Prognostic value and potential biological function of PMSD11 in lung adenocarcinoma
Highlight box
Key findings
• PSMD11 has the potential to be a novel therapeutic target and sensitive biomarker for patients with lung adenocarcinoma (LUAD).
What is known and what is new?
• PSMD11 may be expressed not only in malignant tumor cells but also in immune cells, indicating that PSMD11 may contribute to the shaping of the tumor microenvironment and the immune response in LUAD.
• PSMD11 may have considerable prognostic value for patients with LUAD.
What is the implication, and what should change now?
• Overall, our findings point to the critical role that PSMD11 has in LUAD progression and provide a new therapeutic target and prognostic marker for LUAD.
Introduction
Among cancer types, lung cancer is associated with the highest incidence and the highest cancer-related morbidity and mortality worldwide (1). Non-small cell lung cancer (NSCLC) is the most common type of lung cancer. Lung adenocarcinoma (LUAD), an aggressive pathological subtype of primary lung cancer with a poor prognosis, accounts for the largest number of diagnosed cases of NSCLC (2,3). According to epidemiological data, patients with LUAD have an average 5-year survival rate of <20% even after conventional therapy, molecule targeted therapy, and immunotherapy (4,5). Therefore, there is an urgent necessary to explore novel therapeutic targets and sensitive biomarkers for patients with LUAD.
The ubiquitin proteasome system is critical to maintaining cellular homeostasis (6). It regulates the degradation of intracellular proteins, facilitates DNA repair, drives cell-cycle progression and human-antigen processing, and figures prominently in the degradation of misfolded endoplasmic reticulum proteins. The 26S proteasome is composed of one proteolytically active cylinder-shaped particle (the 20S proteasome), and one or two ATPase-containing complexes (known as the 19S cap complexes) (7). In recent studies, dysfunction of the ubiquitin-proteasome system, which manifests as regulation of gene expression, has been frequently implicated in tumorigenesis (8,9). Hence, extensive research needs to be conducted to fully assess the oncogenic potential of this family of genes. The PSMD family, comprising 14 members in total (PSMD1–14), was demonstrated to be partially involved in the formation of the regulatory complex. Both components, the 20S proteasome and 19S cap complexes, occupy an important place in modulating the proteasome that performs several essential functions, such as catalyzing the unfolding and translocation of substrates into the 20S proteasome. PSMD family members have been identified as potential prognostic biomarkers and immune infiltration signatures for cancer progression and are promising clinical therapeutic targets (10,11).
The 26S non-ATPase regulatory subunit 11 (PSMD11), a member of the S9 family encoding the proteasome subunit, is a non-ATPase subunit of the 19S regulator and can be phosphorylated by AMP-activated protein kinase (12). PSMD11 is a multiprotein complex that participates in the ATP-dependent degradation of ubiquitinated proteins and is essential to regulation of embryonic stem cell proteasome activity. PSMD11 may be critically involved in the emergence and progression of cancer cells (13-15). Certain studies have demonstrated that PSMD11 promotes the progression of hepatocellular carcinoma (HCC) and pancreatic cancer and thus represents a promising therapeutic for these disease (16,17). However, the prognostic value and potential biological function of PMSD11 in LUAD remain unclear.
In our study, we endeavored to comprehensively investigate the prognostic and biological value of PSMD11 in LUAD cohorts. We further sought to clarify the underlying mechanisms of PSMD11 in LUAD tumorigenesis and progression via rigorous bioinformatics analyses, including expression analysis, survival analysis, clinicopathological analysis, immune microenvironment analysis, drug analysis, and cuproptosis analysis. Overall, our findings point to PSMD11 being critically involved in LUAD progression and provide a novel therapeutic target and prognostic marker for LUAD. We present this article in accordance with the TRIPOD reporting checklist (available at https://jtd.amegroups.com/article/view/10.21037/jtd-24-1622/rc).
Methods
Data acquisition and preprocessing
In this study, we acquired a single-cell sequencing data set, GSE203360, comprising six samples, from the Gene Expression Omnibus (GEO; https://www.ncbi.nlm.nih.gov/geo/) database. The marker gene sets used in this study were sourced from the CellMarker website (http://xteam.xbio.top/CellMarker/). Furthermore, we obtained an LUAD dataset from The Cancer Genome Atlas (TCGA) database (https://portal.gdc.cancer.gov/), which includes 59 normal samples and 535 tumor samples. Additionally, we accessed transcriptome data for normal and tumor samples of 33 cancer types from the Genotype-Tissue Expression (GTEx; https://www.gtexportal.org/home/index.html/) database. The study was conducted in accordance with the Declaration of Helsinki (as revised in 2013).
