Diagnostic artificial intelligence model predicts lymph node status in non-small cell lung cancer using simplified examination
Highlight box
Key findings
• Present artificial intelligence (AI) model without enhanced computed tomography (CT) and positron emission tomography (PET) showed 80.0% accuracy, 95.6% specificity and an area under the curve (AUC) of 0.75.
What is known and what is new?
• AI model predicts lymph node metastasis in lung cancer have been reported. However, these models required contrast-enhanced CT and PET.
• We published an AI model using simple examination, which predict preoperative lymph node status with relatively high performance.
What is the implication, and what should change now?
• These models have potential to categorize suitable surgical procedures for lung cancer patients without needing contrast-enhanced CT or PET.
• Surgeons can assess the lymph node status with confirming the presence AI model score and contrast-enhanced CT or PET features, while also consider the range of lymph node metastasis.
Introduction
Lung cancer is the most common malignancy with a high mortality rate and has become a public health concern all over the world. Non-small cell lung cancer (NSCLC) is the most common pathological subtype of lung cancer, accounting for approximately 80% of all cases in Japan (1). The percentage of lymph node metastasis is relatively high, ranging from 8.3% to 15.9% although the clinical stage I lung cancer (2-6). Lymph node metastasis is considered to be closely associated with unfavorable prognosis and recurrence of lung cancer. In addition, lymph node metastasis is directly linked to the staging process and therapeutic strategies for lung cancer. The status of hilar and mediastinal lymph nodes is crucial for the management of lung cancer patients. Therefore, almost all patients clinically diagnosed with lung cancer in Japan routinely undergo preoperative contrast-enhanced computed tomography (CT) and positron emission tomography (PET) to assess the lymph node status. Contrast-enhanced CT reportedly shows 59% diagnostic sensitivity and 78% specificity for lymph node metastasis (7), PET shows 77% sensitivity and 90% specificity (8).
Small and early-stage lung cancers are increasingly being identified due to continued improvements in imaging technologies (9). Previous studies in the field of small and early-stage NSCLC have demonstrated that tumor size and ground glass opacity ratio may predict lymph node metastasis (10-12). However, whether patient with early-stage lung cancer should routinely undergo preoperative chest contrast-enhanced CT and PET remains controversial. Clinicians should consider the necessity of such examinations from the perspectives of both medical economics and radiation exposure.
In recent years, artificial intelligence (AI) models have been widely applied to the development of disease prediction algorithms. Machine learning uses algorithms to identify patterns within large-scale datasets, then those patterns are applied to create a predictive data model. Models for predicting lymph node metastasis have already been reported (13-16). However, most studies in the field of AI have only focused on disease-predicting models. The main purpose of the present study was to develop an AI model that can predict lymph node metastasis in patients with NSCLC using only the information available from medical examinations, medical interviews, blood tests and plain CT without using PET or contrast-enhanced CT. We present the following article in accordance with the TRIPOD reporting checklist (available at https://jtd.amegroups.com/article/view/10.21037/jtd-24-1067/rc).
Methods
Ethical statement
This study was a retrospective analysis conducted in the Department of Thoracic Surgery at Iwate Medical University between January 2011 and October 2022. All study protocols were approved by the institutional review board of Iwate Medical University (No. MH2021-061). The present study was conducted in accordance with the Declaration of Helsinki (as revised in 2013). Individual consent for this retrospective analysis was waived.
Patients
A total of 1,074 patients who underwent anatomical pulmonary resection (segmentectomy, lobectomy and pneumonectomy) with mediastinal lymph node dissection for NSCLC were included. Among these, 86 patients were excluded based on an unknown primary tumor in radiological examination (n=37), unknown smoking history (n=28), and unknown preoperative tumor marker levels (n=21). Finally, a total of 988 patients were eligible for inclusion in this study. Baseline variables collected included age, sex, smoking index, serum concentration of carcinoembryonic antigen (CEA), serum concentration of cytokeratin fragment 19 (CYFRA), tumor side (right or left), lung segment location of the tumor, maximum diameter of the tumor on CT, maximum diameter of the solid part of the tumor, and consolidation-to-tumor ratio. In this study, we did not assess the status of hilar and mediastinal lymph node in CT and PET-CT.
