Prognostic value of ubiquitination-related differentially expressed genes in esophageal squamous cell carcinoma: a comprehensive analysis and future directions
Highlight box
Key findings
• The study identified five key genes (BUB1B, CHEK1, DNMT1, IRAK1, and PRKDC) with prognostic value in esophageal squamous cell carcinoma (ESCC). To improve patient outcomes, new therapies targeting these genes should be developed and further clinically validated.
What is known and what is new?
• Patients with ESCC have a poor prognosis, with a 5-year survival rate of less than 20%. Previous studies have shown that disruptions in ubiquitination pathways are associated with various cancers.
• This study identified five key genes (BUB1B, CHEK1, DNMT1, IRAK1, and PRKDC) with significant prognostic value. Our paper offers new insights into the molecular mechanisms of ESCC, proposes novel biomarkers for prognosis, and suggests potential targets for developing personalized therapies for patients with ESCC.
What is the implication, and what should change now?
• This study is significant for highlighting the prognostic value of ubiquitination-related differentially expressed genes (URDEGs) in ESCC. Our findings suggest that these genes could serve as future biomarkers and potential therapeutic targets. The research emphasizes the need for further clinical validation of the identified URDEGs to establish their utility in clinical practice. Future research should focus on developing targeted therapies that exploit the biological pathways and processes influenced by these genes.
Introduction
Esophageal squamous cell carcinomas (ESCCs) account for approximately 90% of esophageal cancer cases worldwide, and is particularly prevalent in the “esophageal cancer belt” spanning from northern China to the Middle East (1,2). Despite advances in diagnosis and treatment, the 5-year survival rate of patients with ESCC remains below 20% due to detection at an advanced stage, aggressive tumors, and limited effect of treatment (3). Therefore, identifying new biomarkers and therapeutic targets is crucial for the early detection and effective treatment of ESCC.
Growing interest in cancer biology has highlighted the role of posttranslational modifications (PTMs), such as ubiquitination, in tumorigenesis (4). Ubiquitination, the process of attaching ubiquitin to proteins, regulates key cellular functions, including cell cycle, DNA repair, and apoptosis (5). For instance, TRIM26 promotes the development of colorectal cancer by inactivating p53 through ubiquitination, leading to the accumulation of oncogenic proteins and the degradation of tumor suppressor factors (6). Moreover, disruptions in ubiquitination contribute to cancers such as in ESCC and other cancers (7). It has been found that exosomal circRHCG promotes breast cancer metastasis by facilitating M2 polarization, leading to the ubiquitination and degradation of TFEB, which results in poor prognosis for patients with triple-negative breast cancer (8). Similarly, abnormal ubiquitination promotes the progression, drug resistance, and recurrence of melanoma by affecting the unique properties and signaling pathways of cancer stem cell-like cells (CSCs) (9). In addition, the E3 ubiquitin ligase ITCH promotes lysosomal degradation of connexin 43, resulting in the loss of intercellular communication between cancer cells, highlighting ubiquitination’s significance in cancer progression (10).
A few studies have explored the genes linked to ubiquitination in ESCC. Notably, it was found that FBXW7 acts as a tumor suppressor, and that its absence accelerates ESCC progression via increased MAP4 and ERK phosphorylation (11). Additionally, research has revealed that FBXO32 promotes the degradation of cyclin-dependent kinase 9 (CDK9) through ubiquitination, playing a role in the development of esophageal cancer (12). However, a comprehensive analysis of ubiquitination-related differentially expressed genes (URDEGs) in ESCC is lacking and should be conducted given ubiquitination’s critical role in cancer biology. Research in this area has often focused on individual genes or proteins but has not yet employed a systematic approach, hindering the development of effective diagnostic and therapeutic strategies. A comprehensive study integrating differential expression analysis, prognostic evaluation, and functional enrichment of URDEGs across various datasets is needed.
We thus conducted a study that evaluated URDEGs in ESCC, and their prognostic significance. We analyzed independent ESCC datasets from The Cancer Genome Atlas (TCGA), Gene Expression Omnibus (GEO), and our sequencing data to identify URDEGs, comparing gene expression between cancerous and healthy tissues, with a focus on ubiquitination-related genes (URGs). Following this, we used Kaplan-Meier plots and Cox regression analyses to evaluate the prognostic significance of the identified URDEGs for overall survival and clinical outcomes in patients with ESCC. Subsequent enrichment analysis with Gene Ontology (GO) and Kyoto Encyclopedia of Genes and Genomes (KEGG) clarified the roles of these genes in biological processes, molecular functions, and pathways, and indicated their contribution to ESCC pathogenesis. A multivariate Cox regression model was developed using prognostic URDEGs to predict patient outcomes, and was validated with separate datasets. Additionally, protein-protein interaction networks were constructed to identify key hub genes in ESCC and to assess their potential as therapeutic targets. Finally, the interactions between these hub genes and potential therapeutic compounds were characterized to support future drug development. We present this article in accordance with the TRIPOD reporting checklist (available at https://jtd.amegroups.com/article/view/10.21037/jtd-24-1863/rc).
