Association between patient medications and postoperative outcomes in early-stage non-small cell lung cancer
Highlight box
Key findings
• In a retrospective analysis of 9,741 United States veterans with clinical stage I non-small cell lung cancer (NSCLC), a higher number of prescription medications was associated with increased risk of postoperative adverse events and reduced overall survival, even when adjusting for several covariates including age and comorbidity burden.
• We observed an additional 5.7% and 3.5% risk of postoperative adverse events at 90 days with each additional prescription medication acting primarily on the respiratory and nervous systems, respectively.
What is known and what is new?
• It is well-recognized that an accurate comorbidity assessment is essential in patients with early-stage NSCLC to guide candidacy for surgery versus stereotactic body radiation therapy and predict prognosis. However, there is no consensus on how to comprehensively assess comorbidities in patients with NSCLC using real-time data.
• Our analysis newly demonstrates that detailed, patient-level medication assessments can inform risk of adverse short- and long-term outcomes after curative-intent resection of early-stage NSCLC. It appears that patients prescribed a higher number of medications acting primarily on the respiratory and nervous systems are at elevated risk for postoperative adverse events.
What is the implication, and what should change now?
• Based on these findings, prescription medications may be a potential tool to assess comorbidities and their associated perioperative risks for patients with early-stage NSCLC.
• Medication-based risk prediction models could enhance precision lung cancer care by helping to inform prognosis, guide treatment selection, and mitigate adverse events.
Introduction
Lung cancer is the leading cause of cancer-related mortality in the United States (US) (1). It is estimated that 238,340 new cases and 127,070 lung cancer-related deaths occurred nationwide in 2023 (1). The annual national cost of lung cancer care has been estimated to exceed $20 billion and is projected to increase further over the next decade (2). Hence, evidence-based management of lung cancer should be a national priority for optimization of outcomes and resource utilization.
Chronic health conditions are common in older adults, especially in those with lung cancer, and are expected to increase in prevalence with the aging population (3,4). It has previously been shown that lung cancer survivors have the highest comorbidity burden compared to those with other cancers, with greater than 50% of survivors experiencing severe comorbidity burden (4). Compared to the civilian population, US veterans have higher rates of chronic diseases including diabetes, cardiovascular disease, chronic obstructive pulmonary disease (COPD), and cancer, which may be attributable to unique service-related risk factors such as Agent Orange exposure and high rates of tobacco use (5-10). In particular, approximately 8,000 new lung cancers are diagnosed annually in the Veterans Health Administration (VHA) (11).
An accurate comorbidity assessment is essential in patients with early-stage non-small cell lung cancer (NSCLC) to guide candidacy for surgery versus stereotactic body radiation therapy, inform of potential treatment-related risks and adverse events, and predict prognosis. Furthermore, comorbidities, such as COPD and cardiovascular disease, have been shown to influence long-term survival in lung cancer (12-15). Despite the importance of accurate comorbidity assessments, there is no consensus on how to comprehensively assess comorbidities in patients with NSCLC using real-time data. Established comorbidity indices, such as the Charlson Comorbidity Index (CCI) and the Elixhauser Comorbidity Index (ECI), often have limited applicability in clinical settings due to frequently incomplete documentation of comorbidities in modern electronic health records (EHRs) (16-18). These comorbidity indices rely upon administrative codes used for hospital billing, lack specificity, may not be appropriately weighted, and are calculated retrospectively. Furthermore, administrative codes may not reflect disease severity, are infrequently updated, can be discordant between clinicians, and are influenced by hospital billing practices. Hence, there is an unmet need for reliable and real-time comorbidity assessment tools that can accurately assess comorbidity burden and predict outcomes for early-stage NSCLC.
Prescription medications are readily available in EHRs and may serve as a more accurate and up-to-date comorbidity measure for predicting surgical outcomes for NSCLC. Multiple prior studies have demonstrated that perioperative use of bronchodilators can influence postoperative outcomes in lung cancer patients with COPD (19-23). Hence, we hypothesized that both the number and type of prescription medications are associated with postoperative outcomes in patients undergoing definitive resection for NSCLC. We tested this hypothesis in a comprehensive, unique cohort of US veterans diagnosed with early-stage NSCLC. We present this article in accordance with the STROBE reporting checklist (available at https://jtd.amegroups.com/article/view/10.21037/jtd-24-803/rc).
Methods
We conducted a retrospective cohort study of patients diagnosed with clinical stage I NSCLC who underwent curative-intent resection in the VHA between 10/01/2006 and 09/30/2016. Data elements were retrieved using the VHA Informatics and Computing Infrastructure (VINCI) system, which contains clinical and administrative data from multiple national data repositories in the Corporate Data Warehouse (CDW), including CDW-Oncology Raw and Veterans Affairs Surgical Quality Improvement Program (VASQIP) (24). To ensure maximal data capture, this unique dataset was compiled by a team of dedicated abstractors across 24 months using a combination of manual chart reviews and natural language processing techniques. We identified patients with a diagnosis of NSCLC through query of International Classification of Diseases (ICD) for Oncology, third edition codes, and confirmed procedures using ICD-9, ICD-10, or Current Procedural Terminology (CPT) codes. We included adults (age 18 or older) who were diagnosed with clinical stage I (tumors ≤5 cm, node-negative disease) disease, as defined by the American Joint Committee on Cancer (7th edition), and underwent curative-intent surgical resection. Patients who had a missing diagnosis date, received neoadjuvant treatment, or presented with a recurrent cancer were excluded from this study.