Pre- and postoperative staging
Clinical staging was based on chest X-ray, contrast-enhanced CT, magnetic resonance imaging of the brain and PET-CT. All patients underwent the above examinations and assessment of clinical staging. Final histological stage was based on the eighth edition of the American Joint Commission on Cancer tumor, node, metastasis (TNM) staging system (17). Pathological classification was evaluated according to World Health Organization criteria (18). Lymph node station was numbered according to the International Association for the Study of Lung Cancer lymph node map (19).
Radiological evaluations
The lung segment of the tumor was assessed based on the involved pulmonary vein on preoperative CT. When the tumor involved two segments, the segment with the greater proportion of nodule or the involved pulmonary vein was used. The consolidated component was defined as the area of increased opacity that completely obscured underlying vascular markings. The ground glass component was defined as the area showing a slight, homogeneous increase in density that did not obscure underlying vascular markings (20). The consolidation-to-tumor ratio was then defined in this study as the ratio of the maximum diameter of consolidation to maximum tumor diameter.
AI model
To create the AI model, all included patients were randomly divided into two groups: the training group (n=790) and the validation group (n=198) (training:validation =4:1). We built classification model by learning the data divided training group. We attempt to predict the lymph node status using six machine learning algorithms, Support Vector Classification (SVC), k-nearest neighbor algorithm (k-NN), logistic regression (LR), random forest (RF), gradient boosting (GB) and multilayer perceptron (MLP). All models work to discriminate whether positive label or negative label. The SVC is the classification model based on the support vector machines (SVM). The SVM is the training method to find the boundary line which can classify the labels of the training data. The SVC is decided the label based on the boundary line. The k-NN is the predictive method using the training data. The label is decided based on the data which is the nearest distance of the training data. Herein, k is the number of the extraction of the training data. In this study, k is set to 5. The LR is the classification model for binary classification. The LR is the prediction method of the dependent variable probability from the independent variable. Based on the dependent variable probability, the LR decides the label. The RF is the ensemble learning method based on the decision tree. First, some decision trees are generated from the training data in separate. Second, the validation data is classified using the generated decision trees. Finally, based on the results of the output of the decision trees, the label is decided. The GB is also the ensemble learning method based on the decision tree. The GB generate a decision tree and calculate the error. Afterward, the GB generate a decision tree to decrease the error. The processing iterates many times, and some decision tree is generated in series. These generated decision tree decides the label of the validation data. The MLP is the supervised learning algorithm. The MLP can learn multilayers using the training data. The MLP consists of an input layer, hidden layer, and output layer. Weights of hidden layers are updated by the training, and the MLP aims to classify the classes. The MLP can solve non-linear separation problem because of the hidden layers.
We used the models implemented in scikit-learn. Five-fold cross-validation was performed to investigate the generalization of each model. Finally, the average of these five accuracies with the one model was treated as the performance of the model. Also, we calculated the standard deviation (SD) and 95% confidence interval (CI) of area under the curve (AUC) based on the method developed by Hanley et al. (21).
Surgical procedures
Pulmonary resection was performed under general anesthesia with a double-lumen endotracheal tube for single-lung ventilation. The affected lung was deflated as soon as the pleural space was opened, and deflation was maintained throughout most of the operative course. The patient was placed in a lateral decubitus position. Complete thoracoscopic surgery was underwent via three-port under monitor vision (22). Complete and systematic lymph node dissection of hilar and mediastinal area was performed in all lobectomy and segmentectomy cases.
