Combining cardiac and renal biomarkers to establish a clinical early prediction model for cardiac surgery-associated acute kidney injury: a prospective observational study
Highlight box
Key findings
• Combining renal biomarkers (serum cystatin C and urinary N-acetyl-β-D-glucosaminidase) with cardiac biomarkers (N-terminal pro B-type natriuretic peptide and cardiac troponin I) could significantly improve the accuracy of predicting cardiac surgery-associated acute kidney injury (CSA-AKI). The prediction model showed high predictive performance with the area under the curve of the receiver operating characteristic of 0.830 in the modeling cohort and 0.840 in the validation cohort.
What is known and what is new?
• It is known that CSA-AKI is a prevalent complication with poor outcomes. Existing biomarkers individually assess kidney and cardiac function but have limited predictive accuracy. The study presents a novel prediction model that incorporates both renal and cardiac biomarkers, resulting in enhanced predictive capability for CSA-AKI.
What is the implication, and what should change now?
• This study suggests that biomarkers from multiple sources might help in predicting complex clinical complications.
Introduction
Acute kidney injury (AKI) is a common complication following cardiac surgery (1). With approximately 2 million cardiac surgeries performed worldwide annually, the prevalence of cardiac surgery-associated acute kidney injury (CSA-AKI) ranges from 5% to 42% (2). CSA-AKI is closely linked to higher in-hospital mortality rates, prolonged hospital stays, and increased healthcare expenses (3). However, due to the intricate pathophysiology and underlying mechanisms of CSA-AKI, progress in treatment and early prediction have been slow. As a result, accurately predicting the occurrence of CSA-AKI remains a considerable challenge.
Several classical clinical prediction models have been developed to enhance clinicians’ ability to predict CSA-AKI, such as the Cleveland Clinical Score in 2005 (4), the Mehta Score in 2006 (5), and the Simplified Renal Index Score (6). However, these models have not gained widespread use in clinical practice due to variations in AKI criteria, different timing of renal replacement therapy (RRT), and challenges in clinical application. Recognizing this limitation, the Acute Dialysis Quality Initiative Working Group has proposed the integration of biomarkers from diverse sources to enhance predictive accuracy for AKI (7). However, the optimal combination of biomarkers is yet to be determined.
Serum cystatin C (sCysC) is a biomarker that better reflects the estimated glomerular filtration rate (eGFR) (8). It can be completely filtered by the glomerulus, reabsorbed and catabolized in the proximal tubule. However, this process is independent of factors such as gender, body weight, age, muscle mass, and inflammatory response. In our previous study, sCysC was the best predictor of both AKI and severe AKI compared to 16 other biomarkers (9). Another renal biomarker, urinary N-acetyl-β-d-glucosaminidase (uNAG), is derived from proximal, distal tubular cells, and non-renal cells. After tubular injury, uNAG is released into the urine, making it a biomarker that reflects tubular injury (10). Both renal biomarkers have the advantage of being available and stable in detection compared to other renal biomarkers. Previous studies have shown that the early prediction model consisting of sCysC and uNAG has good predictive efficacy for AKI in post-craniotomy, post-total aortic replacement, and septic patients (11-13). Common cardiac biomarkers used to assess cardiac function and myocardial injury include amino-terminal brain natriuretic peptide precursor (NT-proBNP), cardiac troponin I (cTnI), creatine kinase (CK), and creatine kinase-MB (CKMB). Among them, NT-proBNP is a peptide secreted by the ventricle in response to overstretching of cardiomyocytes (14), and cTnI, CK, and CKMB are specific biomarkers reflecting myocardial injury (15). Haines et al. revealed that elevated cTnI and NT-proBNP were independent risk factors for the development of AKI (16). Bucholz et al. pointed out that preoperative elevated CK-MB was associated with an increased risk of CSA-AKI, and its level contributed to the risk stratification of AKI in this group of patients (17).
However, there is currently insufficient evidence regarding the predictive performance of these biomarkers and their combinations specifically in CSA-AKI. Consequently, we conducted a prospective, observational study in the cardiac surgical intensive care unit to assess the correlation between these biomarkers and CSA-AKI, as well as evaluating the predictive effectiveness of an early prediction model for CSA-AKI based on these biomarkers. We present this article in accordance with the TRIPOD reporting checklist (available at https://jtd.amegroups.com/article/view/10.21037/jtd-24-1185/rc).
Methods
Study design and participants
This study was a prospective, observational, single-center, two-stage study. At the first stage, a modeling cohort of adult patients (age ≥18 years) who underwent cardiac surgery at our center from October 2020 to April 2022 were consecutively enrolled. At the second phase, a validation cohort of adult patients who underwent cardiac surgery at our center from May 2022 to December 2022 were consecutively enrolled. Exclusion criteria were (I) patients with a previous history of renal transplantation or nephrectomy; (II) patients with a diagnosis of end-stage renal disease or those who had required RRT prior to surgery; (III) pregnancy status; (IV) patients with a preoperative diagnosis of AKI; and (V) patients who did not consent to enrollment, had missing data on admission, or had incomplete information. This study complied with the Enhanced Reporting of Observational Studies in Epidemiology (18) and the Diagnostic Accuracy Reporting Criteria (19). This was approved by the Ethics Committee of Guangdong Provincial People’s Hospital [approval No. GDREC2015396H(R1)]. Informed consent was waived due to the use of de-identified and anonymized data, ensuring confidentiality and privacy for statistical research purposes only. The study was conducted in accordance with the Declaration of Helsinki (as revised in 2013).
Sample and data collection
Clinical data for each patient were prospectively obtained from electronic medical records and laboratory databases (Table 1). Venous blood and urine specimens were collected immediately after surgery to measure renal biomarkers sCysC and uNAG and cardiac biomarkers NT-proBNP, cTnI, CK, and CKMB. The staff measuring the biomarkers were unaware of the clinical characteristics and the study outcome of each patient. The following variables were included as candidate predictors.
