General anesthesia induces acute cell-free DNA methylation changes in peripheral blood
Highlight box
Key findings
• Acute changes in cell-free DNA (cfDNA) methylation patterns were observed in a very rapid manner after general anesthesia (GA, within 1 hour), and the changes were found enriched in pathways related to tissue damage and repair responses.
• Preoperative identification of specific methylation markers induced by GA could effectively predict postoperative biochemical indicators related to key coagulation functions.
What is known and what is new?
• GA is a common practice of medicine. However, short-term and long-term adverse events could occur after GA and the specific mechanism driving these effects has not yet been well-characterized. Recent studies using high-throughput gene expression assays demonstrated the genotoxicity of anesthetics in patients post-surgery.
• This study investigates the overall impact of GA on DNA methylation patterns utilizing cfDNA samples, and its implications in clinical outcome of postoperative abnormal coagulation functions
What is the implication, and what should change now?
• This study suggests a plausible damage response caused by GA via an epigenetic-dependent manner. By utilizing methylation markers associated with GA, a cfDNA test holds promise as a tool for assessing postoperative blood dysfunction risk in clinical settings.
Introduction
Over 300 million major surgical procedures are being carried out under anesthesia worldwide every year (1). Although the safety of anesthesia has been greatly improved over time, adverse effects and unexpected outcomes still occur. It has been estimated that the death rate due to general anesthesia (GA) is 1 in every 100,000 to 200,000 (2).
Anesthetic gases like isoflurane (ISF)/sevoflurane (SVF) and intravenous anesthetic drugs like propofol (PF) are the most widely used agents in GA. Some studies have demonstrated that ISF is potentially a genotoxic agent capable of inducing DNA damage in patients undergoing GA (3). On the other hand, PF can cause toxicogenomic and long-lasting side effects, especially affecting the central nervous system and cognitive abilities. One of the cerebral complications after surgery includes postoperative delirium (POD), which is statistically associated with PF anesthesia (4). To elucidate the underlying mechanism of the cytotoxic effects, studies have evaluated the impact of anesthesia on gene expression (transcriptome) in cells, tissues, or peripheral blood mononuclear cells (PBMCs), and identified differentially expressed genes (DEGs) involved in DNA damage, cell death, and wound response signaling (5-7).
Although the specific mechanism driving these changes has not been well-characterized, it has been hypothesized to have involved the epigenetic regulation of gene expression. A recent study has demonstrated that the expression of DNA methyltransferases 1 (DNMT1) is significantly increased in the hippocampi of rats with neonatal exposure to ISF (8). Ju et al. have found that hippocampal DNMT3a and DNMT3b levels are increased in a rat model with repeated neonatal SVF exposure, resulting in the hypermethylation of the BDNF gene, a critical modulator of synaptic plasticity (9). Furthermore, Sadahiro et al. have observed dramatic shifts in DNA methylation in the vicinity of genes involved in immune regulation in PBMCs following anesthesia and surgery (10). However, most studies are focused on specific genes, while comprehensive studies profiling genome-wide GA-induced epigenetic changes are still missing.
Postoperative bleeding or blood clots are urgent and life-threatening complications in cancer surgeries. Nearly half of the bleeding does not happen in the in-thoracic surgical sites, whereas blood clots, even if related to the surgery itself, can take weeks or up to a month to occur. Thus, identifying novel risk factors and predictive biomarkers for postoperative blood disorders before surgery is important for reducing unwanted complications. However, there is limited consensus regarding risk factors for this to date, such as age, sex, hemoglobin levels, comorbidities, and additional procedures (11). Besides, there have been minimal efforts in developing a risk prediction model—potential reasons include the complexity of the underlying cause and lack of standard practices. One latest study showed a nomogram to predict perioperative blood transfusion after colorectal surgery using traditional statistical methods and only achieved an area under the operating curve of 0.756 (12).
In this study, we profiled DNA methylation in cell-free DNA (cfDNA) collected from a large cohort of lung nodule patients before and after GA using genome-wide targeted bisulfite sequencing. We hypothesized that GA procedures would trigger whole-body toxicogenomic and induce methylation changes, which are associated with postoperative abnormal coagulation functions. The study’s objective is to provide a more comprehensive understanding of the molecular mechanism of GA-related effects like GA-caused body damages and reactions. We present this article in accordance with the TRIPOD reporting checklist (available at https://jtd.amegroups.com/article/view/10.21037/jtd-24-476/rc).
Methods
Participants and sample collection
The study was conducted in accordance with the Declaration of Helsinki (as revised in 2013). We enrolled patients with malignant and benign pulmonary nodules in the First Affiliated Hospital of Guangzhou Medical University from 2017 to 2019. Inclusion criteria were as follows: (I) adults of 18 years old or older; (II) either sex; (III) single pulmonary nodules detected by standard- or low-dose CT screening with lesion size between 5 to 30 mm; (IV) nodule types including solid nodules (SNs), partial solid nodules (mixed ground-glass nodules, mGGNs) and pure ground-glass nodules (pGGNs). Exclusion criteria were as follows: (I) pregnant or lactating females; (II) patients with cancer history within 2 years prior to enrollment (with the exception of nonmelanoma skin cancer).