Methods
Data and information
The TCGA-ESCC dataset (https://portal.gdc.cancer.gov/), comprising 93 ESCC samples with corresponding patient clinical information, was downloaded TCGA. This included 82 tumor samples and 11 matched normal tissue samples. The count sequencing data were standardized to fragments per kilobase per million mapped fragment format, and clinical data were obtained from UCSC Xena (http://genome.ucsc.edu).
We obtained the GSE20347 expression profile dataset for patients with ESCC from the GEO database using the “GEOquery” R package (version 1.30.0, The R Foundation for Statistical Computing) (13). This dataset includes microarray data from 17 ESCC samples and 17 matched normal tissues. Probe annotations were completed using the GPL571 Affymetrix Human Genome U133A 2.0 Array. All samples from GSE20347 were included in the subsequent analysis. We used our sequenced ESCC expression profile dataset, comprising six tumor tissues and six matched normal tissues. For validation, we used the GSE20347 and ESCC datasets. Comprehensive details regarding the datasets can be found in Table S1.
GeneCards (https://www.genecards.org/) (14) offers detailed information on human genes. On this platform, we filtered for protein-coding URGs with a relevance score above 5.00 using the keyword “Ubiquitination”, yielding 1,162 URGs. We also identified URGs from published literature by searching in PubMed with the keyword “Ubiquitination” (15), which resulted in 11 URGs. Finally, a search of the Molecular Signatures Database (MSigDB) with the keyword “Ubiquitination” produced 16 gene sets, for a total of 542 URGs. After merging and removing duplicates, we identified 1,274 unique URGs. The gene names are listed in Table S2. The study was conducted in accordance with the Declaration of Helsinki (as revised in 2013).
ESCC gene expression
We normalized and analyzed ESCC datasets GSE20347 and TCGA-ESCC using The limma R package to identify mechanisms, biological characteristics, and pathways linked to differentially expressed genes (DEGs). DEGs between tumor and normal groups were identified with |log fold change (FC)| >0.5 and an adjusted P value (adj. P) <0.05. Upregulated DEGs had logFC >0.5 and adj. P=0.05, while downregulated DEGs had logFC <–0.5 and P. adj. =0.05.
The URDEGs, identified from TCGA-ESCC, GSE20347, and ESCC datasets (|logFC| >0.5, adj. P<0.05), were visualized in a Venn diagram. Another Venn diagram was used to illustrate the overlap between Co-DEGs and URGs to find ubiquitination-related common DEGs (Co-DEGs). Kaplan-Meier survival analysis was also conducted on the TCGA-ESCC dataset, with P<0.05 serving as the significance threshold. A gene that met this criterion was considered to be a prognostic URDEG (PURDEG). The outcomes of the differential expression analysis were depicted through volcano plots created with the ggplot2 library and heatmaps produced using the “pheatmap” package in R.
Functional analysis of the DEGs
To further investigate the biological functions, GO and KEGG analyses were conducted on the PURDEGs. Additionally, a gene set enrichment analysis (GSEA) was performed via the GSEA online tool (http://software.broadinstitute.org/gsea/index.jsp), and a gene set variation analysis (GSVA) was conducted using the “GSVA” R package.
Gene mutation analysis
The cBioPortal for Cancer Genomics (15) (http://cbioportal.org) offers a web resource for exploring, visualizing, and analyzing multidimensional cancer genomics data. The database simplifies molecular analysis data from cancer tissues and cell lines into genetic, epigenetic, gene expression, and proteomic events. We analyzed the gene mutation profiles of PURDEGs within the TCGA-ESCC dataset using the cBioPortal database.
CIBERSORT immune infiltration analysis
The CIBERSORT23 algorithm estimates immune cell composition from transcriptome data (16). We used this algorithm to analyze TCGA-ESCC data with the LM22 signature, filtering for immune cell enrichment scores below 0, and generated an immune cell infiltration matrix. We used the Pearson algorithm and ggplot2 package in R language environment to calculate and visualize correlations between immune cells. Next, we computed and plotted correlations between immune cells and hub genes using the TCGA-ESCC dataset with ggplot2.
Prognostic correlation analysis
To develop multivariate Cox models for key genes in the TCGA-ESCC dataset, we initially conducted univariate Cox regression analysis. We then used the R package “rms” to build a multivariate Cox model. Nomograms, which graphically represent relationships between multiple variables, allow for the calculation of a total score by summing individual variable scores based on a set scale. Calibration curves were utilized to evaluate the accuracy and discriminative capacity of the multivariate Cox model by comparing observed outcomes with predicted probabilities. This approach ultimately facilitated an assessment of the model’s goodness of fit. Decision curve analysis (DCA) is another method used to assess the clinical utility of predictive models, diagnostic tests, and molecular markers. We evaluated the model’s accuracy and discriminative power with DCA, creating DCA plots using the R package “ggDCA”.
