Inflammatory markers as predictors of in-hospital mortality in acute exacerbation of chronic obstructive pulmonary disease (AECOPD) patients with acute respiratory failure: insights from the MIMIC-IV database
Highlight box
Key findings
• The in-hospital mortality risk in acute exacerbation of chronic obstructive pulmonary disease (AECOPD) and acute respiratory failure (ARF) patients increased along with the elevated the neutrophil-to-lymphocyte ratio (NLR) and glucose-to-lymphocyte ratio (GLR), while prognostic nutritional index (PNI) showed a negative correlation. In addition, restricted cubic spline (RCS) model indicated a non-linear relationship between NLR and in-hospital mortality, with a significant increase in risk observed when NLR exceeded 6.66. Additionally, a U-shaped curve was noted for PNI, with an inflection point at 43.54, less than which the risk of mortality increased significantly. For GLR, the RCS analysis indicated a linear increase in mortality risk.
What is known and what is new?
• Previous research has established the link between AECOPD, systemic inflammation, and increased mortality risk. Inflammatory markers can predict adverse outcomes in AECOPD.
• NLR, GLR, and PNI have moderate predictive utility for in-hospital mortality in patients with AECOPD and ARF, providing some references for exploring prognostic biomarkers.
What is the implication, and what should change now?
• Focusing on NLR, GLR, and PNI in AECOPD with ARF patients and keeping them within appropriate ranges may be important for those who are with a high risk of in-hospital mortality. Further prospective studies over longer follow-up periods are warranted to establish the potential clinical use of the NLR, GLR, and PNI, singly or as part of a combined predictive model, in early risk stratification and reducing the burden of disease.
Introduction
Chronic obstructive pulmonary disease (COPD) is a widespread chronic respiratory illness that progressively worsens symptoms and deteriorates quality of life. The Global Burden of Disease Study 2019 identifies COPD as the world’s third leading cause of death, responsible for 3.3 million deaths in 2019, thus imposing a substantial societal burden (1). According to the updated Global Initiative for Chronic Obstructive Lung Disease (GOLD) 2024 recommendations, an exacerbation of COPD is defined as an event characterized by dyspnea and/or cough and sputum that worsen in <14 days, which may be accompanied by tachypnea and/or tachycardia and is often associated with increased local and systemic inflammation caused by infection, pollution, or other insult to the airways. These events can be life-threatening and require adequate evaluation and treatment (2). Additionally, acute exacerbation of COPD (AECOPD) is associated with significant hospitalization and mortality, along with health and socioeconomic burdens (2). Acute respiratory failure (ARF) stands out as a common and severe complication among patients with AECOPD (3,4), especially in severe cases requiring mechanical ventilation. Studies indicate markedly high mortality rates for these patients: 20% in-hospital, 48% at 1 year, 58% at 2 years, and 64% at 3 years post-admission (5,6). Early identification of the risks associated with AECOPD and ARF is vital for initiating timely and appropriate interventions. Therefore, it is meaningful to investigate the reliable and accessible biomarkers or predictive indexes on the risk of in-hospital mortality for patients with AECOPD and ARF.
COPD exacerbations typically signal a significant flare-up of inflammatory processes. Both airway and systemic inflammation in AECOPD are linked to disease progression and mortality (7-9). Previous studies have shown the association between AECOPD and various peripheral blood immunoinflammatory cells and molecules, such as neutrophils, eosinophils, lymphocytes, platelets, C-reactive protein (CRP), and fibrinogen (7,10,11). Beyond these, the neutrophil-to-lymphocyte ratio (NLR) and the platelet-to-lymphocyte ratio (PLR) have been reported to be associated with adverse outcomes in hospitalized AECOPD patients (12,13). Another study found that the systemic immune-inflammation index (SII) log-level was associated with COPD risk, lung function, and COPD severity (14). The glucose-to-lymphocyte ratio (GLR) has been assessed for its predictive capacity regarding in-hospital mortality in intensive care unit (ICU)-admitted AECOPD patients (15). The lactate-to-albumin ratio (LAR) was reported to be associated with 28-day mortality in ICU patients with AECOPD (16). Additionally, decreased prognostic nutritional index (PNI) levels at admission were showed to be associated with adverse hospitalization outcomes in patients with AECOPD (17) and indicated a heightened exacerbation risk in elderly COPD patients (18). Taken together, the aforementioned studies have explored the impact of inflammatory biomarkers (NLR, LAR, GLR, SII, PNI, and PLR) on prognosis of patients with AECOPD, few have examined their correlation with AECOPD in patients also suffering from ARF. Whether these inflammatory biomarkers (NLR, LAR, GLR, SII, PNI, and PLR) are prognostic factors for in-hospital mortality in AECOPD and ARF patients is unclear. This study aims to elucidate the prognostic value of NLR, LAR, GLR, SII, PNI, and PLR for in-hospital mortality among AECOPD patients with ARF using data from the Medical Information Mart for Intensive Care IV (MIMIC-IV). We present this article in accordance with the STROBE reporting checklist (available at https://jtd.amegroups.com/article/view/10.21037/jtd-24-1287/rc).
Methods
The study was conducted in accordance with the Declaration of Helsinki (as revised in 2013).
Data source
This retrospective, observational study utilized the MIMIC-IV database (version 2.2), which comprises high-quality medical records of over 250,000 patients admitted to the ICU or emergency room of Beth Israel Deaconess Medical Center (BIDMC) from 2008 through 2019 (19). Access to the MIMIC-IV database was granted to the first author, Qimin Wang (certification ID: 54755644), under the approval of the BIDMC Institutional Review Board. The dataset was de-identified in compliance with privacy regulations, thus negating the need for informed consent.