Pancancer and single-cell analysis
We integrated patient data from the GTEx and TCGA database, conducted differential analysis, and visualized the expression patterns of PSMD11 in each tumor tissue as compared to normal tissue. Additionally, we generated plots illustrating the expression differences of PSMD11 between tumor and normal tissues specifically within LUAD. Subsequently, we employed the “time ROC” package in R (The R Foundation of Statistical Computing) to construct receiver operating characteristic (ROC) curves, evaluating the diagnostic performance of PSMD11 in distinguishing between tumor and normal tissues. An area under the curve (AUC) greater than 0.6 was considered indicative of a favorable diagnostic performance. Furthermore, the uniform manifold approximation and projection (UMAP) algorithm was employed for dimensionality reduction in single-cell data, with seven cellular subgroups being annotated. We then visualized the expression distribution of PSMD11 within these cellular subgroups. Finally, graphical representations were generated to illustrate the expression levels of PSMD11 across the identified seven cellular subgroups.
Differential expression of PSMD11 across distinct clinical traits
Using the median expression level of PSMD11 as a threshold, we categorized the tumor samples from the TCGA-LUAD dataset into low- and high-expression groups. Subsequently, we observed differences between these groups based on five clinical traits [age, gender, race, stage, overall survival (OS) event] and visualized the results through heatmap representations. Additionally, we individually plotted the expression disparities of PSMD11 across 12 distinct clinical traits (age, primary therapy outcome, residual tumor, number pack years smoked, OS event, location, pathologic T stage, anatomic neoplasm subdivision, pathologic N stage, pathologic M stage, pathologic stage, gender). Kaplan-Meier curves were employed to assess variations between the high- and low-expression groups under different survival types [OS, disease-specific survival (DSS), progression-free interval (PFI) (18)] within the TCGA-LUAD cohort.
Construction and validation of prognostic models
We employed univariate Cox regression analysis to verify whether factors including PSMD11, age, gender, and pathologic stage are OS risk factors for patients with LUAD. The results were visualized through forest plots. Subsequently, we introduced multivariate Cox regression analysis, creating forest plots to validate whether these factors are independent overall survival risk factors for patients with LUAD. Furthermore, we conducted univariate Cox analysis to assess the association of these factors on specific prognostic outcomes [OS, progression-free survival (PFS), DSS and PFI]. Incorporating three factors (PSMD11, age, and pathologic stage), we constructed a nomogram prognostic model to predict the 1-, 3-, and 5-year survival probabilities of patients. Calibration curves for 1-, 3-, and 5-year predictions were generated to validate the accuracy of the nomogram prognostic model. Finally, ROC curves were plotted to analyze the diagnostic performance of the nomogram prognostic model at 1, 3, and 5 years, with an AUC greater than 0.6 being considered indicative of favorable diagnostic performance.
Identification and functional enrichment analysis of differentially expressed genes (DEGs)
We conducted differential expression analysis on the TCGA-LUAD dataset, specifically comparing the low- and high-expression groups of PSMD11, leading to the identification of DEGs. The criteria for selecting DEGs were |log fold change (FC)| >1 and adjusted P<0.05. Subsequently, we subjected the identified DEGs to enrichment analysis using Gene Ontology (GO), Kyoto Encyclopedia of Genes and Genomes (KEGG), and gene set enrichment analysis (GSEA). The outcomes of these analyses were visually presented to facilitate interpretation and understanding.
Immune profile analysis
We generated a lollipop chart to illustrate the correlation between PSMD11 and 24 immune cell types. Spearman rank correlation was employed to analyze the correlation between the expression levels of PSMD11 and each immune cell type, and the results were visualized through scatter plots. Furthermore, leveraging the “GSVA” package, we employed the single-sample gene set enrichment analysis (ssGSEA) algorithm to create box plots, comparing the infiltration variances between the low- and high-expression groups across various immune cell types. Heatmaps were used to depict the expression correlations between PSMD11 and each gene within five gene sets [chemokine receptors, chemokines, immune activation genes, immunosuppressive genes, major histocompatibility complex (MHC) genes].
Tumor immune dysfunction and exclusion (TIDE) for immune escape and drug sensitivity analyses
We used a heatmap to illustrate the correlation between PSMD11 and the genes associated with immune escape. Additionally, we analyzed the expression differences of immune checkpoint genes between the low- and high-expression groups of PSMD11, presenting the results through box plots. TIDE analysis was employed to compare the differences in immune treatment response between the PSMD11 low- and high-expression groups, which were visualized through stacked bar charts. Box plots were used to depict the variances between the two groups in TIDE scores, dysfunction scores, and exclusion scores. Finally, we conducted an analysis of the sensitivity differences between the PSMD11 low- and high-expression groups to four drugs (camptothecin, axitinib, docetaxel, gefitinib), presenting the findings through visualizations.
Copper death genes and related analyses
In this study, we used R software to conduct a correlation analysis between the expression levels of PSMD11 and the copper death gene set, which was visually represented through a heatmap. Additionally, we performed Pearson correlation coefficient analysis comparing the expression levels of PSMD11 and three specific genes (DLAT, DLD, and PDHA1), and the results were graphically depicted using scatter plots. Subsequently, we generated box plots to illustrate the expression differences of various copper death genes between the low- and high-expression groups of PSMD11. Finally, employing the Kaplan-Meier algorithm, we plotted survival curves to depict the differences between the PSMD11 low- and high-expression groups across three survival categories.