The study was conducted in accordance with the Declaration of Helsinki (as revised in 2013). The study was approved by the St. Louis VHA’s Research and Development Committee (#1214632), and individual consent for this retrospective analysis was waived.
As previously described by our group and other authors, medication data was obtained for all patients using the CDW Pharmacy Outpatient database, which contains detailed prescription medication information including date of issue, date of fill, number of refills prescribed, administration route, dose prescribed, and administration frequency (19,25). Data from all outpatient prescriptions filled by patients within the VHA system from one-year up to fourteen days prior to surgery were collected. This timeframe was selected as medications prescribed greater than one year before surgery were considered to less accurately reflect a patient’s overall health status at the time of surgery. We categorized prescription medications using the World Health Organization Anatomical Therapeutic Chemical (ATC) Level One classification system, which is considered the gold standard for drug utilization monitoring and research (26). In the ATC classification system, medications are divided into groups at five different levels according to the organ or body system they effect as well as their therapeutic, pharmacological, and chemical properties. The Level One classification system is the broadest level and consists of fourteen anatomical or pharmacological groups (Table 1). Two authors (S.T., C.P.) independently categorized medications into ATC Level One classes. A co-senior author (M.W.S.) then manually reviewed both compiled lists and adjudicated decisions regarding medication classification in discrepant cases.
Table 1
ATC Level One classification | Example medications |
---|---|
A: alimentary tract and metabolism | Ondansetron, omeprazole, metformin |
B: blood and blood forming organs | Warfarin, apixaban, clopidogrel |
C: cardiovascular system | Metoprolol, furosemide, hydralazine |
D: dermatologics | Griseofulvin, benzoyl peroxide |
G: genitourinary system and sex hormones | Testosterone, finasteride, sildenafil |
H: systemic hormonal preparations | Levothyroxine, fludrocortisone |
J: anti-infective for systemic use | Doxycycline, amoxicillin |
L: antineoplastic and immunomodulating agents | Tacrolimus, infliximab, doxorubicin |
M: musculoskeletal system | Methocarbamol, baclofen |
N: nervous system | Oxycodone, levodopa, haloperidol |
P: antiparasitic products, insecticides, and repellents | Hydroxychloroquine, praziquantel |
R: respiratory system | Salmeterol, fluticasone, tiotropium |
S: sensory organs | Pilocarpine, latanoprost |
V: various | Sevelamer, mesna, deferoxamine |
Table contents were obtained using publicly available information from the World Health Organization (https://www.whocc.no/atc_ddd_index/) (26). ATC, anatomical therapeutic chemical.
Several patient-, tumor-, and treatment-related covariates were abstracted from the CDW system. Comorbidities were measured using the composite Charlson-Deyo Comorbidity Index, which was calculated using ICD-9 and ICD-10 codes present from 5 years prior to 1 month after surgery (27). We extracted oncologic and treatment-related covariates for each patient, which included tumor size, grade, histology, year of operation, hospital case load (defined as the volume of lung cancer cases treated at a specific facility in the year prior to surgery), surgical approach, operation type (lobectomy, segmentectomy, wedge resection, or pneumonectomy), lymph node assessment, and pathologic stage.
Statistical analysis
The primary outcome of the study was postoperative adverse events at 30 and 90 days following surgery. This composite endpoint was generated as a binary variable (yes/no) consisting of perioperative mortality and/or the presence of major postoperative complications (defined as a diagnosis of a pneumonia, empyema, myocardial infarction, respiratory failure, renal failure, or stroke within 30 days after surgery). Postoperative complications were identified using the VASQIP database, a reliable and validated method for detection of postoperative adverse events, as well as ICD-9 and ICD-10 diagnosis codes (19,28,29). Multivariable logistic regression models adjusting for patient sociodemographic, comorbidity, tumor, and operative characteristics were used to assess the relationship between the number of prescription medications, ATC Level One medication classes, and postoperative adverse events. Our secondary outcome was overall survival (OS), defined as the time between date of surgery and all-cause mortality. Date of death was determined from the VHA Vital Status Files. OS was censored at the completion of the study’s follow-up period (05/01/2020). OS was presented using the Kaplan-Meier method and assessed using multivariable Cox proportional hazards model.
Model performance with and without inclusion of patient comorbidities was evaluated using the Akaike information criterion (AIC) statistic and c-statistic. The AIC is an estimator of prediction error and the relative quality of statistical models for a given dataset. The c-statistic represents the area under the receiver operator characteristic curve and assesses model discrimination. Model performance for the outcome of 30-day postoperative adverse events with and without comorbidities were as follows: c-statistic with comorbidities is 0.672, and c-statistic without comorbidities is 0.654. The AIC with comorbidities is 7,242 and without comorbidities is 7,327. As shown in Table S1, this trend was also observed when examining model performance for the outcomes of 90-day postoperative adverse events and OS. Both parameters suggested better discrimination and model fit with the inclusion of patient comorbidities for the primary and secondary outcomes.