Study population
The study population was selected using structured query language (SQL) queries to identify patients diagnosed with both AECOPD and ARF within the MIMIC-IV dataset. Primary diagnosis of AECOPD was identified by International Classification of Diseases (ICD)-9 codes 491.21, 491.22 and ICD-10 codes J44.0, J44.1 (20,21). Primary diagnosis of ARF was identified using ICD-9 codes 51881, 51884 and ICD-10 codes J96.0, J96.00, J96.01, J96.02. Patients who were diagnosed with both AECOPD and ARF were included in the study. Unfortunately, the lung function test and annual frequency of exacerbations were not available for this dataset to conduct comprehensive assessment for AECOPD. Only the first hospitalization records were used for patients with multiple admissions to minimize selection bias. Exclusion criteria included patients with incomplete documentation or essential medical records and those missing survival outcome data. The patient selection process is detailed in Figure 1.
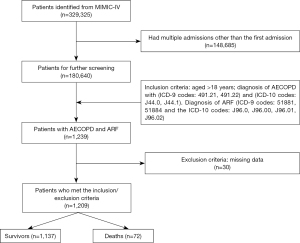
Data extraction and outcome
PostgreSQL 15 software was utilized for data extraction from the MIMIC-IV database. The following variables were extracted for the first time they were measured following the patients’ first hospital admission for analysis: age, gender, race, body mass index (BMI), heart rate (HR), respiratory rate (RR), laboratory parameters including white blood cell (WBC) count, neutrophil count, lymphocyte count, eosinophil count, platelet count, albumin, glucose, lactate, serum blood urea nitrogen (BUN), serum sodium, arterial blood gases [pH, partial pressure of oxygen (pO2), and partial pressure of carbon dioxide (pCO2)], and comorbidities [congestive heart failure (CHF), chronic kidney disease (CKD), malignancy]. The severity of clinical conditions was quantified using the Sequential Organ Failure Assessment (SOFA) score (22) and the Simplified Acute Physiology Score II (SAPS II) (23). Data on treatment therapies such as ipratropium bromide were extracted; However, variables with over 30% missing data, including invasive mechanical ventilation (IMV) and vasopressor use, were excluded from the analysis.
The dependent variables in this study were inflammatory indices including the NLR, LAR, GLR, SII, PLR, and PNI. The formulas used were: NLR: neutrophil count divided by lymphocyte count; LAR: lactate divided by albumin; PLR: platelet count divided by lymphocyte count; GLR: fasting blood glucose divided by lymphocyte count; SII: product of platelet count and the ratio of neutrophil to lymphocyte count; PNI: 10 times the albumin concentration (g/dL) plus 5 times the lymphocyte count (×109/L) (18).
The primary endpoint of this study was in-hospital mortality, evaluated through in-hospital survival records.
Statistical analysis
To mitigate bias from sample exclusion, the percentage of missing values was calculated for each variable, and multiple imputation techniques were employed to estimate missing values for variables with less than 30% missing data; variables exceeding this threshold were omitted from the analysis. Baseline characteristics of patients were summarized as mean ± standard deviation (SD) for normally distributed variables, median with interquartile range (IQR) for non-normally distributed variables, and proportions for categorical variables. Differences were assessed using t-tests, Kruskal-Wallis tests, or Chi-squared tests as appropriate. Multivariable logistic regression was used to analyze the independent association of inflammatory indicators (NLR, PLR, SII, GLR, LAR, and PNI) with in-hospital mortality in AECOPD and ARF patients. The models varied by adjustments: Model 1 adjusted for no covariates; Model 2 for age, sex, race, and BMI; and Model 3 included additional adjustments for HR, RR, WBC, BUN, CHF, CKD, malignancy, SOFA score, SAPS II score, and ipratropium bromide use. Results were presented as odds ratios (ORs) with their respective 95% confidence intervals (CIs). Receiver operating characteristic (ROC) curves were employed to determine the area under the curve (AUC) for each inflammatory marker across the different models, assessing their predictive accuracy. Additionally, a restricted cubic spline (RCS) model was constructed to explore potential dose-response relationships between inflammatory markers and mortality. All statistical tests were conducted as two-sided, with a significance level set at P<0.05. Data analysis was performed using R software (version 4.2.1) with RStudio (version 2022.07.2+567).
Results
Basic characteristics of patients with AECOPD and ARF
The study enrolled 1,209 patients diagnosed with AECOPD and ARF. The median age was 70.00 (61.00, 78.00) years, with 576 patients (47.64%) being male. Of these, 72 patients died in hospital, representing 5.96% of the cohort. Table 1 presents the characteristics of participants. A significant age disparity was noted between the deceased and surviving patients, with the former being notably older [age (years): 76.00 (69.75, 82.25) vs. 70.00 (61.00, 78.00), P<0.001]. Comprehensive analyses revealed significantly differences between the deceased and surviving patients in several clinical and hematological parameters, including WBC count (K/µL) [12.00 (9.07, 15.90) vs. 9.10 (6.90, 12.10), P<0.001], neutrophil count (K/µL) [11.17 (8.73, 15.46) vs. 7.52 (4.96, 11.35), P<0.001], lymphocyte count (K/µL) [0.83 (0.51, 1.43) vs. 1.18 (0.70, 1.86), P=0.006], eosinophils (K/µL) [0.01 (0.00, 0.17) vs. 0.08 (0.01, 0.22), P=0.03], platelet count (K/µL) [202.50 (146.00, 296.00) vs. 243.00 (186.00, 305.00), P=0.004], BUN (mg/dL) [29.50 (18.75, 39.25) vs. 19.00 (14.00, 28.00), P<0.001], glucose (mg/dL) [136.50 (109.50, 178.25) vs. 116.00 (96.00, 153.00), P=0.001], albumin (g/dL) [3.30 (2.70, 3.90) vs. 3.80 (3.20, 4.20), P<0.001], SOFA score [8.00 (5.00, 11.00) vs. 4.00 (2.00,7.00), P<0.001], SAPS II [48.00 (39.75, 57.50) vs. 37.00 (29.00, 45.00), P<0.001], and inflammatory indices such as NLR, GLR, PNI, LAR, and SII.