Cell treatment
Human NSCLC cell lines A549 and H1299 were purchased from the Shanghai Cell Bank of the Chinese Academy of Science. These cell lines were cultured and expanded in Dulbecco’s modified Eagle’s medium (DMEM, Hyclone, Visalia, CA, USA), supplemented with 10% fetal bovine serum (FBS, Hyclone) in 5% CO2 humidified incubator (Thermo, Waltham, MA, USA) at 37 ℃.
Cell transfection
The siRNA for PMSD11 (siPSMD11) and negative control (siNC) were purchased from Tsingke (Beijing, China). The transfection mixture for each well was composed of 20 µL Lipofectamine 2000 (Invitrogen, Carlsbad, CA, USA), siRNAs (50 nM) and 980 µL Opti-MEM (Thermo). After transfection for 8 h, the medium was replaced with complete culture medium and incubated for another 40 h. The transfection efficacy was measured by quantitative real-time polymerase chain reaction (qRT-PCR) and western blotting assay.
RNA isolation and qRT-PCR
Total RNA was extracted from tumor tissues and cells using TRIzol reagent (Invitrogen) as per manufacturer’s protocol. RNA was then reverse transcribed to cDNA using the Thermo Scientific RevertAid RT (#K1691, Thermo). Then qRT-PCR was performed using a SuperScript™ III Platinum™ kit (#11732088, Thermo) according to the manufacturer’s instruction. Relative RNA expression was calculated following the 2−∆∆Ct method and normalized to GAPDH. Primers were synthesized by RiboBio (Guangzhou, China).
The primer sequences are as following:
- PSMD11: forward 5'-TCGCCTGGTCCGATCTCTT-3', reverse 5'-ATGCACTCTAAACACAGCTCG-3'.
- CD276: forward 5'-CTGGCTTTCGTGTGCTGGAGAA-3', reverse 5'-GCTGTCAGAGTGTTTCAGAGGC-3'.
- GAPDH: forward 5'-GGAGCGAGATCCCTCCAAAAT-3', reverse 5'-GGCTGTTGTCATACTTCTCATGG-3'.
Western blotting assay
Tumor tissues and cells were homogenized with RIPA lysis buffer to extract total proteins. After quantification with bicinchoninic acid (BCA) protein assay kit (Thermo), an equal amount of total proteins were loaded and separated in sodium dodecyl sulfate (SDS)-polyacrylamide gel electrophoresis (PAGE) and were transferred to polyvinylidene difluoride membranes (PVDF, Millipore, Bedford, MA, USA). The membranes were then blocked with 5% non-fat milk at room temperature for 2 h and probed with anti-PSMD11 (1:2,000, ab221645, Abcam), anti-CD276 (1:2,000, ab226256, Abcam), and anti-GAPDH (1:2,000, ab9485, Abcam) antibodies overnight at 4 ℃. Next day, the protein bands were incubated with HRP-conjugated goat-anti-mouse (ab6789, Abcam) and goat-anti-rabbit secondary antibody (ab6721, Abcam) at room temperature for 1 h. Protein bands were then visualized after incubation with SuperSignal West Pico PLUS reagent (#34577, Thermo).
Statistical analysis
All statistical analyses were conducted using R software version 4.1.3 software. Various R packages including “gmodels”, “tidyverse”, “ggplot2”, “ggsci”, and “ComplexHeatmap” were employed to generate the results. Drug sensitivity analysis was performed via the “oncoPredict” package. Data in this study are expressed as the mean ± standard deviation (SD) of three independent tests. The t-test and one-way analysis of variance (ANOVA) with the Tukey post hoc test were performed for intergroup comparison and multiple comparisons, respectively. The diagnostic value of PSMD11 was evaluated via the ROC curve. The correlation between PSMD11 with CD-276 levels was measured by the Pearson χ2 test. The diagnostic value of PSMD11 was evaluated by receiver operating characteristic (ROC) curve. Statistical significance was determined as a P value <0.05.
Results
Pancancer analysis and single-cell analysis of PSMD11
We conducted a pancancer analysis of PSMD11 and observed an elevated expression of PSMD11 in tumor tissues across various diseases (Figure 1A). Subsequently, we analyzed the expression differences of PSMD11 between tumor and normal groups in the TCGA-LUAD dataset, revealing a significantly higher expression of PSMD11 in tumor tissues (P<0.001) (Figure 1B,1C). The ROC curve analysis for PSMD11 yielded an AUC value of 0.837, demonstrating its high efficacy in discriminating between tumor and normal tissues (Figure 1D). Employing the UMAP algorithm for dimensionality reduction in single-cell sequencing analysis, we annotated seven distinct cellular subgroups based on marker genes [macrophages, epithelial cells, myeloid cells, endothelial cells, dendritic cells (DCs), CD4 T cells, B cells] (Figure 1E). Furthermore, we delineated the expression distribution of PSMD11 within these cellular subgroups (Figure 1F). Our investigation into the expression patterns of PSMD11 across different cellular subgroups revealed an elevated expression in CD4 T cells, endothelial cells, epithelial cells, and macrophages and a lower expression in B cells, DCs, and myeloid cells (Figure 1G).
