Precise diagnosis and prognosis assessment of malignant lung nodules: a narrative review
Introduction
Pulmonary nodules (PNs) are mainly peripheral solitary or multiple small (≤3 cm in diameter) focal radiographic opacities, which are surrounded by lung parenchyma (1). With the widespread application of low-dose computed tomography (LDCT) in daily clinical practice, the number of solitary nodules detected has significantly increased (2-4).
Malignant lung nodules account for only 5% of all lung nodules, and their management differs significantly from that of benign lung nodules (5-7). Beyond the diagnosis of malignant PNs, there is heterogeneity in prognosis, and standard care may lead to undertreatment in high-risk patients and transitional treatment in low-risk patients with malignant lung nodules. Therefore, accurately distinguishing between benign and malignant PNs, as well as precise risk stratification of malignant PNs, is a critical clinical problem that must be solved urgently.
This review focused on the current progress in the precise diagnosis of malignant PNs in primary lung cancer, to improve clinical decision-making, especially regarding early diagnostics. We also estimated the potential prognosis for patients with malignant diagnoses (Figure 1). This strategy will assist with identification of individuals who might benefit from postoperative multimodal therapy. We present this article in accordance with the Narrative Review reporting checklist (available at https://jtd.amegroups.com/article/view/10.21037/jtd-24-1058/rc).
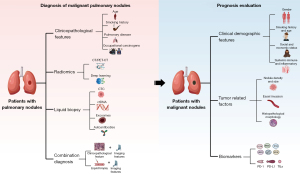
Methods
Primarily, a literature search was conducted in the databases of PubMed and Web of Science for research related to the diagnosis and prognosis of PNs, without setting any restrictions on publication year or study design; the search strategy is shown in Table 1.
Table 1
Items | Specification |
---|---|
Date of search | 3 December 2022 to 15 January 2023 |
Databases and other sources searched | PubMed and Web of Science databases |
Search terms used | MeSH: Solitary Pulmonary Nodule |
Free text search terms: lung nodule and diagnosis; lung nodule and prognostic; lung nodule and prediction; lung nodule and liquid biopsy | |
Timeframe | 1 January 1990 to 15 January 2023 |
Inclusion criteria | English-language literature, without limitation regarding the type of research |
Selection process | The literature search screening process was conducted by M.W., Q.Z., Y.Z., Y.T., J.Y., and S.X. at the same time, and after the initial screening was completed, the final screening was conducted by the six people jointly |
Identification of benign and malignant lung nodules
Classical clinicopathological factors
Classical clinicopathological factors play an important role in distinguishing between benign and malignant nodules. Older adults with PNs have a higher probability of being diagnosed with malignancy, whereas lung cancer is still relatively rare in individuals younger than 35 years and is unusual before the age of 40 years. For each additional decade of life, lung cancer incidence increases steadily (4). Smoking is the main risk factor for lung cancer, with smokers being 10–35 times more likely to have lung cancer than non-smokers, and secondhand smoke is also considered a risk factor (8). Quitting smoking after diagnosis can improve the overall survival (OS) rate and progression-free survival (PFS) time of smokers with early-stage lung cancer (9). The proportion of nonmalignant and malignant causes of death has been shown to be significantly higher in patients with primary resection of stage I non-small cell lung cancer (NSCLC) who smoked for more than 30 years. In addition, the progression of malignant tumors in patients with a smoking history of ≥30 pack-years is higher than that in patients with a history of less than 30 pack-years (10). Therefore, the biological malignancy of the primary lesions and the decrease of airway immune function may be the reasons for the high recurrence rate and poor prognosis of heavy smokers. A study showed that emphysema was an independent risk factor for lung cancer (11). Analysis of the prevalence of lung cancer and emphysema in the National Lung Screening Trial (NLST) showed that the incidence of cancer in patients with emphysema was higher than that in patients without emphysema (25 cancer patients per 1,000 screened emphysema patients vs. 7.5 cancer patients per 1,000 non-emphysema patients screened) (12). The risk of a malignant tumor in patients with pulmonary fibrosis is 4.2 times higher than that in those with emphysema alone, especially idiopathic pulmonary fibrosis, which is also an independent risk factor (13). In addition, chronic obstructive pulmonary disease, exposure to occupational carcinogens (asbestos, cadmium, arsenic, chromium, radon, silica, and kerosene fumes), previous cancer history, and family history of lung cancer also increase the probability of a PN being malignant (14-18).
Radiomics
Radiographic features based on computed tomography (CT) images make a significant contribution to differentiating benign and malignant PNs. The general radiological characteristics in CT imaging of malignant nodules include solid component, margin (clear, blurred), lobulation, speculation, bubble lucency, honeycomb sign, changes in the vessels and pleural indentation signs, and bronchial aeration signs (19,20). The solid component of ground-glass nodules (GGNs) is a critical determinant in predicting their malignancy (21-23). A single-institution study with a follow-up period of 15 years revealed that pure GGNs without solid components are predominantly histologically classified as preinvasive subtypes. In contrast, nodules with a consolidation-to-tumor ratio exceeding 25% are primarily characterized histologically as invasive adenocarcinomas (24). In a prospective multi-institutional cohort study, solid components greater than 2 mm on mediastinal windows were found to be highly indicative of invasive adenocarcinoma (25).
Nodule size is correlated with the risk of malignancy (26). Gierada et al. reported that 598 PNs were diagnosed as lung cancer in the 3-year NLST screening program, of which 70.5% of nodules were larger than 2 cm in diameter and only 15% were less than 1 cm in diameter (27). Recent studies have reported that the malignant tumor probability is 1% for nodules with a diameter less than 6 mm, 1–2% for nodules 6–8 mm in diameter, and over 3% for nodules larger than 8 mm in diameter (26). Part-solid lung nodules are managed according to the size of the solid components. The larger the solid components, the higher the risk of malignancy. When the ground-glass PNs persist for more than 3 months and the diameter is more than 10 mm, the probability of malignancy is 10–50% (28).
Positron emission tomography-computed tomography (PET-CT) imaging is widely used as a noninvasive test to evaluate lung nodules suspicious for cancer. The level of fluorodeoxyglucose (FDG) uptake could also be used to predict patient survival, and FDG uptake is mainly measured according to the maximum standardized uptake value (SUV). A higher maximum SUV (SUVmax) is associated with a higher metabolic rate and a higher degree of malignancy (29). A pooled analysis of 1,330 patients demonstrated that integrated PET/CT imaging provided a sensitivity of 98.7% and a specificity of 58.2% (30). Kim et al. reported that PET-CT performed well in distinguishing benign from malignant for solitary PNs, with a sensitivity of 97% and specificity of 85% (31). Lung magnetic resonance imaging (MRI) is an emerging modality in the early detection of lung cancer, but the major setback of lung MRI in comparison with CT is the detection of subcentimeter nodules (32).
Diagnostic models combined with multiple imaging features can significantly improve accuracy and sensitivity. The Mayo Clinic Model is a well-recognized predictive model that comprises clinicodemographic data of age, cigarette smoking status, and history of extra-thoracic malignant neoplasm and chest radiological data of diameter, location, and edge characteristics (e.g., lobulation, speculation, and shagginess). The model has strong distinguishing ability [area under the curve (AUC) was 0.833 in the developmental data set and 0.801 in the validation data set]. The VA, Brock, and Herder models have been established based on the Mayo Clinic Model (33-36). Al-Ameri et al. verified four such models in a population of patients with lung nodules in the UK, and the Mayo and Brock models had good accuracy in finding the possibility of malignancy nodules on CT scans (37). Among patients who received FDG PET-CT for nodule evaluation, the model that incorporated FDG avidity described by Herder et al. had the highest accuracy (36,37). Risk models use the growing amount of diagnostic information to create more accurate risk assessment of malignancy tumors (38-40). Soardi et al. developed the Bayesian Inference Malignancy Calculator (BIMC) model, including age, smoking, personal history of cancer, nodule diameter, edges and location, volume doubling time, minimum lesion density, contraction-enhanced CT enhancement and FDG uptake; the AUC of the BIMC model was 0.893 in an internal validation (41).