We obtained approval from institutional review board (IRB) at the First Affiliated Hospital of Guangzhou Medical University (No. 2018-81). Written consent and detailed electronic medical records have been obtained for each participant.
Pre-anesthesia blood samples were collected at least 6 hours before GA using ethylenediaminetetraacetic acid (EDTA) tubes (BD, USA, Cat# 367525). Post-anesthesia blood samples were collected within one hour after GA using EDTA tubes, before surgical incision.
Plasma was separated within 2 hours of obtaining the whole blood samples using a standard protocol and stored at −80 ℃ until needed (volume ranged from 3.0 to 5.0 mL). Repeated freezing and thawing were avoided to prevent cfDNA degradation and contamination by genomic DNA.
cfDNA extraction, bisulfite conversion and methylation analysis
cfDNA was isolated using the MagMAX™ Cell-Free DNA Isolation Kit (Thermo Fisher Scientific, USA, Cat# A29319) following the manufacture’s protocol. The concentration of cfDNA was determined with the Qubit™ dsDNA HS Assay Kit (Thermo Fisher Scientific, USA, Cat# Q32854), and its quality was assessed using the Agilent High Sensitivity DNA Kit (USA, Cat# 5067-4626) on an Agilent 2100 Bioanalyzer Instrument (USA). A total of 10 ng of cfDNA, free from significant genomic DNA contamination (as indicated by DNA size distribution in bioanalyzer analysis), was used for subsequent assays.
Bisulfite conversion was carried out using the Zymo EZ DNA Methylation-Direct Kit (Zymo Research, USA, Cat# D5044) according to the manufacturer’s instructions. The cfDNA was first denatured, followed by sodium bisulfite treatment and desulphonation, and incubated at room temperature for 20 minutes. The bisulfite-converted DNA was then purified using a spin column and eluted with 25 µL of Elution Buffer.
Full details of targeted cfDNA methylation sequencing were specified previously (13). In brief, we applied targeted genome methylation analysis using our proprietary AnchorIRIS technology on 10 ng cfDNA, which directly ligates adaptors to the 3’ end of single stranded DNA molecules after bisulfite conversion, to reduce DNA loss due to bisulfite conversion of constructed libraries. AnchorIRISTM pre-library construction was performed using AnchorDx EpiVisioTM Methylation Library Prep Kit (AnchorDx, Guangzhou/China, Cat# A0UX00019) and AnchorDx EpiVisioTM Indexing PCR Kit (AnchorDx, Guangzhou/China, Cat# A2DX00025). The amplified pre-hybridization libraries were purified using magnetic beads, and their concentration was measured with the Qubit™ dsDNA HS Assay Kit. Pre-hybridization libraries with more than 400 ng of DNA were deemed suitable for target enrichment. Target enrichment was then carried out using the AnchorDx EpiVisio™ Target Enrichment Kit (AnchorDx, Guangzhou/China, Cat# A0UX00031). We used a custom-made genome-wide methylation panel, which includes 12,899 pre-selected regions (13). After probe hybridization, DNA pre-hybridization libraries bound with biotinylated probes were pulled down using the Dynabeads M270 streptavidin beads (Thermo Fisher Scientific, USA, Cat# 65306). The enriched pre-hybridization libraries were further amplified with P5 and P7 primers by the KAPA HiFi HotStart Ready Mix (KAPA Biosystems, USA, Cat# KK2602) and final library product was then purified with Agencourt AMPure XP Magnetic Beads (Beckman Coulter, USA, Cat# A63882) and sequenced on the NovaSeq 6000 system (Illumina Inc., USA).
Sequencing data and differentially methylated region (DMR) analysis
The sequencing data were analyzed using previously reported pipeline (13). For differential methylation (DM) analysis, differentially methylated CpG sites were analyzed by comparing pre-anesthesia to post-anesthesia samples under different statistic criteria like false discovery rates (FDRs) <0.05 (using R package DSS version 2.14.0.), and further assembled into DMR, defined as reads containing at least 3 co-methylated CpGs within a sliding window of 5 CpGs or at least 2 co-methylated CpGs within a sliding window of 3 CpGs.
Dimensional reduction analysis, gene ontology (GO) and pathway enrichment analysis
Dimensional reduction analysis was performed using Uniform Manifold Approximation and Projection (UMAP) analysis R package (14). Gene set enrichment analysis and GO analysis were performed using the R-package Metascape (15).
Modeling, correlation and statistical analysis
The random forest-based coagulation-prediction models were constructed using R package RandomForest with 3-fold cross-validation, 100 splits. The model performance was evaluated by area under receiver operating characteristic curve (AUC) using the R package pROC.
Correlation analysis was performed using Pearson correlation methods—the drug dosage for each reagent was retrieved from patients’ electronic medical record (EMR) data. The degree of overall methylation changes for each patient was calculated as follows: ; .
The univariate and multivariate analyses (covariates including gender, age, smoking history, nodule position and type) were conducted using one-way and multivariate analysis of variance (ANOVA) Regression Analysis, utilizing the Python package statsmodels (version 0.9.0).
