Establishment and validation of a prognostic model for idiopathic pulmonary fibrosis based on mitochondrial-related genes
Highlight box
Key findings
• An effective two-gene risk signature linked to mitochondrion in idiopathic pulmonary fibrosis (IPF) has been successfully developed, showcasing its promising potential as a robust and dependable classification tool in clinical practice.
What is known and what is new?
• In recent decades, the dysregulation of multiple mitochondrial functions has emerged as a crucial pathological process in the pathogenesis and progression of IPF. Modulating these pathways holds promise for therapeutic interventions against pulmonary fibrosis. However, the precise association between mitochondria-related genes and the prognosis of IPF patients remains incompletely elucidated.
• We developed a prognostic model for assessing mitochondrial-related risks using microarray expression profiling datasets of IPF patients from the Gene Expression Omnibus (GEO) database, along with their corresponding prognostic information, and subsequently validated its predictive efficacy in the validation set. Furthermore, we conducted an analysis of the functional disparities and patterns of immune cell infiltration between the patient groups classified as high-risk and low-risk based on their respective risk scores.
What is the implication, and what should change now?
• The developed prognostic model may offer novel insights for the clinical prediction and management of IPF prognosis. However, further exploration is required to elucidate the specific mechanisms underlying the impact of this model on the prognosis of IPF patients.
Introduction
Idiopathic pulmonary fibrosis (IPF) is a chronic and progressive disease of unknown etiology, distinctively marked by relentless scarring of the lung parenchyma, resulting in a deterioration of quality of life and an earlier onset of mortality (1-3). The elusive nature of its mechanism renders the prognosis for patients diagnosed with IPF discouraging, as statistics indicate a median survival rate of merely 3 to 5 years from the date of diagnosis (1). The Food and Drug Administration has granted approval for two clinical drugs, nintedanib and pirfenidone, for the management of IPF (4-6). Nevertheless, these medications exhibit limited tolerability and are unable to reverse the lung damage inflicted by IPF or mitigate IPF-related mortality (7,8). Therefore, there is an urgent need to delve deeper into the pathophysiology of IPF and identify novel predictive signatures that are associated with its prognosis.
Mitochondria are the central hub in the mammalian cell and serve as the organelles responsible for regulating the homeostasis, bioenergy production, metabolism, biosynthesis, and apoptosis within eukaryotic cells. Multiple signaling pathways converge and intricately interact to regulate interconnected processes encompassing mitochondrial energetics, biogenesis, generation of reactive oxygen species (ROS), preservation and repair of mitochondrial DNA (mtDNA), mitophagy, and mitochondria-nucleus communication (9). Moreover, mitochondria actively influence other cellular components such as the lysosomes, the endoplasmic reticulum (ER), and cytosolic pathways, creating a mitocellular communication network that is based on a variety of signals and cues, and is involved in other intracellular physiological and pathological processes (10). Mitochondrial dysfunction can significantly compromise various cellular functions and contribute to the onset and progression of multiple diseases (11-13). Therefore, research on mitochondria significantly enhances comprehension of diseases, their associated risk factors, and potential therapeutic avenues.
Many studies have shown that in patients with IPF, there is dysregulation in many regulatory mechanisms controlling mitochondrial function (14-16). Alveolar macrophages are a dynamic and heterogeneous population that play a central role in pulmonary inflammation, tissue homeostasis, and lung fibrosis (17). In IPF patients, these macrophages exhibit significant morphological defects and impaired transcriptional activity, characterized by the accumulation of oxidized and abnormal mitochondria, which correlate with clinical variables of the disease (15). Impaired mitochondrial homeostasis and function, observed in alveolar type II epithelial cells and lung fibroblasts, lead to the accumulation of mitochondrial superoxide, DNA damage, stress, and activation of mTORC1, ultimately promoting a senescent cellular phenotype (14,16). Beyond the cellular and pathological mechanisms, targeting mitochondrial dysfunction offers a promising therapeutic strategy for the treatment of pulmonary fibrosis (18,19). By alleviating and improving mitochondrial function, this approach not only addresses the underlying cellular abnormalities but also has the potential to mitigate disease progression and improve overall lung function (18,19).
Considering mitochondrial dysfunction as a risk factor for IPF, targeting the identification of effective mitochondrial-related biomarkers for IPF prognosis presents an inspiring direction for research. While several studies have developed models related to IPF prognosis to predict patient survival, the establishment of prognostic models incorporating mitochondrial aspects in IPF remains markedly underexplored (20-22).
In this study, we identified and analyzed the function of mitochondrial‑related genes in IPF. In addition, a risk score model based on mitochondrial‑related genes was developed to examine the predictive ability. We further conducted an in-depth analysis to explore the correlation and disparity in immune cell infiltration between high-risk and low-risk groups. These discoveries could potentially offer new perspectives in understanding the molecular basis of IPF, presenting a distinct opportunity for targeted treatment options and promoting the development of individualized therapy approaches for individuals with IPF. We present this article in accordance with the TRIPOD reporting checklist (available at https://jtd.amegroups.com/article/view/10.21037/jtd-24-760/rc).
Methods
Data collection
The study was conducted in accordance with the Declaration of Helsinki (as revised in 2013).
IPF-related microarray expression profiling datasets (GSE28042, GSE70866 and GSE38958) were acquired from the Gene Expression Omnibus (GEO) database (http://www.ncbi.nlm.nih.gov/geo/), among which the GSE28042 dataset was used as the training set, the GSE70866 dataset was used as the validation set and the GSE38958 data set was used to validate the expression of genes screened from the prognostic model. Harvested from the GPL5175 platform, the GSE28042 dataset encapsulates gene expression profiles for 75 patients with IPF, accompanied with survival data. Conversely, the GSE70866 dataset, procured from the GPL14550 platform, assembles gene expression insights for 112 IPF patients, similarly enhanced with vital survival information. Furthermore, the GSE38958 dataset from GPL5175 comprises 70 samples of IPF and 45 control samples. The detailed information regarding the GEO database utilized in this study is presented in Table 1.
