing to build a bridge from a population-based to a more "personalized" approach to cancer staging. CA Cancer J Clin 2017;67:93-9.
- Nicholson AG, Tsao MS, Beasley MB, et al. The 2021 WHO Classification of Lung Tumors: Impact of Advances Since 2015. J Thorac Oncol 2022;17:362-87. [Crossref] [PubMed]
- Austin JH, Müller NL, Friedman PJ, et al. Glossary of terms for CT of the lungs: recommendations of the Nomenclature Committee of the Fleischner Society. Radiology 1996;200:327-31. [Crossref] [PubMed]
- Chen M, Ding L, Deng S, et al. Differentiating the Invasiveness of Lung Adenocarcinoma Manifesting as Ground Glass Nodules: Combination of Dual-energy CT Parameters and Quantitative-semantic Features. Acad Radiol 2024;31:2962-72. [Crossref] [PubMed]
- Liu L, Ni Z, Zhang J, et al. Application of artificial intelligence-based dual source CT scanning in the differentiation of lung adenocarcinoma in situ and minimally invasive adenocarcinoma. Pak J Med Sci 2024;40:271-6. [Crossref] [PubMed]
- Liu M, Duan R, Xu Z, et al. CT-based radiomics combined with clinical features for invasiveness prediction and pathological subtypes classification of subsolid pulmonary nodules. Eur J Radiol Open 2024;13:100584. [Crossref] [PubMed]
- Fan L, Liu SY, Li QC, et al. Multidetector CT features of pulmonary focal ground-glass opacity: differences between benign and malignant. Br J Radiol 2012;85:897-904. [Crossref] [PubMed]
- Heidinger BH, Anderson KR, Nemec U, et al. Lung Adenocarcinoma Manifesting as Pure Ground-Glass Nodules: Correlating CT Size, Volume, Density, and Roundness with Histopathologic Invasion and Size. J Thorac Oncol 2017;12:1288-98. [Crossref] [PubMed]
- Russo L, Charles-Davies D, Bottazzi S, et al. Radiomics for clinical decision support in radiation oncology. Clin Oncol (R Coll Radiol) 2024;36:e269-81. [Crossref] [PubMed]
- Guan Y, Zhang D, Zhou X, et al. Comparison of deep-learning and radiomics-based machine-learning methods for the identification of chronic obstructive pulmonary disease on low-dose computed tomography images. Quant Imaging Med Surg 2024;14:2485-98. [Crossref] [PubMed]
- Xue T, Zhu L, Tao Y, et al. Development and validation of an interpretable delta radiomics-based model for predicting invasive ground-glass nodules in lung adenocarcinoma: a retrospective cohort study. Quant Imaging Med Surg 2024;14:4086-97.
- Yu Z, Xu C, Zhang Y, et al. A triple-classification for the evaluation of lung nodules manifesting as pure ground-glass sign: a CT-based radiomic analysis. BMC Med Imaging 2022;22:133. [Crossref] [PubMed]
- Wang X, Li Q, Cai J, et al. Predicting the invasiveness of lung adenocarcinomas appearing as ground-glass nodule on CT scan using multi-task learning and deep radiomics. Transl Lung Cancer Res 2020;9:1397-406. [Crossref] [PubMed]
- She Y, Zhang L, Zhu H, et al. The predictive value of CT-based radiomics in differentiating indolent from invasive lung adenocarcinoma in patients with pulmonary nodules. Eur Radiol 2018;28:5121-8. [Crossref] [PubMed]
- Travis WD, Brambilla E, Nicholson AG, et al. The 2015 World Health Organization Classification of Lung Tumors: Impact of Genetic, Clinical and Radiologic Advances Since the 2004 Classification. J Thorac Oncol 2015;10:1243-60. [Crossref] [PubMed]
- Rami-Porta R, Bolejack V, Crowley J, et al. The IASLC Lung Cancer Staging Project: Proposals for the Revisions of the T Descriptors in the Forthcoming Eighth Edition of the TNM Classification for Lung Cancer. J Thorac Oncol 2015;10:990-1003.