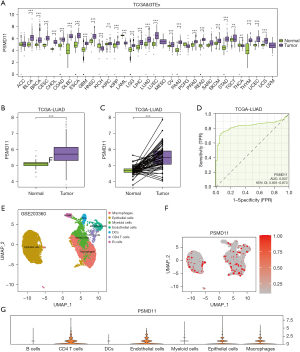
Correlation between PSMD11 expression and clinical characteristics
We collected data and summarized the differences in clinical characteristics between the high- and low-expression levels of PSMD11 in a table (Table S1). For the three clinical characteristics evaluated (age, stage, and OS event), we observed significant differences between the high- and low-expression groups of PSMD11 (P<0.001; Figure 2A). Patients with a smoking history with more than 40 pack-years had a higher expression of PSMD11 (P<0.05; Figure 2B). Additionally, we noted differences in PSMD11 expression between different primary treatment stages, with patients in the progressive disease (PD) group showing the highest PSMD11 expression (P<0.05), while those in stable disease (SD) group showing the lowest PSMD11 expression (P<0.05; Figure 2C).
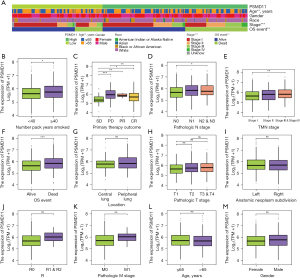
Moreover, in the pathologic N stage, we observed a high expression of PSMD11 in the N2 and N3 stage groups (P<0.05; Figure 2D). A high expression of PSMD11 was also detected in patients with stage 3 and stage 4 disease (P<0.01; Figure 2E). In the OS event group, we found a high expression of PSMD11 in the deceased group and low expression in the surviving group (P<0.001; Figure 2F). There were no statistically significant differences observed for other clinical characteristics (Figure 2G-2M).
Survival curves indicated that in the OS survival curve, regardless of age and pathological stage, the prognosis of the PSMD11 high-expression group was inferior to that of the low-expression group, but smoking status, number pack years smoked, and pathologic N stage were relevant in the PSMD11 high-expression group having a poorer prognosis compared to the low-expression group (P<0.05; Figure S1). In the PFS survival curve, irrespective of age and smoking status, the prognosis of the PSMD11 high-expression group was consistently worse than that of the low-expression group (P<0.05, Figure S2). In the DSS survival curve, among patients aged over 65 years, those who smoked history with less than 40 pack-years, and patients with stage 1 and stage 2, N0 and N1, or M0 disease in the PSMD11 high-expression group demonstrated inferior prognosis compared to those in the low-expression group, but the remaining groups did not exhibit statistically significant differences (P<0.05; Figure S3).
Construction and validation of prognostic models
We conducted univariate Cox regression analyses for different survival outcomes (OS, PFS, DSS), generating scatter plots for single factor Cox regression analysis and forest plots for multivariate Cox regression analysis, both of which identified PSMD11 as a prognostic risk factor in terms of OS, PFS, and DSS (P<0.05; Figure 3A,3B and Figure S4A,S4B). Furthermore, we developed a nomogram survival model (Figure 3C). The calibration curve results demonstrated the high accuracy of our model (Figure 3D), and the ROC curves for 1-, 3-, and 5-year survival all had AUC values >0.6, indicating the excellent performance of the model (Figure 3E-3G).
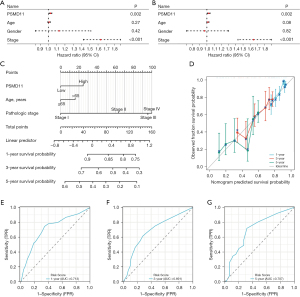
Identification and functional enrichment analysis of DEGs
Detailed information on the DEGs can be found in table available at https://cdn.amegroups.cn/static/public/jtd-24-1622-1.xlsx. Our investigation revealed that DEGs were enriched in 10 pathways, including systemic lupus erythematosus, neuroactive ligand-receptor interaction, alcoholism, neutrophil extracellular trap formation, taste transduction, olfactory transduction, retinol metabolism, nicotine addiction, drug metabolism-cytochrome P450, and pentose and glucoronate interconversions (Figure 4A).