Table 1
Variables | Total (n=1,209) | Survival group (n=1,137) | Death group (n=72) | P value |
---|---|---|---|---|
Age (years) | 70.00 (61.00, 78.00) | 70.00 (61.00, 78.00) | 76.00 (69.75, 82.25) | <0.001 |
Gender | 0.21 | |||
Female | 633 (52.36) | 601 (52.86) | 32 (44.44) | |
Male | 576 (47.64) | 536 (47.14) | 40 (55.56) | |
Race | 0.20 | |||
White | 411 (34.00) | 381 (33.51) | 30 (41.67) | |
Others† | 798 (66.00) | 756 (66.49) | 42 (58.33) | |
BMI (kg/m2) | 28.40 (23.90, 34.00) | 28.40 (24.00, 33.90) | 27.30 (23.67, 34.90) | 0.79 |
HR (beats/min) | 90.00 (78.00, 104.00) | 89.00 (78.00, 103.00) | 94.50 (74.75, 114.00) | 0.23 |
RR (beats/min) | 20.00 (17.00, 25.00) | 20.00 (17.00, 25.00) | 21.00 (18.00, 25.00) | 0.49 |
pH | 7.36 (7.29, 7.41) | 7.36 (7.29, 7.41) | 7.36 (7.29, 7.41) | 0.36 |
pO2 (mmHg) | 72.00 (49.00, 113.00) | 72.00 (49.00, 113.00) | 72.00 (49.00, 113.00) | 0.52 |
pCO2 (mmHg) | 48.00 (41.00, 60.00) | 48.00 (41.00, 60.00) | 48.00 (41.00, 60.00) | 0.65 |
WBC (K/μL) | 9.30 (7.00, 12.40) | 9.10 (6.90, 12.10) | 12.00 (9.07, 15.90) | <0.001 |
Neutrophil (K/μL) | 7.64 (5.07, 11.50) | 7.52 (4.96, 11.35) | 11.17 (8.73, 15.46) | <0.001 |
Lymphocytes (K/μL) | 1.18 (0.68, 1.85) | 1.18 (0.70, 1.86) | 0.83 (0.51, 1.43) | 0.006 |
Eosinophils (K/μL) | 0.08 (0.01, 0.21) | 0.08 (0.01, 0.22) | 0.01 (0.00, 0.17) | 0.03 |
Platelets (K/μL) | 241.00 (185.00, 305.00) | 243.00 (186.00, 305.00) | 202.50 (146.00, 296.00) | 0.004 |
Lactate (mmol/L) | 1.50 (1.10, 2.20) | 1.50 (1.10, 2.20) | 1.70 (1.30, 2.32) | 0.059 |
Fasting blood-glucose (mg/dL) | 117.00 (96.00, 156.00) | 116.00 (96.00, 153.00) | 136.50 (109.50, 178.25) | 0.001 |
Albumin (g/dL) | 3.70 (3.20, 4.10) | 3.80 (3.20, 4.20) | 3.30 (2.70, 3.90) | <0.001 |
BUN (mg/dL) | 19.00 (14.00, 29.00) | 19.00 (14.00, 28.00) | 29.50 (18.75, 39.25) | <0.001 |
Serum sodium (mmol/L) | 139.00 (136.00, 142.00) | 139.00 (136.00, 142.00) | 139.00 (136.00, 142.00) | 0.61 |
SAPS II | 37.00 (30.00, 45.00) | 37.00 (29.00, 45.00) | 48.00 (39.75, 57.50) | <0.001 |
SOFA score | 4.00 (2.00, 7.00) | 4.00 (2.00, 7.00) | 8.00 (5.00, 11.00) | <0.001 |
NLR | 6.54 (3.35, 14.18) | 6.26 (3.22, 13.64) | 11.63 (6.92, 23.07) | <0.001 |
PLR | 205.06 (124.51, 351.35) | 204.96 (124.24, 349.23) | 218.93 (138.36, 397.18) | 0.62 |
SII | 1,591.38 (789.66, 3,503.02) | 1,536.59 (763.30, 3,414.55) | 2,246.37 (1,546.24, 5,238.49) | <0.001 |
PNI | 43.55 (37.55, 48.75) | 43.90 (37.95, 48.95) | 37.80 (30.85, 45.06) | <0.001 |
GLR | 6.02 (3.46, 11.01) | 5.85 (3.44, 10.60) | 9.06 (5.33, 14.72) | <0.001 |
LAR | 0.43 (0.31, 0.61) | 0.42 (0.31, 0.60) | 0.53 (0.37, 0.76) | 0.001 |
Usage of ipratropium bromide | 0.09 | |||
No | 219 (18.11) | 200 (17.59) | 19 (26.39) | |
Yes | 990 (81.89) | 937 (82.41) | 53 (73.61) | |
CKD | 0.008 | |||
No | 755 (62.45) | 699 (61.48) | 56 (77.78) | |
Yes | 454 (37.55) | 438 (38.52) | 16 (22.22) | |
CHF | 0.001 | |||
No | 448 (37.06) | 407 (35.80) | 41 (56.94) | |
Yes | 761 (62.94) | 730 (64.20) | 31 (43.06) | |
Malignancy | 0.56 | |||
No | 1,059 (87.59) | 998 (87.77) | 61 (84.72) | |
Yes | 150 (12.41) | 139 (12.23) | 11 (15.28) |
Data are presented as median (IQR) or n (%). †, Asian, Black, Hispanic or Latino, unknown. BMI, body mass index; HR, heart rate; RR, respiratory rate; pO2, partial pressure of oxygen; pCO2, partial pressure of carbon dioxide; WBC, white blood cell; BUN, blood urea nitrogen; SAPS II, Simplified Acute Physiology Score II; SOFA, Sequential Organ Failure Assessment; NLR, neutrophil-to-lymphocyte ratio; PLR, platelet-to-lymphocyte ratio; SII, the systemic immune-inflammation Index; PNI, prognostic nutritional index; GLR, glucose-to-lymphocyte ratio; LAR, lactate-to-albumin ratio; CKD, chronic kidney disease; CHF, congestive heart failure; IQR, interquartile range.