- van Griethuysen JJM, Fedorov A, Parmar C, et al. Computational Radiomics System to Decode the Radiographic Phenotype. Cancer Res 2017;77:e104-7. [Crossref] [PubMed]
- Travis WD, Brambilla E, Noguchi M, et al. International association for the study of lung cancer/american thoracic society/european respiratory society international multidisciplinary classification of lung adenocarcinoma. J Thorac Oncol 2011;6:244-85. [Crossref] [PubMed]
- Cao J, Yuan P, Wang Y, et al. Survival Rates After Lobectomy, Segmentectomy, and Wedge Resection for Non-Small Cell Lung Cancer. Ann Thorac Surg 2018;105:1483-91. [Crossref] [PubMed]
- Miura K, Ide S, Minamisawa M, et al. Sublobar resection or lobectomy and postoperative respiratory complications in emphysematous lungs. Eur J Cardiothorac Surg 2024;65:ezae061. [Crossref] [PubMed]
- Ettinger DS, Wood DE, Aisner DL, et al. NCCN Guidelines Insights: Non-Small Cell Lung Cancer, Version 2.2021. J Natl Compr Canc Netw 2021;19:254-66. [Crossref] [PubMed]
- National Lung Screening Trial Research Team. Reduced lung-cancer mortality with low-dose computed tomographic screening. N Engl J Med 2011;365:395-409. [Crossref] [PubMed]
- Sun Y, Li C, Jin L, et al. Radiomics for lung adenocarcinoma manifesting as pure ground-glass nodules: invasive prediction. Eur Radiol 2020;30:3650-9. [Crossref] [PubMed]
- Zhu M, Yang Z, Wang M, et al. A computerized tomography-based radiomic model for assessing the invasiveness of lung adenocarcinoma manifesting as ground-glass opacity nodules. Respir Res 2022;23:96. [Crossref] [PubMed]
- Travis WD, Asamura H, Bankier AA, et al. The IASLC Lung Cancer Staging Project: Proposals for Coding T Categories for Subsolid Nodules and Assessment of Tumor Size in Part-Solid Tumors in the Forthcoming Eighth Edition of the TNM Classification of Lung Cancer. J Thorac Oncol 2016;11:1204-23.
- Rami-Porta R, Asamura H, Travis WD, et al. Lung cancer - major changes in the American Joint Committee on Cancer eighth edition cancer staging manual. CA Cancer J Clin 2017;67:138-55.
- Sun JD, Sugarbaker E, Byrne SC, et al. Clinical Outcomes of Resected Pure Ground-Glass, Heterogeneous Ground-Glass, and Part-Solid Pulmonary Nodules. AJR Am J Roentgenol 2024;222:e2330504. [Crossref] [PubMed]
- Lee KH, Goo JM, Park SJ, et al. Correlation between the size of the solid component on thin-section CT and the invasive component on pathology in small lung adenocarcinomas manifesting as ground-glass nodules. J Thorac Oncol 2014;9:74-82. [Crossref] [PubMed]
- Fan L, Fang M, Li Z, et al. Radiomics signature: a biomarker for the preoperative discrimination of lung invasive adenocarcinoma manifesting as a ground-glass nodule. Eur Radiol 2019;29:889-97. [Crossref] [PubMed]
- Gallagher B, Urbanski SJ. The significance of pleural elastica invasion by lung carcinomas. Hum Pathol 1990;21:512-7. [Crossref] [PubMed]
- Chen X, Feng B, Chen Y, et al. Whole-Lesion Computed Tomography-Based Entropy Parameters for the Differentiation of Minimally Invasive and Invasive Adenocarcinomas Appearing as Pulmonary Subsolid Nodules. J Comput Assist Tomogr 2019;43:817-24. [Crossref] [PubMed]
- Yang W, Sun Y, Fang W, et al. High-resolution Computed Tomography Features Distinguishing Benign and Malignant Lesions Manifesting as Persistent Solitary Subsolid Nodules. Clin Lung Cancer 2018;19:e75-83. [Crossref] [PubMed]
- Sakurai H, Nakagawa K, Watanabe S, et al. Clinicopathologic features of resected subcentimeter lung cancer. Ann Thorac Surg 2015;99:1731-8. [Crossref] [PubMed]
- Gillies RJ, Kinahan PE, Hricak H. Radiomics: Images Are More than Pictures, They Are Data. Radiology 2016;278:563-77. [Crossref] [PubMed]
- Shi L, Shi W, Peng X, et al. Development and Validation a Nomogram Incorporating CT Radiomics Signatures and Radiological Features for Differentiating Invasive Adenocarcinoma From Adenocarcinoma In Situ and Minimally Invasive Adenocarcinoma Presenting as Ground-Glass Nodules Measuring 5-10mm in Diameter. Front Oncol 2021;11:618677. [Crossref] [PubMed]
- Li X, Gao Z, Diao H, et al. Lung adenocarcinoma: selection of surgical approaches in solid adenocarcinoma from the viewpoint of clinicopathologic features and tumor microenvironmental heterogeneity. Front Oncol 2024;14:1326626. [Crossref] [PubMed]
- Haralick RM, Sternberg SR, Zhuang X. Image analysis using mathematical morphology. IEEE Trans Pattern Anal Mach Intell 1987;9:532-50. [Crossref] [PubMed]
- Pang H, Yu Z, Li R, et al. MRI-Based Radiomics of Basal Nuclei in Differentiating Idiopathic Parkinson's Disease From Parkinsonian Variants of Multiple System Atrophy: A Susceptibility-Weighted Imaging Study. Front Aging Neurosci 2020;12:587250. [Crossref] [PubMed]
- Weng Q, Zhou L, Wang H, et al. A radiomics model for determining the invasiveness of solitary pulmonary nodules that manifest as part-solid nodules. Clin Radiol 2019;74:933-43. [Crossref] [PubMed]