Deep learning (DL) has been proposed as a promising tool for the classification of malignant nodules (42-44). Ardila et al. used a DL algorithm to predict the malignant probability of lung nodules using current and previous CT volumes, and the result showed excellent performance (AUC of 94.4%) in 6,716 NLST cases (45). DL was shown to be useful in CT scans from the Lung Image Database Consortium and Image Database Resource Initiative (LIDC-IDRI), with an accuracy of 97.6% (46). Heuvelmans et al. retrospectively validated the Lung Cancer Prediction Conditional Neural Network, which showed excellent performance in the identification of benign lung nodules; the overall AUC was 94.5% (47).
Solid biopsy
The relationship between imaging characteristics and the nature of PNs is not always straightforward; the same type of PNs may present with varying imaging features, whereas different nodules can exhibit similar imaging appearances. Consequently, a broader range of methods is employed to assess the nature of PNs. In recent years, the integration of advanced imaging techniques with biopsy methods has significantly enhanced the role of lung biopsy in the qualitative diagnosis of PNs.
Percutaneous lung biopsy involves the precise localization of nodules using CT or ultrasound imaging, measuring the size of the lesion, the distance from the skin, and the angle of needle insertion. A needle with a diameter of approximately 14 mm is then carefully guided into the lesion, allowing for accurate sampling of pathological tissue. This technique boasts several advantages, including high accuracy, minimal invasiveness, and rapid recovery, as it enables direct sampling from the lesion site, thereby avoiding more invasive diagnostic methods such as thoracotomy. However, it is important to note that this procedure carries some risk of complications, including bleeding and pneumothorax.
In addition to percutaneous lung biopsy, endobronchial ultrasound-guided transbronchial needle aspiration (EBUS-TBNA) can also assist in determining the benign or malignant nature of PNs. EBUS-TBNA allows for real-time ultrasound-guided biopsy of mediastinal lesions and lymph nodes outside the large airways, making it an efficient and minimally invasive diagnostic tool. This technique is characterized by high diagnostic accuracy and a low incidence of complications, leading to its widespread application in the diagnosis and staging of lung cancer (48,49). Compared to CT-guided percutaneous lung biopsy, which is also a minimally invasive sampling method, EBUS-TBNA offers distinct advantages in tumor staging and facilitates repeated sampling with fewer complications (50).
Liquid biopsy
Liquid biopsy can be utilized for the diagnosis and treatment of various cancers, including lung cancer, breast cancer, and prostate cancer, enabling non-invasive real-time monitoring of disease progression. The most promising applications of liquid biopsy are screening and early diagnosis, which helps to improve the survival rate and reduce the disease burden. Currently, liquid biopsies mainly include measurements of circulating tumor cells (CTCs), circulating tumor DNA (ctDNA), exosomes, and autoantibodies (AABs) (51,52).
CTCs are cancer cells that circulate in the blood after naturally shedding from original or metastatic tumors. As an important biomarker of liquid biopsy, CTCs have good application prospects in the diagnosis, prognostic prediction, and monitoring of lung cancer (52,53). The enrichment techniques of CTC include immunomagnetic positive/negative enrichment, microfluidic immunocapture, and membrane filtration (54). In recent years, there has been a great improvement in the diagnosis of benign and malignant PNs using CTC. CTCs captured by folic acid receptors and CTC measurement using the ScreenCell Cyto filtration system (ScreenCell, Paris, France) are highly effective for lung cancer diagnosis, with sensitivities of 85.05% and 70.1%, respectively (55,56). CTC isolation using the CellCollector (GILUPI, Potsdam, Germany) in vivo detection method may also be effective for distinguishing between benign and malignant nodules (56). Furthermore, the size of PNs is correlated with the quantity of CTCs. Research has indicated that larger tumors tend to shed more tumor cells into the bloodstream. Specifically, PNs with greater diameter and volume are more likely to yield detectable levels of CTCs in the blood (57).
ctDNA is a small nucleic acid released by apoptotic or necrotic tumor cells. It represents tumor cell genes encoded by circulating free DNA (cfDNA) and serves as a valuable indicator of epigenetic alterations. Plasma has been reported to be a good source of ctDNA (58). However, the main limitation of ctDNA is its relatively low sensitivity when tumor volume or burden is minimal. However, as PNs increase in size and tumor stage advances, the sensitivity of ctDNA detection improves significantly: 45% for stage I, 72% for stage II, 75% for stage III, and 83% for stage IV tumors (59,60). To overcome the limitation of sensitivity of more standard methods, some studies have applied personalized cancer profiling by deep sequencing, tagged AMplicon deep sequencing, Safe-sequencing, and Duplex sequencing for the early diagnosis of lung cancer, but this still resulted in low sensitivity (50%) (61). However, integrated digital error suppression has improved the sensitivity of cancer personalized profiling by deep sequencing (CAPP-Seq). Moreover, methylated promoter detection in the plasma has been shown to be more accurate in the diagnosis of early-stage lung cancer (62-65). Liang et al. combined ctDNA and DNA methylation to distinguish between lung cancer and benign nodules, and the sensitivity in stage IA was 75% (66). Ooki et al. used methylation-specific polymerase chain reaction (PCR) to detect six genes selected in The Cancer Genome Atlas (TCGA) dataset and showed that the sensitivity is 72.1% in stage IA adenocarcinoma (67). Hulbert et al. also detected another six genes identified from TCGA dataset, the results showed that the sensitivity was 65–76% and the specificity was 74–84% (68).
Exosomes are 30–100 nm extracellular vesicles which are composed of nucleotides and proteins secreted by most cell types and abundantly present in body fluids (69). Exosomes are considered the most potential biomarkers for diagnosing early lung cancer. Jin et al. found that exosomal microRNA (miRNA) profiles in plasma could identify stage I NSCLC, with a sensitivity of 80.253%, specificity of 92.3%, and an AUC of 0.899 (70). They also found four adenocarcinoma-specific exosomal miRNAs (miR-181-5p, miR30a-3p, miR-30e-3p, and miR-361-5p) and three squamous cell carcinoma (SCC)-specific exosome miRNAs (miR-10b-5p, miR-15b-5p, and miR320b) with promising diagnostic power to distinguish adenocarcinoma from SCC (AUC values of adenocarcinomas and SCC were 0.936 and 0.911, respectively) (70). A systematic meta-analysis revealed that miRNAs still play an important role in the diagnostic of early-stage lung cancer, with a sensitivity and specificity of 80% and 81%, respectively (71). Bianchi et al. studied the potential role of circulating miRNAs in the serum of high-risk individuals, and a panel of 34-miRNAs (miR-test) showed that the diagnostic accuracy was 80% in asymptomatic high-risk patients with early-stage NSCLC (72). Li et al. proposed that exo-growth arrest-specific transcript 5 could be used to identify patients with stage I NSCLC, with an AUC value of 0.822 (73).
AABs are produced by the abnormal exposure or presentation of tumor-associated antigens to promote autoimmune reactions. The relevant antigen produced during tumorigenesis is recognized by the immune system, which in turn produces tumor-related AABs that can be used as tumor markers to assist in the diagnosis of lung cancer (74,75). Measurement of AABs in serum can be a less invasive, long-term, and stable strategy for the early detection of lung cancer (76). Lastwika et al. reported that four AABs (FCGR2A, EPB41L3, LINGO1, and S100A7L2) were detected using high-density protein arrays, with an AUC of 0.78 in indeterminate PNs (77). Wang et al. found that seven AAB (7-AAB) panel tests had significantly higher predictive capability for malignant nodules than radiological modalities. The test had 90.2% sensitivity and 92.7% positive predictive value (PPV) (78). AABs are more effective when combined with traditional methods. Ling et al. found that the 7-AAB panel combined with the Mayo model were more reliable in early detection of lung cancer than the 7-AAB panel alone or the Mayo alone, with a sensitivity of 93.5% (79). Leidinger et al. reported that an AAB spectrum, consisting of 1,827 integer intensity values ranging from 0 to 255, could distinguish between patients from controls without any lung disease, with a specificity of 97.6%, sensitivity of 75.9%, and an accuracy of 92.9% in stage IA/IB tumors (80).