As detailed in Table 1, median values for NLR [11.63 (6.92, 23.07) vs. 6.26 (3.22, 13.64), P<0.001], GLR [9.06 (5.33, 14.72) vs. 5.85 (3.44, 10.60), P<0.001], LAR [0.53 (0.37, 0.76) vs. 0.42 (0.31, 0.60), P=0.001], and SII [2,246.37 (1,546.24, 5,238.49) vs. 1,536.59 (763.30, 3,414.55), P<0.001] were substantially higher in the death group compared to survivors. Conversely, the median PNI was lower in the death group [37.80 (30.85, 45.06) vs. 43.09 (37.95, 48.95), P<0.001].
Correlation of inflammatory indicators with in-hospital mortality in AECOPD and ARF patients
This analysis evaluated the influence of six inflammatory indicators (NLR, PLR, SII, GLR, LAR, and PNI) on in-hospital mortality among patients with AECOPD and ARF. Multivariable logistic regression models were employed to adjust for confounding variables, with detailed findings presented in Table 2. Unadjusted analysis (Model 1) showed that elevated NLR (OR =1.023; 95% CI: 1.011–1.035; P<0.001), GLR (OR =1.029; 95% CI: 1.010–1.046; P=0.002), and LAR (OR =1.712; 95% CI: 1.214–2.394; P=0.001) significantly predicted increased in-hospital mortality. Conversely, PNI (OR =0.933; 95% CI: 0.905–0.960; P<0.001) was negatively correlated with mortality risk. PLR did not show a significant association.
Table 2
Variables | Model I | Model II | Model III | |||||
---|---|---|---|---|---|---|---|---|
OR (95% CI) | P value | OR (95% CI) | P value | OR (95% CI) | P value | |||
NLR | 1.023 (1.011, 1.035) | <0.001 | 1.024 (1.011, 1.036) | <0.001 | 1.017 (1.002, 1.030) | 0.02 | ||
PLR | 1.000 (0.999, 1.001) | 0.50 | 1.000 (0.999, 1.001) | 0.50 | 1.000 (0.999, 1.001) | 0.40 | ||
SII | 1.000 (1.000, 1.000) | 0.03 | 1.000 (1.000, 1.000) | 0.02 | 1.000 (1.000, 1.000) | 0.20 | ||
PNI | 0.933 (0.905, 0.960) | <0.001 | 0.934 (0.905, 0.963) | <0.001 | 0.961 (0.930, 0.992) | 0.02 | ||
GLR | 1.029 (1.010, 1.046) | 0.002 | 1.028 (1.008, 1.046) | 0.004 | 1.028 (1.005, 1.050) | 0.01 | ||
LAR | 1.712 (1.214, 2.394) | 0.001 | 1.790 (1.245, 2.527) | <0.001 | 0.948 (0.592, 1.450) | 0.80 |
Model I: unadjusted; model II: model I + age, gender, race and BMI; model III: model II + HR, RR, WBC, BUN, CHF, CKD, malignancy, SOFA, SAPS II, and use of ipratropium-bromide. OR, odds ratio; CI, confidence interval; NLR, neutrophil-to-lymphocyte ratio; PLR, platelet-to-lymphocyte ratio; SII, systemic immune-inflammation index; PNI, prognostic nutritional index; GLR, glucose-to-lymphocyte ratio; LAR, lactate-to-albumin ratio; BMI, body mass index; HR, heart rate; RR, respiratory rate; WBC, white blood cell; BUN, blood urea nitrogen; CHF, congestive heart failure; CKD, chronic kidney disease; SOFA, Sequential Organ Failure Assessment; SAPS II, Simplified Acute Physiology Score II.
Similar outcomes were observed in Model 2, which adjusted for age, gender, race, and BMI. Further adjustments in Model 3, which included HR, RR, WBC, BUN, CHF, CKD, malignancy, SOFA score, SAPS II score, and use of ipratropium bromide, confirmed that high levels of NLR (OR =1.017; 95% CI: 1.002–1.030; P=0.02) and GLR (OR =1.028; 95% CI: 1.005–1.050; P=0.01) remained predictive of increased in-hospital mortality, while LAR, PLR, and SII were not significantly related (P>0.05). The negative correlation of PNI with mortality persisted (OR =0.961; 95% CI: 0.930–0.992; P=0.02).