Table 1
Category | Variables |
---|---|
Baseline data | Age, gender, weight, hypertension, diabetes mellitus, CAD, CKD, hyperlipidemia, preoperative use of nephrotoxic medications [defined as having used the following medications within 5 days prior to surgery (20): non-steroidal antipyretic analgesics, immunosuppressants, ACEIs, ARBs] mannitol, angiographic agents, and nephrotoxic antibiotics such as acyclovir, vancomycin, aminoglycosides, amphotericin B |
Preoperative date | Echocardiographic data included LVEF, sCr, hemoglobin, D-dimer, and serum albumin |
Postoperative data | Renal biomarkers (sCysC and uNAG), cardiac biomarkers (CK, CKMB, cTnI, NT-proBNP), APACHE II scores (15), hourly urine output and daily sCr during ICU stay |
Surgical data | Emergency surgery, heart valve surgery, CABG, CABG combined with heart valve surgery, other types of cardiac surgery (corrective surgery for congenital heart disease, aortic sinus aneurysm repair, etc.), transfusion, blood transfusion, duration of CPB, duration of ACC, and minimum intraoperative temperature |
Outcome | The onset and stage of AKI, duration of mechanical ventilation, ICU LOS, CRRT, IABP, and ECMO, total hospital costs, and in-hospital mortality |
CAD, coronary artery disease; CKD, chronic kidney disease; ACEIs, angiotensin-converting enzyme inhibitors; ARBs, angiotensin II receptor blockers; LVEF, left ventricular ejection fraction; sCr, serum creatinine; sCysC, serum cystatin C; uNAG, urine N-acetyl-β-D-glucosidase; CK, creatine kinase; CKMB, creatine kinase isoenzyme; cTnI, cardiac troponin I; NT-proBNP, N-terminal B-type natriuretic peptide precursor; APACHE II, acute physiologic and chronic health evaluation II; ICU, intensive care unit; CABG, coronary artery bypass grafting; CPB, cardiopulmonary bypass; ACC, aortic cross clamping; AKI, acute kidney injury; LOS, length of stay; CRRT, continuous renal replacement therapy; IABP, intra-aortic balloon pump; ECMO, extracorporeal membrane pulmonary oxygenation.
Diagnosis and definitions
According to the latest diagnostic criteria, CSA-AKI is defined as having undergone cardiac surgery in the past week and meeting the AKI criteria of the Kidney Disease Improving Global Outcomes (KDIGO) (21). AKI is defined as having any of the following criteria: an increase in sCr by ≥0.3 mg/dL (≥26.5 µmol/L) within 48 hours; or an increase in sCr by ≥1.5 times baseline, which is known or presumed to have occurred within the prior 7 days; or a urine volume <0.5 mL/kg/h for 6 hours. AKI is staged for severity according to the KDIGO criteria (Table 2).
Table 2
Stage | sCr | Urine output |
---|---|---|
1 | 1.5–1.9 times baseline or | <0.5 mL/kg/h for 6–12 hours |
≥0.3 mg/dL (≥26.5 μmol/L) increase | ||
2 | 2.0–2.9 times baseline | <0.5 mL/kg/h for ≥12 hours |
3 | 3.0 times baseline or | <0.5 mL/kg/h for ≥24 hours or |
Increase in sCr to ≥4.0 mg/dL (≥353.6 μmol/L) or | Anuria for ≥12 hours | |
Initiation of RRT or | ||
In patients <18 years, decrease in eGFR to <35 mL/min per 1.73 m2 |
AKI, acute kidney injury; sCr, serum creatinine; RRT, renal replacement therapy; eGFR, estimated glomerular filtration rate.
According to a previous study (22), baseline sCr values (including modeling cohort n1 and validation cohort n2) were determined according to the following principles:
- Latest sCr values measured between 1 month and 1 year prior to admission (n1=181, n2=79).
- Stable sCr values measured more than 1 year prior to admission when patients were younger than 40 years (a stable sCr value is defined as fluctuations within 15% of the minimum sCr value on admission) (n1=65, n2=28).
- sCr values that measured more than 1 year before admission and with values lower than the sCr value at this admission (n1=48, n2=19).
- If a patient was not diagnosed with AKI on admission, sCr values between 3 and 39 days prior to admission and lower than the sCr value on admission could be selected (n1=56, n2=12).
- The lowest sCr value was selected if one of the following conditions existed: the first sCr value on admission (n1=302, n2=155), the last sCr value in the cardiac surgical intensive care unit (n1=30, n2=18), and the lowest sCr value after discharge for follow-up at 1 year (n1=7, n2=2).
Statistical methods
Grouping and data processing
Patients were divided into AKI group or non-AKI group according to whether postoperative AKI occurred. Differences in baseline characteristics, perioperative data, and outcomes were compared between the two groups. Measurement data were presented as mean ± standard deviation if normally distributed, and independent samples t-test was used for comparison between the two groups. Measurement data that did not meet normal distribution were presented as median and interquartile range (P25, P75), and the Wilcoxon test was used for comparison between the two groups. Count data were presented as frequencies and rates (percentages), and comparisons between groups were made using the Pearson chi-square test or Fisher’s exact probability method.
Predictor screening and clinical early prediction modeling
The least absolute shrinkage and selection operator (LASSO) method was used to screen predictors and construct the model. LASSO regression, also known as penalized regression, is a method that imposes penalties on variables with high variance to eliminate the number of variables and is suitable for reducing high-dimensional data and improving the predictive accuracy and interpretability of the model (23). We used LASSO binary regression to include demographic and clinical characteristic variables and screened for statistically significant predictors to establish a CSA-AKI clinical prediction model.
Performance evaluation of the prediction model
In this study, the following methods were used to assess the performance of the prediction model:
- Receiver operating characteristic (ROC) curves for the prediction model were created and used to assess the accuracy of the model.