Table 1
Metadata | GSE28042 | GSE70866 | GSE38958 | GSE135893 | |||||||
---|---|---|---|---|---|---|---|---|---|---|---|
CON | IPF | CON | IPF | CON | IPF | CON | IPF | ||||
Case | 19 | 75 | 20 | 112 | 45 | 70 | 12 | 19 | |||
Agea, years | 53.3±10.2 | 69.0±8.2 | 61.3±8.3 | 68.0±10.1 | 69.3±9.3 | 68.2±7.2 | 35.2±10.1 | 64.8±6.0 | |||
Gender | |||||||||||
Male | 12 (63.2%) | 52 (69.3%) | 16 (80.0%) | 93 (83.0%) | 27 (60.0%) | 58 (82.9%) | 9 (75.0%)b | 12 (63.2%) | |||
Female | 7 (36.8%) | 23 (30.7%) | 4 (20.0%) | 19 (17.0%) | 18 (40.0%) | 12 (17.1%) | 2 (16.7%)b | 7 (36.8%) | |||
Data setsc | Training sets | Validation sets | External data sets | External data sets | |||||||
Platformsd | GPL6480 | GPL14550 | GPL5175 | GPL18573, 20301, 24676 | |||||||
Data type | RNA microarray | RNA microarray | RNA microarray | Single-cell RNA-sequencing |
a, the “age” of this group of patients is presented in the format of mean ± standard deviation. b, in the GSE135893 dataset, one individual in the control group is missing gender data, which is labeled as ‘unknown’. c, training sets and validation sets refer to the datasets used for the development and validation of the prognostic model, respectively. The external data sets represent additional datasets, beyond the training and validation sets, used for external validation of gene expression. d, the specific GPL platforms within the GEO datasets that were utilized in our study. CON, control group; IPF, idiopathic pulmonary fibrosis group. GEO, Gene Expression Omnibus; GPL, Gene Expression Omnibus Platform.
There were 1,136 mitochondrial-related genes downloaded from Human MitoCarta3.0 Database website (https://www.broadinstitute.org/mitocarta/mitocarta30-inventory-mammalian-mitochondrial-proteins-and-pathways).
Identification of differentially expressed genes (DEGs)
The “limma” package within R (version 4.3.2) was utilized to identify DEGs between normal and IPF samples, as well as between high-risk and low-risk groups within the training set (23). P<0.05 and |log2 fold change (FC)| >0.2 were set as cut-off values. The “ggplot2” package in R was leveraged to create a volcano plot for visualizing the DEGs, while a Venn diagram was utilized to highlight the mitochondrial-related DEGs between the DEGs and mitochondrial-related genes, employing the jvenn tool for this purpose (http://www.bioinformatics.com.cn/static/others/jvenn/index.html) (24).
Construction and validation of a prognostic model based on mitochondrial‑related genes
Firstly, the mitochondrial-related DEGs were screened for prognosis-related genes using univariate Cox regression analysis in the training set. Subsequently, to prevent overfitting, we employed least absolute shrinkage and selection operator (LASSO) regression analysis to isolate the crucial mitochondrial-associated DEGs. Ultimately, a multivariate Cox regression analysis was performed to select the mitochondrial-associated genes for the establishment of a prognostic model based on risk scores. The results were presented through Forest plots generated by the “forestplot” R package.
The risk score for each sample was determined using the prescribed formula: Risk score= [(expression value of gene 1 × β1) + (expression value of gene 2 × β2) +…+ (expression value of gene n × βn)], where β is the corresponding gene’s regression coefficient index. Samples were stratified into the high-risk group and low-risk group on the basis of the median value of risk score. Kaplan-Meier analysis and log-rank test were performed to compare the survival differences between the two risk groups using “survival” R package. Receiver operating characteristic (ROC) curves were conducted to assess the model’s prediction accuracy using “timeROC” R package. Multivariate Cox regression analysis was performed to evaluate whether the risk score model is an independent factor in IPF.
Construction of nomogram and calibration curves
Utilizing the “rms” package in R software, nomograms were created based on both training and validation sets to estimate the survival probability of individuals with IPF. Additionally, to evaluate the precision of predictions for 1-, 2-, and 3-year survival rates, calibration curves were plotted using these sets.
Functional enrichment analysis
Gene Ontology (GO), Kyoto Encyclopedia of Genes and Genomes (KEGG) analysis and gene set enrichment analyses (GSEA) were performed by the “clusterProfiler” package to explore the biological functions and signaling pathways with the criteria of FDR <0.05 and P<0.05 and visualized by “ggplot2” package.
Analysis of immune cell infiltration
To delve into the disparities in immune cell profiles existing between IPF samples sourced from high-risk and low-risk populations, we utilize the training set data to conduct an accurate assessment of immune infiltration, leveraging its robustness for reliable and insightful analysis. The CIBERSORT algorithm uses gene expression data to estimate the proportions of different immune cell types in a mixed tissue sample, leveraging a machine learning approach with a known reference dataset. Its key strengths include high accuracy, robustness in noisy data, and the ability to detect subtle differences in immune cell composition (25). The relative abundance of the immune cells between high- and low-risk groups was compared using the Wilcox test. Subsequently, we conducted a comprehensive correlation analysis to assess the relationships between the two candidate genes and the various immune cell types. Additionally, we performed an in-depth analysis to evaluate the correlations among the different immune cell populations themselves.