Combined diagnosis
The combination of classical diagnostic techniques and liquid biopsy can greatly improve the sensitivity and accuracy of distinguishing benign nodules from malignant nodules (81,82). Yang et al. developed a patient risk model and a nodule risk model based on clinical information, risk factors, and LDCT that exhibited high efficacy in classification. The AUC was 0.704 in the training dataset and 0.719 in the validation dataset. For the nodule risk model, the AUC of the training dataset was 0.915, and that of the validation dataset was 0.584 (83). The combination of AABs with CT could improve diagnostic performance, with high specificity (>92%) and PPV (>70%) (84). Lin et al. showed that the combination of plasma biomarkers and radiological features could more accurately identify lung cancer, with a sensitivity and specificity of 89.9% and 90.9%, respectively (85). Xi et al. established a prediction model for early-stage NSCLC by 3 miRNAs (miRNA-146a, -200b, and -7) and CT features (pleural indentation and speculation); the model had 92.9% sensitivity and 83.3% specificity in the training set (86).
Recent advancements in the diagnostic methods for PNs have been notable. However, multiple studies indicate that only 10–25% of pure GGNs exhibit enlargement or progression upon follow-up. Additionally, just 40% of part-solid nodules show an increase in size or solid component during monitoring (87,88). These findings suggest that not all PNs necessitate intervention or surgical treatment. Current guidelines recommend surgical intervention under specific criteria: (I) persistent pure GGNs with a diameter of ≥15 mm, solid nodules with a diameter of ≥8 mm, or persistent part-solid nodules with a solid component of ≥5 mm that are highly suspected of malignancy; and (II) stability or growth after dynamic follow-up, defined as an increase of ≥2 mm in the maximum diameter of the nodule or the maximum diameter of the solid component (26).
Prognosis evaluation of malignant lung nodules
Approximately 4% of PNs are malignant, and most malignant PNs (peripheral) are relatively in the early stage of disease (stage I or stage IA) (28). The Lung Cancer Study Group (LCSG) trial established that lobectomy with systematic lymph node dissection or lymphatic sampling without adjuvant therapy is the standard treatment for stage IA lung cancer (89). However, the prognosis of stage IA lung cancer is heterogenous. Some high-risk patients have poor biological performance, a high risk of recurrence and metastasis, and poor prognosis and may thus require active clinical intervention. Some low-risk individuals have indolent biological characteristics, excellent prognosis, and low risk of metastasis and recurrence, and radical surgical treatment may not be required. Downgrading surgical resection and the intensity of clinical treatment will not decrease prognosis. Therefore, an accurate evaluation of the prognosis of stage IA lung cancer is key to risk stratification and precision medicine.
Demographic and clinical prognostication
Clinicodemographic features, including sex, age, smoking history, economic status, and systemic immune status, are all significant independent risk prognostic factors for early lung cancer. The JCOG0201 study showed that age >65 years was a potential risk factor for recurrence-free survival (RFS) in stage IA NSCLC (90). Smoking pack-years is also an important clinical prognostic factor to evaluate the overall long-term survival of patients with stage I primary resection of NSCLC (91). The risk of cancer development and mortality has been shown to be significantly lower in patients who stop smoking than in those who continue to smoke (92,93). Ou et al. reported that old age, male gender, non-surgical treatment, and poor histological grade were associated with an increased mortality risk. Meanwhile, bronchioloalveolar carcinoma and Asian race were associated with a reduced risk of death from stage I NSCLC (94). Lower socioeconomic status, racial and ethnic disparities are also prognostic factors in patients with early-stage lung cancer. The indexes of systemic immunity and inflammation reflect the state of autoimmune function and are related to the occurrence, proliferation, angiogenesis, and metastasis of tumors, which are of great significance to the prognosis of stage I NSCLC (95,96). Takahashi et al. showed that a high preoperative neutrophil-to-lymphocyte ratio (NLR) was an important predictor of poor prognosis, which was related to more frequent distant metastases in patients with completely resected stage I NSCLC (97). Platelets play important roles in blood clotting, fibrinolysis, inflammatory responses, and neoplasia, and also significantly impact the growth, survival, and metastasis of tumor cells. A meta-analysis showed that high NLR, high platelet/lymphocyte ratio, and low lymphocyte/monocyte ratio were significant predictors of poor prognosis (98,99).
Tumor-related factors
Nodule characteristics, such as size, density, and pathological subtypes, influence prognosis. Tumor size is an important indicator of prognosis in patients with early-stage lung cancer. Koike et al. retrospectively evaluated 3,315 patients with stage I disease who underwent complete surgical resection and found that tumor size was an independent prognostic factor for postoperative survival (100). A study including 255 patients with stage IA NSCLC who underwent surgical resection showed that solid tumor volume was an independent influencing factor of disease-free survival (DFS), and the evaluation of tumor volume was more accurate than cross-sectional measurements (101). Tumor size has a marked impact on lymph node metastasis; the larger the tumor diameter, the higher the probability of lymph node metastasis (102,103). A meta-analysis showed that tegafur-uracil is associated with significantly better survival than surgery alone in patients with stage IA (T>2, ≤3 cm) NSCLC (104). Sensitivity to chemotherapy increased as the tumor size increased (105). Rami-porta et al. found that tumor size ranging from 1 cm to 5 cm is associated with prognosis, with each centimeter increase being associated with a significantly different prognosis (106). Therefore, according to the primary tumor size, the 8th edition of the tumor-node-metastasis (TNM) staging system of lung cancer was subdivided into T1a (≤1 cm), T1b (>1, ≤2 cm), and T1c (>2, ≤3 cm).
Nodule density is also crucial for prognosis. PNs are often divided into three categories: pure solid nodules, pure GGNs, and partially solid nodules (PSNs) (107). The risk of prognosis in solid nodules is significantly higher than that in PSNs and pure GGNs. Meanwhile, pure GGNs have an excellent prognosis (108). Ye et al. retrospectively evaluated 1,212 patients with stage IA lung adenocarcinoma (LUAD) and divided them into the GGN, PSN, and pure solid nodule groups. The 5-year NSCLC-related RFS rates were 99.43%, 91.74%, and 58.08% (P<0.0001), and the 5-year NSCLC-related OS were 100%, 98.13%, and 80.27% (P<0.0001), respectively (109). Consolidation tumor ratio (CTR) was also reported to be a significant prognostic factor in early-stage NSCLC (108,110). Matsunaga et al. demonstrated that the prevalence of lymph node metastasis increased significantly with increasing CTR, and CTR was an independent prognostic factor for DFS (111). Yip et al. found that both DFS and OS were higher for category PSNs <80% than for category PSNs ≥80% (112). Therefore, in the International Association for Lung Cancer TNM staging system 8th edition suggested that clinical T staging should be based on the solid tumor size rather than on the whole size of these lesions. However, PSNs with ground-glass components are a special type of tumor. Hattori et al. evaluated 1,181 surgical resection clinical N0 M0 NSCLC patients and found that maximum tumor size and CTR were not correlated with poor OS in partial solid lung cancer on imaging (113). Therefore, the estimation of prognosis for early-stage NSCLC based on solid components remains controversial.
The combination of tumor size and nodule density is an important factor for prognostic estimation. In a prospective multi-institution study (JCOG0201), the 10-year RFS of ground-glass opacities (GGOs) patients with CTR ≤0.25 and tumor length ≤2 cm was 94.1% (114). Lesions typically showing pure GGN measuring ≤3 cm are classified as Tis and part-solid nodules with a solid component measuring ≤0.5 cm as T1mi. These patients have a 100% or near 100% DFS if the lesion is completely resected (115). The JCOG0804 trial also confirmed that patients with stage IA LUAD with T ≤2 cm and CTR ≤0.25 could benefit from sublobular treatment (mainly wedge-shaped) (116). The JCOG0802 trial showed that segment resection was better for stage IA LUAD with T ≤2 cm and CTR >0.5, ≤1 (117). Based on these results, localized resection can achieve an OS equivalent to or even more beneficial than standard lobectomy in patients with tumor size ≤2 cm, especially those with predominant GGO LUAD, with RFS, and the surgical method can be directly determined based on imaging features.