Statistical analysis
JMP version 14 statistical software (SAS Institute, Cary, NC, USA) was applied for statistical analyses in present study. Categorical variables were compared between groups using Wilcoxon rank-sum test or Pearson’s chi-square test. Differences between groups were defined significant for values of P<0.05. Continuous data were described as mean ± SD, and categorical data were described as count and proportion.
Results
Patient characteristics
Clinical data of the 988 patients (790 patients in the training group, 198 patients in the validation group) are summarized in Table 1. The total cohort comprised 586 men and 402 women with a mean age of 69.4 years. Mean smoking index was 505.2. Blood tests showed a mean serum concentrations of 3.2 ng/mL for CEA and 2.1 ng/mL for CYFRA. Plain CT of the chest showed a right-side tumor in 609 cases and left-side tumor in 379. The most common tumor segment was segment 6 (142 cases). Mean tumor size was 24 mm, and mean consolidation-to-tumor ratio was 89%. Histopathological diagnosis showed pN1 in 8.4% of case, and pN2 in 13.4%. No clinical characteristics differed significant between the training and validation groups.
Table 1
Variables | Training group (n=790) | Validation group (n=198) | P value |
---|---|---|---|
Age (years) | 69.5±8.8 | 69.4±8.7 | 0.71 |
Sex | 0.46 | ||
Male | 464 (58.7) | 122 (61.6) | |
Female | 326 (41.3) | 76 (38.4) | |
Brinkman index | 490.9±576.9 | 562.2±603.5 | 0.17 |
CEA (ng/mL) | 7.5±26.1 | 5.8±8.5 | 0.36 |
CYFRA (ng/mL) | 2.9±2.9 | 3.0±4.5 | 0.49 |
Tumor side | 0.19 | ||
Right | 479 (60.6) | 130 (65.7) | |
Left | 311 (39.4) | 68 (34.3) | |
Tumor segment | 0.53 | ||
S1 | 73 (9.2) | 22 (11.1) | |
S1+2 | 106 (13.4) | 24 (12.1) | |
S2 | 106 (13.4) | 34 (17.1) | |
S3 | 93 (11.8) | 25 (12.6) | |
S4 | 42 (5.3) | 7 (3.5) | |
S5 | 38 (4.8) | 10 (5.1) | |
S6 | 118 (14.9) | 24 (12.1) | |
S7 | 1 (0.1) | 2 (1.0) | |
S8 | 64 (8.1) | 13 (6.6) | |
S9 | 77 (9.8) | 18 (9.1) | |
S10 | 72 (9.1) | 19 (9.6) | |
Total tumor size (mm) | 27.3±13.4 | 27.5±14.8 | 0.64 |
Solid component size (mm) | 24.6±14.4 | 25.1±16.0 | 0.98 |
Consolidation/tumor ratio (%) | 88.5±0.2 | 89.2±0.2 | 0.47 |
Histological subtype | 0.74 | ||
Adenocarcinoma | 586 (74.2) | 162 (81.8) | |
Squamous cell carcinoma | 156 (19.7) | 31 (15.7) | |
Others | 48 (6.1) | 5 (2.5) | |
Number of dissected lymph nodes | 17.9 | 18.1 | 0.80 |
Lymph node metastasis positive | 172 (21.8) | 43 (21.7) | 0.99 |
Pathological N stage | 0.98 | ||
N0 | 618 (78.2) | 155 (78.3) | |
N1 | 67 (8.5) | 16 (8.1) | |
N2 | 105 (13.3) | 27 (13.6) |
Continuous data are expressed as mean ± standard deviation, and categorical data are described as count and proportion. CEA, carcinoembryonic antigen; CYFRA, cytokeratin fragment 19.