Further exploration using an RCS model indicated a non-linear relationship between NLR and in-hospital mortality, as depicted in Figure 2A (P for non-linearity =0.001), with a significant increase in risk observed when NLR exceeded 6.66. Additionally, a U-shaped curve was noted for PNI, with an inflection point at 43.54. On the left side of the inflection point, the PNI was negatively correlated with the risk of in-hospital mortality (P for non-linearity <0.001; Figure 2B). For GLR, the RCS analysis indicated a linear increase in mortality risk, although this was not statistically significant for non-linearity (P=0.12; Figure 2C).
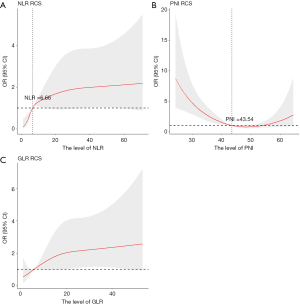
Evaluating the predictive efficacy of inflammatory markers for in-hospital mortality in AECOPD and ARF patients
The predictive capacities of the NLR, GLR, and PNI regarding in-hospital mortality in patients with AECOPD and ARF were assessed using ROC curve analysis. The AUC for NLR was 0.684 (95% CI: 0.627–0.741), denoting a moderate predictive utility. The AUC for PNI was slightly lower at 0.663 (95% CI: 0.557–0.691), with GLR trailing at an AUC of 0.624 (95% CI: 0.557–0.691), suggesting that NLR is marginally more effective than PNI and GLR in assessing the risk of in-hospital mortality. For further details, see Figure 3.
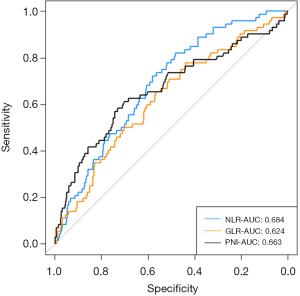
Discussion
This study assessed the prognostic significance of inflammatory markers (NLR, PLR, SII, GLR, LAR, and PNI) for in-hospital mortality among patients with AECOPD and ARF, utilizing data from the MIMIC-IV dataset. The observed in-hospital mortality rate was 5.96%, aligning with the findings of prior studies (3,24). Both NLR and PNI demonstrated non-linear relationships with mortality outcomes in RCS analysis, with inflection points at 6.66 and 43.54, respectively. Elevated GLR were linked with increased mortality risk. These results persisted even after adjusting for covariates. The AUC values for NLR, PNI, and GLR in predicting mortality risk were 0.684, 0.663, and 0.624, respectively, highlighting their potential as easily obtained indicators for identifying patients at elevated risk and guiding timely clinical interventions.
The connection between AECOPD, increased airway, and systemic inflammation is well-documented (9,25). Systemic inflammation in AECOPD may accelerate the decline in lung function and exacerbate comorbid conditions (26). Severe episodes of AECOPD leading to ARF are known to have poor prognoses.
Recent literature has frequently highlighted the correlation between NLR and clinical outcomes in patients with AECOPD. Numerous studies have found that elevated NLR is independently associated with increased mortality in these patients (13,27). These observations support the proposition that the presence of relatively higher NLR values reflects a local (airways) and/or systemic pro-inflammatory state. This study identified that NLR levels were markedly higher in deceased patients compared to survivors. A non-linear relationship between NLR and in-hospital mortality was observed, with a significant increase in risk when NLR exceeded 6.66. Crucially, NLR continued to serve as an independent predictor of mortality, even after adjustments for potential confounders, underscoring its utility as a prognostic biomarker for in-hospital mortality in patients with AECOPD and ARF.
The possible underlying pathological and physiological mechanisms underlying NLR and AECOPD may be closely related to inflammatory activity, immune dysfunction and systemic inflammatory state. The NLR evaluation offers insights into two separate immune pathways, reflecting the body’s overall inflammatory condition. Neutrophil is the key inflammatory cells in the pathogenesis of AECOPD, participating in pathogen clearance and releasing inflammatory mediators (28). Second, the immune system of AECOPD patients is in a state of dysregulation, with possible abnormalities in both the number and functionality of lymphocytes (9), contributing to elevated NLR values. Third, AECOPD is not only limited to the lung, but also associated with systemic inflammation. This is typically characterized by elevated levels of cytokines, chemokines, and inflammatory proteins or abnormal cell counts (9). The elevated NLR represents the imbalance between inflammation, immunity, and tissue destruction and repair, as well as persistent local and systemic inflammation, including increased risks for ARF and other complications (13).
Platelets play a crucial role as mediators of inflammation and thrombosis. Research indicates that thrombocytopenia correlates with poor outcomes in AECOPD and other respiratory diseases (29-31). Our findings showed that non-survivors exhibited significantly lower platelet counts compared to survivors. Regarding the PLR, our analysis revealed no significant differences in PLR values between survivors and deceased AECOPD and ARF patients, aligning with previous research that found no substantial link between PLR and mortality (27,32). Similarly, increases in the SII, derived from lymphocyte, neutrophil, and platelet counts, were associated with elevated all-cause mortality risk in COPD patients (33). Although significant variations in SII were noted between the survival and death groups in our study, multivariate analysis did not establish independent associations. This result could be attributed to simultaneous reductions in both components of the SII—platelet and lymphocyte counts—pointing to their collective impact on increasing in-hospital mortality risk.