Descriptive statistics were reported as means with standard deviations (SD) for continuous variables and as frequencies with proportions for categorical variables. Missing data points were minimal and categorized as unknown. All statistical tests were two-sided, with P values less than 0.05 deemed statistically significant. Analyses were conducted in SAS version 9.3 (SAS Institute Inc., Cary, NC, USA).
Results
In total, 9,741 veterans met inclusion criteria and were included in the analysis. The median age of the study cohort was 67.0 [interquartile range (IQR): 62.3–73.0] years. Most veterans were male (n=9,383, 96.3%), of White race (n=8,060, 82.7%), were smoking at the time of surgery (n=5,697, 58.5%), and underwent lobectomy (n=6,907, 70.9%). The mean Charlson-Deyo Comorbidity Index score was 6.89 (SD: 2.22). Detailed characteristics of the study cohort are shown in Table 2 (19). Major postoperative complications occurred in 1,351 (13.9%) veterans and occurred most frequently following lobectomy (n=1,047/6,907, 15.2%). The rate of major postoperative complications stratified by operation type is detailed in Table S2. The rate of postoperative mortality at 30 days was 2.2% (n=196). Deaths occurred in 5,760 (59.1%) veterans over a median follow-up of 6.1 (IQR: 2.5–11.4) years.
Table 2
Patient characteristics | Study cohort, No. (%, n=9,741) |
---|---|
Age (years), mean (SD) | 67.61 (7.89) |
Sex, n (%) | |
Female | 358 (3.68) |
Male | 9,383 (96.32) |
Race, n (%) | |
Black | 1,457 (14.96) |
Other | 131 (1.34) |
White | 8,060 (82.74) |
Unknown | 93 (0.95) |
Body mass index (kg/m2), n (%) | |
<18.5 | 307 (3.19) |
18.5–24.9 | 3,276 (34.04) |
25–29.9 | 3,464 (36.03) |
30–34.9 | 1,833 (19.07) |
≥35 | 734 (7.63) |
Smoking status (at time of surgery), n (%) | |
Current | 5,697 (58.48) |
Former | 3,912 (40.16) |
Never | 132 (1.36) |
Charlson-Deyo Comorbidity Index, median (IQR) | 6.89 (2.22) |
Distance from hospital (m), n (%) | |
≤10 | 2,131 (21.88) |
11–50 | 3,929 (40.33) |
>50 | 3,681 (37.79) |
Area deprivation index, n (%) | |
Quartile 1 (least deprived) | 2,357 (24.29) |
Quartile 2 | 2,459 (25.34) |
Quartile 3 | 2,523 (26.00) |
Quartile 4 (most deprived) | 2,365 (24.37) |
Histology, n (%) | |
Adenocarcinoma | 5,192 (53.30) |
Squamous cell carcinoma | 3,292 (33.80) |
Other | 1,257 (12.90) |
Grade, n (%) | |
I | 1,218 (13.28) |
II | 4,827 (52.64) |
III | 2,991 (32.62) |
IV | 133 (1.45) |
Tumor size (mm), n (%) | |
≤10 | 890 (9.14) |
11–20 | 3,922 (40.26) |
21–30 | 2,693 (27.65) |
31–40 | 1,501 (15.41) |
41–50 | 729 (7.48) |
Delayed operation (>12 weeks), n (%) | 3,045 (31.26) |
Surgical approach, n (%) | |
Thoracotomy | 5,686 (58.53) |
Minimally invasive | 4,028 (41.47) |
Resection type, n (%) | |
Lobectomy | 6,907 (70.91) |
Pneumonectomy | 155 (1.59) |
Segmentectomy | 540 (5.54) |
Wedge resection | 2,139 (21.96) |
Lymph node sampling, n (%) | |
<3 N2 and/or <1 N1 | 7,184 (73.75) |
≥3 N2 and ≥1 N1 | 2,557 (26.25) |
Surgical margin, n (%) | |
R0 | 9,321 (96.71) |
R1/R2 | 317 (3.29) |
Pathologic upstage, n (%) | |
No | 8,487 (87.13) |
Yes | 1,254 (12.87) |
Table adapted with permission from AME Publishing Company (19). SD, standard deviation; IQR, interquartile range.
The median number of prescription medications filled in the year prior to surgery was 11 (IQR: 7–16). A median of 5 (IQR: 4–7) unique ATC Level One classes were represented among these prescription medications. As detailed in Table 3, 8,050 (82.6%), 7,468 (76.7%), and 6,289 (64.6%) veterans were prescribed at least one medication from the ATC cardiovascular, nervous, and respiratory system classes, respectively. In a multivariable linear model, a higher number of prescription medications was associated with younger age, higher Charlson-Deyo Comorbidity Index score, increased body mass index, female sex, black race, prior tobacco use, living closer to the treatment facility, high area deprivation index, squamous cell carcinoma, and sublobar resection (all P<0.05).