AI model performance
The present study, established 6 prediction models using the 10 independent clinical factors. Areas under the curve (AUCs) in the training group for SVC, k-NN, LR, RF, GB and MLP were 0.63, 0.84, 0.73, 0.99, 0.95 and 0.76, respectively (Figure 1). In the validation group, AUCs for SVC, k-NN, LR, RF, GB and MLP were 0.60, 0.63, 0.70, 0.74, 0.75 and 0.70, respectively (Figure 2). We then assessed the specificity and performance of the six models (Table 2). Specificity for SVC, k-NN, LR, RF, GB and MLP was 0.99, 0.92, 0.97, 0.97, 0.96 and 0.96, respectively. Diagnostic accuracy for SVC, k-NN, LR, RF, GB and MLP was 0.78, 0.76, 0.77, 0.80, 0.80 and 0.78, respectively. Overall, these results demonstrated that the GB model offers the best predictive performance, followed by the RF model.
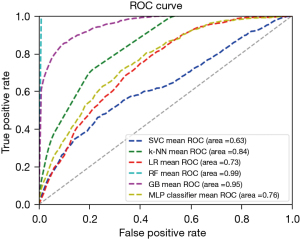
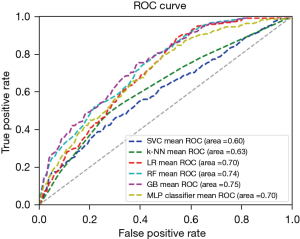
Table 2
Methods | Accuracy | Sensitivity | Specificity | AUC | SD | 95% CI |
---|---|---|---|---|---|---|
SVC | 0.78 | 0 | 0.99 | 0.60 | 0.05 | 0.50–0.70 |
k-NN | 0.76 | 0.17 | 0.92 | 0.63 | 0.05 | 0.53–0.72 |
LR | 0.77 | 0.06 | 0.97 | 0.70 | 0.05 | 0.61–0.80 |
RF | 0.80 | 0.21 | 0.97 | 0.74 | 0.05 | 0.64–0.83 |
GB | 0.80 | 0.24 | 0.96 | 0.75 | 0.05 | 0.66–0.84 |
MLP | 0.78 | 0.13 | 0.96 | 0.70 | 0.05 | 0.61–0.79 |
AI, artificial intelligence; AUC, area under the curve; SD, standard deviation; CI, confidence interval; SVC, Support Vector Classification; k-NN, k-nearest neighbor algorithm; LR, logistic regression; RF, random forest; GB, gradient boosting; MLP, multilayer perceptron.
Permutation feature importance (PFI)
We further analyzed the data obtained from the GB and RF models for PFI. Figure 3 shows the PFI of the 10 clinical variables in each model. Serum CEA level was ranked first in each model, followed by maximum solid-part diameter. In the GB model, serum CYFRA concentration, age, and consolidation-to-tumor ratio made up the remainder of the top five crucial variables, similar to the RF model.
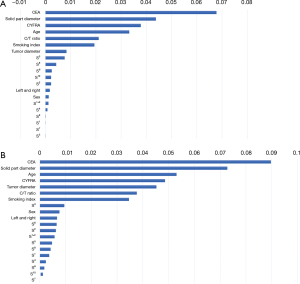
Discussion
Recent developments in AI technology and machine learning have increased research into disease prediction. These technologies hold promise for predicting tumor metastasis, tumor diagnosis and prognosis by analyzing and training on large-scale medical records within a short period. Several favorable studies have already reported the use of AI models for the prediction of lymph node metastasis from malignant tumors such as osteosarcoma, breast cancer and thyroid carcinoma (23-25). However, the potential of AI technologies in the medical arena depends on application in the clinical field.