Quantitative real time-polymerase chain reaction (qRT-PCR) validation for the expression of BUB1B, CHEK1, DNMT1, IRAK1, and PRKDC
qRT-PCR confirmed the expression of BUB1B, CHEK1, DNMT1, IRAK1, and PRKDC in 14 esophageal cancer and adjacent normal tissue pairs; the related primers were obtained from BioSune (Shanghai, China) and are listed in Table S3. Complement DNA was synthesized from 1 µg of total RNA using RTIII All-in-One Mix with dsDNase (Monad Biotech, Shanghai, China). qRT-PCR was performed on a QuantStudio 6 Flex system (Applied Biosystems, Thermo Fisher, Waltham, MA, USA) with Hieff qPCR SYBR Green Master Mix, Low Rox (Yeasen Biotechnology, Shanghai, China). The reaction protocol included an initial 10-minute denaturation at 95 ℃, followed by 40 cycles of 95 ℃ for 15 seconds and 60 ℃ for 1 minute. GAPDH served as the reference gene, and gene expression levels were calculated using the 2−ΔΔCt method.
Statistical analysis
Data processing and analysis were conducted using R software version 4.2.2. For normally distributed continuous variables, the independent t-test was used, while the Mann-Whitney test was applied for nonnormally distributed variables. Categorical variables were compared with the chi-square test or the Fisher exact test. Survival analysis was conducted via R “survival” package, with Kaplan-Meier curves illustrating survival differences and the log-rank test assessing their significance. Both univariate and multivariate Cox regression analyses were performed. Two-sided P values <0.05 were deemed significant.
Results
Examination of genes with varied expression in ESCC
The entire study workflow is illustrated in Figure S1. Using the R Limma package, we analyzed gene expression differences between ESCC tumors and normal tissues in the TCGA-ESCC and ESCC datasets, identifying the DEGs. We identified 56,743 genes in TCGA-ESCC dataset, with 12,853 of these meeting the criteria of |logFC| >0.5 and an adj. P<0.05. Among these, 6,137 genes were upregulated (higher expression in cancer) and 6,716 were downregulated (higher expression in normal tissues). A volcano plot was constructed to depict the results of the differential analysis conducted on this dataset (refer to Figure 1A). Figure 1B shows a volcano plot of the differential analysis for the GSE20347 dataset, with 12,548 genes being identified. Of these, 2,852 genes met the criteria of |logFC| >0.5 and adj. P<0.05, with 1,483 upregulated and 1,369 downregulated genes. We identified 31,263 genes from the ESCC dataset identified, with 6,154 meeting the criteria of |logFC| >0.5 and adj. P<0.05. Among these, 3,464 genes were upregulated and 2,690 were downregulated. Figure 1C shows a volcano plot of these results. We identified 943 Co-DEGs by overlapping DEGs with |logFC| >0.5 and adj. P<0.05 from TCGA-ESCC, GSE20347, and the ESCC datasets (Figure 1D). From these Co-DEGs, we found 85 URDEGs by intersecting them with URGs, with the results being illustrated in a Venn diagram (Figure 1E).

Identification of predictive genes from URDEGs in the TCGA-ESCC data
Kaplan-Meier survival plots were created for 85 URDEGs, yielding five PURDEGs with significant P values (P<0.05): BUB1B, CHEK1, DNMT1, IRAK1, and PRKDC (Figure 2A-2E). We then analyzed the differential expression of these genes in cancer versus normal groups within TCGA-ESCC, GSE20347, and another ESCC dataset using Venn diagrams (Figure 2F-2H). Heatmaps created using the R package “heatmap” were used to display the differential expression outcomes (Figure 2F-2H).
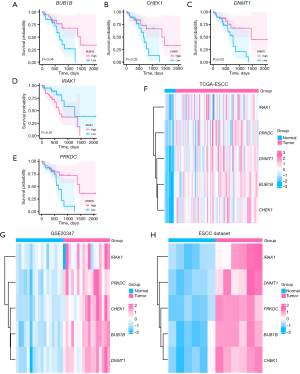
Differential expression analysis of PURDEGs
We conducted a correlation study on the expression differences of five PURDEGs (BUB1B, CHEK1, DNMT1, IRAK1, and PRKDC) between cancerous and healthy tissues in patients with ESCC, using data from TCGA-ESCC, GSE20347, and an additional ESCC dataset (Table S4). The differential expression of these five PURDEGs in TCGA dataset was assessed using the Wilcoxon rank-sum test. In TCGA-ESCC dataset, BUB1B, CHEK1, DNMT1, IRAK1, and PRKDC were significantly upregulated in tumor samples compared to healthy samples (P<0.001 for BUB1B, CHEK1, DNMT1, and PRKDC; P<0.01 for IRAK1) (Figure 3A).
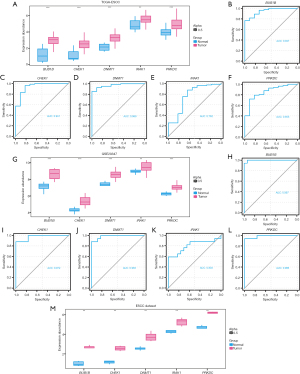
We further evaluated the diagnostic accuracy of the five PURDEGs in the TCGA-ESCC datasets using receiver operating characteristic (ROC) curves (Figure 3B-3F). In TCGA-ESCC dataset, BUB1B [area under the curve (AUC) =0.947], CHEK1 (AUC =0.941), and DNMT1 (AUC =0.969) demonstrated high accuracy, while IRAK1 (AUC =0.762) and PRKDC (AUC =0.855) demonstrated relatively lower accuracy.