Kaplan-Meier survival analysis
Data of non-small lung cancer (NSLC) patients in The Cancer Genome Atlas (TCGA) project were downloaded (16). Only patients with survival information, methylation data were included for further analysis. A total of 461 lung adenocarcinoma (LUAD) and 368 lung squamous cell carcinoma (LUSC) samples were included in our study. Kaplan-Meier analysis was used to assess the overall survival (OS) differences between the high-methylation and low-methylation groups for each target gene.
Results
Study design
Using a comparative retrospective study design, we measured the methylation profiles of cfDNA in a cohort of 1,006 lung-nodule patients administered for surgical procedures. Plasma samples were collected in 529 pre-GA lung-nodule patients (the control group, 413 malignant, and 116 benign nodules) and 477 post-GA patients (the case group, 367 malignant, and 110 benign nodules). For the control group, plasmas were collected at least 6 hours before GA while within 1 hour after GA (before surgery) for the case group. Methylation profiles were analyzed by targeted bisulfite sequencing using a genome-wide enrichment panel covering 12,899 informative methylation regions and 105,844 CpG sites (Figure S1). Our analysis focused on identifying DMRs reflecting methylation level changes and biological significance induced by GA. An overview of the study design is summarized in Figure 1. The demographic characteristics of the 1,006 enrolled lung-nodule patients are presented in Table 1.
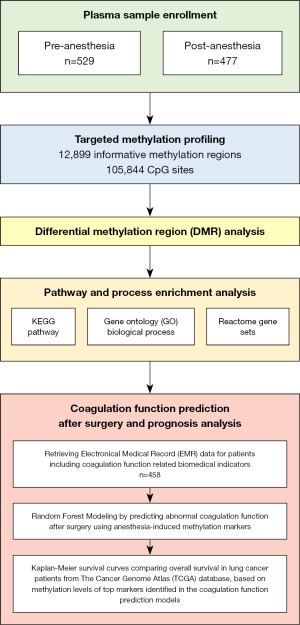
Table 1
Variable | Pre-GA | Post-GA | |||
---|---|---|---|---|---|
Benign nodule group | Malignant nodule group | Benign nodule group | Malignant nodule group | ||
Age, years, median [range] | 52 [30–76] | 59 [24–86] | 54 [27–85] | 60 [27–86] | |
Gender | |||||
Male | 65 | 184 | 66 | 159 | |
Female | 51 | 229 | 44 | 208 | |
Smoking history status | |||||
Never | 83 | 304 | 84 | 298 | |
Former | 14 | 53 | 5 | 16 | |
Current | 19 | 54 | 21 | 52 | |
No data | 0 | 2 | 0 | 1 | |
Nodule location | |||||
Upper lobe | 59 | 263 | 53 | 219 | |
Middle lobe | 43 | 28 | 9 | 19 | |
Lower lobe | 14 | 122 | 48 | 129 | |
Histopathology | |||||
Benign diagnosis | |||||
Granuloma | 56 | – | 7 | – | |
Hamartoma | 12 | – | 13 | – | |
Other | 52 | – | 90 | – | |
Cancer diagnosis | |||||
Small-cell | – | 1 | – | 1 | |
Non-small cell | – | – | – | – | |
Adenocarcinoma | – | 381 | – | 346 | |
Squamous cell | – | 17 | – | 11 | |
Others | – | 14 | – | 9 | |
Tumor staging (AJCC) | |||||
Stage 0–1 | – | 362 | – | 351 | |
Stage 2 | – | 19 | – | 6 | |
Stage 3 | – | 11 | – | 10 | |
Stage 4 | – | 0 | – | 0 | |
Unknown | – | 21 | – | 0 | |
Anesthesia time, mins, average ± std | – | – | 192.6±56.6 | 227.3±56.3 | |
Surgery time, mins, average ± std | – | – | 124.3±50.3 | 148.2±53.5 |
GA, general anesthesia; AJCC, American Joint Committee on Cancer; std, standard deviation.
cfDNA methylation level changes associated with GA
We first evaluated the overall changes in DNA methylation level induced by GA using UMAP analysis (14), a classical dimensionality reduction method, comparing pre- and post-GA patients. The UMAP analysis significantly separated pre-GA and post-GA populations based on methylation signals (Figure 2A).
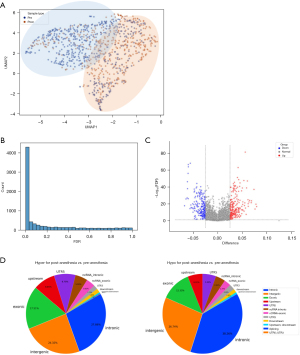
We identified a total of 4,562 significant DMRs (FDRs <0.05, 676 DMRs had an FDR <1E−10), with 1,529 DMRs hypermethylated and 3,033 DMRs hypomethylated in the pre-GA group (Figure 2B,2C, available online: https://cdn.amegroups.cn/static/public/jtd-24-476-1.xlsx). These DMRs were primarily located in the targeted genes’ intronic, intergenic, and exonic regions (Figure 2D). The top-ranked DMR (chr2:25499643-25499844), which was significantly hypermethylated following the anesthesia (DNA methylation level change =5.61%, FDR =4.51E−82), is located in the intronic region of DNMT3A gene on Chromosome 2 (available online: https://cdn.amegroups.cn/static/public/jtd-24-476-1.xlsx). DNMT3A encodes an enzyme that catalyzes the transfer of methyl groups to specific CpG structures in DNA and is responsible for de novo DNA methylation. This observation is consistent with a previous study showing that SVF exposure could induce hippocampal DNMT3A upregulation in a rat model (9).