- Calibration curves can show the relationship between the prediction results of the model and the cohort observations, where the perfectly calibrated model follows a 45-degree line. In this study, we presented the model fit with calibration curves and used Bootstrap for internal validation.
Decision curve analysis (DCA) is a method for evaluating clinical prediction models, diagnostic assays, and biomarkers (24). DCA can integrate patient or decision maker preferences into the analysis to represent the net clinical benefit of model-based predictive decision making, thereby compensating for the shortcomings of evaluation methods such as the area under the curve of the receiver operating characteristic (ROC-AUC). Therefore, we used DCA to evaluate the clinical efficacy of the prediction model.
Plotting and validation of the risk nomogram
A CSA-AKI risk nomogram was drawn according to the clinical early prediction model established by LASSO regression. In a nomogram, each risk factor has its own score axis, and the corresponding points can be obtained at the top of the nomogram along the vertical line. The scores for all variables were summed to give a total score based on each patient’s specific risk factors. With the total score, the probability of CSA-AKI can be obtained on the prediction line at the bottom of the nomogram. Then, Nomo-ROC and Nomo-DCA were used to further analyze the rationality and diagnostic performance of the nomogram. Finally, a validation cohort was established to validate the nomogram externally.
R statistical software (version 3.4.1; http://www.R-project.org) was used in this study to complete all statistical analyses and graphic production, and a two-tailed P value of <0.05 was considered statistically significant.
Results
Preoperative characteristics and outcomes of patients in the modeling cohort
A total of 746 adult patients underwent cardiac surgery at our center from October 2020 to April 2022. After excluding patients with end-stage renal disease, pregnancy status, renal transplantation, preoperative AKI status, and missing data, 689 patients were enrolled in the modeling cohort (Figure 1). Patients were divided into AKI group and non-AKI group according to the KDIGO criteria. Table 3 showed the baseline characteristics, general information, and outcomes of the modeling cohort.
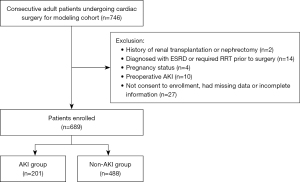
Table 3
Variables | Total (n=689) | AKI (n=201) | Non-AKI (n=488) | P values |
---|---|---|---|---|
Male | 417 (60.5) | 109 (54.2) | 308 (63.1) | 0.03* |
Age (years) | 57.0 (48.0, 64.0) | 62.0 (54.0, 68.0) | 55.0 (46.0, 62.0) | <0.001* |
Weight (kg) | 60.0 (53.0, 67.0) | 58.0 (52.0, 67.0) | 60.0 (53.0, 67.0) | 0.28 |
Medical history | ||||
Hypertension | 158 (22.9) | 71 (35.3) | 87 (17.8) | <0.001* |
Diabetes mellitus | 62 (9.0) | 27 (13.4) | 35 (7.2) | 0.01* |
Hyperlipidemia | 5 (0.7) | 1 (0.5) | 4 (0.8) | >0.99 |
CAD | 12 (1.7) | 6 (3.0) | 6 (1.2) | 0.12 |
CKD | 5 (0.7) | 3 (1.5) | 2 (0.4) | 0.15 |
Nephrotoxic drugs | 33 (4.8) | 10 (5.0) | 23 (4.7) | >0.99 |
Pre-op LVEF (%) | 63.0 (57.0, 67.0) | 63.0 (55.0, 67.0) | 63.0 (57.0, 67.0) | 0.56 |
Pre-op examination | ||||
Hemoglobin (g/L) | 134.0 (120.0, 145.0) | 129.0 (115.0, 139.0) | 136.0 (123.0, 146.0) | <0.001* |
D-dimer (ng/mL) | 360.0 (240.0, 830.0) | 500.0 (290.0, 1,110.0) | 330.0 (220.0, 722.5) | <0.001* |
Albumin (g/L) | 38.5 (36.3,40.9) | 37.7 (35.1, 40.1) | 38.8 (36.6, 41.3) | <0.001* |
Baseline sCr (µmol/L) | 78.0 (65.3, 93.6) | 77.6 (63.2, 97.3) | 78.5 (66.8, 92.7) | 0.65 |
Outcomes | ||||
Hospital costs (10k CNY) | 14.8 (11.8, 19.4) | 19.7 (15.7, 26.3) | 13.5 (10.8, 16.9) | <0.001* |
In-hospital mortality | 20 (2.9) | 15 (7.5) | 5 (1.0) | <0.001* |
IABP | 43 (6.2) | 27 (13.4) | 16 (3.3) | <0.001* |
CRRT | 19 (2.8) | 15 (7.5) | 4 (0.8) | <0.001* |
ECMO | 11 (1.6) | 6 (3.0) | 5 (1.0) | 0.09 |
MV (h) | 20.0 (13.0, 43.0) | 43.0 (19.0, 100.0) | 17.0 (10.8, 24.0) | <0.001* |
ICU LOS (h) | 45.0 (27.0, 112.0) | 110.0 (46.0, 208.0) | 42.0 (22.0, 68.2) | <0.001* |
AKI staging | ||||
Stage 1 | 132 (65.7) | |||
Stage 2 | 48 (23.9) | |||
Stage 3 | 21 (10.4) |
Data were presented as median (interquartile range) or n (%). *, there is a statistical difference. AKI, acute kidney injury; CAD, coronary artery disease; CKD, chronic kidney disease; Nephrotoxic drugs, including immunosuppressants, angiotensin-converting enzyme inhibitors, angiotensin receptor blockers, non-steroidal anti-inflammatory drugs, antibiotics (including vancomycin, acyclovir, amphotericin B, aminoglycosides), mannitol, angiography agents, etc.; Pre-op, preoperative; LVEF, left ventricular ejection fraction; sCr, serum creatinine; CNY, Chinese yuan; IABP, intra-aortic balloon pump; CRRT, continuous renal replacement therapy; ECMO, extracorporeal membrane oxygenation; MV, mechanical ventilation; ICU LOS, intensive care unit length of stay.