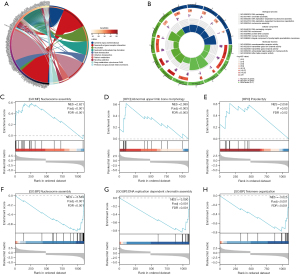
Further analysis showed that DEGs were predominantly enriched in pathways related to DNA replication and membrane-gated channel functions (Figure 4B). The enrichment score line plots demonstrated upregulation in the high-expression group for histone deacetylase binding, abnormal upper limb bone morphology, and polydactyly [normalized enrichment score (NES) >2.0; false discovery rate (FDR) <0.05; Figure 4C-4E]. Conversely, nucleosome assembly, DNA replication-dependent chromatin assembly, and telomere organization were upregulated in the low-expression group (NES <−3.0; FDR <0.001; Figure 4F-4H).
Immunological correlation and drug sensitivity analysis via TIDE
In our immune infiltration analysis, we observed that PSMD11 exhibited the strongest positive correlation with Th2 cell abundance (R=0.411; P<0.001). Moreover, PSMD11 was positively correlated with Tgd cell abundance (R=0.214; P<0.001). Conversely, PSMD11 demonstrated the highest negative correlation with the abundance of B cells (R=−0.299; P<0.001) and mast cells (R=−0.261; P<0.001). Notably, PSMD11 had a predominantly negative correlation with most immune cells (Figure 5A). These findings were further validated by the scatter plots (P<0.05; Figure 5B-5E; Figure S5A-S5L).
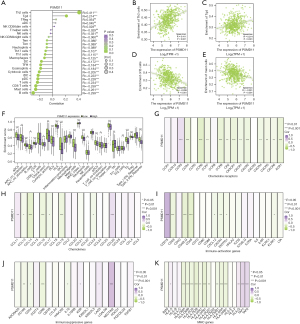
Through analysis of box plots, we concluded that the PSMD11 low-expression group exhibited relatively higher immune infiltration scores (P<0.05). Specifically, the median score of the MHC class I group was higher than that of the other groups (P<0.001); meanwhile, the median score of natural killer (NK) cell group was lower than that of other groups (P<0.05) (Figure 5F). Heatmap analysis revealed that PSMD11 demonstrated a negative correlation with the expression of a majority of genes in the chemokine receptor gene set (P<0.01), whereas it had a positive correlation with the expression of the CCR3 gene (P<0.001; Figure 5G). Additionally, PSMD11 exhibited a negative correlation with the expression of most genes in the chemokine gene set (P<0.05) and exhibited a negative correlation with CCL26 gene expression (P<0.001; Figure 5H).
Furthermore, PSMD11 exhibited a predominantly negative correlation with most genes in the immune activation gene set (P<0.05; Figure 5I) and a positive correlation with the expression of the majority of genes in the immunosuppressive gene set (P<0.05, Figure 5J). Moreover, a substantial negative correlation was observed between PSMD11 and the expression of most genes in the MHC gene set (P<0.05; Figure 5K). The heatmap indicated that PSMD11 was negatively correlated with most genes in the immune escape-related genes (P<0.05) but showed a positive correlation with CD276 gene expression (P<0.001; Figure 6A). We validated that the knockdown of PSMD11 reduced the expression of CD276 in A549 and H1299 cells but failed to affect the mRNA expression of CD276 in A549 and H1299 cells (Figure S6A,S6B).
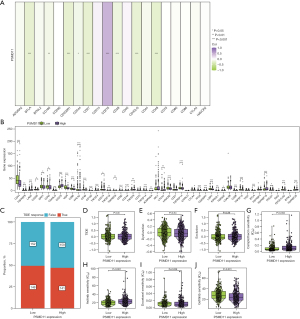
Additionally, based on the box plots, it was evident that most immune checkpoint genes were downregulated in the PSMD11 high-expression group (P<0.05), while most immune checkpoint genes were upregulated in the low-expression group (P<0.05; Figure 6B). TIDE analysis indicated that the differences between the two expression groups of PSMD11 was not statistically significant, implying an absence of statistical association between PSMD11 expression and TIDE (Figure 6C-6F).
Regarding drug sensitivity analysis, we observed that the low-expression group exhibited higher sensitivity to camptothecin and axitinib (P<0.01, Figure 6G,6H), whereas the high-expression group had a higher sensitivity to docetaxel and gefitinib (P<0.05; Figure 6I,6J).
Correlation between expression levels of PSMD11 and cuproptosis genes
In our investigation, we observed that high PSMD11 expression was associated with the majority of the cuproptosis genes (P<0.05, Figure 7A). Additionally, we found that the expression of DLAT, DLD, and PDHA1 was positively correlated with the expression of PSMD11 (P<0.001; Figure 7B-7D). Analyzing the box plots representing the expression differences between PSMD11 and cuproptosis genes, we concluded that in the PSMD11 high-expression group, most of cuproptosis genes were upregulated (P<0.05), while in the low-expression group, most of the cuproptosis genes were downregulated (P<0.05; Figure 7E). Notably, the expression level of DLST in different PSMD11 groups was higher than that of the other genes (P<0.05; Figure 7E).