Our study found that a higher PNI was independently correlated with decreased in-hospital mortality, even after adjusting for potential confounders. The risk of in-hospital mortality decreased significantly with PNI level up to the inflection point (PNI =43.54). Another retrospective cohort study consistent with ours, reported that the incidence of adverse hospitalization outcomes in AECOPD patients significantly decreased with PNI level up to the inflection point (PNI =42) (17). In Zhou et al.’s study, When PNI was ≤38.58, AECOPD patients had a higher risk of hypercapnic respiratory failure (34).
PNI is an indicator that reflects inflammation, immune response, and nutritional health status (35). First, PNI was an objective indicator for evaluating nutrition. Studies have found that COPD patients often have higher energy demands and are at an increased risk of malnutrition (36,37). Second, PNI calculation relies on circulating lymphocytes. It is worth noting that malnutrition weakens immune defenses, and low circulating lymphocyte counts are associated with poorer outcomes in infection-related disorders such as COPD (38), coronavirus disease 2019 (COVID-19) patients (39) and sepsis (40). In recent years, studies have also shown that PNI has significance in predicting the prognosis of COPD (17,34,41). Malnutrition reduced muscle mass, weakened the strength of respiratory muscles, and impaired immune defense (42). These effects can increase the risk of infection and exacerbate airflow limitation, thereby raising the likelihood of respiratory failure in AECOPD patients (17,41). Consequently, nutritional assessments and interventions should be integral to the treatment regimen for AECOPD patients with ARF upon hospital admission.
Additionally, this study found that the GLR is positively associated with in-hospital mortality, independently of other factors. This aligns with findings from earlier studies showing that higher GLR levels predict worse outcomes for AECOPD patients, particularly those admitted to ICU (43,44). While the exact mechanisms linking higher blood glucose levels with respiratory impairment remain under investigation, systemic inflammation has been proposed as a potential mediator (45). Thus, GLR might effectively reflect the combined impact of hyperglycemia and compromised immunity in these patients.
In initial analyses (Model 1 and Model 2), an increase in LAR was significantly associated with an elevated risk of in-hospital mortality among patients with AECOPD and ARF. However, this relationship became non-significant in the fully adjusted Model 3, indicating that other confounding factors may influence the association between LAR and mortality. These findings suggest a complex interaction between LAR and in-hospital mortality, where LAR alone does not solely drive mortality risk; instead, various biological and environmental factors may also contribute.
This research is pioneering in its focus on the prognostic values of NLR, LAR, GLR, PNI, PLR, and SII on admission to hospital for predicting in-hospital mortality in patients with AECOPD and ARF. By adjusting for multiple confounding factors, the study enhances the reliability and applicability of its findings. The results may aid in identifying reliable biomarkers for determining which patients are at high risk of in-hospital mortality, potentially guiding more targeted clinical interventions.
Despite its contributions, this study acknowledges several limitations. Firstly, being a retrospective analysis from a single center, it is subject to inherent selection bias. Secondly, while the use of ICD-9 and ICD-10 codes was instrumental in identifying our study cohort within the MIMIC-IV database, we acknowledge several limitations associated with this approach. The reliance on ICD coding for case identification may not fully capture the clinical nuances and variations in disease presentation that a physician’s assessment would provide. Additionally, coding practices can vary across institutions and over time, which may introduce variability in case identification. Thirdly, we recognize that our study had a lower number of positive events (in-hospital deaths), which could potentially affect the power of our study to detect smaller effect sizes or rare outcomes. Due to database constraints, critical variables such as smoking status, annual frequency of exacerbations, pulmonary function, and the use of corticosteroids or antibiotics were not available, potentially impacting the study’s outcomes. Fourthly, despite adjustments for known confounders, the possibility of residual and unmeasured confounding factors cannot be eliminated completely. Lastly, serum biomarkers were the first measurements when they were admitted into the hospital and their temporal stability is still unclear. Future studies should be prospective, ideally with extended follow-ups and more detailed clinical data, to identify the best biomarker, or combination of biomarkers with or without other clinical characteristics, for prognosis in patients with AECOPD and ARF.
Conclusions
NLR, GLR, and PNI on admission to hospital have moderate predictive utility for in-hospital mortality in patients with AECOPD and ARF. Both NLR and PNI demonstrate non-linear relationships with mortality outcomes, with inflection points at 6.66 and 43.54, respectively. Elevated NLR with the value over 6.66, significantly increase the odds of the in-hospital mortality. When the PNI was less than 43.54, the risk of in-hospital mortality was negatively correlated with the PNI levels.
Acknowledgments
Funding: None.
Footnote
Reporting Checklist: The authors have completed the STROBE reporting checklist. Available at https://jtd.amegroups.com/article/view/10.21037/jtd-24-1287/rc
Peer Review File: Available at https://jtd.amegroups.com/article/view/10.21037/jtd-24-1287/prf
Conflicts of Interest: All authors have completed the ICMJE uniform disclosure form (available at https://jtd.amegroups.com/article/view/10.21037/jtd-24-1287/coif). The authors have no conflicts of interest to declare.
Ethical Statement: The authors are accountable for all aspects of the work in ensuring that questions related to the accuracy or integrity of any part of the work are appropriately investigated and resolved. The study was conducted in accordance with the Declaration of Helsinki (as revised in 2013).
Open Access Statement: This is an Open Access article distributed in accordance with the Creative Commons Attribution-NonCommercial-NoDerivs 4.0 International License (CC BY-NC-ND 4.0), which permits the non-commercial replication and distribution of the article with the strict proviso that no changes or edits are made and the original work is properly cited (including links to both the formal publication through the relevant DOI and the license). See: https://creativecommons.org/licenses/by-nc-nd/4.0/.