Overall, there were 201 (29.2%) cases of CSA-AKI in the cohort, with 132 (65.7%) were in stage 1, 48 (23.9%) in stage 2, and 21 (10.4%) in stage 3. Compared to the non-AKI group, patients in the AKI group were older [62.0 (54.0, 68.0) vs. 55.0 (46.0, 62.0) years old, P<0.001] and had a higher proportion of hypertension (35.3% vs. 17.8%, P<0.001) and diabetes mellitus (13.4% vs. 7.2%, P=0.01). Regarding laboratory results, patients in the AKI group had higher preoperative D-dimer (DDI) levels [500.0 (290.0, 1,110.0) vs. 330.0 (220.0, 722.5) ng/mL, P<0.001], but had lower serum albumin concentration [37.7 (35.1, 40.1) vs. 38.8 (36.6, 41.3) g/L, P<0.001] and hemoglobin [129.0 (115.0, 139.0) vs. 136.0 (123.0, 146.0) g/L, P<0.001] than those in the non-AKI group. However, there was no statistical difference in the preoperative use of nephrotoxic drugs, cardiac function, and baseline sCr between the two groups. Notably, patients in the AKI group had a higher proportion of postoperative adverse outcomes, which were associated with higher in-hospital mortality, higher healthcare costs, higher rates of IABP and CRRT, and longer duration of mechanical ventilation and ICU stay.
Intraoperative and postoperative characteristics of patients in the modeling cohort
The surgical procedures, intraoperative, and postoperative characteristics of the modeling cohort are shown in Table 4. Compared to the non-AKI group, the AKI group had a higher proportion of emergency surgery (5.5% vs. 1.8%, P=0.02) and CABG combined with valve replacement (10.4% vs. 4.7%, P=0.009). Patients in the AKI group had a longer CPB time [175.0 (130.0, 214.0) vs. 135.0 (106.8, 173.0) min. P<0.001] and ACC time [103.0 (73.0, 138.0) vs. 84.0 (62.0, 110.0) min, P<0.001], and had lower minimum intraoperative temperatures [30.9 (29.9, 31.7) vs. 31.1 (30.3, 32.0) ℃, P=0.005]. There were no significant differences in other surgical procedures (valve replacement, CABG, and other procedures), intraoperative transfusions of red blood cells and hydroxyethyl starch between the two groups.
Table 4
Variables | Total (n=689) | AKI (n=201) | Non-AKI (n=488) | P value |
---|---|---|---|---|
Surgical procedures | ||||
Valve surgery | 545 (79.1) | 145 (72.1) | 400 (82.0) | 0.07 |
CABG | 51 (7.4) | 21 (10.4) | 30 (6.1) | 0.06 |
CABG + valve surgery | 44 (6.4) | 21 (10.4) | 23 (4.7) | 0.009* |
Other procedures | 49 (7.1) | 14 (7.0) | 35 (7.2) | >0.99 |
Intra-op data | ||||
CPB duration (min) | 145.0 (113.0, 186.0) | 175.0 (130.0, 214.0) | 135.0 (106.8, 173.0) | <0.001* |
ACC duration (min) | 88.0 (64.0, 118.0) | 103.0 (73.0, 138.0) | 84.0 (62.0, 110.0) | <0.001* |
Minimum temperature (℃) | 31.0 (30.1, 31.9) | 30.9 (29.9, 31.7) | 31.1 (30.3, 32.0) | 0.005* |
Intra-op transfusion | ||||
RBC (U) | 2.0 (1.5, 2.6) | 2.3 (1.7, 3.1) | 1.8 (1.3, 2.3) | 0.07 |
Hydroxyethyl starch (mL) | 354.5 (288.6, 424.3) | 388.7 (338.4, 440.3) | 326.5 (276.7, 378.9) | 0.60 |
Emergency surgery | 20 (2.9) | 11 (5.5) | 9 (1.8) | 0.02* |
Post-op data | ||||
APACHE II scores | 9.8 (6.8, 12.8) | 11.8 (7.5, 16.1) | 8.6 (6.2, 11.0) | <0.001* |
sCysC (mg/L) | 1.0 (0.8, 1.3) | 1.2 (0.9, 1.7) | 0.9 (0.7, 1.1) | <0.001* |
uNAG (U/g Cre) | 8.0 (1.6, 20.8) | 12.2 (4.7, 25.7) | 4.8 (1.3, 18.2) | <0.001* |
CK (U/L) | 530.0 (393.0, 746.0) | 635.0 (451.0, 848.0) | 505.0 (365.0, 690.2) | <0.001* |
CK-MB (U/L) | 61.2 (42.2, 87.8) | 69.9 (47.8, 101.8) | 58.9 (40.3, 83.0) | <0.001* |
NT-proBNP (pg/mL) | 1,565.0 (636.6, 3,556.0) | 2,638.0 (1,041.0, 5,000.0) | 1,336.2 (536.9, 2,864.2) | <0.001* |
cTnI (ng/mL) | 654.6 (304.4, 1,183.0) | 925.5 (465.8, 2,031.0) | 554.5 (265.8, 1,027.0) | <0.001* |
Data were presented as median (interquartile range) or n (%).*, there is a statistical difference. AKI, acute kidney injury; CABG, coronary artery bypass grafting; Other procedures, including correction of congenital heart disease, repair of aortic sinus aneurysm, etc.; Intra-op, intraoperative; CPB, cardiopulmonary bypass; ACC, aortic cross-clamping; RBC, red blood cell; Post-op, postoperative; APACHE, acute physiology and chronic health evaluation; sCysC, serum cystatin C; uNAG, urine N-acetyl-β-D-glucosidase; CK, creatine kinase; CK-MB, creatine kinase isoenzyme; NT-proBNP, N-terminal B-type natriuretic peptide precursor; cTnI, cardiac troponin I.