These 4,562 DMRs were located across 2,555 unique genes (available online: https://cdn.amegroups.cn/static/public/jtd-24-476-1.xlsx), which distributed across different chromosomes (Figure 3A) and are not affected by different intubation methods (Figure S2). GO pathway analysis based on the 2,555 genes highlighted a significant enrichment for functional pathways associated with biological processes including pattern specification process, forebrain development, skeletal and bone system development, and multiple other organs development pathways, as well as cell and different organ morphogenesis pathways, and extra-cellular matrix (ECM) remodeling like cell-adhesion pathway and stroma/fibroblast activation pathways (FDR <1E−10) and molecular function pathways like DNA-binding RNA polymerase II regulation, GTPase regulator activity and actin binding pathways (FDR <1E−5, Figure 3B and available online: https://cdn.amegroups.cn/static/public/jtd-24-476-2.xlsx). Kyoto Encyclopedia of Genes and Genomes (KEGG) pathway analysis enriched signal pathways including PI3K-AKT pathway, Regulation of actin cytoskeleton, Calcium signaling pathways and Ras signaling pathways (FDR <0.01, Figure 3B and available online: https://cdn.amegroups.cn/static/public/jtd-24-476-3.xlsx). Correlation analysis using shared DMRs between general anesthetic reagents dosage and degree of overall methylation changes showed a positive albeit weak correlation trending across different reagents like desflurane, PF, atracurium and SVF (Figure S3). Taken together, the data suggested that acute methylation changes occurred quickly after GA, and enriched functional pathways based on annotated genes highlighted tissue re-formation, development, and stroma activation-related pathways.
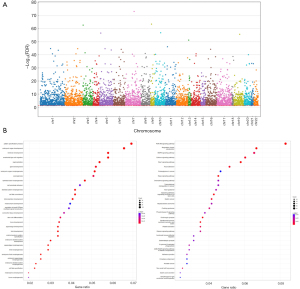
Anesthesia-induced DMRs accurately predict abnormal coagulation function indicators after surgery
Previous studies suggest that local anesthesia may affect perioperative coagulation functions (17,18). To explore whether there is a connection between GA-induced methylation changes and post-operative coagulation function alterations, we obtained available electronic medical records from 458 out of 477 lung-nodule patients (the post-GA group). We retrieved coagulation-related biochemical test results for each patient before and after surgery, including indicators of white blood cells, thrombocytes, hemoglobin, neutrophils, lymphocytes, monocytes, prothrombin time (PT), international normalized ratio (INR), activated-partial-thromboplastin-time (APTT), and D-dimer (Figure 4A and available online: https://cdn.amegroups.cn/static/public/jtd-24-476-4.xlsx). For each indicator, we included only patients within the normal range before surgery and divided them into the case (abnormal after surgery) and control groups (still normal after surgery) (Table S1). In the case group, APTT/PT/INR/D-dimer abnormally increased after surgery, white blood cell/neutrophil/monocyte increased while hemoglobin/lymphocyte decreased after surgery (available online: https://cdn.amegroups.cn/static/public/jtd-24-476-4.xlsx). Based on the overlapping 1,352 GA-induced DMR features, prediction models were derived using Random Forest (3-fold cross-validation, 100 splits) based on the performance of differentiating abnormal patients after surgery. As a result, we found methylation models using only 20 markers could accurately predict indicators including APTT (AUC 0.81), INR (AUC 0.87), D-dimer (AUC 0.82), neutrophil (AUC 0.84), and monocyte (AUC 0.79) (Figure 4B and available online: https://cdn.amegroups.cn/static/public/jtd-24-476-5.xlsx). Both univariate and multivariate analyses of clinical factors, which included variables such as gender, age, smoking history, nodule position, and nodule type, demonstrated that the APTT and INR methylation models remained independent of these factors (P>0.05). However, it was observed that age could potentially influence the D-dimer methylation models (P=0.006, Table 2). However, further analysis showed the GA-associated methylation markers failed to predict the patient length of stay after surgery, which could be due to interference from other complicated factors like complications and psycho-emotional status (Figure S4). These data suggested that post-anesthesia methylation levels of the GA-induced methylation markers could well predict the abnormal biochemical indicators related to the coagulation function after surgery.