Furthermore, histological subtype significantly affects the prognosis of stage IA lung cancer. Micropapillary- and solid-predominant adenocarcinomas have a significantly higher risk of recurrence than other types (118-120). Tumor spread through air spaces (STAS) of tumor cells is closely related to the prognosis of stage I NSCLC patients, and STAS is an important risk factor for recurrence after limited resection in early-stage lung cancer (121,122). In addition, lymph vascular invasion, vessel invasion, and poor tumor differentiation are risk factors for recurrence and poor prognosis in stage IA NSCLC (123-126). Studies have reported that lepidic-predominant LUAD has favorable prognosis. Meanwhile, patients with micropapillary or solid components are correlated with lymph node metastasis, and adjuvant chemotherapy may be beneficial in micropapillary patients with stage IA (127-129). Hence, due to the requirement of the main features of lepidic growth and the lack of vascular, pleural, or air space invasion to classify a tumor as minimally invasive adenocarcinoma, it is possible that a tumor measuring ≤5 mm would be categorized as invasive adenocarcinoma and therefore be T1a, but not T1mi. Visceral pleural invasion was classified as T2 in the 8th edition of the TNM staging.
Molecular biomarkers
Molecular biomarkers also play an important role in prognosis evaluation in early-stage lung cancer. Izar et al. showed that completely resected stage I epidermal growth factor receptor (EGFR) mutation-positive NSCLC was associated with a significantly lower recurrence rate and longer 5-year DFS and OS than was wild-type EGFR (130). KRAS status is an independent prognostic risk factor; patients with mutations have significantly worse DFS and OS than do patients with KRAS-wild type (131). The positive expression of human epidermal growth factor receptor 2 (HER2) also indicates worse 5-year OS in stage I disease (132). Overexpression of vascular endothelial growth factor is related to poor survival in early-stage NSCLC patients (133). P53 mutations are potential markers of poor prognosis in stage I LUAD patients. Graziano et al. reported that P53 mutations are prevalent in 47% of patients with stage IB NSCLC, and a significant correlation between shorter DFS and OS was observed in the patients with P53 protein expression (134,135). Herbst et al. found that a lower matrix metalloproteinase (MMP)-to-E-cadherin ratio (≤2) was significantly associated with longer OS and lower disease recurrence rates (136). D’Amico et al. found that C-erbb-2 expression is a significant risk factor for tumor recurrence in stage I lung cancer (137). Ruffini et al. retrospectively evaluated 1,290 patients who underwent surgery for primary lung tumor and found that tumor-infiltrating lymphocyte levels were correlated with improved the survival rate of SCCs, especially in early-stage disease (138). The combination of multiple biomarkers will more accurately predict prognosis. Sandoval et al. found that the hypermethylation of five genes (HIST1H4F, PCDHGB6, NPBWR1, ALX1, and HOXA9) was related to shorter RFS and affected the outcome of stage I NSCLC. A signature based on the number of hypermethylated events was used to distinguish between high-risk and low-risk NSCLC patients in stage I (139). A study that investigated 956 patients with stage I LUAD showed that immune markers (FoxP3, FoxP3/CD3, IL-7R, and IL-12Rβ2) were independently associated with recurrence. A meta-analysis suggested that positive programmed death-ligand 1 (PD-L1) expression was related to prominently shorter DFS and significantly worse OS (140). A meta-analysis indicated that PD-L1 expression was related to markedly shorter DFS and significantly worse OS (141).
Perspective
As the prevalence of PNs has significantly increased, it is particularly important to differentially diagnose benign and malignant PNs using minimally invasive or non-invasive methods to avoid overdiagnosis. Liquid biopsy has become an important research topic and a direction for future research in the early diagnosis of lung cancer in recent years. However, liquid biopsy currently does not have clear thresholds and standard operating protocols, and, unlike CT, it cannot assess tumor staging. Despite the great potential of the existing methodologies, there is no single molecular biomarker of lung cancer for routine clinical practice. Further studies are required.
First, biomarkers combined with imaging features may be more effective in the diagnosis of lung cancer, and the criteria for distinguishing benign and malignant nodules should be improved. Minimal residual disease (MRD) monitoring is a promising strategy, and preoperative ctDNA testing to determine patient prognosis has good potential. Second, patients with nodule diameter ≤2 cm, pure adenocarcinoma in situ (AIS) histology, GGO attenuation in >50% of the diameter on CT, or volume doubling time >400 days may be considered for sublobar resection. Meanwhile, non-surgical patients with early-stage NSCLC should be considered for conventional or stereotactic radiotherapy. For patients with early-stage lung cancer with slow-growing disease or those who are not candidates for surgery, noninvasive ablative therapies are necessary. The genomic differences between fast- and slow-growing diseases need to be clarified. The molecular events that mediate disease progression require further investigation.
Studies have suggested that adjuvant targeted therapies could improve PFS in early-stage NSCLC but may not convert to improved OS. The possible reason for the improvement in PFS but not in OS could be a result of tyrosine kinase inhibitors not being able to completely eliminant micrometastatic disease. Neoadjuvant chemotherapy has potential advantages, including improved tolerability, possible staging reduction, and earlier treatment of micrometastases in early-stage NSCLC. In parallel, immune checkpoint therapy has become an indispensable treatment, especially for patients who lack actionable oncogenic drivers. Despite future challenges, the discovery and application of new biomarkers will promote the development of precision medicine and improvement of outcomes in patients with early-stage NSCLC.
This review summarizes the research progress in the clinical management of malignant PNs, but it did not investigate the clinical application and prevalence of various research advances, as a comprehensive investigation of clinical application is not readily feasible.
Conclusions
Clinicopathological features and imaging characteristics play an important role in the diagnosis and prognosis assessment of early lung cancer, but liquid biopsy and artificial intelligence are also promising owing to their convenience and accuracy.
Acknowledgments
We thank Jianfei Zhu and Wenchen Wang for critically editing the manuscript.
Funding: This study was funded by
Footnote
Reporting Checklist: The authors have completed the Narrative Review reporting checklist. Available at https://jtd.amegroups.com/article/view/10.21037/jtd-24-1058/rc
Peer Review File: Available at https://jtd.amegroups.com/article/view/10.21037/jtd-24-1058/prf
Conflicts of Interest: All authors have completed the ICMJE uniform disclosure form (available at https://jtd.amegroups.com/article/view/10.21037/jtd-24-1058/coif). The authors have no conflicts of interest to declare.
Ethical Statement: The authors are accountable for all aspects of the work in ensuring that questions related to the accuracy or integrity of any part of the work are appropriately investigated and resolved.
Open Access Statement: This is an Open Access article distributed in accordance with the Creative Commons Attribution-NonCommercial-NoDerivs 4.0 International License (CC BY-NC-ND 4.0), which permits the non-commercial replication and distribution of the article with the strict proviso that no changes or edits are made and the original work is properly cited (including links to both the formal publication through the relevant DOI and the license). See: https://creativecommons.org/licenses/by-nc-nd/4.0/.