Quantitative analysis of protein expression of candidate genes
Lung tissues were procured from patients diagnosed with IPF and healthy donors at Tongji Hospital, with IPF diagnoses conforming to the American Thoracic Society (ATS)/European Respiratory Society (ERS) consensus diagnostic criteria. This research endeavor received the endorsement of the Human Assurance Committee at Tongji Hospital, ensuring ethical compliance (TJ-IRB20231297). The acquired lung tissues underwent homogenization in RIPA lysis buffer, a standardized procedure for extracting proteins. Subsequently, these proteins underwent meticulous Western blotting analysis, utilizing well-established protocols and employing specific primary antibodies sourced from Proteintech: GAPDH (Catalog No. 10494-1-AP), ARMCX2 (Catalog No. 12200-1-AP), and ACOT11 (Catalog No. 10776-1-AP) (26).
Advanced analysis of GEO single-cell sequencing datasets for cellular localization
Leveraging the publicly available GSE135893 Single-cell RNA-sequencing dataset from GEO, we conducted a comprehensive and rigorous analysis of gene cellular localization, incorporating 19 IPF patient samples and 12 control samples that were extracted for this study to gain insights into their distinct expression patterns. Drawing upon the author’s comprehensively annotated rds file, we implemented R version 4.3.2 in conjunction with Seurat v5 to ingest the data and streamline its conversion to the most up-to-date format using UpdateSeuratObject function (27). Subsequently, we adopted Dimplot, FeaturePlot and DotPlot functionalities, ensuring a specialized and visual representation of the cellular distribution of candidate genes, initially presented on a uniform manifold approximation and projection (UMAP) plot and further reinforced through a dot plot analysis.
Statistical analysis
All analyses except western blotting were performed with R version 4.3.2 and the corresponding packages. The correlation was evaluated using the Spearman method. A Wilcox test was used to analyze the expression value of genes in different groups. P<0.05 was defined as statistically significant. The results obtained from the western blotting experiments were rigorously analyzed and subsequently visualized using GraphPad Prism 9 software.
Results
Identification and functional enrichment analysis of mitochondrial-related DEGs in IPF
The detailed workflow of our current study is shown in Figure 1. As shown in Figure 2A, 5,426 DEGs were screened and visualized via volcano maps between normal and IPF groups (available online: https://cdn.amegroups.cn/static/public/jtd-24-760-1.xlsx). To facilitate subsequent validation, we excluded genes that were not sequenced in the validation set. Subsequently, an overlap analysis was conducted to delineate 254 putative mitochondrial-related DEGs within the context of IPF, with the results visually represented through a Venn diagram, of which 161 genes were upregulated and 93 genes were downregulated (Figure 2B, available online: https://cdn.amegroups.cn/static/public/jtd-24-760-2.xlsx).
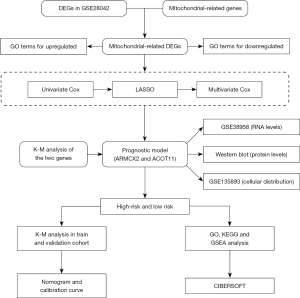
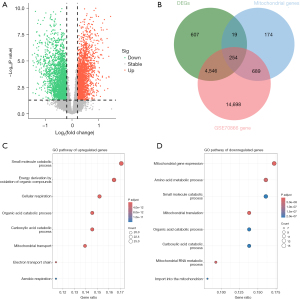
GO enrichment analysis were then carried out to uncover important roles of upregulated and downregulated mitochondrial-related DEGs in IPF. The roles of upregulated DEGs encompass the small molecule catabolic process, energy derivation by oxidation of organic compounds, cellular respiration, organic acid catabolic process, carboxylic acid catabolic process, among others. Regarding the downregulate DEGs, they were mainly related to mitochondrial gene expression, amino acid metabolic process, small molecule catabolic process, mitochondrial translation, organic acid catabolic process, carboxylic acid catabolic process, and additional processes (Figure 2C,2D, available online: https://cdn.amegroups.cn/static/public/jtd-24-760-3.xlsx).
Construction of a mitochondrial-related prognostic model in the train set
Subsequently, we proceeded with the initial construction of a mitochondrial-related prognostic model (Figure 3). Based on above 254 mitochondrial-related DEGs, 28 genes were further selected as potential risk factors for the prognosis of patients with IPF through univariate Cox regression analysis and presented in descending order of hazard ratio (HR) value through the forest plot (P<0.05, Figure 3A, available online: https://cdn.amegroups.cn/static/public/jtd-24-760-4.xlsx). The gene number was further narrowed down to 6 according to LASSO regression analysis and to 2 by multivariate Cox regression analysis (P<0.05, Figure 3B,3C, Table S1). Finally, 2 mitochondrial-related DEGs, including ARMCX2 and ACOT11, were utilized to establish a prognostic model for patients with IPF (P<0.05, Figure 3D, Table S2).
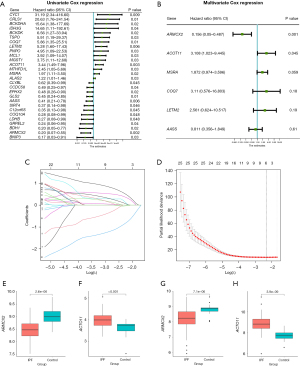
As depicted in Figure 3E,3F, increased expression levels of ACOT11 and decreased expression levels of ARMCX2 were noted in IPF samples relative to normal tissues in the train set. Further K-M analysis showed that patients with high expression of ACOT11 (P=0.01) and low expression of ARMCX2 (P=0.03) had shorter survival time, which is consistent with the previous hazard ratio of the two factors (Figure S1A,S1B).
Then, the risk score for each patient with IPF in both training and validation cohorts was computed based on the following formula: Risk score = ARMCX2 × (−2.04) + ACOT11 × 1.65.