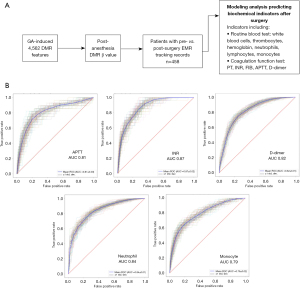
Table 2
Variable | APTT model | INR model | D-dimer model | ||||||||
---|---|---|---|---|---|---|---|---|---|---|---|
df value | F value | P value | df value | F value | P value | df value | F value | P value | |||
Univariate analysis | |||||||||||
Gender | 1.00 | 0.01 | 0.90 | 1.00 | 0.00 | 0.95 | 1.00 | 0.73 | 0.39 | ||
Residual (gender) | 294.00 | 312.00 | 264.00 | ||||||||
Age | 1.00 | 0.27 | 0.60 | 1.00 | 2.40 | 0.12 | 1.00 | 17.18 | 0.006 | ||
Residual (age) | 294.00 | 312.00 | 264.00 | ||||||||
Smoking history | 2.00 | 0.56 | 0.57 | 2.00 | 0.02 | 0.98 | 2.00 | 1.09 | 0.34 | ||
Residual (smoking history) | 293.00 | 311.00 | 263.00 | ||||||||
Nodule position | 3.00 | 0.18 | 0.91 | 3.00 | 0.75 | 0.52 | 3.00 | 1.49 | 0.22 | ||
Residual (nodule position) | 292.00 | 310.00 | 262.00 | ||||||||
Nodule type | 3.00 | 0.25 | 0.86 | 3.00 | 0.39 | 0.76 | 3.00 | 1.66 | 0.18 | ||
Residual (nodule type) | 292.00 | 310.00 | 262.00 | ||||||||
Multivariate analysis | |||||||||||
Gender | 1.00 | 0.01 | 0.90 | 1.00 | 0.00 | 0.95 | 1.00 | 0.79 | 0.38 | ||
Residual (gender) | 286.00 | 304.00 | 256.00 | ||||||||
Age | 1.00 | 0.28 | 0.60 | 1.00 | 2.38 | 0.12 | 1.00 | 17.43 | 0.006 | ||
Residual (age) | 286.00 | 304.00 | 256.00 | ||||||||
Smoking history | 2.00 | 0.69 | 0.50 | 2.00 | 0.00 | 1.00 | 2.00 | 0.73 | 0.48 | ||
Residual (smoking history) | 286.00 | 304.00 | 256.00 | ||||||||
Nodule position | 3.00 | 0.16 | 0.92 | 3.00 | 0.84 | 0.47 | 3.00 | 1.95 | 0.12 | ||
Residual (nodule position) | 286.00 | 304.00 | 256.00 | ||||||||
Nodule type | 3.00 | 1.68 | 0.17 | 3.00 | 0.59 | 0.63 | 3.00 | 2.05 | 0.11 | ||
Residual (nodule type) | 286.00 | 304.00 | 256.00 |
df value, degree of freedom value; APTT, activated-partial-thromboplastin-time; INR, international normalized ratio.
GA-induced DMRs could predict OS in non-small cell lung cancer patients
Activation of coagulation has been known as an impact on the clinical course of lung cancer, and the blood coagulation status has a significant prognostic value (19,20). To analyze the potential role of coagulation function related to the GA-induced DMRs in the OS of non-small cell lung cancer patients, we downloaded LUAD and LUSC clinical data and whole-genome methylation sequencing data from the TCGA database. We then selected and combined the top 5 methylation markers from each prediction model of APTT, INR, D-dimer, monocyte, and neutrophil for the OS prognosis analysis. Among all these 25 methylation markers, the high methylation level of cg02032606 (APTT model), cg25471923 (INR model), and cg22157239 (Neutrophil model) was associated with poor OS of LUAD (n=461, P<0.05). The high methylation level of cg02032606 (APTT model) and low methylation level of cg10438649 (APTT model), cg05024121 (D-dimer model), and cg06598461 (Neutrophil model) was associated with poor OS of LUSC (Figure 5A,5B, n=368, P<0.05). Notably, cg02032606 (within hypermethylated GA-induced DMR), of which the hypermethylation level could predict overall worse survival for both LUAD and LUSC patients, was located in a gene named DLX-4 that has been shown to induce cancer cells to undergo epithelial to mesenchymal transition and enhance tumor migration (21,22). Further prognosis prediction in the LUAD and LUSC subgroups, stratified by gender (male vs. female), age (using a median age of 68 as the threshold), and disease stage (stage I–II vs. stage III–IV), showed that while GA-induced DMRs can predict OS for the LUAD and LUSC groups, they only significantly stratify OS for some of the subgroups (Figures S5,S6).
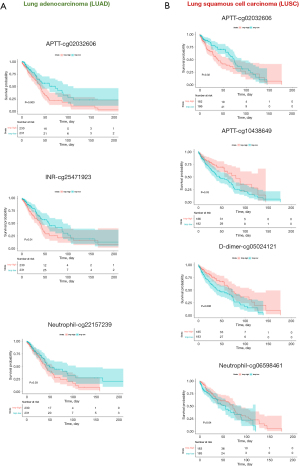
Discussion
In the present study, we evaluated the influence of anesthesia on cfDNA methylation patterns before and shortly after GA in a group of lung-nodule patients. Based on the genome-wide targeted methylation sequencing analysis, we observed rapid and acute changes in cfDNA methylation level following the GA procedure. The DMRs represented genes are known to participate in various processes, including cell cycle and DNA repair, inflammation, signal transduction, and transcriptional regulation. Besides, the GA-induced DMRs before surgery could well predict abnormal coagulation function indicators after surgery and prognosis in lung cancer patients. To the best of our knowledge, this represents the first and largest study to explore acute changes in cfDNA methylation patterns following GA systematically.