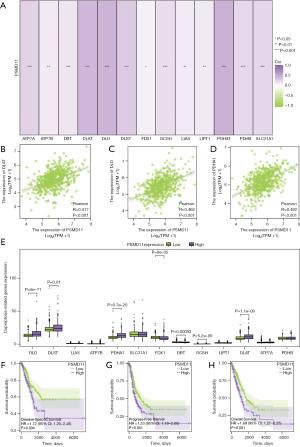
Survival analysis using Kaplan-Meier curves revealed that the prognosis of the PSMD11 high-expression group was inferior to that of the low-expression group in terms of DSS, PFI, and OS curves (P<0.01; Figure 7F-7H).
Discussion
With the highest incidence among cancers, lung cancer is a significant global health issue, responsible for the bulk of cancer-related illnesses and deaths (19). Despite the introduction of promising novel therapies, pertinent epidemiological data have shown that those with LUAD have a poor prognosis due to late diagnosis and resistance to anticancer therapy (2). The significant tumor heterogeneity—both intra- and intertumoral—that dictates the many cellular and molecular features of the disease is one of the primary treatment problems for LUAD. In this study, we aimed comprehensively explore the function of PSMD11 in the emergence and progression of LUAD.
PSMD11 has been proven to regulate the breakdown of ubiquitinated proteins and be associated with tumor progression (12,14,15). However, the prognostic value and potential biological function of PMSD11 in LUAD had been unclear. In this study, we constructed a pancancer analysis of PSMD11, observed the elevated expression of PSMD11 in tumor tissues across various diseases, and found a significantly higher expression of PSMD11 in tumor tissues by analyzing the PSMD11 expression differences between tumor and normal tissues in TCGA-LUAD dataset. Subsequently, we delineated the expression distribution of PSMD11 within seven cellular subgroups in single-cell sequencing data, which demonstrated PSMD11 had a higher expression in CD4 T-cells, endothelial cells, epithelial cells, and macrophages. Moreover, PSMD11 was expressed not only in malignant tumor cell but also in immune cells, suggesting that PSMD11 may shape the tumor microenvironment and contribute to immune escape in LUAD.
Additionally, we investigated the prognostic value of PSMD11 by collecting data and examining the differences in clinical characteristics between the PSMD11 high- and low-expression levels in patients with LUAD. We identified three clinical characteristics (age, stage, and OS event) that were significantly different between the high- and low-PSMD11 expression groups. Notably, patients with PD stage and lymph node metastasis stage had the highest PSMD11 expression. These results demonstrated that the PSMD11 significantly associated with malignant tumor progression and lymph nodes metastasis in LUAD. These findings are consistent with those suggesting the result that overexpression of PSMD11 enhances the proliferation, migration, invasion, and tumor growth of lung carcinoma cells (13). Furthermore, we conducted univariate Cox regression analyses for different survival outcomes (OS, PFS, DSS) and generated scatter plots for single-factor Cox regression analysis and forest plots for multivariate Cox regression analysis. These results suggested PSMD11 to be a prognostic risk factor in OS, PFS, and DSS. Moreover, we developed a nomogram survival model that demonstrated good accuracy. Therefore, PSMD11 may have considerable prognostic value in patients with LUAD.
We analyzed the DEGs to explore the downstream pathways regulated by a high level of PSMD11 expression in LUAD. The subsequent results showed that DEGs were predominantly enriched in pathways related to DNA replication and membrane-gated channel functions. In eukaryotic cells, DNA replication is critical for the maintenance of genomic integrity. A variety of cellular stresses affecting DNA replication, which are collectively termed replication stress, cause genomic instability in both normal and cancer cells (20). Therefore, DNA replication stress is a major factor causing genomic instability and is crucial cancer cell vulnerability (21,22). Further analysis of enrichment score line plots indicated that nucleosome assembly, DNA replication-dependent chromatin assembly, and telomere organization were upregulated in the low-expression group. These results suggest the high levels of DNA damage which result to DNA replication stress happened in LUAD with high PSMD11 expression.
The tumor microenvironment comprises neoplastic cells, stromal cells, and diversity of immune cells, and these components interact through complex cellular and molecular mechanisms, which collectively determine tumor progression, metastasis, and clinical outcomes (23). There is a growing body of evidence indicating that the tumor microenvironment plays a key role in the development and prognosis of LUAD (24,25). In our immune infiltration analysis, we observed that PSMD11 exhibited the strongest positive correlation with Th2, Tgd, and Treg cells and the highest negative correlation with B, mast, and CD8+ T cells. These immune cells may both contribute to hinder tumor invasiveness: CD8+ T cells and NK cells mediate antitumoral responses and are associated with better prognosis. Conversely, Tregs secrete inhibitory cytokines, such as transforming growth factor (TGF)-β and interleukin (IL)-10, contributing to cancer progression via angiogenesis and immunosuppression through inhibiting the antitumor effect of T-helper 1 (Th1) cells and attracting activated Th2 cells (26-28). Th2 cells and their secreted cytokines are associated with an unfavorable prognosis in LUAD (29). Th2 cells can promote immunosuppression by increasing the population of M2 macrophages in the tumor microenvironment via secreting IL-5 and IL-13 (30). Malignant tumors, in facilitating their progression, activate immune checkpoints to establish immunosuppressive microenvironments. The heatmap in our study illustrated that PSMD11 is negatively correlated with most immune escape-related genes,but is a positively correlated with CD276 gene expression (P<0.001). We further explore the relationship between PSMD11 and CD267 in NSCLC cell line and demonstrated PSMD11 affect the protein level of CD276 but cannot affect the mRNA level of PSMD11. PSMD11 is a ubiquitinated proteins able to ubiquitin the protein of CD276. More studies will be required to clarify this discrepancy.