Patients were divided into high-risk and low-risk subgroups based on the median risk score. K-M curves showed that patients in high-risk group had worse overall survival (OS) (P<0.001, Figure 4A). To assess the accuracy of prognostic risk models in predicting 1-, 3-, and 5-year OS, ROC curves were plotted with AUC values of 0.76, 0.87, and 0.88, respectively (Figure 4B). The relationship between the risk score and the survival time, survival status, and risk ranking, and a heatmap of the expressions of the 2 genes were shown in Figure 3C. Taken together, these results demonstrated the robustness of our risk model in predicting the prognosis of patients with IPF (Figure 4C).
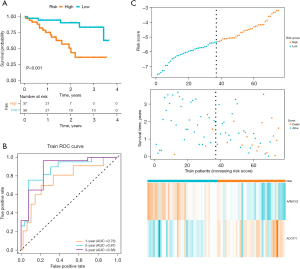
Verification of the prognostic model in the validation set
The robustness of the prognostic risk model was further confirmed in validation datasets (Figure 5A-5C). To further verify the accuracy of the established prognostic model, we obtained 112 IPF patients from GSE70866 and calculated the risk score using the same formula in the training set. According to the median value of the risk score, 56 patients in this cohort were classified into the low-risk group and 56 patients into the high-risk group (Figure 5C). In line with that of the training cohort, patients in high-risk group also had worse prognosis in the validation cohorts by Kaplan Meier analysis (P=0.008, Figure 5A). Meanwhile, ACOT11 was highly expressed in the high-risk group and ARMCX2 was lowly expressed in the high-risk group (Figure 3G,3H). Further K-M analysis showed that patients with high expression of ACOT11 (P<0.001) and low expression of ARMCX2 (P=0.03) had shorter survival time (Figure S2A,S2B). In GSE70866 dataset, the AUC values of the ROC curve for 1-, 3- and 5-year survival were 0.66, 0.73, and 0.66, respectively (Figure 5B). As a result, it was clear that the model we established has acceptable accuracy of predicting prognosis in the validation set.
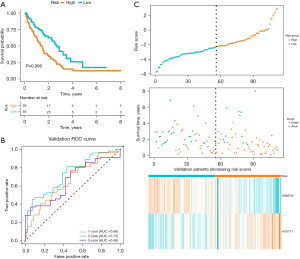
Independent prognostic survey and construction of nomogram and calibration curves
To verify whether the risk score and clinical characteristics could act as independent prognostic factors, we performed multivariate independent prognosis analyses in train and validation sets. As shown in Figure S3A,S3B, only the risk score could be an independent predictor.
In order to provide clinicians with a better quantitative method to forecast the prognosis of the patients with IPF, we established nomograms combining age, gender and risk scores by using train and validation sets, respectively (Figure 6A-6D). Both nomograms showed that the risk score was an important factor among various clinical parameters (Figure 6A,6C). Furthermore, we constructed calibration curves, which showed that the nomogram had a good match with the actual survival of patients with IPF (Figure 6B,6D). Based on these findings, we concluded that our prognostic model could be used to accurately predict the OS of IPF patients.
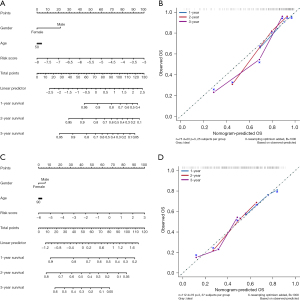
Functional enrichment analysis of the DEGs in high‑ and low‑risk groups
We further conducted functional enrichment analyses of the DEGs in high-risk and low-risk groups in the training cohorts (available online: https://cdn.amegroups.cn/static/public/jtd-24-760-5.xlsx). GO enrichment analysis indicated that the differential genes annotated to biological processes were involved in glycerolipid biosynthetic process, glycerolipid metabolic process and phospholipid metabolic process, and other processes of this kind. Differential genes annotated to cellular component categories were mainly enriched in primary lysosome, azurophil granule and cell-substrate junction. Differential genes annotated to molecular function categories were mainly enriched in actin binding, DNA-binding transcription repressor activity and protein serine kinase activity (Figure 7A, available online: https://cdn.amegroups.cn/static/public/jtd-24-760-6.xlsx). The pathways obtained by KEGG analysis were lysosome, B cell receptor signaling pathway, autophagy, melanogenesis, efferocytosis, long-term potentiation, and thyroid cancer (Figure 7B, available online: https://cdn.amegroups.cn/static/public/jtd-24-760-7.xlsx). The GSEA results revealed a significant correlation between the risk score and Epithelial cell signaling in Helicobacter pylori infection, Lysosome function, Pathogenic Escherichia coli infection, and synaptic vesicle cycle in the high-risk group. Furthermore, a downregulation was observed in the Ribosome pathway (Figure 7C). The detailed GSEA results for the high-risk and low-risk groups were presented in https://cdn.amegroups.cn/static/public/jtd-24-760-8.xlsx.
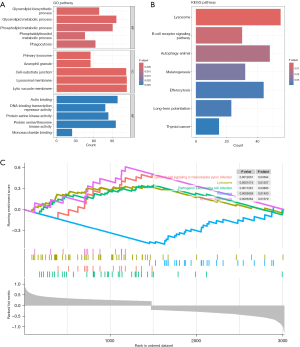
Analysis of immune cell infiltration
Several immune cells were closely related with progression of pulmonary fibrosis and prognosis of patients with IPF. It is reasonable to check the relationship between the risk score and the immune cell infiltration in IPF. In the training set, we employed the CIBERSORT algorithm to perform a detailed analysis of immune cell infiltration (Figure 8A-8D). As illustrated in Figure 8A,8C, the five cell types exhibiting the highest infiltration rates within IPF samples from the training dataset comprise monocytes, T cells CD8, B cells naive, NK cells resting and T cells CD4 memory activated. Furthermore, we conducted a comprehensive analysis of the correlation patterns between ARMCX2, ACOT11, and the proportions of various immune infiltrating cell subsets. Our findings revealed that most of these correlations exhibited opposing trends, particularly notable in the cases of monocytes and naive B cells, suggesting distinct and potentially antagonistic roles of ARMCX2 and ACOT11 in modulating the immune microenvironment (Figure 8B). Ultimately, we embarked on a thorough comparative analysis of the intricate correlations among distinct immune cell subsets, the outcomes of which are vividly illustrated in the heatmap featured in Figure 8D. Notably, the analysis revealed robust correlations not only between T cells CD4 memory activated, B cells naive, and dendritic cells activated but also between NK cells activated, T cells gamma delta, and Eosinophils, among others. These findings provide a specialized and nuanced understanding of the intricate interplay and dynamics that underpin the immune microenvironment (Figure 8D).