Similarly, we analyzed the differential expression of the five PURDEGs in the GSE20347 dataset (Figure 3G), and these genes were also significantly upregulated in the tumor group. In the GSE20347 dataset, BUB1B, CHEK1, DNMT1, and PRKDC exhibited high accuracy, with AUCs of 0.997, 0.972, 0.983, and 0.986, respectively, while IRAK1 showed lower accuracy (Figure 3H-3L). Additionally, in the ESCC dataset, the five PURDEGs were also significantly upregulated in the tumor group (Figure 3M).
Panoramic analysis of mutations and copy number variations in the TCGA-ESCC dataset
We analyzed the single-nucleotide polymorphisms (SNPs) in patients with ESCC with the TCGA-ESCC dataset, focusing on upregulated genes. Using the R package “maftools”, we identified nine main mutation types in URGs, predominantly missense mutations (Figure 4A). Most mutations were SNPs, with fewer insertions and deletions. The most common single-nucleotide variant (SNV) among patients with ESCC was C to T, followed by C to A and T to C (Figure 4A). We analyzed TCGA-ESCC dataset for URG mutations (Figure 4B) and found significant correlations (P<0.05) among several mutated genes, including OBSCN, DYNC2H1, LAMA1, and TTN.
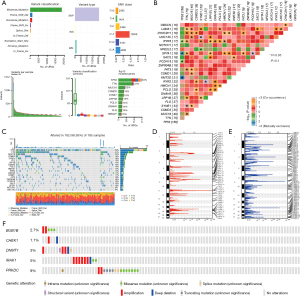
TP53 had the highest SNP occurrence in the TCGA-ESCC dataset, with eight mutation types (Figure 4C). The top 10 genes with the most SNPs were TP53, TTN, MUC16, CSMD3, SYNE1, FLG, LRP1B, DNAH5, PCLO, and HMCN1, with missense mutations being the most common. SNP statuses for the top 20 genes in patients with ESCC are also shown in Figure 4C. Using Genomic Identification of Significant Targets In Cancer (GISTIC) 2.0 on TCGA-ESCC dataset, we found more gene deletions than amplifications (Figure 4D,4E). cBioPortal analysis of five PURDEGs in the same dataset revealed amplifications to be the main mutation type (Figure 4F).
Functional enrichment (GO) analysis and pathway enrichment (KEGG) analysis of PURDEGs
We performed a GO enrichment analysis (Table S5) to investigate the biological functions, molecular roles, cellular structures, and pathways linked to the five PURDEGs (BUB1B, CHEK1, DNMT1, IRAK1, PRKDC) in ESCC. Significant terms had adj. P values and false-discovery rates (q values) below 0.05. The analysis revealed that these genes are mainly involved in mitotic cell cycle checkpoint signaling, cell cycle checkpoint signaling, and regulation of smooth muscle cell growth. Figure 5A shows the GO functional enrichment analysis results in a bubble chart, while Figure 5B-5D display the biological process (BP), cellular component (CC), and molecular function (MF) pathways as network diagrams. We conducted a KEGG pathway enrichment analysis on the five PURDEGs (Table S6), revealing significant enrichment in pathways of cell cycle, HIV-1 infection, Human T lymphotropic virus type 1 (HTLV-1 infection), and non-homologous end-joining. Bar plots (Figure 5E) and network diagrams (Figure 5F) were used to visualize gene expression in these pathways. These findings suggest that the five PURDEGs may play roles in ESCC, particularly in cell cycle regulation, immune response, and DNA repair.
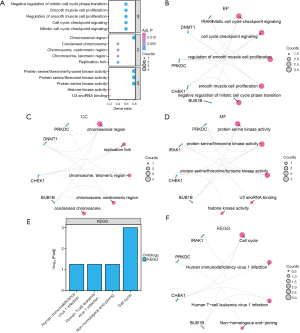
GSEA for TCGA-ESCC dataset
We used GSEA on TCGA-ESCC dataset (Figure 6A) to evaluate the impact of gene expression on ESCC development, focusing on related BPs, CCs, and MFs with significance thresholds of adj. P<0.05 and false-discovery rate <0.25. The findings revealed that the genes within the cancer cohort of the TCGA-ESCC dataset were markedly enriched in several pathways, including “reactome cellular senescence” (Figure 6B), “reactome oxidative stress-induced senescence” (Figure 6C), “reactome pre-Notch expression and processing” (Figure 6D), “reactome T-cell factor (TCF)-dependent signaling in response to wingless/integrated (WNT)” (Figure 6E), and “reactome signaling by Notch” (Figure 6F), among others (Figures 6B-6F, Table S7).