Table 3
Anatomical Therapeutic Chemical Level One Class | Total number of patients prescribed at least one medication (%, n=9,741) | Mean number of prescription medications per patient (standard deviation) |
---|---|---|
A: alimentary tract and metabolism | 6,254 (64.2) | 1.17 (1.2) |
B: blood and blood forming organs | 3,160 (32.4) | 0.43 (0.7) |
C: cardiovascular system | 8,050 (82.6) | 2.56 (2.0) |
D: dermatologics | 3,359 (34.5) | 0.59 (1.0) |
G: genitourinary system and sex hormones | 3,861 (39.6) | 0.57 (0.8) |
H: systemic hormonal preparations | 2,281 (23.4) | 0.27 (0.5) |
J: anti-infective for systemic use | 4,899 (50.3) | 1.02 (1.4) |
L: antineoplastic and immunomodulating agents | 574 (5.9) | 0.07 (0.3) |
M: musculoskeletal system | 3,698 (38.0) | 0.53 (0.8) |
N: nervous system | 7,468 (76.7) | 2.50 (2.3) |
P: antiparasitic products, insecticides, and repellents | 101 (1.0) | 0.01 (0.1) |
R: respiratory system | 6,289 (64.6) | 2.02 (2.2) |
S: sensory organs | 999 (10.3) | 0.17 (0.6) |
V: various | 1,669 (17.1) | 0.17 (0.4) |
In multivariable analyses, a higher number of prescription medications was associated with increased risk of postoperative adverse events at 30 days [multivariable-adjusted odds ratio (aOR), 1.016; 95% confidence interval (CI): 1.007–1.026] and 90 days (aOR: 1.015; 95% CI: 1.006–1.024). A higher number of prescription medications was also associated with increased risk of mortality [multivariable-adjusted hazard ratio (aHR), 1.019; 95% CI: 1.014–1.023]. Within a subgroup of patients with a high comorbidity burden (Charlson-Deyo Comorbidity Index score of 6–8), a higher number of prescription medications was also associated with increased risk of mortality (P<0.001; Figure 1).
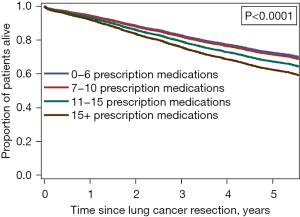
Inclusion of each ATC Level One medication class as a covariate (yes/no) in the multivariable regression model revealed that veterans prescribed medications from the ATC respiratory system class had increased odds of 30-day (aOR: 1.255; 95% CI: 1.095–1.439; Figure 2A) and 90-day (aOR: 1.254; 95% CI: 1.097–1.434; Figure 2B) postoperative adverse events compared to patients not prescribed these medications. Veterans prescribed medications from the ATC anti-infective for systemic use class had decreased odds of 30-day (aOR: 0.856; 95% CI: 0.753–0.972) and 90-day (aOR: 0.860; 95% CI: 0.759–0.975) postoperative adverse events compared to patients not prescribed these medications. Veterans prescribed medications from the ATC blood and blood forming organs (aHR, 1.084; 95% CI: 1.020–1.152), systemic hormonal preparations (aHR, 1.135; 95% CI: 1.063–1.211), nervous system (aHR, 1.114; 95% CI: 1.040–1.194), and respiratory system (aHR, 1.073; 95% CI: 1.009–1.140) classes had higher risk of mortality in the multivariable-adjusted model (Figure 3).
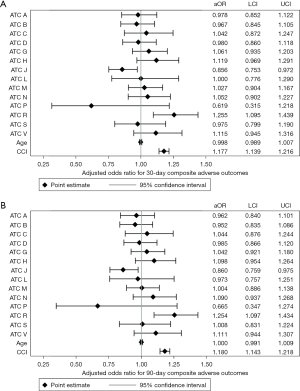
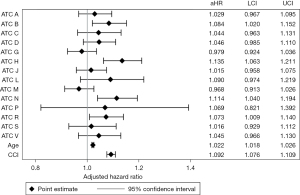
Each additional prescription medication from the ATC respiratory system class was associated with a 6.2% increased risk for postoperative adverse events at 30 days (aOR: 1.062; 95% CI: 1.031–1.093; Table 4). Increased odds for postoperative adverse events at 90 days were observed with each additional prescription medication from the ATC respiratory (aOR: 1.057; 95% CI: 1.027–1.088) and nervous system (aOR: 1.035; 95% CI: 1.005–1.066) classes. Compared to patients not prescribed medications from these drug classes, there was elevated risk for mortality with each additional medication prescribed from the ATC alimentary tract and metabolism (aHR, 1.034; 95% CI: 1.007–1.061), cardiovascular (aHR, 1.026; 95% CI: 1.009–1.042), systemic hormonal preparations (aHR, 1.072; 95% CI: 1.016–1.130), antineoplastic and immunomodulating agents (aHR, 1.108; 95% CI: 1.013–1.212), nervous (aHR, 1.028; 95% CI: 1.014–1.042), and respiratory (aHR, 1.017; 95% CI: 1.004–1.031) system classes.