References
- Gould MK, Tang T, Liu IL, et al. Recent Trends in the Identification of Incidental Pulmonary Nodules. Am J Respir Crit Care Med 2015;192:1208-14. [Crossref] [PubMed]
- Oudkerk M, Liu S, Heuvelmans MA, et al. Lung cancer LDCT screening and mortality reduction - evidence, pitfalls and future perspectives. Nat Rev Clin Oncol 2021;18:135-51. [Crossref] [PubMed]
- Horeweg N, van Rosmalen J, Heuvelmans MA, et al. Lung cancer probability in patients with CT-detected pulmonary nodules: a prespecified analysis of data from the NELSON trial of low-dose CT screening. Lancet Oncol 2014;15:1332-41. [Crossref] [PubMed]
- Ettinger DS. Ten years of progress in non-small cell lung cancer. J Natl Compr Canc Netw 2012;10:292-5. [Crossref] [PubMed]
- Wang C, Wu Y, Shao J, et al. Clinicopathological variables influencing overall survival, recurrence and post-recurrence survival in resected stage I non-small-cell lung cancer. BMC Cancer 2020;20:150. [Crossref] [PubMed]
- Ball D, Mai GT, Vinod S, et al. Stereotactic ablative radiotherapy versus standard radiotherapy in stage 1 non-small-cell lung cancer (TROG 09.02 CHISEL): a phase 3, open-label, randomised controlled trial. Lancet Oncol 2019;20:494-503. [Crossref] [PubMed]
- Goldstraw P, Chansky K, Crowley J, et al. The IASLC Lung Cancer Staging Project: Proposals for Revision of the TNM Stage Groupings in the Forthcoming (Eighth) Edition of the TNM Classification for Lung Cancer. J Thorac Oncol 2016;11:39-51. [Crossref] [PubMed]
- Kobayashi Y, Sakao Y, Deshpande GA, et al. The association between baseline clinical-radiological characteristics and growth of pulmonary nodules with ground-glass opacity. Lung Cancer 2014;83:61-6. [Crossref] [PubMed]
- Hoffman RM, Atallah RP, Struble RD, et al. Lung Cancer Screening with Low-Dose CT: a Meta-Analysis. J Gen Intern Med 2020;35:3015-25. [Crossref] [PubMed]
- Hoffman RM, Sanchez R. Lung Cancer Screening. Med Clin North Am 2017;101:769-85. [Crossref] [PubMed]
- Wilson DO, Weissfeld JL, Balkan A, et al. Association of radiographic emphysema and airflow obstruction with lung cancer. Am J Respir Crit Care Med 2008;178:738-44. [Crossref] [PubMed]
- de Torres JP, Bastarrika G, Wisnivesky JP, et al. Assessing the relationship between lung cancer risk and emphysema detected on low-dose CT of the chest. Chest 2007;132:1932-8. [Crossref] [PubMed]
- Kwak N, Park CM, Lee J, et al. Lung cancer risk among patients with combined pulmonary fibrosis and emphysema. Respir Med 2014;108:524-30. [Crossref] [PubMed]
- Wood DE, Kazerooni EA, Baum SL, et al. Lung Cancer Screening, Version 3.2018, NCCN Clinical Practice Guidelines in Oncology. J Natl Compr Canc Netw 2018;16:412-41. [Crossref] [PubMed]
- Ang L, Chan CPY, Yau WP, et al. Association between family history of lung cancer and lung cancer risk: a systematic review and meta-analysis. Lung Cancer 2020;148:129-37. [Crossref] [PubMed]
- Cannon-Albright LA, Carr SR, Akerley W. Population-Based Relative Risks for Lung Cancer Based on Complete Family History of Lung Cancer. J Thorac Oncol 2019;14:1184-91. [Crossref] [PubMed]
- Deng Y, Wang M, Tian T, et al. The Effect of Hexavalent Chromium on the Incidence and Mortality of Human Cancers: A Meta-Analysis Based on Published Epidemiological Cohort Studies. Front Oncol 2019;9:24. [Crossref] [PubMed]
- Park HY, Kang D, Shin SH, et al. Chronic obstructive pulmonary disease and lung cancer incidence in never smokers: a cohort study. Thorax 2020;75:506-9. [Crossref] [PubMed]
- Wilson R, Devaraj A. Radiomics of pulmonary nodules and lung cancer. Transl Lung Cancer Res 2017;6:86-91. [Crossref] [PubMed]
- Liu Y, Balagurunathan Y, Atwater T, et al. Radiological Image Traits Predictive of Cancer Status in Pulmonary Nodules. Clin Cancer Res 2017;23:1442-9. [Crossref] [PubMed]
- Maeyashiki T, Suzuki K, Hattori A, et al. The size of consolidation on thin-section computed tomography is a better predictor of survival than the maximum tumour dimension in resectable lung cancer. Eur J Cardiothorac Surg 2013;43:915-8. [Crossref] [PubMed]
- Murakawa T, Konoeda C, Ito T, et al. The ground glass opacity component can be eliminated from the T-factor assessment of lung adenocarcinoma. Eur J Cardiothorac Surg 2013;43:925-32. [Crossref] [PubMed]
- Tsutani Y, Miyata Y, Mimae T, et al. The prognostic role of pathologic invasive component size, excluding lepidic growth, in stage I lung adenocarcinoma. J Thorac Cardiovasc Surg 2013;146:580-5. [Crossref] [PubMed]
- Sawada S, Yamashita N, Sugimoto R, et al. Long-term Outcomes of Patients With Ground-Glass Opacities Detected Using CT Scanning. Chest 2017;151:308-15. [Crossref] [PubMed]
- Kakinuma R, Noguchi M, Ashizawa K, et al. Natural History of Pulmonary Subsolid Nodules: A Prospective Multicenter Study. J Thorac Oncol 2016;11:1012-28. [Crossref] [PubMed]
- MacMahon H, Naidich DP, Goo JM, et al. Guidelines for Management of Incidental Pulmonary Nodules Detected on CT Images: From the Fleischner Society 2017. Radiology 2017;284:228-43. [Crossref] [PubMed]
- Gierada DS, Pinsky P, Nath H, et al. Projected outcomes using different nodule sizes to define a positive CT lung cancer screening examination. J Natl Cancer Inst 2014;106:dju284. [Crossref] [PubMed]
- Mazzone PJ, Lam L. Evaluating the Patient With a Pulmonary Nodule: A Review. JAMA 2022;327:264-73. [Crossref] [PubMed]
- Hui Z, Wei F, Ren H, et al. Primary tumor standardized uptake value (SUVmax) measured on (18)F-FDG PET/CT and mixed NSCLC components predict survival in surgical-resected combined small-cell lung cancer. J Cancer Res Clin Oncol 2020;146:2595-605. [Crossref] [PubMed]
- Wang HQ, Zhao L, Zhao J, et al. Analysis on early detection of lung cancer by PET/CT scan. Asian Pac J Cancer Prev 2015;16:2215-7. [Crossref] [PubMed]
- Kim SK, Allen-Auerbach M, Goldin J, et al. Accuracy of PET/CT in characterization of solitary pulmonary lesions. J Nucl Med 2007;48:214-20.
- Sim AJ, Kaza E, Singer L, et al. A review of the role of MRI in diagnosis and treatment of early stage lung cancer. Clin Transl Radiat Oncol 2020;24:16-22. [Crossref] [PubMed]
- Swensen SJ, Silverstein MD, Ilstrup DM, et al. The probability of malignancy in solitary pulmonary nodules. Application to small radiologically indeterminate nodules. Arch Intern Med 1997;157:849-55.