References
- Safiri S, Carson-Chahhoud K, Noori M, et al. Burden of chronic obstructive pulmonary disease and its attributable risk factors in 204 countries and territories, 1990-2019: results from the Global Burden of Disease Study 2019. BMJ 2022;378:e069679. [Crossref] [PubMed]
- Global Initiative for Chronic Obstructive Lung Disease. 2024 GOLD Report. Global Strategy for Prevention, Diagnosis and Management of COPD: 2024 Report. Available online: https://goldcopd.org/2024-gold-report/
- Zhou R, Pan D. Association between admission heart rate and in-hospital mortality in patients with acute exacerbation of chronic obstructive pulmonary disease and respiratory failure: a retrospective cohort study. BMC Pulm Med 2024;24:111. [Crossref] [PubMed]
- Prediletto I, Giancotti G, Nava S. COPD Exacerbation: Why It Is Important to Avoid ICU Admission. J Clin Med 2023;12:3369. [Crossref] [PubMed]
- Steriade AT, Johari S, Sargarovschi N, et al. Predictors of outcome of noninvasive ventilation in severe COPD exacerbation. BMC Pulm Med 2019;19:131. [Crossref] [PubMed]
- Breen D, Churches T, Hawker F, et al. Acute respiratory failure secondary to chronic obstructive pulmonary disease treated in the intensive care unit: a long term follow up study. Thorax 2002;57:29-33. [Crossref] [PubMed]
- Qian Y, Cai C, Sun M, et al. Analyses of Factors Associated with Acute Exacerbations of Chronic Obstructive Pulmonary Disease: A Review. Int J Chron Obstruct Pulmon Dis 2023;18:2707-23. [Crossref] [PubMed]
- Brightling C, Greening N. Airway inflammation in COPD: progress to precision medicine. Eur Respir J 2019;54:1900651. [Crossref] [PubMed]
- Xu J, Zeng Q, Li S, et al. Inflammation mechanism and research progress of COPD. Front Immunol 2024;15:1404615. [Crossref] [PubMed]
- Hu Y, Long H, Cao Y, et al. Prognostic value of lymphocyte count for in-hospital mortality in patients with severe AECOPD. BMC Pulm Med 2022;22:376. [Crossref] [PubMed]
- Zhang J, Chen F, Wang Y, et al. Early detection and prediction of acute exacerbation of chronic obstructive pulmonary disease. Chin Med J Pulm Crit Care Med 2023;1:102-7. [Crossref] [PubMed]
- Zinellu A, Zinellu E, Pau MC, et al. A Comprehensive Systematic Review and Meta-Analysis of the Association between the Neutrophil-to-Lymphocyte Ratio and Adverse Outcomes in Patients with Acute Exacerbation of Chronic Obstructive Pulmonary Disease. J Clin Med 2022;11:3365. [Crossref] [PubMed]
- Liao QQ, Mo YJ, Zhu KW, et al. Platelet-to-Lymphocyte Ratio (PLR), Neutrophil-to-Lymphocyte Ratio (NLR), Monocyte-to-Lymphocyte Ratio (MLR), and Eosinophil-to-Lymphocyte Ratio (ELR) as Biomarkers in Patients with Acute Exacerbation of Chronic Obstructive Pulmonary Disease (AECOPD). Int J Chron Obstruct Pulmon Dis 2024;19:501-18. [Crossref] [PubMed]
- Song YD, Bai XM, Ma J. The association of systemic immune-inflammation index with lung function, risk of COPD and COPD severity: A population-based study. PLoS One 2024;19:e0303286. [Crossref] [PubMed]
- Hu T, Liu X, Liu Y. Usefulness of Glucose to Lymphocyte Ratio to Predict in-Hospital Mortality in Patients with AECOPD Admitted to the Intensive Care Unit. COPD 2022;19:158-65. [Crossref] [PubMed]
- Xie J, Liu H, He Q, et al. Relationship between lactate-to-albumin ratio and 28-day mortality in patients with exacerbation of chronic obstructive pulmonary disease admitted to the Intensive Care Unit. Eur J Med Res 2024;29:258. [Crossref] [PubMed]
- Yuan FZ, Xing YL, Xie LJ, et al. The Relationship Between Prognostic Nutritional Indexes and the Clinical Outcomes of Patients with Acute Exacerbation of Chronic Obstructive Pulmonary Disease. Int J Chron Obstruct Pulmon Dis 2023;18:1155-67. [Crossref] [PubMed]
- Suzuki E, Kawata N, Shimada A, et al. Prognostic Nutritional Index (PNI) as a Potential Prognostic Tool for Exacerbation of COPD in Elderly Patients. Int J Chron Obstruct Pulmon Dis 2023;18:1077-90. [Crossref] [PubMed]
- Johnson A, Bulgarelli L, Pollard T, et al. MIMIC-IV. PhysioNet. 2023. Available online: https://physionet.org/content/mimiciv/2.2/
- Slee VN. The International Classification of Diseases: ninth revision (ICD-9). Ann Intern Med 1978;88:424-6. [Crossref] [PubMed]
- World Health Organization. ICD-10. International Statistical Classification of Diseases and Related Health Problems: Tenth Revision 1992, Volume 1. Wkly Epidemiol Rec 1992;67:203-4.