Both PF and SVF, which are widely used in GA, have been reported to modulate gene expression related to DNA damage in various tissue samples and PBMCs. Cukurova et al. demonstrated that inhalation agents like SVF could cause severe DNA damage and inflammation response in the bronchoalveolar cells (23). In another study, researchers evaluated the genotoxicological effects of ISF in the lymphocytes and organs of rats and demonstrated that ISF exposure resulted in significant DNA damage in rat lymphocytes, bone marrow, spleen, brain, liver, and lung (24). It is now known that GA results in hypoxia due to malfunction of the respiratory and circulatory systems, which further leads to the production of a large amount of oxygen free radicals, and can directly damage DNA molecules. A similar phenomenon was also observed in PBMCs of subjects who underwent GA: oxidized DNA bases occurred after 15 min of ISF exposure associated with an enhancement of DNA repair activity (6). In our cfDNA methylation analysis, we also found a significant enrichment of biological and molecular pathways involved in tissue damage and repair, e.g., organ development/morphogenesis, cell migration and stroma activation pathways, as well as Ca2+ dependent and RAS/ERK activation pathways, which are implicated as key mediator pathways in response to tissue damage (25,26). Of note, immune responses like activation of T cells differentiation pathway were also observed, which is consistent with the previous study showing GA-induced changes in DNA methylation associated with inflammation and the immune response in PBMCs (10). Besides, these methylation changes were also independent of different intubation methods. Taken together, our study indicated a damage response caused by GA; however, unlike previous gene-expression studies, methylation-related changes highlight more on damage repair and protective reactions.
Considering the origin of cfDNA and previous reports on GA-induced changes in PBMC, it is highly plausible that the methylation changes we observed came from PBMCs. However, we also observed that most deregulated methylation genes were involved in multiple tissue types’ formation and developmental pathways, including lung, heart, digestive tracts, and neural systems. A previous study using gene expression analysis have found that anesthesia can induce toxicological effect on different tissues before the surgery like atrial tissue (27). These observations suggested that the damages caused by GA are not limited to PBMCs, but more likely a whole-body organ reaction—and the apoptosis of cells from different organs contribute to the observed cfDNA methylation changes. In addition, it is striking to see how quickly these changes can occur, considering the time interval between prior- and post-GA plasma collection was within an hour. While research concerning the intraindividual longitudinal alterations in DNA methylation dynamics is limited, there is a growing body of evidence suggesting that an individual’s DNA methylation pattern can undergo modifications under various stimulus over both short- (hours to days) (28-30) and extended (months to years) periods (31). Furthermore, a prior study noted significant alterations in cfDNA mutation profiling within hours of anesthesia administration (32). Nevertheless, whether these methylation changes are transient or stable is still unclear. Wiegand et al. observed that the enduring impact of methylation alterations caused by mental stress can persist for up to a week (33). Thus, it is conceivable that the methylation changes induced by GA may have a long-lasting impact.
Postoperative hemorrhage or blood clotting is a common complication that can occur after any surgical procedure and is associated with coagulation derangement. Our study identified specific GA-induced methylation markers, before surgery, that could well predict key coagulation function-related biochemical indicators after surgery, including APTT, INR, D-dimer, and key elements of extrinsic coagulation cascade like monocyte and neutrophils. Of note, post-operative abnormal up-regulation of APTT/INR is usually suggested as a reduced clotting ability (related to the hemorrhage complications), whereas abnormal up-regulation of D-dimer/monocyte/neutrophil as an increased clotting ability. These suggest that GA could induce whole-body-wise changes in the methylation levels and corresponding gene-expression levels, which could predispose patients to surgical-related blood coagulation disorders (either up-regulation or down-regulation of coagulation function). Besides, the methylation level of certain top markers from the coagulation predictive models were also closely associated with the OS of lung cancer patients. Thus, a cfDNA test using GA-associated methylation markers could potentially serve as a promising tool to predict potential postoperative complications such as blood coagulation abnormalities, enabling early preventive measures and prognosis of lung cancer in the clinic setting.
There are some limitations in this study. Firstly, it did not employ a longitudinal design, and the pre-operative and post-operative samples were not obtained from the same set of patients. To validate the findings, future research should focus on collecting samples from the same individuals. Additionally, in our prognosis analyses using TCGA datasets, the variation in cohort sizes, e.g., in the stage III–IV subgroups, poses a limitation. Some subgroups have significantly fewer samples compared to the overall cohorts, and this issue is worsened when splitting subgroups into methylation-high and methylation-low categories, resulting in some groups having fewer than 30 samples. These limitations highlight the need for larger sample sizes to validate our findings on GA-induced DMR stratifying OS in lung cancer patients. Secondly, the correlation between gene expression and methylation in this study was indirect. Moreover, a majority of the patients included in the study underwent the administration of multiple anesthesia reagents as per the anesthesia guidelines. While this approach helps mitigate the influence of variations resulting from different types of reagents during the analysis, it may not allow us to discern potential differences in methylation patterns among various reagents.