Table 4
ATC class | 30-day postoperative adverse events | 90-day postoperative adverse events | Overall survival |
---|---|---|---|
A | 0.977 (0.923–1.034) | 0.975 (0.922–1.031) | 1.034 (1.007–1.061)* |
B | 0.993 (0.909–1.085) | 0.984 (0.902–1.073) | 1.027 (0.987–1.069) |
C | 1.014 (0.978–1.050) | 1.012 (0.978–1.048) | 1.026 (1.009–1.042)* |
D | 0.993 (0.933–1.058) | 0.996 (0.936–1.059) | 1.006 (0.979–1.035) |
G | 1.025 (0.951–1.104) | 1.008 (0.937–1.085) | 0.969 (0.936–1.003) |
H | 1.041 (0.924–1.171) | 1.021 (0.908–1.147) | 1.072 (1.016–1.130)* |
J | 0.965 (0.921–1.012) | 0.967 (0.923–1.013) | 1.007 (0.986–1.028) |
L | 1.006 (0.817–1.239) | 1.000 (0.815–1.226) | 1.108 (1.013–1.212)* |
M | 1.009 (0.933–1.092) | 0.998 (0.924–1.078) | 0.979 (0.944–1.016) |
N | 1.029 (0.998–1.060) | 1.035 (1.005–1.066)* | 1.028 (1.014–1.042)* |
P | 0.625 (0.318–1.229) | 0.666 (0.348–1.275) | 1.097 (0.845–1.424) |
R | 1.062 (1.031–1.093)* | 1.057 (1.027–1.088)* | 1.017 (1.004–1.031)* |
S | 0.971 (0.879–1.073) | 0.986 (0.896–1.086) | 1.013 (0.970–1.057) |
V | 1.126 (0.950–1.334) | 1.121 (0.948–1.324) | 1.018 (0.939–1.103) |
Data are reported as adjusted odds ratio or hazard ratio with 95% confidence interval. *, statistical significance. ATC, Anatomical Therapeutic Chemical.
Discussion
The presence of comorbid conditions informs treatment selection and prognosis in veterans with early-stage NSCLC. Reliable comorbidity assessment is a critical component of high-quality lung cancer care. Over the last three decades, several comorbidity indices have been developed for prediction of morbidity and mortality. The commonly cited CCI was first developed as a weighted comorbidity index to predict risk of mortality and has since been adapted by integrating ICD and CPT codes (17,30,31). Similarly, the ECI includes 30 comorbidities and was developed as a comorbidity measure for use in administrative database research (18). While these comorbidity indices have been frequently used in retrospective outcomes research, they are rarely used to make treatment decisions and have not been readily integrated into EHR platforms or patient-centered oncology care models. Established comorbidity indices rely on the accurate collection of ICD and CPT codes, which are primarily used by hospitals for reimbursement and to report diagnoses, procedures, and services rendered. As comorbidities are often underreported in hospital administrative data, this may be an inaccurate method to quantify comorbidity burden in cancer patients (16). Additionally, an updated list of administrative codes is usually unavailable at the time of a patient’s clinic visit as these data elements are coded by abstractors at various times. Hence, there are significant limitations to the use of these comorbidity assessment tools for patients with NSCLC.
Prescription medications may be a reliable, easy-to-use, and real-time comorbidity measurement tool for patients with NSCLC. In contrast to administrative codes, medication lists are readily accessible for each patient in modern EHR platforms and are reconciled with each clinical encounter, providing a real-time reflection of an individual’s current comorbidity status. Additionally, administrative data obtained for coding and reimbursement of clinical encounters does not accurately represent a patient’s true comorbidity burden and has limited ability to determine disease severity or control within a specific diagnosis (Table 5). Review of prescription medications can provide more detailed information regarding comorbidity severity and disease control (e.g., a single prescribed bronchodilator for well-controlled COPD compared to multiple steroid prescriptions for recurrent COPD exacerbations). Furthermore, prescription medications are patient-specific and can highlight the distinct health factors that may influence an individual’s outcomes after treatment for NSCLC.
Table 5
Health problem list | Prescription medications |
---|---|
Hypertension | Aspirin 81 mg PO daily |
Chronic obstructive pulmonary disease | Albuterol 90 mcg/actuation inhaler, inhale 2 puffs every 6 hours PRN |
Non-small cell lung cancer | Diltiazem XR 180 mg PO daily |
Chronic pain | Lisinopril 10 mg PO daily |
Colon cancer screening | Loratadine 10 mg PO daily |
Allergic rhinitis | Metoprolol succinate ER 50 mg PO daily |
Congestive heart failure | Oxycodone 5 mg PO QID PRN |
Encounter for tetanus, diphtheria, pertussis (Tdap) vaccine | Tiotropium bromide 2.5 mcg/actuation inhaler, inhale 2 puffs daily |
History of asthma | |
History of snake bite | |
Diabetes mellitus | |
History of motor vehicle accident | |
Generalized abdominal pain | |
Metacarpal fracture of the hand | |
History of anxiety | |
Cellulitis | |
Vertigo | |
Obesity | |
Hypothyroidism | |
History of seizure |
PO, per os; PRN, as needed; XR/ER, extended-release; QID, four times a day.