Although our study provides strong evidence to support PSMD11 as a prognostic marker and clarifies the potential biological mechanism of progression in LUAD, several limitations should be addressed. First, our study only involved public databases, and verification of our findings and elucidation of potential biological mechanisms of LUAD should be examined in vitro and in vivo. Second, further validation should be performed in clinical LUAD samples to minimize errors. Third, those patients with LUAD had been treated by differently strategies, which could cause biases in clinical outcomes. These limitations notwithstanding, our investigation suggests that PSMD11 may be valuable for informing prognosis and represents a potential avenue for innovative therapeutic interventions in patients with LUAD.
Conclusions
In this study, we comprehensively and systematically analyzed the association between PSMD11 expression and clinical characteristics, prognostic value, and potential biological mechanisms of progression in LUAD. The results may advance LUAD research and aid clinicians in accurately predicting the prognosis of patients with LUAD and improving personalized treatment strategies.
Acknowledgments
Funding: This work was supported by
Footnote
Reporting Checklist: The authors have completed the TRIPOD reporting checklist. Available at https://jtd.amegroups.com/article/view/10.21037/jtd-24-1622/rc
Data Sharing Statement: Available at https://jtd.amegroups.com/article/view/10.21037/jtd-24-1622/dss
Peer Review File: Available at https://jtd.amegroups.com/article/view/10.21037/jtd-24-1622/prf
Conflicts of Interest: All authors have completed the ICMJE uniform disclosure form (available at https://jtd.amegroups.com/article/view/10.21037/jtd-24-1622/coif). The authors have no conflicts of interest to declare.
Ethical Statement: The authors are accountable for all aspects of the work in ensuring that questions related to the accuracy or integrity of any part of the work are appropriately investigated and resolved. The study was conducted in accordance with the Declaration of Helsinki (as revised in 2013).
Open Access Statement: This is an Open Access article distributed in accordance with the Creative Commons Attribution-NonCommercial-NoDerivs 4.0 International License (CC BY-NC-ND 4.0), which permits the non-commercial replication and distribution of the article with the strict proviso that no changes or edits are made and the original work is properly cited (including links to both the formal publication through the relevant DOI and the license). See: https://creativecommons.org/licenses/by-nc-nd/4.0/.
References
- Bray F, Laversanne M, Sung H, et al. Global cancer statistics 2022: GLOBOCAN estimates of incidence and mortality worldwide for 36 cancers in 185 countries. CA Cancer J Clin 2024;74:229-63. [Crossref] [PubMed]
- Succony L, Rassl DM, Barker AP, et al. Adenocarcinoma spectrum lesions of the lung: Detection, pathology and treatment strategies. Cancer Treat Rev 2021;99:102237. [Crossref] [PubMed]
- Devarakonda S, Morgensztern D, Govindan R. Genomic alterations in lung adenocarcinoma. Lancet Oncol 2015;16:e342-51. [Crossref] [PubMed]
- Xi Y, Xi L, Tan J, et al. Comprehensive analysis of lung adenocarcinoma: Unveiling differential gene expression, survival-linked genes, subtype stratification, and immune landscape implications. Environ Toxicol 2024; Epub ahead of print. [Crossref]
- Li F, Li F, Zhao D, et al. Predictors of cancer-specific survival and overall survival among patients aged ≥60 years with lung adenocarcinoma using the SEER database. J Int Med Res 2024;52:3000605241240993. [Crossref] [PubMed]
- Magnati S, Alladio E, Bracco E. A Survey on the Expression of the Ubiquitin Proteasome System Components HECT- and RBR-E3 Ubiquitin Ligases and E2 Ubiquitin-Conjugating and E1 Ubiquitin-Activating Enzymes during Human Brain Development. Int J Mol Sci 2024;25:2361. [Crossref] [PubMed]
- Adams J. The proteasome: structure, function, and role in the cell. Cancer Treat Rev 2003;29:3-9. [Crossref] [PubMed]
- Spano D, Catara G. Targeting the Ubiquitin-Proteasome System and Recent Advances in Cancer Therapy. Cells 2023;13:29. [Crossref] [PubMed]