Conclusions
In summary, this large cohort study demonstrated that GA could result in acute cfDNA methylation changes, which are associated with tissue damage and repair responses in different organs. Gaining a deeper understanding of the molecular patterns and mechanisms underlying anesthesia-induced injury can provide valuable insights. Furthermore, these GA-induced methylation changes are associated with postoperative coagulation functions and could serve as a promising predictive tool for coagulation dysfunction after surgery.
Acknowledgments
We thank all the participants for their generosity.
Funding: This study was supported by
Footnote
Reporting Checklist: The authors have completed the TRIPOD reporting checklist. Available at https://jtd.amegroups.com/article/view/10.21037/jtd-24-476/rc
Data Sharing Statement: Available at https://jtd.amegroups.com/article/view/10.21037/jtd-24-476/dss
Peer Review File: Available at https://jtd.amegroups.com/article/view/10.21037/jtd-24-476/prf
Conflicts of Interest: All authors have completed the ICMJE uniform disclosure form (available at https://jtd.amegroups.com/article/view/10.21037/jtd-24-476/coif). J.H. serves as the Executive Editor-in-Chief of Journal of Thoracic Disease. W.L. serves as an unpaid editorial board member of Journal of Thoracic Disease from December 2022 to November 2025. X.L. is an employee of AnchorDx, Inc. H.Y., J.T., H.L. and Zhiwei Chen are employees of AnchorDx Medical Co., Ltd. J.B.F. receives stocks from AnchorDx Medical Co., Ltd. The other authors have no conflicts of interest to declare.
Ethical Statement: The authors are accountable for all aspects of the work in ensuring that questions related to the accuracy or integrity of any part of the work are appropriately investigated and resolved. The study was conducted in accordance with the Declaration of Helsinki (as revised in 2013). We obtained approval from institutional review board (IRB) at The First Affiliated Hospital of Guangzhou Medical University (No. 2018-81). Written consent and detailed electronic medical records have been obtained for each participant.
Open Access Statement: This is an Open Access article distributed in accordance with the Creative Commons Attribution-NonCommercial-NoDerivs 4.0 International License (CC BY-NC-ND 4.0), which permits the non-commercial replication and distribution of the article with the strict proviso that no changes or edits are made and the original work is properly cited (including links to both the formal publication through the relevant DOI and the license). See: https://creativecommons.org/licenses/by-nc-nd/4.0/.
References
- Dobson GP. Trauma of major surgery: A global problem that is not going away. Int J Surg 2020;81:47-54. [Crossref] [PubMed]
- Siddiqui BA, Kim PY. Anesthesia Stages. In: StatPearls. Treasure Island (FL): StatPearls Publishing; 2024.
- Karabiyik L, Sardaş S, Polat U, et al. Comparison of genotoxicity of sevoflurane and isoflurane in human lymphocytes studied in vivo using the comet assay. Mutat Res 2001;492:99-107. [Crossref] [PubMed]
- Luo C, Zou W. Cerebral monitoring of anaesthesia on reducing cognitive dysfunction and postoperative delirium: a systematic review. J Int Med Res 2018;46:4100-10. [Crossref] [PubMed]
- Upton DH, Popovic K, Fulton R, et al. Anaesthetic-dependent changes in gene expression following acute and chronic exposure in the rodent brain. Sci Rep 2020;10:9366. [Crossref] [PubMed]
- Alleva R, Tognù A, Tomasetti M, et al. Effect of different anaesthetic techniques on gene expression profiles in patients who underwent hip arthroplasty. PLoS One 2019;14:e0219113. [Crossref] [PubMed]
- Wang YY, Chang EQ, Zhu RL, et al. An atlas of dynamic peripheral blood mononuclear cell landscapes in human perioperative anaesthesia/surgery. Clin Transl Med 2022;12:e663. [Crossref] [PubMed]
- Wu Z, Zhao P. Epigenetic Alterations in Anesthesia-Induced Neurotoxicity in the Developing Brain. Front Physiol 2018;9:1024. [Crossref] [PubMed]
- Ju LS, Jia M, Sun J, et al. Hypermethylation of Hippocampal Synaptic Plasticity-Related genes is Involved in Neonatal Sevoflurane Exposure-Induced Cognitive Impairments in Rats. Neurotox Res 2016;29:243-55. [Crossref] [PubMed]
- Sadahiro R, Knight B, James F, et al. Major surgery induces acute changes in measured DNA methylation associated with immune response pathways. Sci Rep 2020;10:5743. [Crossref] [PubMed]