In the present study, we hypothesized that an AI model could predict lymph node metastasis in patients with NSCLC using information from medical examinations, medical interviews, blood tests and plain CT of the chest. Indeed, our AI models showed high performance for predicting lymph node metastasis (80.0% accuracy, 95.6% specificity, and an AUC of 0.79). These simple examinations assumed a situation in which the patient would normally be undergoing detailed preoperative examinations such as PET and contrast-enhanced CT. On the other hand, non-AI method which predicts lymph node metastasis In NSCLC have been reported. Guinde et al. established Quebec prediction model which had a specificity, positive predictive value and negative predictive value of 81.1%, 28.3% and 96.8%, respectively (26). Although Quebec prediction model needs the findings of PET-CT imaging, the performance seems equivalent to our AI model. Therefore, we conduct that AI predicting model should be discussed how to reduce the invasive examination, not discuss the performance. Our findings suggest that AI models for early-stage NSCLC may help clinicians avoid aggressive examinations, providing more opportunities for aggressive examination of patient with advanced NSCLC.
In 2022, the Japan Clinical Oncology Group and West Japan Oncology Group noted the noninferiority of segmentectomy for early-stage NSCLC compared to lobectomy in terms of 5-year overall and relapse-free survival rates (JCOG0802/WJOG4607L) (27). This trial established the role of segmentectomy for the surgical treatment of less than 2cm NSCLC. However, higher rates of locoregional recurrence after segmentectomy still constitute and have been reported 10.5% in JCOG0802/WJOG4607L trial. Additionally, the most frequent recurrence in segmentectomy was lymph node (41.8%) in this trial. Therefore, accurate preoperative evaluation of lymph node status is extremely important for sublobar resection of NSCLC. AI technology for assessing lymph node status could thus be applied to determine the surgical indications for early-stage NSCLC.
PFI in this study showed the serum CEA and CYFRA level, maximum solid-part diameter, age and consolidation-to-tumor ratio were strong variables of predicting lymph node metastasis. These findings in AI model match those the clinical features which relates lymph node metastasis reported previously (3-6). Interestingly, AI model in this study recognized segment 6 and segment 3 were higher risk of lymph node metastasis. This result may corroborate the tumor centrality, were considered the anatomically shorter distance to hilar lymph nodes in segment 6 or segment 3 compared with the other segment. Watanabe et al. and Handa et al. reported that tumor originating from the superior segment showed a significantly higher incidence of lymph node metastasis than the basal segment (28,29). AI model in this study may help clinicians to understand the risk of lymph node metastasis by confirming the PFI variables.
This study showed several limitations that warrant consideration. First, the dataset was obtained from a single institute. Our findings thus may not be generalizable to other institutions and a larger, multicenter study is necessary to validate the present findings. In addition, any clinical applications in far to be defined. Second, the present study analyzed only data on pathological nodal status, and we could not assess the prognosis of patients with NSCLC.
Conclusions
We demonstrated that an AI model without access to information from PET or contrast-enhanced CT could predict preoperative lymph node status with relatively high performance. Surgeons can assess the lymph node status by confirming the presence AI model score and PET or contrast-enhanced CT features, while also considering the range of lymph node metastasis in anatomical lung resection.
Acknowledgments
Funding: None.
Footnote
Reporting Checklist: The authors have completed the TRIPOD reporting checklist. Available at https://jtd.amegroups.com/article/view/10.21037/jtd-24-1067/rc
Data Sharing Statement: Available at https://jtd.amegroups.com/article/view/10.21037/jtd-24-1067/dss
Peer Review File: Available at https://jtd.amegroups.com/article/view/10.21037/jtd-24-1067/prf
Conflicts of Interest: All authors have completed the ICMJE uniform disclosure form (available at https://jtd.amegroups.com/article/view/10.21037/jtd-24-1067/coif). The authors have no conflicts of interest to declare.
Ethical Statement: The authors are accountable for all aspects of the work in ensuring that questions related to the accuracy or integrity of any part of the work are appropriately investigated and resolved. All study protocols were approved by the institutional review board of Iwate Medical University (No. MH2021-061). The present study was conducted in accordance with the Declaration of Helsinki (as revised in 2013). Individual consent for this retrospective analysis was waived.