- Gould MK, Ananth L, Barnett PG, et al. A clinical model to estimate the pretest probability of lung cancer in patients with solitary pulmonary nodules. Chest 2007;131:383-8. [Crossref] [PubMed]
- McWilliams A, Tammemagi MC, Mayo JR, et al. Probability of cancer in pulmonary nodules detected on first screening CT. N Engl J Med 2013;369:910-9. [Crossref] [PubMed]
- Herder GJ, van Tinteren H, Golding RP, et al. Clinical prediction model to characterize pulmonary nodules: validation and added value of 18F-fluorodeoxyglucose positron emission tomography. Chest 2005;128:2490-6. [Crossref] [PubMed]
- Al-Ameri A, Malhotra P, Thygesen H, et al. Risk of malignancy in pulmonary nodules: A validation study of four prediction models. Lung Cancer 2015;89:27-30. [Crossref] [PubMed]
- Choi W, Oh JH, Riyahi S, et al. Radiomics analysis of pulmonary nodules in low-dose CT for early detection of lung cancer. Med Phys 2018;45:1537-49. [Crossref] [PubMed]
- Liu A, Wang Z, Yang Y, et al. Preoperative diagnosis of malignant pulmonary nodules in lung cancer screening with a radiomics nomogram. Cancer Commun (Lond) 2020;40:16-24. [Crossref] [PubMed]
- Guo HY, Lin JT, Huang HH, et al. Development and Validation of a (18)F-FDG PET/CT-Based Clinical Prediction Model for Estimating Malignancy in Solid Pulmonary Nodules Based on a Population With High Prevalence of Malignancy. Clin Lung Cancer 2020;21:47-55. [Crossref] [PubMed]
- Soardi GA, Perandini S, Motton M, et al. Assessing probability of malignancy in solid solitary pulmonary nodules with a new Bayesian calculator: improving diagnostic accuracy by means of expanded and updated features. Eur Radiol 2015;25:155-62. [Crossref] [PubMed]
- Massion PP, Antic S, Ather S, et al. Assessing the Accuracy of a Deep Learning Method to Risk Stratify Indeterminate Pulmonary Nodules. Am J Respir Crit Care Med 2020;202:241-9. [Crossref] [PubMed]
- Bonavita I, Rafael-Palou X, Ceresa M, et al. Integration of convolutional neural networks for pulmonary nodule malignancy assessment in a lung cancer classification pipeline. Comput Methods Programs Biomed 2020;185:105172. [Crossref] [PubMed]
- Li D, Mikela Vilmun B, Frederik Carlsen J, et al. The Performance of Deep Learning Algorithms on Automatic Pulmonary Nodule Detection and Classification Tested on Different Datasets That Are Not Derived from LIDC-IDRI: A Systematic Review. Diagnostics (Basel) 2019;9:207. [Crossref] [PubMed]
- Ardila D, Kiraly AP, Bharadwaj S, et al. End-to-end lung cancer screening with three-dimensional deep learning on low-dose chest computed tomography. Nat Med 2019;25:954-61. [Crossref] [PubMed]
- da Silva GLF, Valente TLA, Silva AC, et al. Convolutional neural network-based PSO for lung nodule false positive reduction on CT images. Comput Methods Programs Biomed 2018;162:109-18. [Crossref] [PubMed]
- Heuvelmans MA, van Ooijen PMA, Ather S, et al. Lung cancer prediction by Deep Learning to identify benign lung nodules. Lung Cancer 2021;154:1-4. [Crossref] [PubMed]
- Gu P, Zhao YZ, Jiang LY, et al. Endobronchial ultrasound-guided transbronchial needle aspiration for staging of lung cancer: a systematic review and meta-analysis. Eur J Cancer 2009;45:1389-96. [Crossref] [PubMed]
- Varela-Lema L, Fernández-Villar A, Ruano-Ravina A. Effectiveness and safety of endobronchial ultrasound-transbronchial needle aspiration: a systematic review. Eur Respir J 2009;33:1156-64. [Crossref] [PubMed]
- Munoz ML, Lechtzin N, Li QK, et al. Bronchoscopy with endobronchial ultrasound guided transbronchial needle aspiration vs. transthoracic needle aspiration in lung cancer diagnosis and staging. J Thorac Dis 2017;9:2178-85. [Crossref] [PubMed]
- Mattox AK, Bettegowda C, Zhou S, et al. Applications of liquid biopsies for cancer. Sci Transl Med 2019;11:eaay1984.
- Mateo J, Gerlinger M, Rodrigues DN, et al. The promise of circulating tumor cell analysis in cancer management. Genome Biol 2014;15:448. [Crossref] [PubMed]
- Tamminga M, de Wit S, Schuuring E, et al. Circulating tumor cells in lung cancer are prognostic and predictive for worse tumor response in both targeted- and chemotherapy. Transl Lung Cancer Res 2019;8:854-61. [Crossref] [PubMed]
- Rushton AJ, Nteliopoulos G, Shaw JA, et al. A Review of Circulating Tumour Cell Enrichment Technologies. Cancers (Basel) 2021;13:970. [Crossref] [PubMed]
- Yin W, Zhu J, Ma B, et al. Overcoming Obstacles in Pathological Diagnosis of Pulmonary Nodules through Circulating Tumor Cell Enrichment. Small 2020;16:e2001695. [Crossref] [PubMed]
- Mascalchi M, Maddau C, Sali L, et al. Circulating tumor cells and microemboli can differentiate malignant and benign pulmonary lesions. J Cancer 2017;8:2223-30. [Crossref] [PubMed]
- Katoh M, Neumaier M, Nezam R, et al. Correlation of circulating tumor cells with tumor size and metastatic load in a spontaneous lung metastasis model. Anticancer Res 2004;24:1421-5.
- Vallée A, Marcq M, Bizieux A, et al. Plasma is a better source of tumor-derived circulating cell-free DNA than serum for the detection of EGFR alterations in lung tumor patients. Lung Cancer 2013;82:373-4. [Crossref] [PubMed]
- Duffy MJ. Circulating tumor DNA (ctDNA) as a biomarker for lung cancer: Early detection, monitoring and therapy prediction. Tumour Biol 2024;46:S283-95. [Crossref] [PubMed]
- Phallen J, Sausen M, Adleff V, et al. Direct detection of early-stage cancers using circulating tumor DNA. Sci Transl Med 2017;9:eaan2415. [Crossref] [PubMed]
- Newman AM, Bratman SV, To J, et al. An ultrasensitive method for quantitating circulating tumor DNA with broad patient coverage. Nat Med 2014;20:548-54. [Crossref] [PubMed]
- Forshew T, Murtaza M, Parkinson C, et al. Noninvasive identification and monitoring of cancer mutations by targeted deep sequencing of plasma DNA. Sci Transl Med 2012;4:136ra68. [Crossref] [PubMed]
- Kinde I, Wu J, Papadopoulos N, et al. Detection and quantification of rare mutations with massively parallel sequencing. Proc Natl Acad Sci U S A 2011;108:9530-5. [Crossref] [PubMed]
- Kennedy SR, Schmitt MW, Fox EJ, et al. Detecting ultralow-frequency mutations by Duplex Sequencing. Nat Protoc 2014;9:2586-606. [Crossref] [PubMed]
- Newman AM, Lovejoy AF, Klass DM, et al. Integrated digital error suppression for improved detection of circulating tumor DNA. Nat Biotechnol 2016;34:547-55. [Crossref] [PubMed]
- Liang W, Zhao Y, Huang W, et al. Non-invasive diagnosis of early-stage lung cancer using high-throughput targeted DNA methylation sequencing of circulating tumor DNA (ctDNA). Theranostics 2019;9:2056-70. [Crossref] [PubMed]
- Ooki A, Maleki Z, Tsay JJ, et al. A Panel of Novel Detection and Prognostic Methylated DNA Markers in Primary Non-Small Cell Lung Cancer and Serum DNA. Clin Cancer Res 2017;23:7141-52. [Crossref] [PubMed]
- Hulbert A, Jusue-Torres I, Stark A, et al. Early Detection of Lung Cancer Using DNA Promoter Hypermethylation in Plasma and Sputum. Clin Cancer Res 2017;23:1998-2005. [Crossref] [PubMed]
- Liu S, Zhan Y, Luo J, et al. Roles of exosomes in the carcinogenesis and clinical therapy of non-small cell lung cancer. Biomed Pharmacother 2019;111:338-46. [Crossref] [PubMed]
- Jin X, Chen Y, Chen H, et al. Evaluation of Tumor-Derived Exosomal miRNA as Potential Diagnostic Biomarkers for Early-Stage Non-Small Cell Lung Cancer Using Next-Generation Sequencing. Clin Cancer Res 2017;23:5311-9. [Crossref] [PubMed]