- Ferreira FL, Bota DP, Bross A, et al. Serial evaluation of the SOFA score to predict outcome in critically ill patients. JAMA 2001;286:1754-8. [Crossref] [PubMed]
- Le Gall JR, Lemeshow S, Saulnier F. A new Simplified Acute Physiology Score (SAPS II) based on a European/North American multicenter study. JAMA 1993;270:2957-63. [Crossref] [PubMed]
- Steer J, Gibson J, Bourke SC. The DECAF Score: predicting hospital mortality in exacerbations of chronic obstructive pulmonary disease. Thorax 2012;67:970-6. [Crossref] [PubMed]
- Ritchie AI, Wedzicha JA. Definition, Causes, Pathogenesis, and Consequences of Chronic Obstructive Pulmonary Disease Exacerbations. Clin Chest Med 2020;41:421-38. [Crossref] [PubMed]
- Barnes PJ. Inflammatory mechanisms in patients with chronic obstructive pulmonary disease. J Allergy Clin Immunol 2016;138:16-27. [Crossref] [PubMed]
- Emami Ardestani M, Alavi-Naeini N. Evaluation of the relationship of neutrophil-to lymphocyte ratio and platelet-to-lymphocyte ratio with in-hospital mortality in patients with acute exacerbation of chronic obstructive pulmonary disease. Clin Respir J 2021;15:382-8. [Crossref] [PubMed]
- Vaitkus M, Lavinskiene S, Barkauskiene D, et al. Reactive oxygen species in peripheral blood and sputum neutrophils during bacterial and nonbacterial acute exacerbation of chronic obstructive pulmonary disease. Inflammation 2013;36:1485-93. [Crossref] [PubMed]
- Rahimi-Rad MH, Soltani S, Rabieepour M, et al. Thrombocytopenia as a marker of outcome in patients with acute exacerbation of chronic obstructive pulmonary disease. Pneumonol Alergol Pol 2015;83:348-51. [Crossref] [PubMed]
- Lopez-Delgado JC, Rovira A, Esteve F, et al. Thrombocytopenia as a mortality risk factor in acute respiratory failure in H1N1 influenza. Swiss Med Wkly 2013;143:w13788. [Crossref] [PubMed]
- Fawzy A, Anderson JA, Cowans NJ, et al. Association of platelet count with all-cause mortality and risk of cardiovascular and respiratory morbidity in stable COPD. Respir Res 2019;20:86. [Crossref] [PubMed]
- Yao C, Wang L, Shi F, et al. Optimized combination of circulating biomarkers as predictors of prognosis in AECOPD patients complicated with Heart Failure. Int J Med Sci 2021;18:1592-9. [Crossref] [PubMed]
- Ye C, Yuan L, Wu K, et al. Association between systemic immune-inflammation index and chronic obstructive pulmonary disease: a population-based study. BMC Pulm Med 2023;23:295. [Crossref] [PubMed]
- Zhou Z, Wang Y, Wang Y, et al. A Diagnostic Nomogram for Predicting Hypercapnic Respiratory Failure in Patients with Acute Exacerbation of Chronic Obstructive Pulmonary Disease. Int J Chron Obstruct Pulmon Dis 2024;19:1079-91. [Crossref] [PubMed]
- Hu Y, Cao Q, Wang H, et al. Prognostic nutritional index predicts acute kidney injury and mortality of patients in the coronary care unit. Exp Ther Med 2021;21:123. [Crossref] [PubMed]
- Yogesh M, Patel J, Makwana N, et al. The triad of physiological challenges: investigating the intersection of sarcopenia, malnutrition, and malnutrition-sarcopenia syndrome in patients with COPD - a cross-sectional study. BMC Pulm Med 2024;24:71. [Crossref] [PubMed]
- Liu H, Song J, Wang Z, et al. Investigation of nutrition status and analysis of 180-day readmission factors in elderly hospitalized patients with COPD. Aging Clin Exp Res 2024;36:155. [Crossref] [PubMed]
- Semenzato U, Biondini D, Bazzan E, et al. Low-Blood Lymphocyte Number and Lymphocyte Decline as Key Factors in COPD Outcomes: A Longitudinal Cohort Study. Respiration 2021;100:618-30. [Crossref] [PubMed]
- Chen G, Zhao X, Chen X, et al. Early decrease in blood lymphocyte count is associated with poor prognosis in COVID-19 patients: a retrospective cohort study. BMC Pulm Med 2023;23:453. [Crossref] [PubMed]
- Wang Z, Zhang W, Chen L, et al. Lymphopenia in sepsis: a narrative review. Crit Care 2024;28:315. [Crossref] [PubMed]
- Xu Y, Yan Z, Li K, et al. Association between nutrition-related indicators with the risk of chronic obstructive pulmonary disease and all-cause mortality in the elderly population: evidence from NHANES. Front Nutr 2024;11:1380791. [Crossref] [PubMed]
- Zhao X, Su R, Hu R, et al. Sarcopenia index as a predictor of clinical outcomes among older adult patients with acute exacerbation of chronic obstructive pulmonary disease: a cross-sectional study. BMC Geriatr 2023;23:89. [Crossref] [PubMed]
- Kim V, Kretschman DM, Sternberg AL, et al. Weight gain after lung reduction surgery is related to improved lung function and ventilatory efficiency. Am J Respir Crit Care Med 2012;186:1109-16. [Crossref] [PubMed]
- Lin L, Shi J, Kang J, et al. Analysis of prevalence and prognosis of type 2 diabetes mellitus in patients with acute exacerbation of COPD. BMC Pulm Med 2021;21:7. [Crossref] [PubMed]
- Lin CS, Liu CC, Yeh CC, et al. Diabetes risks and outcomes in chronic obstructive pulmonary disease patients: Two nationwide population-based retrospective cohort studies. PLoS One 2017;12:e0181815. [Crossref] [PubMed]