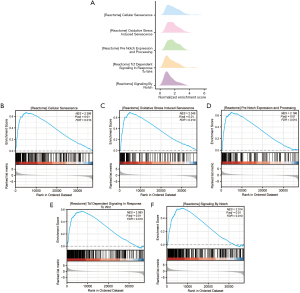
GSVA of enrichment in TCGA-ESCC dataset
We used GSVA to compare hallmark gene sets between cancerous and healthy samples in the TCGA-ESCC dataset, which yielded significant differences (adj. P <0.05) in 34 gene sets (see Figure 7 and Table S8). Out of these, 24 pathways, including hallmark E2F targets, hallmark G2M checkpoint, and hallmark MYC targets v.1, exhibited notably elevated enrichment scores in the cancer cohort relative to the healthy cohort (adj. P. <0.05). Conversely, 10 pathways, including hallmark bile acid metabolism, hallmark pancreas beta cells, and hallmark adipogenesis, exhibited notably higher enrichment scores in the healthy group than in the cancer group (adj. P<0.05). We used GSVA to analyze the expression levels of 34 key pathways in the TCGA-ESCC dataset. Heatmaps (Figure 7A) and comparative charts (Figure 7B) were generated using PHeatmap in R and the Mann-Whitney test, respectively. All pathways showed statistically significant expression differences between cancerous and healthy groups (P<0.05).
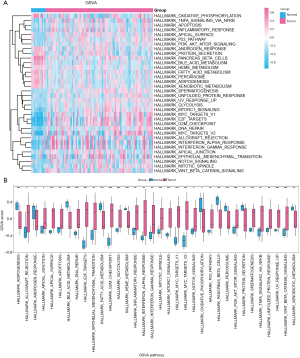
Evaluation of key genes and immune profiling via CIBERSORT in TCGA-ESCC dataset
We analyzed TCGA-ESCC dataset to determine if the expression of five hub genes (BUB1B, CHEK1, DNMT1, IRAK1, and PRKDC) correlated with the prognosis of patients with ESCC. CHEK1 expression was found to vary significantly (P<0.05) with T stage, age, and survival outcomes (Figure 8A-8C). Using the CIBERSORT method, we evaluated the presence of 22 immune cell types in high- and low-score groups within TCGA-ESCC dataset. Figure 8D is bar graph of the infiltration levels of 20 immune cells (values above zero) across all samples. Additionally, Figure 8E depicts the relationships between these 20 immune cells, revealing mostly negative correlations. We analyzed the link between 20 immune cell types and 5 key genes (BUB1B, CHEK1, DNMT1, IRAK1, and PRKDC) (Figure 8F). The results showed a significant positive correlation between M0 macrophages and BUB1B and between resting memory T cells CD4 and PRKDC. Conversely, resting mast cells were negatively correlated with BUB1B, CHEK1, and DNMT1.
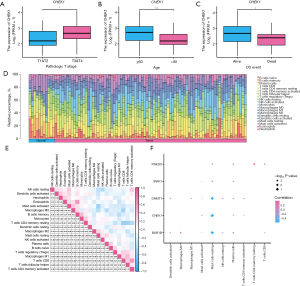
Development of via multivariate cox regression model for TCGA-ESCC dataset
We conducted univariate Cox regression on the five key genes (BUB1B, CHEK1, DNMT1, IRAK1, and PRKDC) in TCGA-ESCC dataset to evaluate their prognostic significance. Genes with P values below 0.1 were included in a multivariate Cox regression model (Table S9). The univariate analysis results are displayed in the forest plot in Figure 9A. We performed nomogram analysis to evaluate the model’s predictive ability and generated a nomogram chart (Figure 9B). This tool uses multivariate regression to assign scores to factors, with a total score being calculated to represent an event’s probability.
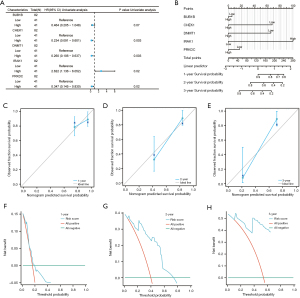
As seen in Figure 9B, the multivariate Cox model identifies IRAK1 in TCGA-ESCC dataset as a significantly stronger predictor than the other factors. Figure 9C-9E show the assessment of the nomogram’s calibration at 1, 2, and 3 years, respectively, with calibration curves. The x-axis shows the model’s predicted survival probability, while the y-axis shows the observed survival probability, with colored lines and points indicating the model’s predictions over time. Their performance appears to improve near the gray reference line. We used DCA to assess the clinical utility of our multivariate Cox model at 1-year (Figure 9F), 2-year (Figure 9G), and 3-year (Figure 9H) intervals, as shown in Figure 9F-9H. In the DCA graph (Figure 9F-9H), the horizontal axis shows the threshold probability, and the vertical axis shows the net benefit. The model’s effectiveness can be discerned by observing to what degree the curve stays above the “All positive” and “All negative” lines, with a broader range of x-values indicating better performance. Our multivariate Cox regression model showed the highest clinical predictive performance at 2 years, followed by 3 years, and then 1 year.
Validation of EUB1B, CHEK1, DNMT1, IRAK1, and PRKDC via qRT-PCR
To validate the RNA sequencing and bioinformatics findings, qRT-PCR was used to measure the expression of the key URGs, namely BUB1B, CHEK1, DNMT1, IRAK1, and PRKDC, in ESCC and adjacent healthy tissues (see Table S3 and Figure 10). In ESCC tissues, BUB1B and PRKDC were significantly overexpressed (P<0.05 and P<0.01, respectively), while CHEK1 and IRAK1 showed non-significantly elevated levels (P=0.06 and P=0.07, respectively). Meanwhile, DNMT1 was significantly upregulated (<0.05).