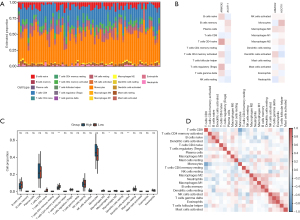
Validation of RNA and protein levels of candidate genes and cellular localization
Finally, we utilized external datasets, including microarray and single-cell RNA-sequencing data, and collected lung tissue samples from IPF patients to validate the RNA expression, protein levels, and cellular localization of the genes ARMCX2 and ACOT11. As shown in Figure 9A, the IPF samples in the GSE38958 dataset exhibited increased expression levels of ACOT11 (P=0.02) and decreased expression levels of ARMCX2 (P=0.001) compared to normal tissues. Subsequently, Western blot analysis of lung tissue from IPF patients and control groups revealed a decrease in ARMCX2 expression (P<0.001), while ACOT11 was undetectable (Figure 9B,9C). We further analyzed public single-cell RNA sequencing data from the GSE135893 dataset, selecting data from 19 IPF patients and 12 controls. The results showed that ARMCX2 is primarily localized in stromal cells, including mesothelial cells and various types of fibroblasts, while ACOT11 is mainly localized in ciliated cells. The experiments and analyses described above further explored and validated the expression levels and cellular localization of the genes.
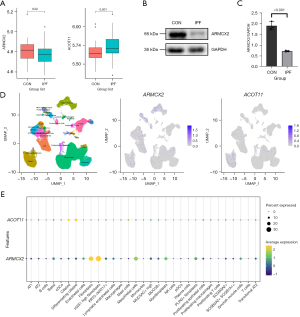
Discussion
The prognosis for patients with IPF is typically unfavorable due to the variable rate of disease progression and significant heterogeneity observed (28). Most individuals with IPF experience a gradual decline in lung function, which can be exacerbated by factors such as infections, exposure to environmental pollutants, or underlying medical conditions (29). This progressive deterioration may ultimately lead to irreversible respiratory failure necessitating mechanical ventilation and often resulting in a diminished quality of life (30). However, it is important to note that the clinical course of IPF varies among patients. While most individuals exhibit a gradual decline in lung function, approximately 10–15% undergo an abrupt deterioration characterized by rapid respiratory failure requiring immediate medical intervention (31,32). The diverse nature of IPF poses challenges in predicting disease trajectory on an individual basis; hence personalized care and treatment plans tailored to specific clinical presentations and disease progression are crucial for managing this devastating condition effectively.
The significance of mitochondrial function and behavior in maintaining cellular and organismal health is widely acknowledged. Consequently, mounting evidence has emerged linking various diseases across different aspects of health to mitochondrial dysfunction (33-35). With the advancing understanding of the role played by mitochondria in human well-being, researchers are increasingly focusing on comprehending the specific functioning of mitochondrial molecules and their potential involvement in driving IPF development. A hallmark feature of IPF is the widespread occurrence of cellular senescence (36). In alveolar type II epithelial cells, alveolar macrophages, and lung fibroblasts, disruptions in mitochondrial homeostasis and function have been identified, resulting in the accumulation of mitochondrial superoxide, mitophagy, DNA damage, cellular stress, activation of mTORC1, and elevated levels of mitochondrial reactive oxygen species (mtROS) (14-16). These pathological changes drive cellular senescence and contribute to the progression of fibrosis (14-16). In addition to these processes, further mitochondrial-related mechanisms also play a critical role in the pathogenesis of IPF. The expression of mitochondrial antiviral signaling protein (MAVS) was found to be upregulated in bleomycin-induced mice, and pharmacological downregulation of MAVS attenuated pulmonary fibrosis (37). Additionally, pulmonary fibrosis involves mitochondrial fusion, with the specific involvement of mitochondrial fusion proteins mitofusin1 (MFN1) and mitofusin2 (MFN2) in ACE2 cells being exacerbated by fatty acid synthase deficiency, thereby aggravating BLM-induced pulmonary fibrosis (38). Other mitochondrial functions, including mitochondrial transfer, mitochondria-related energy metabolism, and mitophagy, play significant roles in the pathogenesis of IPF as well (39-41). The exploration of the correlation between mitochondrial-related genes and IPF is imperative. Therefore, we have leveraged the differential gene expression profiles associated with mitochondrial function in IPF to identify key genes, aiming to elucidate the clinical significance of mitochondrial dysfunction in IPF from a prognostic perspective.
In our comprehensive research, we utilized two GEO datasets to develop and validate a robust prognostic model based on risk scores, specifically tailored to predict survival outcomes by meticulously analyzing the expression patterns of genes related to mitochondria. The utilization of these two GEO datasets, which encompassed an array of clinical samples and extensive patient information, provided an unparalleled opportunity to establish a highly accurate and reliable risk score prognostic model in IPF. Univariate Cox regression analysis, LASSO regression analysis, and multivariate Cox regression analysis were utilized to identify two independent mitochondrial-related genes that significantly impact the prognosis of IPF: ARMCX2 and ACOT11. ARMCX2 is lowly expressed in IPF patients, and a higher expression level is associated with a poorer prognosis. On the other hand, ACOT11 is upregulated in IPF and is also linked to a poor prognosis. Subsequently, the expression of these 2 genes and the corresponding coefficients were used to construct a prognostic model. Moreover, our research endeavored to explore the functional significance of mitochondrial-related genes and DEGs in patients categorized into high-risk and low-risk groups. To achieve this, we meticulously employed multiple functional enrichment methods, including GO, KEGG and GSEA analysis. These comprehensive functional enrichment approaches enabled us to investigate the pathways regulated by mitochondrial-related genes and DEGs in high and low-risk patients. Finally, we utilized the training group data to compare immune cell infiltration and analyze the correlation between risk values and various immune cells.