- Liu X, Wang M, Wang Q, et al. A ubiquitin-proteasome system-related signature to predict prognosis, immune infiltration, and therapy efficacy for breast cancer. Immunol Res 2024;72:368-82. [Crossref] [PubMed]
- Li Y, Liu X, Zhao F, et al. Comprehensive analysis of PSMD family members and validation of PSMD9 as a potential therapeutic target in human glioblastoma. CNS Neurosci Ther 2024;30:e14366. [Crossref] [PubMed]
- Xuan DTM, Wu CC, Kao TJ, et al. Prognostic and immune infiltration signatures of proteasome 26S subunit, non-ATPase (PSMD) family genes in breast cancer patients. Aging (Albany NY) 2021;13:24882-913. [Crossref] [PubMed]
- Moreno D, Viana R, Sanz P. Two-hybrid analysis identifies PSMD11, a non-ATPase subunit of the proteasome, as a novel interaction partner of AMP-activated protein kinase. Int J Biochem Cell Biol 2009;41:2431-9. [Crossref] [PubMed]
- Huang Q, Tian R, Yu J, et al. Identification of PSMD11 as a novel cuproptosis- and immune-related prognostic biomarker promoting lung adenocarcinoma progression. Cancer Med 2024;13:e7379. [Crossref] [PubMed]
- Sahni S, Krisp C, Molloy MP, et al. PSMD11, PTPRM and PTPRB as novel biomarkers of pancreatic cancer progression. Biochim Biophys Acta Gen Subj 2020;1864:129682. [Crossref] [PubMed]
- Zhang C, Xu T, Ji K, et al. An integrative analysis reveals the prognostic value and potential functions of PSMD11 in hepatocellular carcinoma. Mol Carcinog 2023;62:1355-68. [Crossref] [PubMed]
- Sun L, Liu Z, Wu Z, et al. PSMD11 promotes the proliferation of hepatocellular carcinoma by regulating the ubiquitination degradation of CDK4. Cell Signal 2024;121:111279. [Crossref] [PubMed]
- Wang L, Zhao L, Wei G, et al. Homoharringtonine could induce quick protein synthesis of PSMD11 through activating MEK1/ERK1/2 signaling pathway in pancreatic cancer cells. J Cell Biochem 2018;119:6644-56. [Crossref] [PubMed]
- Alexandrov LB, Nik-Zainal S, Wedge DC, et al. Signatures of mutational processes in human cancer. Nature 2013;500:415-21. [Crossref] [PubMed]
- Ferlay J, Colombet M, Soerjomataram I, et al. Cancer statistics for the year 2020: An overview. Int J Cancer 2021; Epub ahead of print. [Crossref]
- Saxena S, Zou L. Hallmarks of DNA replication stress. Mol Cell 2022;82:2298-314. [Crossref] [PubMed]
- da Costa AABA, Chowdhury D, Shapiro GI, et al. Targeting replication stress in cancer therapy. Nat Rev Drug Discov 2023;22:38-58. [Crossref] [PubMed]
- Zhang J, Dai Q, Park D, et al. Targeting DNA Replication Stress for Cancer Therapy. Genes (Basel) 2016;7:51. [Crossref] [PubMed]
- Bharadwaj D, Mandal M. Tumor microenvironment: A playground for cells from multiple diverse origins. Biochim Biophys Acta Rev Cancer 2024;1879:189158. [Crossref] [PubMed]
- Kanemura H, Yokoyama T, Nakajima R, et al. The Tumor Immune Microenvironment Is Associated With Recurrence in Early-Stage Lung Adenocarcinoma. JTO Clin Res Rep 2024;5:100658. [Crossref] [PubMed]
- Sun X, Meng F, Nong M, et al. Single-cell dissection reveals the role of aggrephagy patterns in tumor microenvironment components aiding predicting prognosis and immunotherapy on lung adenocarcinoma. Aging (Albany NY) 2023;15:14333-71. [Crossref] [PubMed]
- Lambrechts D, Wauters E, Boeckx B, et al. Phenotype molding of stromal cells in the lung tumor microenvironment. Nat Med 2018;24:1277-89. [Crossref] [PubMed]
- Kim HR, Park HJ, Son J, et al. Tumor microenvironment dictates regulatory T cell phenotype: Upregulated immune checkpoints reinforce suppressive function. J Immunother Cancer 2019;7:339. [Crossref] [PubMed]
- Chen K, Zhu Z, Zhang N, et al. Tumor-Infiltrating CD4+ Lymphocytes Predict a Favorable Survival in Patients with Operable Esophageal Squamous Cell Carcinoma. Med Sci Monit 2017;23:4619-32. [Crossref] [PubMed]
- Zuo S, Wei M, Wang S, et al. Pan-Cancer Analysis of Immune Cell Infiltration Identifies a Prognostic Immune-Cell Characteristic Score (ICCS) in Lung Adenocarcinoma. Front Immunol 2020;11:1218. [Crossref] [PubMed]
- Badmann S, Heublein S, Mayr D, et al. M2 Macrophages Infiltrating Epithelial Ovarian Cancer Express MDR1: A Feature That May Account for the Poor Prognosis. Cells 2020;9:1224. [Crossref] [PubMed]