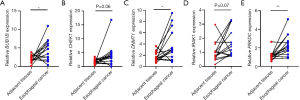
Discussion
This study conducted a comprehensive analysis of URDEGs in ESCC, leading to the identification of a subset of PURDEGs. Five PURDEGs—BUB1B, CHEK1, DNMT1, IRAK1, and PRKDC—were identified and confirmed to have a significant correlation with overall survival rates in patients with ESCC. The expression levels of these genes varied between cancerous and healthy tissues, showing significant prognostic value and indicating their potential as biomarkers for predicting ESCC outcomes. Moreover, functional enrichment studies underscored their participation in essential biological activities, including cell cycle control and DNA repair, highlighting their importance in the development of ESCC.
Our findings align with and build upon existing research on the role of ubiquitination in cancer. Deubiquitinases play a crucial role in regulating cellular homeostasis, and their dysregulation is associated with diseases such as cancer, affecting the tumor microenvironment and immune evasion (17). Dysregulated ubiquitination has been implicated in various cancers, including ESCC, and contributes to tumor progression and therapy resistance (18). Prior research has demonstrated that ubiquitination pathways critically regulate the stability of oncogenes and tumor suppressors, thus influencing cancer cell proliferation and survival (19).
In addition, our identification of PURDEGs including BUB1B and CHEK1, key players in mitotic checkpoint signaling and DNA damage response, underscores the importance of ubiquitination in genomic stability and cancer prevention. Notably, BUB1B, a mitotic checkpoint kinase, is overexpressed in various cancers, such as lung and colorectal cancer, and promotes chromosomal instability and tumor progression (20,21). Our research shows that BUB1B is significantly upregulated in ESCC and linked to poor outcomes, suggesting it could indicate aggressive cancer types. Similarly, CHEK1, a key regulator of the DNA damage response, is associated with resistance to DNA-damaging treatments in various cancers (22). Identifying CHEK1 as a prognostic marker in ESCC indicates its role in helping cancer cells survive genotoxic stress.
Moreover, we identified DNMT1, IRAK1, and PRKDC as prognostic markers in ESCC. DNMT1, essential for maintaining DNA methylation during cell division, is associated with altered gene methylation that can inactivate tumor suppressors and promote cancer progression (23,24). Our findings provide novel evidence that higher DNMT1 levels in ESCC correlate with poor patient outcomes, suggesting that DNMT1-driven epigenetic changes are crucial to ESCC development. IRAK1, a kinase involved in inflammatory signaling, promotes cancer cell growth and survival via NF-κB activation (25). The finding of an association between IRAK1 and the poor prognosis of patients with ESCC aligns with a study (25), confirming its potential as a therapeutic target in inflammation-driven cancers. PRKDC, a DNA-dependent protein kinase catalytic subunit (DNA-PKcs), is essential for non-homologous end-joining in DNA repair. Elevated PRKDC levels are linked to resistance against radiotherapy and chemotherapy in multiple cancers (26). Our results indicate that PRKDC may serve as a marker for therapeutic resistance in ESCC, highlighting the need for targeted treatments to counteract PRKDC-related resistance.
Our analysis of the five key genes—BUB1B, CHEK1, DNMT1, IRAK1, and PRKDC—demonstrated variability in their diagnostic accuracy, as indicated by their respective AUC values. Specifically, genes such as DNMT1 and PRKDC, which possess AUC values approaching 1.0, exhibit high sensitivity and specificity, thereby indicating robust prognostic predictive power for ESCC. Conversely, genes like IRAK1, characterized by comparatively lower AUC values, may exhibit limitations in sensitivity or specificity, leading to reduced predictive accuracy. Future research should aim to further enhance the specificity and sensitivity of these markers.
Furthermore, analyses utilizing GO, KEGG, and GSEA analyses revealed that five PURDEGs are significantly enriched in various signaling pathways associated with the cell cycle, DNA repair, cellular aging, among others. Notably, BUB1B and CHEK1 exhibit significant enrichment in the cell cycle checkpoint pathway, which plays a critical role during cell division. Dysregulation of this pathway may contribute to the accelerated proliferation of ESCC cells, thereby facilitating tumor growth and progression (27). The Non-Homologous End Joining (NHEJ) repair pathway exhibits a significant enrichment of PURDEGs, with a notable upregulation of the PRKDC gene. This upregulation may augment the DNA repair capacity of ESCC tumor cells, thereby contributing to their enhanced survival rates and resistance to therapeutic interventions (28). In addition, GSEA indicates that cellular senescence and oxidative stress pathways play critical roles in tumor progression. Specifically, senescent cells within the tumor microenvironment may facilitate cancer cell proliferation, invasion, and metastasis through the secretion of the senescence-associated secretory phenotype (SASP), while oxidative stress may promote tumor progression by inducing genetic mutations (29). The Notch and WNT signaling pathways are integral to processes such as cell proliferation, differentiation, and fate determination. In ESCC, dysregulated activation of Notch and WNT/β-catenin signaling pathways may enhance cancer cell proliferation, invasiveness, and metastatic potential (30). Consequently, the aberrant expression of genes including BUB1B, CHEK1, DNMT1, IRAK1, and PRKDC is likely to contribute significantly to the development and progression of ESCC, highlighting these genes as potential therapeutic targets for intervention.