ARMCX2 is a significant member of the ARMadillo Repeat-Containing proteins on the X chromosome (ARMCX) family, crucial in the malignant transformation of cells and associated with the risk and prognosis of several diseases (42,43). Researchers have discovered that certain individuals belonging to the ARMCX2 exhibited reduced expression levels in various epithelial-origin cancers, such as those affecting the lungs, prostate, colon, and pancreas (44,45). Previous study has indicated that ARMCX2 expression is elevated in surrounding tissues compared to tumor tissues, leading to improved survival outcomes for gastric cancer patients, thereby suggesting its potential as a biomarker for gastric cancer (42). In CCL4-induced liver fibrosis in mice, ARMCX2, as a non-structural component of the liver extracellular matrix (ECM), functions as a potential regulatory factor in hepatic repair, actively contributing to tissue regeneration and the repair process (44).
The ACOT11 gene belongs to the acyl-CoA thioesterase (ACOT) family, which encodes enzymes capable of hydrolyzing fatty acyl-CoA esters into free fatty acids and CoA (46). The current study found that ACOT11 has been identified as a significant prognostic indicator in various tumors (47-49). For instance, the expression of ACOT11 in patients with LUAD is strongly associated with enhanced cell proliferation and an unfavorable prognosis (47,48,50,51). The knockdown of ACOT11 exhibited inhibitory effects on cell growth, migration, invasion, and apoptosis in vitro (50). Consistent with these observations, an in vivo study using a xenograft model demonstrated that ACOT11 knockdown significantly attenuated tumor size and weight (50). Moreover, the expression of ACOT11 was significantly downregulated in clear cell renal cell carcinoma (ccRCC) samples confirmed by both ccRCC cell lines and clinical specimens at both mRNA and protein levels, indicating its potential as a promising biomarker for the diagnosis and prognosis of ccRCC (52).
Previous studies have not investigated the roles of ARMCX2 and ACOT11 in IPF or in murine models of pulmonary fibrosis. To validate our findings, we employed the GSE38958 dataset as an external reference to evaluate the expression levels of these genes. The results corroborated our initial observations. Furthermore, at the protein level, analysis of lung tissue samples from IPF patients revealed that ARMCX2 expression was significantly downregulated, consistent with its RNA expression. In contrast, ACOT11 was not detected in lung tissue, which may be attributed to inconsistencies between RNA and protein expression levels or its expression falling below the detectable threshold. Moving forward, we plan to collect additional samples from IPF patients, such as peripheral blood mononuclear cells (PBMCs) and bronchoalveolar lavage fluid (BALF), and further explore the underlying mechanisms in pulmonary fibrosis using mouse models.
However, there are several limitations in this study. Firstly, the involvement of mitochondrial-related genes in the progression of IPF could not be established due to insufficient data on pulmonary function and other disease-related parameters of IPF patients. Secondly, the findings of our study were largely derived from bioinformatics analysis, and a comprehensive exploration of the specific mechanisms underlying their prognostic impact remains to be conducted. Requiring additional validation in real-world settings, the specific clinical predictive capability of this approach should be further substantiated.
Conclusions
In this research, we have devised a predictive model utilizing the genes ARMCX2 and ACOT11, which effectively forecast the prognosis of individuals diagnosed with IPF. Our discoveries propose that these genes exhibit potential as biomarkers for prognosticating OS in IPF patients. Furthermore, our investigation has enhanced our comprehension of the correlation between mitochondria and IPF through functional enrichment analysis and scrutiny of immune cell infiltration.
Acknowledgments
We extend our sincere appreciation to all patients who generously contributed their data for this study, as well as to the providers of publicly accessible datasets.
Funding: This study was supported by
Footnote
Reporting Checklist: The authors have completed the TRIPOD reporting checklist. Available at https://jtd.amegroups.com/article/view/10.21037/jtd-24-760/rc
Peer Review File: Available at https://jtd.amegroups.com/article/view/10.21037/jtd-24-760/prf
Conflicts of Interest: All authors have completed the ICMJE uniform disclosure form (available at https://jtd.amegroups.com/article/view/10.21037/jtd-24-760/coif). The authors have no conflicts of interest to declare.
Ethical Statement: The authors are accountable for all aspects of the work in ensuring that questions related to the accuracy or integrity of any part of the work are appropriately investigated and resolved. This study was performed in accordance with the Declaration of Helsinki (as revised in 2013). This research endeavor received the endorsement of the Human Assurance Committee at Tongji Hospital, ensuring ethical compliance (TJ-IRB20231297).
Open Access Statement: This is an Open Access article distributed in accordance with the Creative Commons Attribution-NonCommercial-NoDerivs 4.0 International License (CC BY-NC-ND 4.0), which permits the non-commercial replication and distribution of the article with the strict proviso that no changes or edits are made and the original work is properly cited (including links to both the formal publication through the relevant DOI and the license). See: https://creativecommons.org/licenses/by-nc-nd/4.0/.