Our analysis of a large national cohort of veterans demonstrates that detailed, patient-level medication assessments can inform risk of adverse short- and long-term outcomes after resection of early-stage NSCLC. We found that a higher number of prescription medications was associated with increased risk of postoperative adverse events and reduced OS, even when adjusting for several covariates including age and comorbidity burden. It appears that patients prescribed a higher number of medications acting primarily on the respiratory and nervous systems are at elevated risk for postoperative adverse events. We observed an additional 5.7% and 3.5% risk of postoperative adverse events at 90 days post-surgery with each additional prescription medications from the ATC respiratory and nervous system classes, respectively. These two ATC classes include medications that are commonly prescribed to older adults, such as bronchodilators for COPD, analgesics for chronic pain, and psychotropic medications. In this study, approximately 65% and 77% of patients were prescribed medications from the ATC respiratory and nervous system classes, respectively. These findings build on prior analyses from our group which revealed that the perioperative use of opiates and inhaled COPD medications are associated with increased risk of adverse outcomes after pulmonary resection (19,32).
Based on these findings, prescription medications may be a potential tool to assess comorbidities and their associated perioperative risks for patients with early-stage NSCLC. The potential association between prescription medications and patient outcomes for other cancer types also warrants special attention and should be explored in future studies. Additional research efforts should also target defining the relationship between prescription medications, comorbid disease diagnoses, and cancer treatment allocation. If larger studies validate these findings, a predictive model using prescription medications for perioperative risk stratification could be adopted into clinical practice given the automated integration of medication lists into EHRs for real-time comorbidity assessments. Medication-based risk prediction models could enhance precision lung cancer care by helping to inform prognosis, guide treatment selection, and mitigate adverse events.
As previously described by our group, a limitation of this work is that the number of prescription medications consists only of the outpatient prescriptions that were filled by the patient before surgery and did not include inpatient medications or prescriptions from other healthcare systems outside of the VHA (19). Additionally, we were unable to assess medication adherence as the analysis was completed retrospectively. Since the study population primarily consisted of male US veterans, further research is also needed to determine if these findings apply to the broader population, including women. While we examined the frequencies of several common medical comorbidities and major complications after lung cancer resection, we were limited in our ability to account for all potential patient comorbidities (e.g., nutritional status, anemia) and postoperative complications (e.g., atrial arrhythmias, unexpected return to the operating room) in the analysis. Despite these limitations, we believe that this study has several strengths. Specifically, it presents data from a uniquely compiled, nationally representative dataset which contains a comprehensive list of covariates and minimal missing data elements. Additionally, we believe that VHA pharmacy data is ideally suited for this analysis as it is recognized that veterans have more equitable access to care, compared to patients with other insurance types, and low rates of cost-related medication non-adherence (33). Medications, procedures, and provider visits are available to veterans with often minimal or no copayments (34-36). Therefore, assessment of prescription medications is considered reliable in the VHA population (19,25,37,38).
Conclusions
Collectively, our findings suggest that prescription medications may be a reliable tool to assess comorbidities and perioperative risk for patients with NSCLC. In this study, patients prescribed a higher number of medications acting primarily on the respiratory and nervous systems appear to be at elevated risk for postoperative adverse events after curative-intent resection. Routine assessment of prescription medications prior to elective surgery may help identify lung cancer patients who may benefit from additional medical optimization prior to elective surgical resection. Future development of medication-based risk prediction models could enhance precision lung cancer care by helping to inform prognosis, guide treatment selection, and mitigate adverse events.
Acknowledgments
This work was presented at the e-Poster Session of the 60th Annual Meeting of The Society of Thoracic Surgeons, San Antonio, Texas, January 27–29, 2024.
Funding: This work was supported by
Footnote
Reporting Checklist: The authors have completed the STROBE reporting checklist. Available at https://jtd.amegroups.com/article/view/10.21037/jtd-24-803/rc
Data Sharing Statement: Available at https://jtd.amegroups.com/article/view/10.21037/jtd-24-803/dss
Peer Review File: Available at https://jtd.amegroups.com/article/view/10.21037/jtd-24-803/prf
Conflicts of Interest: All authors have completed the ICMJE uniform disclosure form (available at https://jtd.amegroups.com/article/view/10.21037/jtd-24-803/coif). D.K. serves as an unpaid editorial board member of Journal of Thoracic Disease from April 2024 to June 2026. S.T. was supported by the Washington University School of Medicine (WUSM) Surgical Oncology Basic Science and Translational Research Training Program grant T32CA009621, from the National Cancer Institute. D.B.E. Jr receives support from VHA 1I01HX002475-01A2 grant. N.E.R. was supported in part by the WUSM StARR Program in Cross-Disciplinary Oncology Clinician-Scientist Training R38CA255575. B.T.H. was funded in part by NIH grant 5T32HL007776-25. B.T.H. is a former consultant at Oncocyte Corporation and was an MBA intern (at Eli Lilly and Company). Y.Y. was funded in part through a VHA 1I01HX002475-01A2 grant. D.K. is supported by NIH grants 1P01AI116501, R01HL094601, R01HL151078, U01163086-01, United States, Veterans Administration Merit Review grant 1I01BX002730, United States, and The Foundation for Barnes-Jewish Hospital, United States. S.H.C. was funded in part through a VHA 1I01HX002475-01A2 grant. V.P. has received the following grants for projects: 1I01HX002475-01A2, R01HL146856, R01CA258681, MATF. V.P. has received payments from PrecisCa (panel discussions) and V.P.’s spouse owns stock in Intuitive Surgical. M.W.S. has funding through the Congressionally Directed Medical Research Program DoD W81XWH-22-1-0602 and has received speaking fees from Pfizer. The other authors have no conflicts of interest to declare.