In terms of postoperative data, patients in the AKI group had higher postoperative APACHE II scores [11.8 (7.5, 16.1) vs. 8.6 (6.2, 11.0), P<0.001], postoperative sCysC [1.2 (0.9, 1.7) vs. 0.9 (0.7, 1.1) mg/L, P<0.001], uNAG [12.2 (4.7, 25.7) vs. 4.8 (1.3, 18.2) U/g Cre, P<0.001], serum CK [635.0 (451.0, 848.0) vs. 505.0 (365.0, 690.2) U/L, P<0.001], CK-MB [69.9 (47.8, 101.8) vs. 58.9 (40.3, 83.0) U/L, P<0.001], NT-proBNP [2,638.0 (1,041.0, 5,000.0) vs. 1,336.2 (536.9, 2,864.2) pg/mL, P<0.001], and cTnI [925.5 (465.8, 2,031.0) vs. 554.5 (265.8, 1,027.0) ng/mL, P<0.001].
Comparison of clinical characteristics and outcomes of patients with different stages of AKI in the modeling cohort
Clinical data and outcomes of the AKI stage subgroups in the modeling cohort are shown in Table 5. In the AKI group, the levels of postoperative sCysC, uNAG, cTnI, and APACHE II scores increased with increasing AKI stage, whereas preoperative serum albumin, hemoglobin concentration, and minimum intraoperative temperature decreased with increasing AKI stage. In addition, the percentage of emergency surgery and ECMO was significantly higher in patients with stage 3 AKI than in other subgroups. In terms of adverse outcomes, patients with stage 3 AKI had a mortality rate of 19.0%, the incidence of CRRT and IABP was 38.1% and 33.3%, respectively, which were significantly higher than those of other subgroups. These patients also had higher hospital costs, prolonged duration of mechanical ventilation and ICU stay.
Table 5
Variables | Total (n=689) | Non-AKI (n=488) | AKI stage 1 (n=132) | AKI stage 2 (n=48) | AKI stage 3 (n=21) | P value |
---|---|---|---|---|---|---|
Male, n (%) | 417 (60.5) | 308 (63.1) | 75 (56.8) | 24 (50.0) | 10 (47.6) | 0.12 |
Age (years) | 57.0 (48.0, 64.0) | 55.0 (46.0, 62.0) | 62.5 (54.0, 68.0) | 62.0 (54.8, 67.2) | 65.0 (53.0, 68.0) | <0.001* |
Weight (kg) | 60.0 (53.0, 67.0) | 60.0 (53.0, 67.0) | 58.0 (52.0, 67.0) | 57.2 (52.5, 67.2) | 56.0 (52.5, 70.0) | 0.75 |
Medical history | ||||||
Hypertension | 158 (22.9) | 87 (17.8) | 47 (35.6) | 14 (29.2) | 10 (47.6) | <0.001* |
Diabetes mellitus | 62 (9.0) | 35 (7.2) | 17 (12.9) | 7 (14.6) | 3 (14.3) | 0.047 |
Hyperlipidemia | 5 (0.7) | 4 (0.8) | 0 (0.0) | 0 (0.0) | 1 (4.8) | 0.19 |
CAD | 12 (1.7) | 6 (1.2) | 6 (4.5) | 0 (0.0) | 0 (0.0) | 0.10 |
CKD | 5 (0.7) | 2 (0.4) | 2 (1.5) | 0 (0.0) | 1 (4.8) | 0.01 |
Nephrotoxic drugs | 33 (4.8) | 23 (4.7) | 5 (3.8) | 4 (8.3) | 1 (4.8) | 0.07 |
Pre-op LVEF (%) | 63.0 (57.0, 67.0) | 63.0 (57.0, 67.0) | 63.0 (55.0, 67.0) | 62.0 (54.8, 66.0) | 64.0 (57.0, 66.0) | 0.86 |
Pre-op examination | ||||||
Hemoglobin (g/L) | 134.0 (120.0, 145.0) | 136.0 (123.0, 146.0) | 129.0 (115.8, 138.0) | 128.5 (118.0, 141.5) | 125.0 (96.0, 142.0) | 0.001* |
D-Dimer (ng/mL) | 360.0 (240.0, 830.0) | 330.0 (220.0, 722.5) | 510.0 (295.0, 1,132.5) | 390.0 (275.0, 915.0) | 670.0 (320.0, 1,190.0) | <0.001* |
Albumin (g/L) | 38.5 (36.3, 40.9) | 38.8 (36.6, 41.3) | 37.7 (35.1, 39.6) | 38.1 (36.0, 40.6) | 37.0 (32.5, 40.1) | 0.001* |
Baseline sCr (µmol/L) | 78.0 (65.3, 93.6) | 78.5 (66.8, 92.7) | 76.8 (62.7, 96.4) | 79.5 (63.3, 91.0) | 82.1 (71.1, 150.5) | 0.25 |
Surgical procedures | ||||||
Valve surgery | 545 (79.1) | 400 (82.0) | 94 (71.2) | 32 (66.7) | 19 (90.5) | 0.18 |
CABG | 51 (7.4) | 30 (6.1) | 15 (11.4) | 6 (12.5) | 0 (0.0) | 0.06 |
CABG + valve surgery | 44 (6.4) | 23 (4.7) | 12 (9.1) | 7 (14.6) | 2 (9.5) | 0.02* |
Other procedures | 49 (7.1) | 35 (7.2) | 11 (8.3) | 3 (6.2) | 0 (0.0) | 0.71 |
Intra-op data | ||||||
CPB duration (min) | 145.0 (113.0, 186.0) | 135.0 (106.8, 173.0) | 172.0 (125.0, 200.0) | 189.5 (139.0, 251.8) | 175.0 (143.0, 211.0) | <0.001* |