Our study advances ESCC research by identifying and validating PURDEGs and uncovering key molecular mechanisms. BUB1B, CHEK1, DNMT1, IRAK1, and PRKDC were identified as potential prognostic biomarkers for personalized treatments. Functional and pathway analyses revealed disruptions in cell cycle control and DNA repair, suggesting new therapeutic targets. Finally, the integration of various omics data clarified the complex molecular pathways in ESCC progression.
Despite the promising findings our study has produced, certain limitations should be noted. First, the results relied on retrospective data, and thus future prospective studies are required for clinical validation. Secondly, as our sample size was small, especially in validation cohorts, larger studies are need for greater generalizability. Third, our focus on a subset of URGs might not have fully captured the complexity of ubiquitination in ESCC. Investigating PURDEGs in different ESCC subcategories is thus essential for identifying patient groups that may benefit from specific treatments.
Conclusions
Our study offers an in-depth examination of genes associated with ubiquitination in ESCC and identified crucial PURDEGs that could serve as prognostic indicators and therapeutic targets. Although additional studies are necessary to confirm these results and examine their medical implications, our research establishes a foundation for future exploration of ubiquitination’s role in ESCC and various other cancers.
Acknowledgments
We would like to thank Editage for providing thesis revision support.
Funding: This study was supported in part by grants from
Footnote
Reporting Checklist: The authors have completed the TRIPOD reporting checklist. Available at https://jtd.amegroups.com/article/view/10.21037/jtd-24-1863/rc
Peer Review File: Available at https://jtd.amegroups.com/article/view/10.21037/jtd-24-1863/prf
Conflicts of Interest: All authors have completed the ICMJE uniform disclosure form (available at https://jtd.amegroups.com/article/view/10.21037/jtd-24-1863/coif). A.M. received $2,000 for speaker and participation fee from ASCO Advantage Upper GI cancer program; received $2,000 for attending 2 meetings from Curio Science; received $2,000 for attending 1 meeting from MD Outlook. The other authors have no conflicts of interest to declare.
Ethical Statement: The authors are accountable for all aspects of the work in ensuring that questions related to the accuracy or integrity of any part of the work are appropriately investigated and resolved. The study was conducted in accordance with the Declaration of Helsinki (as revised in 2013).
Open Access Statement: This is an Open Access article distributed in accordance with the Creative Commons Attribution-NonCommercial-NoDerivs 4.0 International License (CC BY-NC-ND 4.0), which permits the non-commercial replication and distribution of the article with the strict proviso that no changes or edits are made and the original work is properly cited (including links to both the formal publication through the relevant DOI and the license). See: https://creativecommons.org/licenses/by-nc-nd/4.0/.
References
- Bray F, Laversanne M, Sung H, et al. Global cancer statistics 2022: GLOBOCAN estimates of incidence and mortality worldwide for 36 cancers in 185 countries. CA Cancer J Clin 2024;74:229-63. [Crossref] [PubMed]
- Abnet CC, Arnold M, Wei WQ. Epidemiology of Esophageal Squamous Cell Carcinoma. Gastroenterology 2018;154:360-73. [Crossref] [PubMed]
- Zhou X, Bao W, Zhu X, et al. Molecular characteristics and multivariate survival analysis of 43 patients with locally advanced or metastatic esophageal squamous cell carcinoma. J Thorac Dis 2024;16:1843-53. [Crossref] [PubMed]
- Liu F, Chen J, Li K, et al. Ubiquitination and deubiquitination in cancer: from mechanisms to novel therapeutic approaches. Mol Cancer 2024;23:148. [Crossref] [PubMed]
- Rivas V, González-Muñoz T, Albitre Á, et al. GRK2-mediated AKT activation controls cell cycle progression and G2 checkpoint in a p53-dependent manner. Cell Death Discov 2024;10:385. [Crossref] [PubMed]
- Lu H, Tan Z, Ko H, et al. Tripartite motif-containing protein 26 promotes colorectal cancer growth by inactivating p53. Res Sq [Preprint] 2024;rs.3.rs-3782833. doi:
10.21203/rs.3.rs-3782833/v1 . - Jiang J, Dong X, Liu J, et al. TRIM67 Promotes Non-Small Cell Lung Cancer Development by Positively Regulating the Notch Pathway through DLK1 Ubiquitination. J Cancer 2024;15:1870-9. [Crossref] [PubMed]
- Shen HY, Xu JL, Zhang W, et al. Exosomal circRHCG promotes breast cancer metastasis via facilitating M2 polarization through TFEB ubiquitination and degradation. NPJ Precis Oncol 2024;8:22. [Crossref] [PubMed]
- Al Hmada Y, Brodell RT, Kharouf N, et al. Mechanisms of Melanoma Progression and Treatment Resistance: Role of Cancer Stem-like Cells. Cancers (Basel) 2024;16:470. [Crossref] [PubMed]
- Totland MZ, Knudsen LM, Rasmussen NL, et al. The E3 ubiquitin ligase ITCH negatively regulates intercellular communication via gap junctions by targeting connexin43 for lysosomal degradation. Cell Mol Life Sci 2024;81:171. [Crossref] [PubMed]
- Pan Y, Liu J, Gao Y, et al. FBXW7 loss of function promotes esophageal squamous cell carcinoma progression via elevating MAP4 and ERK phosphorylation. J Exp Clin Cancer Res 2023;42:75. [Crossref] [PubMed]
- Song XQ, Chen BB, Jin YM, et al. DNMT1-mediated epigenetic suppression of FBXO32 expression promoting cyclin dependent kinase 9 (CDK9) survival and esophageal cancer cell growth. Cell Cycle 2024;23:262-78. [Crossref] [PubMed]
- Hu N, Clifford RJ, Yang HH, et al. Genome wide analysis of DNA copy number neutral loss of heterozygosity (CNNLOH) and its relation to gene expression in esophageal squamous cell carcinoma. BMC Genomics 2010;11:576. [Crossref] [PubMed]
- Stelzer G, Rosen N, Plaschkes I, et al. The GeneCards Suite: From Gene Data Mining to Disease Genome Sequence Analyses. Curr Protoc Bioinformatics 2016;54:1.30.1-1.30.33.