- Goldhaber SZ. Risk factors for venous thromboembolism. J Am Coll Cardiol 2010;56:1-7. [Crossref] [PubMed]
- Kim Y, Bagante F, Gani F, et al. Nomogram to predict perioperative blood transfusion for hepatopancreaticobiliary and colorectal surgery. Br J Surg 2016;103:1173-83. [Crossref] [PubMed]
- Liang W, Chen Z, Li C, et al. Accurate diagnosis of pulmonary nodules using a noninvasive DNA methylation test. J Clin Invest 2021;131:e145973. [Crossref] [PubMed]
- Becht E, McInnes L, Healy J, et al. Dimensionality reduction for visualizing single-cell data using UMAP. Nat Biotechnol 2018; Epub ahead of print. [Crossref] [PubMed]
- Zhou Y, Zhou B, Pache L, et al. Metascape provides a biologist-oriented resource for the analysis of systems-level datasets. Nat Commun 2019;10:1523. [Crossref] [PubMed]
- Tomczak K, Czerwińska P, Wiznerowicz M. The Cancer Genome Atlas (TCGA): an immeasurable source of knowledge. Contemp Oncol (Pozn) 2015;19:A68-77. [Crossref] [PubMed]
- Hahnenkamp K, Theilmeier G, Van Aken HK, et al. The effects of local anesthetics on perioperative coagulation, inflammation, and microcirculation. Anesth Analg 2002;94:1441-7. [Crossref] [PubMed]
- Merchán-Galvis A, Anaya R, Rodriguez M, et al. Quality of Life and Post-Surgical Complications in Patients on Chronic Antiplatelet Therapy with Proximal Femur Fracture: 12-Month Follow-Up after Implementing a Strategy to Shorten the Time to Surgery. J Clin Med 2023;12:1130. [Crossref] [PubMed]
- Tas F, Kilic L, Serilmez M, et al. Clinical and prognostic significance of coagulation assays in lung cancer. Respir Med 2013;107:451-7. [Crossref] [PubMed]
- Ma M, Cao R, Wang W, et al. The D-dimer level predicts the prognosis in patients with lung cancer: a systematic review and meta-analysis. J Cardiothorac Surg 2021;16:243. [Crossref] [PubMed]
- Zhang L, Yang M, Gan L, et al. DLX4 upregulates TWIST and enhances tumor migration, invasion and metastasis. Int J Biol Sci 2012;8:1178-87. [Crossref] [PubMed]
- Zheng A, Wei Y, Zhao Y, et al. The role of cancer-associated mesothelial cells in the progression and therapy of ovarian cancer. Front Immunol 2022;13:1013506. [Crossref] [PubMed]
- Cukurova Z, Cetingok H, Ozturk S, et al. DNA damage effects of inhalation anesthetics in human bronchoalveolar cells. Medicine (Baltimore) 2019;98:e16518. [Crossref] [PubMed]
- Kim H, Oh E, Im H, et al. Oxidative damages in the DNA, lipids, and proteins of rats exposed to isofluranes and alcohols. Toxicology 2006;220:169-78. [Crossref] [PubMed]
- Eming SA, Martin P, Tomic-Canic M. Wound repair and regeneration: mechanisms, signaling, and translation. Sci Transl Med 2014;6:265sr6. [Crossref] [PubMed]
- Ghilardi SJ, O'Reilly BM, Sgro AE. Intracellular signaling dynamics and their role in coordinating tissue repair. Wiley Interdiscip Rev Syst Biol Med 2020;12:e1479. [Crossref] [PubMed]
- Yang Z, Zhang M, Wang G, Wei P, Gao S. Identification of anesthetic-induced expression changes using DNA microarray. Mol Med Rep 2015;11:589-96. [Crossref] [PubMed]
- Elbere I, Silamikelis I, Ustinova M, et al. Significantly altered peripheral blood cell DNA methylation profile as a result of immediate effect of metformin use in healthy individuals. Clin Epigenetics 2018;10:156. [Crossref] [PubMed]
- Hajkova P. Epigenetic reprogramming--taking a lesson from the embryo. Curr Opin Cell Biol 2010;22:342-50. [Crossref] [PubMed]
- Glahn A, Riera Knorrenschild R, Rhein M, et al. Alcohol-induced changes in methylation status of individual CpG sites, and serum levels of vasopressin and atrial natriuretic peptide in alcohol-dependent patients during detoxification treatment. Eur Addict Res 2014;20:143-50. [Crossref] [PubMed]
- Ciccarone F, Tagliatesta S, Caiafa P, et al. DNA methylation dynamics in aging: how far are we from understanding the mechanisms? Mech Ageing Dev 2018;174:3-17. [Crossref] [PubMed]
- Alleva R, Tomasetti M, Solenghi MD, et al. Lymphocyte DNA damage precedes DNA repair or cell death after orthopaedic surgery under general anaesthesia. Mutagenesis 2003;18:423-8. [Crossref] [PubMed]
- Wiegand A, Blickle A, Brückmann C, et al. Dynamic DNA Methylation Changes in the COMT Gene Promoter Region in Response to Mental Stress and Its Modulation by Transcranial Direct Current Stimulation. Biomolecules 2021;11:1726. [Crossref] [PubMed]