ACC duration (min) | 88.0 (64.0, 118.0) | 84.0 (62.0, 110.0) | 104.0 (70.0, 129.2) | 104.5 (74.8, 147.2) | 102.0 (86.0, 142.0) | <0.001* |
Minimum temperature (℃) | 31.0 (30.1, 31.9) | 31.1 (30.3, 32.0) | 30.9 (30.0, 31.8) | 30.8 (29.6, 31.6) | 30.6 (29.7, 31.4) | 0.03* |
Intra-op transfusion | ||||||
RBC (U) | 2.0 (1.5, 2.6) | 1.8 (1.3, 2.3) | 1.9 (1.4, 2.5) | 1.8 (1.3, 2.4) | 2.0 (1.5, 2.5) | 0.09 |
Hydroxyethyl starch (mL) | 354.5 (288.6, 424.3) | 326.5 (276.7, 378.9) | 365.5 (316.0, 410.7) | 398.3 (338.4, 454.5) | 386.5 (326.7, 448.9) | 0.27 |
Emergency surgery | 20 (2.9) | 9 (1.8) | 6 (4.5) | 3 (6.3) | 2 (9.5) | 0.02* |
Post-op data | ||||||
APACHE II scores | 9.8 (6.8, 12.8) | 8.6 (6.2, 11.0) | 10.3 (8.1, 12.6) | 12.2 (9.7, 14.6) | 13.8 (11.7, 15.9) | <0.001* |
sCysC (mg/L) | 1.0 (0.8, 1.3) | 0.9 (0.7, 1.1) | 1.1 (0.9, 1.4) | 1.4 (1.1, 1.9) | 1.9 (1.2, 2.6) | <0.001* |
uNAG (U/g Cre) | 8.0 (1.6, 20.8) | 4.8 (1.3, 18.2) | 10.9 (3.2, 18.6) | 19.3 (10.6, 32.4) | 24.2 (20.8, 30.5) | <0.001* |
CK (U/L) | 530.0 (393.0, 746.0) | 505.0 (365.0, 690.2) | 547.5 (422.0, 778.0) | 754.0 (533.8, 952.5) | 643.0 (448.0, 843.0) | <0.001* |
CK-MB (U/L) | 61.2 (42.2, 87.8) | 58.9 (40.3, 83.0) | 69.8 (47.7, 93.5) | 76.0 (48.9, 117.6) | 69.1 (50.0, 119.0) | 0.001* |
NT-proBNP (pg/mL) | 1,565.0 (636.6, 3,556.0) | 1,336.2 (536.9, 2,864.2) | 2,451.0 (901.0, 4,523.2) | 2,549.5 (1,047.8, 5,253.5) | 3,678.0 (2,115.0, 13,684.0) | <0.001* |
cTnI (ng/mL) | 654.6 (304.4, 1,183.0) | 554.5 (265.8, 1,027.0) | 843.6 (396.6, 1,507.0) | 985.5 (669.6, 2,555.0) | 1,076.0 (864.7, 2,657.0) | <0.001* |
Outcomes | ||||||
ICU LOS (h) | 45.0 (27.0, 112.0) | 42.0 (22.0, 68.2) | 85.0 (40.8, 161.2) | 134.0 (79.2, 212.5) | 222.0 (154.0, 383.0) | <0.001* |
CRRT | 19 (2.8) | 4 (0.8) | 3 (2.3) | 4 (8.3) | 8 (38.1) | <0.001* |
IABP | 43 (6.2) | 16 (3.3) | 9 (6.8) | 11 (22.9) | 7 (33.3) | <0.001* |
ECMO | 11 (1.6) | 5 (1.0) | 1 (0.8) | 3 (6.3) | 2 (9.5) | 0.005* |
MV (h) | 20.0 (13.0, 43.0) | 17.0 (10.8, 24.0) | 25.0 (18.0, 72.2) | 65.0 (37.5, 142.2) | 132.0 (47.0, 218.0) | <0.001* |
Hospital costs (10k CNY) | 14.8 (11.8, 19.4) | 13.5 (10.8, 16.9) | 17.8 (14.1, 23.3) | 21.6 (18.0, 27.4) | 30.0 (20.8, 36.8) | <0.001* |
In-hospital mortality | 20 (2.9) | 5 (1.0) | 3 (2.3) | 8 (16.7) | 4 (19.0) | <0.001* |
Data were presented as median (interquartile range) or n (%). *, there is a statistical difference. CSA-AKI, cardiac surgery-associated acute kidney injury; CAD, coronary artery disease; CKD, chronic kidney disease; Nephrotoxic drugs, including immunosuppressants, angiotensin-converting enzyme inhibitors, angiotensin receptor blockers, non-steroidal anti-inflammatory drugs, antibiotics (including vancomycin, acyp-clovir, amphotericin B, aminoglycosides), mannitol, angiography agents, etc.; Pre-op, preoperative; LVEF, left ventricular ejection fraction; CABG, coronary artery bypass grafting; Other procedures, including correction of congenital heart disease, repair of aortic sinus aneurysm, etc.; Intra-op, intraoperative; CPB, cardiopulmonary bypass, ACC, aortic cross clamping; RBC, red blood cell; Post-op, postoperative; APACHE, acute physiologic and chronic health evaluation; sCysC, serum cystatin C; uNAG, urine N-acetyl-β-D-glucosidase; NT-proBNP, N-terminal B-type natriuretic peptide precursor; cTnI, cardiac troponin I; ICU LOS, intensive care unit length of stay; CRRT, continuous renal replacement therapy; IABP, intra-aortic balloon pump; ECMO, extracorporeal membrane oxygenation; MV, mechanical ventilation; CNY, Chinese yuan.