Ethical Statement: The authors are accountable for all aspects of the work in ensuring that questions related to the accuracy or integrity of any part of the work are appropriately investigated and resolved. The study was conducted in accordance with the Declaration of Helsinki (as revised in 2013). The study was approved by the St. Louis VHA’s Research and Development Committee (#1214632), and individual consent for this retrospective analysis was waived.
Open Access Statement: This is an Open Access article distributed in accordance with the Creative Commons Attribution-NonCommercial-NoDerivs 4.0 International License (CC BY-NC-ND 4.0), which permits the non-commercial replication and distribution of the article with the strict proviso that no changes or edits are made and the original work is properly cited (including links to both the formal publication through the relevant DOI and the license). See: https://creativecommons.org/licenses/by-nc-nd/4.0/.
References
- Siegel RL, Miller KD, Wagle NS, et al. Cancer statistics, 2023. CA Cancer J Clin 2023;73:17-48. [Crossref] [PubMed]
- Mariotto AB, Enewold L, Zhao J, et al. Medical Care Costs Associated with Cancer Survivorship in the United States. Cancer Epidemiol Biomarkers Prev 2020;29:1304-12. [Crossref] [PubMed]
- Sarfati D, Koczwara B, Jackson C. The impact of comorbidity on cancer and its treatment. CA Cancer J Clin 2016;66:337-50. [Crossref] [PubMed]
- Bluethmann SM, Mariotto AB, Rowland JH. Anticipating the "Silver Tsunami": Prevalence Trajectories and Comorbidity Burden among Older Cancer Survivors in the United States. Cancer Epidemiol Biomarkers Prev 2016;25:1029-36. [Crossref] [PubMed]
- Agha Z, Lofgren RP, VanRuiswyk JV, et al. Are patients at Veterans Affairs medical centers sicker? A comparative analysis of health status and medical resource use. Arch Intern Med 2000;160:3252-7. [Crossref] [PubMed]
- Betancourt JA, Granados PS, Pacheco GJ, et al. Exploring Health Outcomes for U.S. Veterans Compared to Non-Veterans from 2003 to 2019. Healthcare (Basel) 2021;9:604. [Crossref] [PubMed]
- Eibner C, Krull H, Brown KM, et al. Current and Projected Characteristics and Unique Health Care Needs of the Patient Population Served by the Department of Veterans Affairs. Rand Health Q 2016;5:13. [PubMed]
- Heiden BT, Eaton DB Jr, Chang SH, et al. Comparison Between Veteran and Non-Veteran Populations With Clinical Stage I Non-small Cell Lung Cancer Undergoing Surgery. Ann Surg 2023;277:e664-9. [Crossref] [PubMed]
- Subramanian MP, Eaton DB Jr, Labilles UL, et al. Exposure to Agent Orange is associated with increased recurrence after surgical treatment of stage I non-small cell lung cancer. J Thorac Cardiovasc Surg 2024;167:1591-1600.e2. [Crossref] [PubMed]
- Committee to Review the Health Effects in Vietnam Veterans of Exposure to Herbicides (Ninth Biennial Update); Board on the Health of Select Populations; Institute of Medicine. Veterans and Agent Orange: Update 2012. Washington (DC): National Academies Press (US); 2014.
- Zullig LL, Jackson GL, Dorn RA, et al. Cancer incidence among patients of the U.S. Veterans Affairs Health Care System. Mil Med 2012;177:693-701. [Crossref] [PubMed]
- Morishima T, Matsumoto Y, Koeda N, et al. Impact of Comorbidities on Survival in Gastric, Colorectal, and Lung Cancer Patients. J Epidemiol 2019;29:110-5. [Crossref] [PubMed]
- Kravchenko J, Berry M, Arbeev K, et al. Cardiovascular comorbidities and survival of lung cancer patients: Medicare data based analysis. Lung Cancer 2015;88:85-93. [Crossref] [PubMed]
- Zhai R, Yu X, Shafer A, et al. The impact of coexisting COPD on survival of patients with early-stage non-small cell lung cancer undergoing surgical resection. Chest 2014;145:346-53. [Crossref] [PubMed]
- Hernandez D, Cheng CY, Hernandez-Villafuerte K, et al. Survival and comorbidities in lung cancer patients: Evidence from administrative claims data in Germany. Oncol Res 2022;30:173-85. [Crossref] [PubMed]
- Powell H, Lim LL, Heller RF. Accuracy of administrative data to assess comorbidity in patients with heart disease. an Australian perspective. J Clin Epidemiol 2001;54:687-93. [Crossref] [PubMed]
- Charlson ME, Pompei P, Ales KL, et al. A new method of classifying prognostic comorbidity in longitudinal studies: development and validation. J Chronic Dis 1987;40:373-83. [Crossref] [PubMed]
- Elixhauser A, Steiner C, Harris DR, et al. Comorbidity measures for use with administrative data. Med Care 1998;36:8-27. [Crossref] [PubMed]