- Li Y, An L, Jia Z, et al. Identification of Ubiquitin-Related Gene-Pair Signatures for Predicting Tumor Microenvironment Infiltration and Drug Sensitivity of Lung Adenocarcinoma. Cancers (Basel) 2022;14:3478. [Crossref] [PubMed]
- Chen B, Khodadoust MS, Liu CL, et al. Profiling Tumor Infiltrating Immune Cells with CIBERSORT. Methods Mol Biol 2018;1711:243-59. [Crossref] [PubMed]
- Hsu SK, Chou CK, Lin IL, et al. Deubiquitinating enzymes: potential regulators of the tumor microenvironment and implications for immune evasion. Cell Commun Signal 2024;22:259. [Crossref] [PubMed]
- Jayaprakash S, Hegde M. Unraveling the Potential Role of NEDD4-like E3 Ligases in Cancer. Int J Mol Sci 2022;23:12380. [Crossref] [PubMed]
- Xiao S, Chen J, Wei Y, et al. BHLHE41 inhibits bladder cancer progression via regulation of PYCR1 stability and thus inactivating PI3K/AKT signaling pathway. Eur J Med Res 2024;29:302.
- Chen J, Liao Y, Fan X. Prognostic and clinicopathological value of BUB1B expression in patients with lung adenocarcinoma: a meta-analysis. Expert Rev Anticancer Ther 2021;21:795-803. [Crossref] [PubMed]
- Zeng Q, Zhang S, He L, et al. Knockdown of BUB1B Inhibits the Proliferation, Migration, and Invasion of Colorectal Cancer by Regulating the JNK/c-Jun Signaling Pathway. Cancer Biother Radiopharm 2024;39:236-46. [Crossref] [PubMed]
- Krishnaraj J, Yamamoto T, Ohki R. p53-Dependent Cytoprotective Mechanisms behind Resistance to Chemo-Radiotherapeutic Agents Used in Cancer Treatment. Cancers (Basel) 2023;15:3399. [Crossref] [PubMed]
- Huang F, Wu X, Du Q, et al. Systematic Characterization of DNA Methyltransferases Family in Tumor Progression and Antitumor Immunity. Technol Cancer Res Treat 2024;23:15330338241260658. [Crossref] [PubMed]
- Kanai Y. Molecular pathological approach to cancer epigenomics and its clinical application. Pathol Int 2024;74:167-86. [Crossref] [PubMed]
- Lei C, Gao Z, Lv X, et al. Saikosaponin-b2 Inhibits Primary Liver Cancer by Regulating the STK4/IRAK1/NF-κB Pathway. Biomedicines 2023;11:2859. [Crossref] [PubMed]
- Piwocka O, Piotrowski I, Suchorska WM, et al. Dynamic interactions in the tumor niche: how the cross-talk between CAFs and the tumor microenvironment impacts resistance to therapy. Front Mol Biosci 2024;11:1343523. [Crossref] [PubMed]
- Wu H, Zhu P, Shu P, et al. Screening and verification of hub genes in esophageal squamous cell carcinoma by integrated analysis. Sci Rep 2024;14:6894. [Crossref] [PubMed]
- Wang G, Guo S, Zhang W, et al. A Comprehensive Analysis of Alterations in DNA Damage Repair Pathways Reveals a Potential Way to Enhance the Radio-Sensitivity of Esophageal Squamous Cell Cancer. Front Oncol 2020;10:575711. [Crossref] [PubMed]
- Zhang H, Hong K, Song Q, et al. Integrative Analysis and Validation of a Cancer-associated Fibroblasts Senescence-related Signature for Risk Stratification and Therapeutic Prediction in Esophageal Squamous Cell Carcinoma. J Cancer 2024;15:5742-61. [Crossref] [PubMed]
- Abbaszadegan MR, Riahi A, Forghanifard MM, et al. WNT and NOTCH signaling pathways as activators for epidermal growth factor receptor in esophageal squamous cell carcinoma. Cell Mol Biol Lett 2018;23:42. [Crossref] [PubMed]