Open Access Statement: This is an Open Access article distributed in accordance with the Creative Commons Attribution-NonCommercial-NoDerivs 4.0 International License (CC BY-NC-ND 4.0), which permits the non-commercial replication and distribution of the article with the strict proviso that no changes or edits are made and the original work is properly cited (including links to both the formal publication through the relevant DOI and the license). See: https://creativecommons.org/licenses/by-nc-nd/4.0/.
References
- Sekine I, Shintani Y, Shukuya T, et al. A Japanese lung cancer registry study on demographics and treatment modalities in medically treated patients. Cancer Sci 2020;111:1685-91. [Crossref] [PubMed]
- D'Cunha J, Herndon JE 2nd, Herzan DL, et al. Poor correspondence between clinical and pathologic staging in stage 1 non-small cell lung cancer: results from CALGB 9761, a prospective trial. Lung Cancer 2005;48:241-6. [Crossref] [PubMed]
- Veeramachaneni NK, Battafarano RJ, Meyers BF, et al. Risk factors for occult nodal metastasis in clinical T1N0 lung cancer: a negative impact on survival. Eur J Cardiothorac Surg 2008;33:466-9. [Crossref] [PubMed]
- Park HK, Jeon K, Koh WJ, et al. Occult nodal metastasis in patients with non-small cell lung cancer at clinical stage IA by PET/CT. Respirology 2010;15:1179-84. [Crossref] [PubMed]
- Li L, Ren S, Zhang Y, et al. Risk factors for predicting the occult nodal metastasis in T1-2N0M0 NSCLC patients staged by PET/CT: potential value in the clinic. Lung Cancer 2013;81:213-7. [Crossref] [PubMed]
- Cho S, Song IH, Yang HC, et al. Predictive factors for node metastasis in patients with clinical stage I non-small cell lung cancer. Ann Thorac Surg 2013;96:239-45. [Crossref] [PubMed]
- Birim O, Kappetein AP, Stijnen T, et al. Meta-analysis of positron emission tomographic and computed tomographic imaging in detecting mediastinal lymph node metastasis in nonsmall cell lung cancer. Ann Thorac Surg 2005;79:375-82. [Crossref] [PubMed]
- Schmidt-Hansen M, Baldwin DR, Hasler E, et al. PET-CT for assessing mediastinal lymph node involvement in patients with suspected resectable non-small cell lung cancer. Cochrane Database Syst Rev 2014;2014:CD009519. [Crossref] [PubMed]
- International Early Lung Cancer Action Program Investigators. Survival of patients with stage I lung cancer detected on CT screening. N Engl J Med 2006;355:1763-71. [Crossref] [PubMed]
- Zhang Y, Fu F, Wen Z, et al. Segment Location and Ground Glass Opacity Ratio Reliably Predict Node-Negative Status in Lung Cancer. Ann Thorac Surg 2020;109:1061-8. [Crossref] [PubMed]
- Moon Y, Kim KS, Lee KY, et al. Clinicopathologic Factors Associated With Occult Lymph Node Metastasis in Patients With Clinically Diagnosed N0 Lung Adenocarcinoma. Ann Thorac Surg 2016;101:1928-35. [Crossref] [PubMed]
- Moon Y, Park JK, Lee KY, et al. Consolidation/Tumor Ratio on Chest Computed Tomography as Predictor of Postoperative Nodal Upstaging in Clinical T1N0 Lung Cancer. World J Surg 2018;42:2872-8. [Crossref] [PubMed]
- Kudo SE, Ichimasa K, Villard B, et al. Artificial Intelligence System to Determine Risk of T1 Colorectal Cancer Metastasis to Lymph Node. Gastroenterology 2021;160:1075-1084.e2. [Crossref] [PubMed]
- Feng M, Zhang J, Zhou X, et al. Application of an Interpretable Machine Learning Model to Predict Lymph Node Metastasis in Patients with Laryngeal Carcinoma. J Oncol 2022;2022:6356399. [Crossref] [PubMed]
- Kawaguchi Y, Matsuura Y, Kondo Y, et al. The predictive power of artificial intelligence on mediastinal lymphnode metastasis. Gen Thorac Cardiovasc Surg 2021;69:1545-52. [Crossref] [PubMed]
- Shimada Y, Kudo Y, Maehara S, et al. Artificial intelligence-based radiomics for the prediction of nodal metastasis in early-stage lung cancer. Sci Rep 2023;13:1028. [Crossref] [PubMed]
- Brierley JD, Gospodarowicz MK, Wittekind C, editors. Union for International Cancer Control (UICC) TNM Classfication of Malignant Tumors (8th edition). New York Wiley-Blackwell, 2017.