Clinical early prediction model based on LASSO regression
We included all 31 preselected variables from the modeling cohort of 689 postoperative cardiac patients and used LASSO regression to develop a clinical prediction model. In LASSO regression, the regularization parameter λ was obtained by 10-fold cross-validation with the minimum criterion. Figure 2A shows the LASSO coefficient profiles generated from the log(λ) series for all variables selected. The partial likelihood difference curves were plotted by Log(λ), and vertical dashed lines are plotted at the minimum criterion and the optimal value of the 1-SE criterion (Figure 2B). In this study, log(λ) was determined to be 0.03064213 (1-SE criterion) after using 10-fold cross-validation. After plotting vertical lines at the determined values using 10-fold cross-validation, the 11 best nonzero coefficient predictors were screened out, which were age, hypertension, baseline sCr, preoperative albumin level, preoperative hemoglobin concentration, postoperative NT-proBNP, postoperative cTnI, CABG combined with valve surgery, duration of CPB, sCysC, and uNAG. The parameters of each factor in the model are shown in Table 6.
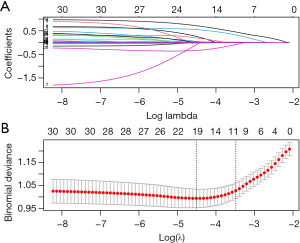
Table 6
Variables | OR | 95% CI | P value |
---|---|---|---|
Age | 1.039 | 1.020–1.059 | <0.001 |
Hypertension | 2.111 | 1.339–3.327 | 0.001 |
Baseline sCr | 0.998 | 0.998–0.999 | 0.04 |
Pre-op albumin | 0.957 | 0.905–1.013 | 0.13 |
Pre-op hemoglobin | 0.991 | 0.980–1.002 | 0.10 |
Post-op NT-proBNP | 1.000 | 1.000–1.000 | 0.005 |
Post-op cTnI | 1.000 | 1.000–1.001 | <0.001 |
CABG + valve surgery | 0.596 | 0.329–1.081 | 0.09 |
CPB duration | 1.007 | 1.004–1.011 | <0.001 |
sCysC | 2.694 | 1.742–4.165 | <0.001 |
uNAG | 1.017 | 1.006–1.028 | 0.003 |
CSA-AKI, cardiac surgery-associated acute kidney injury; sCr, serum creatinine; Pre-op, preoperative; Post-op, postoperative; NT-proBNP, N-terminal B-type natriuretic peptide precursor; cTnI, cardiac troponin I; CABG, coronary artery bypass grafting; CPB, cardiopulmonary bypass; sCysC, serum cystatin C; uNAG, urine N-acetyl-β-D-glucosidase; OR, odds ratio; CI, confidence interval.
Predictive effects of relevant variables on CSA-AKI
Based on the screening results of the LASSO regression, we further used ROC to evaluate the predictive effectiveness of the measures independently associated with CSA-AKI. Figure 3 shows the ROC and box plots of age (Figure 3A,3B), CPB duration (Figure 3C,3D), sCysC (Figure 3E,3F), uNAG (Figure 3G,3H), cTnI (Figure 3I,3J), and NT-proBNP (Figure 3K,3L) for the prediction of CSA-AKI independently, with ROC-AUC of 0.650 (0.610–0.700), 0.670 (0.630–0.710), 0.730 (0.680–0.770), 0.650 (0.610–0.690), 0.650 (0.610–0.700), and 0.640 (0.590–0.690), respectively. As can be seen, these variables alone are not powerful enough to predict CSA-AKI.
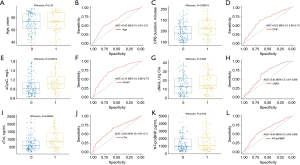
Model effectiveness evaluation
We developed a CSA-AKI clinical prediction model including 11 variables screened by LASSO regression including age, hypertension, baseline sCr, preoperative albumin, preoperative hemoglobin, postoperative NT-proBNP, cTnI, sCysC, uNAG, CABG combined with valve surgery, and CPB duration. Then we used ROC, calibration curve, DCA to evaluate their differentiation, calibration and clinical benefit, respectively. Figure 4A shows that the ROC-AUC of the model was 0.830 (0.800–0.860), suggesting that its differentiation and prediction efficacy were satisfactory. Figure 4B shows the calibration curve of the model, suggesting that its prediction accuracy was good. Figure 4C shows the DCA of the model, with the vertical coordinate representing the net benefit, and the horizontal coordinate representing the threshold probability (i.e., the expected benefit of no treatment is equal to that of full treatment), the red line representing the early prediction model, the green dashed line representing full treatment, and the blue dashed line representing no treatment. The results showed that this model increased net clinical benefit than the “all treatment” or “no treatment” scenarios at a threshold probability in the range of 0.09–0.80.
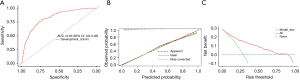
Establishment of validation cohort and external validation of the model
This study continued to enroll 313 adult cardiac surgery patients from May 2022 through December 2022 according to the aforementioned inclusion and exclusion criteria, which were used to establish an external validation cohort (Figure S1). Table S1 shows the clinical data and outcomes of the validation cohort compared to the modeling cohort. A total of 124 patients in the validation cohort had AKI within one week after surgery, with an incidence rate of 39.6%, which was higher than that in the modeling cohort. Of these, 77 cases had stage 1 AKI (24.6%), 25 cases had stage 2 AKI (8.0%), and 22 cases had stage 3 AKI (7.0%). In terms of the patient distribution, the validation cohort had a higher incidence of stage 1 AKI (24.6% vs. 19.2%, P=0.002) and stage 3 AKI (7.0% vs. 3.0%, P=0.03) than the modeling cohort. Based on the variables screened by LASSO regression, the CSA-AKI early prediction model for the validation cohort was established with the variables of age, hypertension, baseline sCr, preoperative albumin, preoperative hemoglobin, CABG combined with valve surgery, CPB duration, and postoperative NT-proBNP, cTnI, sCysC, and uNAG. Figure 5A shows that the ROC-AUC was 0.840 (0.790–0.880), which was not significantly different from the ROC-AUC of the modeling cohort [0.830 (0.800–0.860), P>0.99]. The calibration curve indicated a good fit of the model (Figure 5B).