References
- Spagnolo P, Kropski JA, Jones MG, et al. Idiopathic pulmonary fibrosis: Disease mechanisms and drug development. Pharmacol Ther 2021;222:107798. [Crossref] [PubMed]
- Selman M, Pardo A. Idiopathic Pulmonary Fibrosis: From Common Microscopy to Single-Cell Biology and Precision Medicine. Am J Respir Crit Care Med 2024;209:1074-81. [Crossref] [PubMed]
- Shenderov K, Collins SL, Powell JD, et al. Immune dysregulation as a driver of idiopathic pulmonary fibrosis. J Clin Invest 2021;131:e143226. [Crossref] [PubMed]
- Richeldi L, Fletcher S, Adamali H, et al. No relevant pharmacokinetic drug-drug interaction between nintedanib and pirfenidone. Eur Respir J 2019;53:1801060. [Crossref] [PubMed]
- Sundarakrishnan A, Chen Y, Black LD, et al. Engineered cell and tissue models of pulmonary fibrosis. Adv Drug Deliv Rev 2018;129:78-94. [Crossref] [PubMed]
- Wang M, Liu AD, Niu Q, et al. Blockade of phosphotyrosine pathways suggesting SH2 superbinder as a novel therapy for pulmonary fibrosis. Theranostics 2022;12:4513-35. [Crossref] [PubMed]
- Ahangari F, Becker C, Foster DG, et al. Saracatinib, a Selective Src Kinase Inhibitor, Blocks Fibrotic Responses in Preclinical Models of Pulmonary Fibrosis. Am J Respir Crit Care Med 2022;206:1463-79. [Crossref] [PubMed]
- Dempsey TM, Sangaralingham LR, Yao X, et al. Clinical Effectiveness of Antifibrotic Medications for Idiopathic Pulmonary Fibrosis. Am J Respir Crit Care Med 2019;200:168-74. [Crossref] [PubMed]
- Kaarniranta K, Uusitalo H, Blasiak J, et al. Mechanisms of mitochondrial dysfunction and their impact on age-related macular degeneration. Prog Retin Eye Res 2020;79:100858. [Crossref] [PubMed]
- Mottis A, Herzig S, Auwerx J. Mitocellular communication: Shaping health and disease. Science 2019;366:827-32. [Crossref] [PubMed]
- Xu F, Wu Y, Yang Q, et al. Engineered Extracellular Vesicles with SHP2 High Expression Promote Mitophagy for Alzheimer's Disease Treatment. Adv Mater 2022;34:e2207107. [Crossref] [PubMed]
- Hu Q, Xu J, Wang L, et al. SUCLG2 Regulates Mitochondrial Dysfunction through Succinylation in Lung Adenocarcinoma. Adv Sci (Weinh) 2023;10:e2303535. [Crossref] [PubMed]
- Zhuang L, Jia K, Chen C, et al. DYRK1B-STAT3 Drives Cardiac Hypertrophy and Heart Failure by Impairing Mitochondrial Bioenergetics. Circulation 2022;145:829-46. [Crossref] [PubMed]
- Bueno M, Lai YC, Romero Y, et al. PINK1 deficiency impairs mitochondrial homeostasis and promotes lung fibrosis. J Clin Invest 2015;125:521-38. [Crossref] [PubMed]
- Tsitoura E, Vasarmidi E, Bibaki E, et al. Accumulation of damaged mitochondria in alveolar macrophages with reduced OXPHOS related gene expression in IPF. Respir Res 2019;20:264. [Crossref] [PubMed]
- Schuliga M, Pechkovsky DV, Read J, et al. Mitochondrial dysfunction contributes to the senescent phenotype of IPF lung fibroblasts. J Cell Mol Med 2018;22:5847-61. [Crossref] [PubMed]
- Hou F, Xiao K, Tang L, et al. Diversity of Macrophages in Lung Homeostasis and Diseases. Front Immunol 2021;12:753940. [Crossref] [PubMed]
- Guo F, Xu F, Li S, et al. Amifostine ameliorates bleomycin-induced murine pulmonary fibrosis via NAD(+)/SIRT1/AMPK pathway-mediated effects on mitochondrial function and cellular metabolism. Eur J Med Res 2024;29:68. [Crossref] [PubMed]
- Ding L, Yang Y, Wang Z, et al. Qimai Feiluoping decoction inhibits mitochondrial complex I-mediated oxidative stress to ameliorate bleomycin-induced pulmonary fibrosis. Phytomedicine 2023;112:154707. [Crossref] [PubMed]
- Chen H, Xia Z, Qing B, et al. Analysis of necroptosis-related prognostic genes and immune infiltration in idiopathic pulmonary fibrosis. Front Immunol 2023;14:1119139. [Crossref] [PubMed]
- He Y, Yao T, Zhang Y, et al. Pyroptosis-related signatures predict immune characteristics and prognosis in IPF. Heliyon 2024;10:e23683. [Crossref] [PubMed]
- Huang G, Xu X, Ju C, et al. Identification and validation of autophagy-related gene expression for predicting prognosis in patients with idiopathic pulmonary fibrosis. Front Immunol 2022;13:997138. [Crossref] [PubMed]
- Ritchie ME, Phipson B, Wu D, et al. limma powers differential expression analyses for RNA-sequencing and microarray studies. Nucleic Acids Res 2015;43:e47. [Crossref] [PubMed]
- Bardou P, Mariette J, Escudié F, et al. jvenn: an interactive Venn diagram viewer. BMC Bioinformatics 2014;15:293. [Crossref] [PubMed]
- Newman AM, Liu CL, Green MR, et al. Robust enumeration of cell subsets from tissue expression profiles. Nat Methods 2015;12:453-7. [Crossref] [PubMed]
- Hu Y, Yu J, Wang Q, et al. Tartrate-Resistant Acid Phosphatase 5/ACP5 Interacts with p53 to Control the Expression of SMAD3 in Lung Adenocarcinoma. Mol Ther Oncolytics 2020;16:272-88. [Crossref] [PubMed]