- Yang Y, Hu Z, Zhou Y, et al. The clinical use of circulating microRNAs as non-invasive diagnostic biomarkers for lung cancers. Oncotarget 2017;8:90197-214. [Crossref] [PubMed]
- Bianchi F, Nicassio F, Marzi M, et al. A serum circulating miRNA diagnostic test to identify asymptomatic high-risk individuals with early stage lung cancer. EMBO Mol Med 2011;3:495-503. [Crossref] [PubMed]
- Li C, Lv Y, Shao C, et al. Tumor-derived exosomal lncRNA GAS5 as a biomarker for early-stage non-small-cell lung cancer diagnosis. J Cell Physiol 2019;234:20721-7. [Crossref] [PubMed]
- Zaenker P, Gray ES, Ziman MR. Autoantibody Production in Cancer--The Humoral Immune Response toward Autologous Antigens in Cancer Patients. Autoimmun Rev 2016;15:477-83. [Crossref] [PubMed]
- Tan HT, Low J, Lim SG, et al. Serum autoantibodies as biomarkers for early cancer detection. FEBS J 2009;276:6880-904. [Crossref] [PubMed]
- Broodman I, Lindemans J, van Sten J, et al. Serum Protein Markers for the Early Detection of Lung Cancer: A Focus on Autoantibodies. J Proteome Res 2017;16:3-13. [Crossref] [PubMed]
- Lastwika KJ, Kargl J, Zhang Y, et al. Tumor-derived Autoantibodies Identify Malignant Pulmonary Nodules. Am J Respir Crit Care Med 2019;199:1257-66. [Crossref] [PubMed]
- Wang W, Zhuang R, Ma H, et al. The diagnostic value of a seven-autoantibody panel and a nomogram with a scoring table for predicting the risk of non-small-cell lung cancer. Cancer Sci 2020;111:1699-710. [Crossref] [PubMed]
- Ling Z, Chen J, Wen Z, et al. The Value of a Seven-Autoantibody Panel Combined with the Mayo Model in the Differential Diagnosis of Pulmonary Nodules. Dis Markers 2021;2021:6677823. [Crossref] [PubMed]
- Leidinger P, Keller A, Heisel S, et al. Identification of lung cancer with high sensitivity and specificity by blood testing. Respir Res 2010;11:18. [Crossref] [PubMed]
- Horeweg N, van der Aalst CM, Thunnissen E, et al. Characteristics of lung cancers detected by computer tomography screening in the randomized NELSON trial. Am J Respir Crit Care Med 2013;187:848-54. [Crossref] [PubMed]
- Abbosh C, Birkbak NJ, Swanton C. Early stage NSCLC - challenges to implementing ctDNA-based screening and MRD detection. Nat Rev Clin Oncol 2018;15:577-86. [Crossref] [PubMed]
- Yang D, Zhang X, Powell CA, et al. Probability of cancer in high-risk patients predicted by the protein-based lung cancer biomarker panel in China: LCBP study. Cancer 2018;124:262-70. [Crossref] [PubMed]
- Massion PP, Healey GF, Peek LJ, et al. Autoantibody Signature Enhances the Positive Predictive Power of Computed Tomography and Nodule-Based Risk Models for Detection of Lung Cancer. J Thorac Oncol 2017;12:578-84. [Crossref] [PubMed]
- Lin Y, Leng Q, Jiang Z, et al. A classifier integrating plasma biomarkers and radiological characteristics for distinguishing malignant from benign pulmonary nodules. Int J Cancer 2017;141:1240-8. [Crossref] [PubMed]
- Xi K, Wang W, Wen Y, et al. Combining Plasma miRNAs and Computed Tomography Features to Differentiate the Nature of Pulmonary Nodules. Front Oncol 2019;9:975. [Crossref] [PubMed]
- Aoki T. Growth of pure ground-glass lung nodule detected at computed tomography. J Thorac Dis 2015;7:E326-8. [Crossref] [PubMed]
- Kobayashi Y, Mitsudomi T. Management of ground-glass opacities: should all pulmonary lesions with ground-glass opacity be surgically resected? Transl Lung Cancer Res 2013;2:354-63. [Crossref] [PubMed]
- Feld R, Rubinstein L, Thomas PA. Adjuvant chemotherapy with cyclophosphamide, doxorubicin, and cisplatin in patients with completely resected stage I non-small cell lung cancer. An LCSG Trial. Chest 1994;106:307S-9S.
- Tsutani Y, Suzuki K, Koike T, et al. High-Risk Factors for Recurrence of Stage I Lung Adenocarcinoma: Follow-up Data From JCOG0201. Ann Thorac Surg 2019;108:1484-90. [Crossref] [PubMed]
- Fujisawa T, Iizasa T, Saitoh Y, et al. Smoking before surgery predicts poor long-term survival in patients with stage I non-small-cell lung carcinomas. J Clin Oncol 1999;17:2086-91. [Crossref] [PubMed]
- Parsons A, Daley A, Begh R, et al. Influence of smoking cessation after diagnosis of early stage lung cancer on prognosis: systematic review of observational studies with meta-analysis. BMJ 2010;340:b5569. [Crossref] [PubMed]
- Ordóñez-Mena JM, Schöttker B, Mons U, et al. Quantification of the smoking-associated cancer risk with rate advancement periods: meta-analysis of individual participant data from cohorts of the CHANCES consortium. BMC Med. 2016;14:62. [Crossref] [PubMed]
- Ou SH, Zell JA, Ziogas A, et al. Prognostic factors for survival of stage I nonsmall cell lung cancer patients: a population-based analysis of 19,702 stage I patients in the California Cancer Registry from 1989 to 2003. Cancer 2007;110:1532-41. [Crossref] [PubMed]
- Grivennikov SI, Greten FR, Karin M. Immunity, inflammation, and cancer. Cell 2010;140:883-99. [Crossref] [PubMed]
- Huang W, Wang S, Zhang H, et al. Prognostic significance of combined fibrinogen concentration and neutrophil-to-lymphocyte ratio in patients with resectable non-small cell lung cancer. Cancer Biol Med 2018;15:88-96. [Crossref] [PubMed]
- Takahashi Y, Horio H, Hato T, et al. Prognostic Significance of Preoperative Neutrophil-Lymphocyte Ratios in Patients with Stage I Non-small Cell Lung Cancer After Complete Resection. Ann Surg Oncol 2015;22:S1324-31. [Crossref] [PubMed]
- Yang HB, Xing M, Ma LN, et al. Prognostic significance of neutrophil-lymphocyteratio/platelet-lymphocyteratioin lung cancers: a meta-analysis. Oncotarget 2016;7:76769-78. [Crossref] [PubMed]
- Li W, Ma G, Wu Q, et al. Prognostic value of lymphocyte-to-monocyte ratio among Asian lung cancer patients: a systematic review and meta-analysis. Oncotarget 2017;8:110606-13. [Crossref] [PubMed]
- Koike T, Tsuchiya R, Goya T, et al. Prognostic factors in 3315 completely resected cases of clinical stage I non-small cell lung cancer in Japan. J Thorac Oncol 2007;2:408-13. [Crossref] [PubMed]
- Takenaka T, Yamazaki K, Miura N, et al. The Prognostic Impact of Tumor Volume in Patients with Clinical Stage IA Non-Small Cell Lung Cancer. J Thorac Oncol 2016;11:1074-80. [Crossref] [PubMed]
- Ding N, Mao Y, Gao S, et al. Predictors of lymph node metastasis and possible selective lymph node dissection in clinical stage IA non-small cell lung cancer. J Thorac Dis 2018;10:4061-8. [Crossref] [PubMed]
- Yu X, Li Y, Shi C, et al. Risk factors of lymph node metastasis in patients with non-small cell lung cancer ≤ 2 cm in size: A monocentric population-based analysis. Thorac Cancer 2018;9:3-9. [Crossref] [PubMed]
- Hamada C, Tsuboi M, Ohta M, et al. Effect of postoperative adjuvant chemotherapy with tegafur-uracil on survival in patients with stage IA non-small cell lung cancer: an exploratory analysis from a meta-analysis of six randomized controlled trials. J Thorac Oncol 2009;4:1511-6. [Crossref] [PubMed]
- Visbal AL, Leighl NB, Feld R, et al. Adjuvant Chemotherapy for Early-Stage Non-small Cell Lung Cancer. Chest 2005;128:2933-43. [Crossref] [PubMed]
- Rami-Porta R, Bolejack V, Crowley J, et al. The IASLC Lung Cancer Staging Project: Proposals for the Revisions of the T Descriptors in the Forthcoming Eighth Edition of the TNM Classification for Lung Cancer. J Thorac Oncol 2015;10:990-1003.