- Tohmasi S, Eaton DB Jr, Heiden BT, et al. Inhaled medications for chronic obstructive pulmonary disease predict surgical complications and survival in stage I non-small cell lung cancer. J Thorac Dis 2023;15:6544-54. [Crossref] [PubMed]
- Shin SH, Shin S, Im Y, et al. Effect of perioperative bronchodilator therapy on postoperative pulmonary function among lung cancer patients with COPD. Sci Rep 2021;11:8359. [Crossref] [PubMed]
- Bölükbas S, Eberlein M, Eckhoff J, et al. Short-term effects of inhalative tiotropium/formoterol/budenoside versus tiotropium/formoterol in patients with newly diagnosed chronic obstructive pulmonary disease requiring surgery for lung cancer: a prospective randomized trial. Eur J Cardiothorac Surg 2011;39:995-1000. [Crossref] [PubMed]
- Suzuki H, Sekine Y, Yoshida S, et al. Efficacy of perioperative administration of long-acting bronchodilator on postoperative pulmonary function and quality of life in lung cancer patients with chronic obstructive pulmonary disease. Preliminary results of a randomized control study. Surg Today 2010;40:923-30. [Crossref] [PubMed]
- Azuma Y, Sano A, Sakai T, et al. Prognostic and functional impact of perioperative LAMA/LABA inhaled therapy in patients with lung cancer and chronic obstructive pulmonary disease. BMC Pulm Med 2021;21:174. [Crossref] [PubMed]
- Massarweh NN, Kaji AH, Itani KMF. Practical Guide to Surgical Data Sets: Veterans Affairs Surgical Quality Improvement Program (VASQIP). JAMA Surg 2018;153:768-9. [Crossref] [PubMed]
- Logan CD, Hudnall MT, Schlick CJR, et al. Venous Thromboembolism Chemoprophylaxis Adherence Rates After Major Cancer Surgery. JAMA Netw Open 2023;6:e2335311. [Crossref] [PubMed]
- World Health Organization. The Anatomical Therapeutic Chemical (ATC) Classification System and the Defined Daily Dose (DDD) Index 2024. Accessed on January 05, 2024. Available online: https://www.whocc.no/atc_ddd_index/
- Quan H, Sundararajan V, Halfon P, et al. Coding algorithms for defining comorbidities in ICD-9-CM and ICD-10 administrative data. Med Care 2005;43:1130-9. [Crossref] [PubMed]
- Mull HJ, Borzecki AM, Loveland S, et al. Detecting adverse events in surgery: comparing events detected by the Veterans Health Administration Surgical Quality Improvement Program and the Patient Safety Indicators. Am J Surg 2014;207:584-95. [Crossref] [PubMed]
- Davis CL, Pierce JR, Henderson W, et al. Assessment of the reliability of data collected for the Department of Veterans Affairs national surgical quality improvement program. J Am Coll Surg 2007;204:550-60. [Crossref] [PubMed]
- Deyo RA, Cherkin DC, Ciol MA. Adapting a clinical comorbidity index for use with ICD-9-CM administrative databases. J Clin Epidemiol 1992;45:613-9. [Crossref] [PubMed]
- Quan H, Li B, Couris CM, et al. Updating and validating the Charlson comorbidity index and score for risk adjustment in hospital discharge abstracts using data from 6 countries. Am J Epidemiol 2011;173:676-82. [Crossref] [PubMed]
- Subramanian MP, Sahrmann JM, Nickel KB, et al. Assessment of Preoperative Opioid Use Prevalence and Clinical Outcomes in Pulmonary Resection. Ann Thorac Surg 2021;111:1849-57. [Crossref] [PubMed]
- Gaffney A, Bor DH, Himmelstein DU, et al. The Effect Of Veterans Health Administration Coverage On Cost-Related Medication Nonadherence. Health Aff (Millwood) 2020;39:33-40. [Crossref] [PubMed]
- Mulligan CR, Meram AD, Proctor CD, et al. Unlimited access to care: effect on racial disparity and prognostic factors in lung cancer. Cancer Epidemiol Biomarkers Prev 2006;15:25-31. [Crossref] [PubMed]
- Kondo K, Low A, Everson T, et al. Prevalence of and Interventions to Reduce Health Disparities in Vulnerable Veteran Populations: A Map of the Evidence. Washington (DC): Department of Veterans Affairs (US); 2017.
- Heiden BT, Eaton DB Jr, Chang SH, et al. Racial Disparities in the Surgical Treatment of Clinical Stage I Non-Small Cell Lung Cancer Among Veterans. Chest 2022;162:920-9. [Crossref] [PubMed]
- Ingram K, Pickett C, Eaton DB Jr, et al. Cardiovascular medications and overall survival in metastatic hormone-sensitive prostate cancer. J Clin Oncol 2024;42:75. [Crossref]
- Karunanandaa K, Pickett C, Eaton DB Jr, et al. Number of prescription medications and overall survival in veterans with metastatic hormone-sensitive prostate cancer. J Clin Oncol 2024;42:68. [Crossref]