- Travis WD, Brambilla E, Nicholson AG, et al. WHO Classification of Tumors of the Lung, Pleura, Thymus and Heart. 4th ed. International Agency for Research on Cancer, Lyon, 2015.
- Rusch VW, Asamura H, Watanabe H, et al. The IASLC lung cancer staging project: a proposal for a new international lymph node map in the forthcoming seventh edition of the TNM classification for lung cancer. J Thorac Oncol 2009;4:568-77.
- Suzuki K, Koike T, Asakawa T, et al. A prospective radiological study of thin-section computed tomography to predict pathological noninvasiveness in peripheral clinical IA lung cancer (Japan Clinical Oncology Group 0201). J Thorac Oncol 2011;6:751-6. [Crossref] [PubMed]
- Hanley JA, McNeil BJ. The meaning and use of the area under a receiver operating characteristic (ROC) curve. Radiology 1982;143:29-36. [Crossref] [PubMed]
- Tomoyasu M, Deguchi H, Kudo S, et al. Evaluation of pulmonary artery bleeding during thoracoscopic pulmonary resection for lung cancer. Thorac Cancer 2022;13:3001-6. [Crossref] [PubMed]
- Wu Q, Deng L, Jiang Y, et al. Application of the Machine-Learning Model to Improve Prediction of Non-Sentinel Lymph Node Metastasis Status Among Breast Cancer Patients. Front Surg 2022;9:797377. [Crossref] [PubMed]
- Feng JW, Ye J, Qi GF, et al. LASSO-based machine learning models for the prediction of central lymph node metastasis in clinically negative patients with papillary thyroid carcinoma. Front Endocrinol (Lausanne) 2022;13:1030045. [Crossref] [PubMed]
- Li W, Liu Y, Liu W, et al. Machine Learning-Based Prediction of Lymph Node Metastasis Among Osteosarcoma Patients. Front Oncol 2022;12:797103. [Crossref] [PubMed]
- Guinde J, Bourdages-Pageau E, Collin-Castonguay MM, et al. A Prediction Model to Optimize Invasive Mediastinal Staging Procedures for Non-small Cell Lung Cancer in Patients With a Radiologically Normal Mediastinum: The Quebec Prediction Model. Chest 2021;160:2283-92. [Crossref] [PubMed]
- Saji H, Okada M, Tsuboi M, et al. Segmentectomy versus lobectomy in small-sized peripheral non-small-cell lung cancer (JCOG0802/WJOG4607L): a multicentre, open-label, phase 3, randomized, controlled, non-inferiority trial. Lancet 2022;399:1607-17. [Crossref] [PubMed]
- Watanabe S, Suzuki K, Asamura H. Superior and basal segment lung cancers in the lower lobe have different lymph node metastatic pathways and prognosis. Ann Thorac Surg 2008;85:1026-31. [Crossref] [PubMed]
- Handa Y, Tsutani Y, Tsubokawa N, et al. Prognosis of Superior Versus Basal Segment Stage I Non-small Cell Lung Cancer. Ann Thorac Surg 2017;104:1896-901. [Crossref] [PubMed]