- Hao Y, Stuart T, Kowalski MH, et al. Dictionary learning for integrative, multimodal and scalable single-cell analysis. Nat Biotechnol 2024;42:293-304. [Crossref] [PubMed]
- Ley B, Ryerson CJ, Vittinghoff E, et al. A multidimensional index and staging system for idiopathic pulmonary fibrosis. Ann Intern Med 2012;156:684-91. [Crossref] [PubMed]
- Liu GY, Budinger GRS, Dematte JE. Advances in the management of idiopathic pulmonary fibrosis and progressive pulmonary fibrosis. BMJ 2022;377:e066354. [Crossref] [PubMed]
- Wollin L, Distler JHW, Redente EF, et al. Potential of nintedanib in treatment of progressive fibrosing interstitial lung diseases. Eur Respir J 2019;54:1900161. [Crossref] [PubMed]
- Luppi F, Cerri S, Taddei S, et al. Acute exacerbation of idiopathic pulmonary fibrosis: a clinical review. Intern Emerg Med 2015;10:401-11. [Crossref] [PubMed]
- Oda K, Yatera K, Fujino Y, et al. Efficacy of concurrent treatments in idiopathic pulmonary fibrosis patients with a rapid progression of respiratory failure: an analysis of a national administrative database in Japan. BMC Pulm Med 2016;16:91. [Crossref] [PubMed]
- Chan DC. Mitochondrial Dynamics and Its Involvement in Disease. Annu Rev Pathol 2020;15:235-59. [Crossref] [PubMed]
- Chow J, Rahman J, Achermann JC, et al. Mitochondrial disease and endocrine dysfunction. Nat Rev Endocrinol 2017;13:92-104. [Crossref] [PubMed]
- Murphy MP, Hartley RC. Mitochondria as a therapeutic target for common pathologies. Nat Rev Drug Discov 2018;17:865-86. [Crossref] [PubMed]
- Schafer MJ, White TA, Iijima K, et al. Cellular senescence mediates fibrotic pulmonary disease. Nat Commun 2017;8:14532. [Crossref] [PubMed]
- Kim SH, Lee JY, Yoon CM, et al. Mitochondrial antiviral signaling protein is crucial for the development of pulmonary fibrosis. Eur Respir J 2021;57:2000652. [Crossref] [PubMed]
- Chung KP, Hsu CL, Fan LC, et al. Mitofusins regulate lipid metabolism to mediate the development of lung fibrosis. Nat Commun 2019;10:3390. [Crossref] [PubMed]
- Huang T, Lin R, Su Y, et al. Efficient intervention for pulmonary fibrosis via mitochondrial transfer promoted by mitochondrial biogenesis. Nat Commun 2023;14:5781. [Crossref] [PubMed]
- Pokharel MD, Garcia-Flores A, Marciano D, et al. Mitochondrial network dynamics in pulmonary disease: Bridging the gap between inflammation, oxidative stress, and bioenergetics. Redox Biol 2024;70:103049. [Crossref] [PubMed]
- Roque W, Romero F. Cellular metabolomics of pulmonary fibrosis, from amino acids to lipids. Am J Physiol Cell Physiol 2021;320:C689-95. [Crossref] [PubMed]
- Wang T, Zhong H, Qin Y, et al. ARMCX Family Gene Expression Analysis and Potential Prognostic Biomarkers for Prediction of Clinical Outcome in Patients with Gastric Carcinoma. Biomed Res Int 2020;2020:3575038. [Crossref] [PubMed]
- Wang F, Wang C, Li B, et al. Identification of angiogenesis-related subtypes, the development of a prognosis model, and features of tumor microenvironment in colon cancer. Biotechnol Appl Biochem 2024;71:45-60. [Crossref] [PubMed]
- Klaas M, Kangur T, Viil J, et al. The alterations in the extracellular matrix composition guide the repair of damaged liver tissue. Sci Rep 2016;6:27398. [Crossref] [PubMed]
- Rosales-Reynoso MA, Ochoa-Hernández AB, Aguilar-Lemarroy A, et al. Gene expression profiling identifies WNT7A as a possible candidate gene for decreased cancer risk in fragile X syndrome patients. Arch Med Res 2010;41:110-118.e2. [Crossref] [PubMed]
- Kirkby B, Roman N, Kobe B, et al. Functional and structural properties of mammalian acyl-coenzyme A thioesterases. Prog Lipid Res 2010;49:366-77. [Crossref] [PubMed]
- Shen GY, Yang PJ, Zhang WS, et al. Identification of a Prognostic Gene Signature Based on Lipid Metabolism-Related Genes in Esophageal Squamous Cell Carcinoma. Pharmgenomics Pers Med 2023;16:959-72. [Crossref] [PubMed]
- Jin X, Liu D, Kong D, et al. Dissecting the alternation landscape of mitochondrial metabolism-related genes in lung adenocarcinoma and their latent mechanisms. Aging (Albany NY) 2023;15:5482-96. [Crossref] [PubMed]
- Reinert T, Modin C, Castano FM, et al. Comprehensive genome methylation analysis in bladder cancer: identification and validation of novel methylated genes and application of these as urinary tumor markers. Clin Cancer Res 2011;17:5582-92. [Crossref] [PubMed]
- Liang C, Wang X, Zhang Z, et al. ACOT11 promotes cell proliferation, migration and invasion in lung adenocarcinoma. Transl Lung Cancer Res 2020;9:1885-903. [Crossref] [PubMed]
- Liu KT, Yeh IJ, Chou SK, et al. Regulatory mechanism of fatty acid‑CoA metabolic enzymes under endoplasmic reticulum stress in lung cancer. Oncol Rep 2018;40:2674-82. [Crossref] [PubMed]
- Xu CL, Chen L, Li D, et al. Acyl-CoA Thioesterase 8 and 11 as Novel Biomarkers for Clear Cell Renal Cell Carcinoma. Front Genet 2020;11:594969. [Crossref] [PubMed]