- Naidich DP, Bankier AA, MacMahon H, et al. Recommendations for the management of subsolid pulmonary nodules detected at CT: a statement from the Fleischner Society. Radiology 2013;266:304-17. [Crossref] [PubMed]
- Hattori A, Matsunaga T, Hayashi T, et al. Prognostic Impact of the Findings on Thin-Section Computed Tomography in Patients with Subcentimeter Non-Small Cell Lung Cancer. J Thorac Oncol 2017;12:954-62. [Crossref] [PubMed]
- Ye T, Deng L, Wang S, et al. Lung Adenocarcinomas Manifesting as Radiological Part-Solid Nodules Define a Special Clinical Subtype. J Thorac Oncol 2019;14:617-27. [Crossref] [PubMed]
- Hattori A, Matsunaga T, Takamochi K, et al. Prognostic impact of a ground glass opacity component in the clinical T classification of non-small cell lung cancer. J Thorac Cardiovasc Surg 2017;154:2102-2110.e1. [Crossref] [PubMed]
- Matsunaga T, Suzuki K, Takamochi K, et al. What is the radiological definition of part-solid tumour in lung cancer?†. Eur J Cardiothorac Surg 2017;51:242-7. [Crossref] [PubMed]
- Yip R, Li K, Liu L, et al. Controversies on lung cancers manifesting as part-solid nodules. Eur Radiol 2018;28:747-59. [Crossref] [PubMed]
- Hattori A, Matsunaga T, Takamochi K, et al. Neither Maximum Tumor Size nor Solid Component Size Is Prognostic in Part-Solid Lung Cancer: Impact of Tumor Size Should Be Applied Exclusively to Solid Lung Cancer. Ann Thorac Surg 2016;102:407-15. [Crossref] [PubMed]
- Suzuki K, Koike T, Asakawa T, et al. A prospective radiological study of thin-section computed tomography to predict pathological noninvasiveness in peripheral clinical IA lung cancer (Japan Clinical Oncology Group 0201). J Thorac Oncol 2011;6:751-6. [Crossref] [PubMed]
- Travis WD, Asamura H, Bankier AA, et al. The IASLC Lung Cancer Staging Project: Proposals for Coding T Categories for Subsolid Nodules and Assessment of Tumor Size in Part-Solid Tumors in the Forthcoming Eighth Edition of the TNM Classification of Lung Cancer. J Thorac Oncol 2016;11:1204-23.
- Suzuki K, Watanabe SI, Wakabayashi M, et al. A single-arm study of sublobar resection for ground-glass opacity dominant peripheral lung cancer. J Thorac Cardiovasc Surg 2022;163:289-301.e2. [Crossref] [PubMed]
- Suzuki K, Saji H, Aokage K, et al. Comparison of pulmonary segmentectomy and lobectomy: Safety results of a randomized trial. J Thorac Cardiovasc Surg 2019;158:895-907. [Crossref] [PubMed]
- Hung JJ, Jeng WJ, Chou TY, et al. Prognostic value of the new International Association for the Study of Lung Cancer/American Thoracic Society/European Respiratory Society lung adenocarcinoma classification on death and recurrence in completely resected stage I lung adenocarcinoma. Ann Surg 2013;258:1079-86. [Crossref] [PubMed]
- Warth A, Muley T, Meister M, et al. The novel histologic International Association for the Study of Lung Cancer/American Thoracic Society/European Respiratory Society classification system of lung adenocarcinoma is a stage-independent predictor of survival. J Clin Oncol 2012;30:1438-46. [Crossref] [PubMed]
- Hung JJ, Yeh YC, Jeng WJ, et al. Predictive value of the international association for the study of lung cancer/American Thoracic Society/European Respiratory Society classification of lung adenocarcinoma in tumor recurrence and patient survival. J Clin Oncol 2014;32:2357-64. [Crossref] [PubMed]
- Kadota K, Nitadori JI, Sima CS, et al. Tumor Spread through Air Spaces is an Important Pattern of Invasion and Impacts the Frequency and Location of Recurrences after Limited Resection for Small Stage I Lung Adenocarcinomas. J Thorac Oncol 2015;10:806-14. [Crossref] [PubMed]
- Masai K, Sakurai H, Sukeda A, et al. Prognostic Impact of Margin Distance and Tumor Spread Through Air Spaces in Limited Resection for Primary Lung Cancer. J Thorac Oncol 2017;12:1788-97. [Crossref] [PubMed]
- Kuo SW, Chen JS, Huang PM, et al. Prognostic significance of histologic differentiation, carcinoembryonic antigen value, and lymphovascular invasion in stage I non-small cell lung cancer. J Thorac Cardiovasc Surg 2014;148:1200-1207.e3. [Crossref] [PubMed]
- Kobayashi N, Toyooka S, Soh J, et al. Risk factors for recurrence and unfavorable prognosis in patients with stage I non-small cell lung cancer and a tumor diameter of 20 mm or less. J Thorac Oncol 2007;2:808-12. [Crossref] [PubMed]
- Tsuchiya T, Akamine S, Muraoka M, et al. Stage IA non-small cell lung cancer: vessel invasion is a poor prognostic factor and a new target of adjuvant chemotherapy. Lung Cancer 2007;56:341-8. [Crossref] [PubMed]
- Noma D, Inamura K, Matsuura Y, et al. Prognostic Effect of Lymphovascular Invasion on TNM Staging in Stage I Non-Small-cell Lung Cancer. Clin Lung Cancer 2018;19:e109-22. [Crossref] [PubMed]
- Sasada S, Miyata Y, Mimae T, et al. Impact of Lepidic Component Occupancy on Effects of Adjuvant Chemotherapy for Lung Adenocarcinoma. Ann Thorac Surg 2015;100:2079-86. [Crossref] [PubMed]
- Qian F, Yang W, Wang R, et al. Prognostic significance and adjuvant chemotherapy survival benefits of a solid or micropapillary pattern in patients with resected stage IB lung adenocarcinoma. J Thorac Cardiovasc Surg 2018;155:1227-1235.e2. [Crossref] [PubMed]
- Wang C, Yang J, Lu M. Micropapillary Predominant Lung Adenocarcinoma in Stage IA Benefits from Adjuvant Chemotherapy. Ann Surg Oncol 2020;27:2051-60. [Crossref] [PubMed]
- Izar B, Sequist L, Lee M, et al. The impact of EGFR mutation status on outcomes in patients with resected stage I non-small cell lung cancers. Ann Thorac Surg 2013;96:962-8. [Crossref] [PubMed]
- Izar B, Zhou H, Heist RS, et al. The prognostic impact of KRAS, its codon and amino acid specific mutations, on survival in resected stage I lung adenocarcinoma. J Thorac Oncol 2014;9:1363-9. [Crossref] [PubMed]
- Xia Q, Zhu Z, Wang J, et al. Expression and association of HER2 with prognosis in early-stage (T1-T2N0M0) non-small cell lung cancer. Tumour Biol 2012;33:1719-25. [Crossref] [PubMed]
- Liao M, Wang H, Lin Z, et al. Vascular endothelial growth factor and other biological predictors related to the postoperative survival rate on non-small cell lung cancer. Lung Cancer 2001;33:125-32. [Crossref] [PubMed]
- Xu S, Wang Y, Ren F, et al. Impact of genetic alterations on outcomes of patients with stage I nonsmall cell lung cancer: An analysis of the cancer genome atlas data. Cancer Med 2020;9:7686-94. [Crossref] [PubMed]
- Graziano SL, Gu L, Wang X, et al. Prognostic significance of mucin and p53 expression in stage IB non-small cell lung cancer: a laboratory companion study to CALGB 9633. J Thorac Oncol 2010;5:810-7. [Crossref] [PubMed]
- Herbst RS, Yano S, Kuniyasu H, et al. Differential expression of E-cadherin and type IV collagenase genes predicts outcome in patients with stage I non-small cell lung carcinoma. Clin Cancer Res 2000;6:790-7.
- D'Amico TA, Aloia TA, Moore MB, et al. Molecular biologic substaging of stage I lung cancer according to gender and histology. Ann Thorac Surg 2000;69:882-6. [Crossref] [PubMed]
- Ruffini E, Asioli S, Filosso PL, et al. Clinical significance of tumor-infiltrating lymphocytes in lung neoplasms. Ann Thorac Surg 2009;87:365-71; discussion 371-2. [Crossref] [PubMed]
- Sandoval J, Mendez-Gonzalez J, Nadal E, et al. A prognostic DNA methylation signature for stage I non-small-cell lung cancer. J Clin Oncol 2013;31:4140-7. [Crossref] [PubMed]
- Suzuki K, Kadota K, Sima CS, et al. Clinical impact of immune microenvironment in stage I lung adenocarcinoma: tumor interleukin-12 receptor β2 (IL-12Rβ2), IL-7R, and stromal FoxP3/CD3 ratio are independent predictors of recurrence. J Clin Oncol 2013;31:490-8. [Crossref] [PubMed]
- Shi T, Zhu S, Guo H, et al. The Impact of Programmed Death-Ligand 1 Expression on the Prognosis of Early Stage Resected Non-Small Cell Lung Cancer: A Meta-Analysis of Literatures. Front Oncol 2021;11:567978. [Crossref] [PubMed]