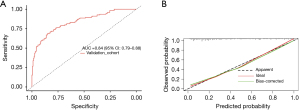
Plotting the nomogram of the CSA-AKI clinical prediction model
To facilitate the clinical use of the early prediction model, we drew a nomogram for CSA-AKI (Figure 6). This nomogram allows the clinician to obtain the corresponding score on the scale based on the values of each variable. The sum of each score gives the calculated “RISK” on the total score axis, which is the risk for CSA-AKI. Figure 7 shows the Nomo-ROC and Nomo-DCA of the nomogram. The ROC-AUC of the nomogram is significantly larger than the ROC-AUC of each variable in the model (Figure 7A). And the area under the DCA curve is also larger than the area under the DCA curve of each variable in the model (Figure 7B). These results suggested the rationality and the diagnostic effectiveness of the nomogram were satisfactory.
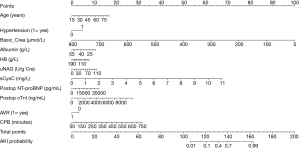
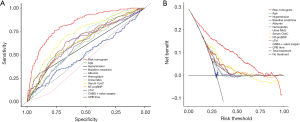
Discussion
According to KDIGO criteria, the incidence of CSA-AKI in our study was 29.2%. Patients with CSA-AKI exhibited a significant association with increased in-hospital morbidity and mortality, prolonged ICU length of stay, and escalated healthcare costs. These findings align with the previous study (2), further emphasizing the critical role of CSA-AKI as a risk factor for postoperative mortality. In this prospective study, we developed and validated an early prediction model for CSA-AKI, incorporating the renal biomarkers sCysC and uNAG, along with the cardiac biomarkers NT-proBNP and cTnI. Implementing this prediction model for CSA-AKI might enable early diagnostic evaluation, facilitate the prompt identification of high-risk patients, and support individualized interventions.
This study unveiled several key findings regarding postoperative CSA-AKI. Patients who developed CSA-AKI were predominantly characterized by advanced age, hypertension, and diabetes mellitus, highlighting the influence of multiple factors in its occurrence. Regression analysis further identified several independent risk factors for CSA-AKI, including age, history of hypertension, CPB time, and postoperative elevations of NT-proBNP, cTnI, sCysC, and uNAG. The associations between age, hypertension, and CSA-AKI have been previously reported in the literature (25,26). CPB is recognized as a common risk factor for CSA-AKI, with underlying mechanisms of immune system activation, tissue edema, and neutrophil-mediated inflammation (27). Our results showed a significant correlation between prolonged CPB and CSA-AKI. Therefore, it is imperative to focus on improving surgical techniques and minimizing CPB time to mitigate the incidence of CSA-AKI.
Our study also revealed that elevated levels of NT-proBNP and cTnI immediately after surgery were independent risk factors for CSA-AKI. NT-proBNP and cTnI are commonly used cardiac biomarkers to assess heart function and myocardial injury. NT-proBNP is a peptide secreted by the ventricle in response to overstretching of cardiomyocytes, and its role is to promote sodium diuresis (14). A previous study has found a correlation between renal dysfunction and elevated vena cava pressure and dilated cardiac ventricular walls in patients with heart disease. In patients with elevated vena cava pressure, the increased reverse pressure is transmitted uniformly through the venous system. The increased pressure in the renal veins results in renal congestion and leads to decreased eGRF and sodium excretion (28). Thus, NT-proBNP physiologically reflects ventricular dilatation and correlates with the development of AKI. Palazzuoli et al. found that NT-proBNP had a good predictive value for the development of AKI in patients with heart failure (29). Additionally, NT-proBNP is recognized as a biomarker of “cardiac and renal burden” in emergency patients, serving as one of the risk factors for CSA-AKI (30).
Elevated cTnI is widely recognized as the most sensitive and specific biomarker for myocardial injury, with mechanisms involving myocardial malperfusion, inflammatory stress, and mechanical injuries (15). Haines et al. reported a significant elevation of both cTnI and NT-proBNP in patients with AKI requiring urgent RRT, and these biomarkers were associated with AKI at any given time point (16). Consistent with these findings, our study demonstrated a significant association between postoperative elevations of NT-proBNP and cTnI and the occurrence of AKI following cardiac surgery. This suggests that CSA-AKI may be linked to factors such as excessive fluid loading, impaired heart function, myocardial injury, and malperfusion. Consequently, the management of patients undergoing cardiac surgery should prioritize fluid management, myocardial protection, and optimization of myocardial perfusion to mitigate the risk of CSA-AKI.
Furthermore, this study identified elevated levels of sCysC and uNAG immediately following cardiac surgery as independent risk factors for CSA-AKI. Presently, sCysC is recognized as a more sensitive indicator of glomerular filtration function compared to serum creatinine (sCr). A meta-analysis demonstrated that sCysC exhibited diagnostic superiority to sCr, with a detection time that was 24–48 hours earlier in diagnosing AKI (31). Additionally, Haase-Fielitz et al. reported that sCysC exhibited a sensitivity of 71% and specificity of 53% in diagnosing AKI within 6 hours after cardiac surgery in adult patients (32). Consequently, sCysC has gained widespread use as a valuable tool in assessing AKI risk in high-risk patient populations.
NAG is a lysosomal enzyme produced in the distal and proximal tubular cells of the kidney. It cannot be filtered by the glomerular filtration membrane due to its large molecular mass. Thus, it is a sensitive marker for detecting tubular injury because it is released directly into the urine during tubular injury (33). Some studies have suggested that uNAG is significantly elevated in patients with AKI and has some predictive value (11,12). In another study on AKI in critically ill patients, the ROC-AUC of uNAG was only 0.68, which was not very predictive (34). Further evidence is required to establish the predictive value of uNAG in AKI. In our present study, immediate levels of both sCysC and uNAG following cardiac surgery were significantly higher in patients who developed CSA-AKI. Moreover, the levels of both biomarkers increased with the severity of AKI, underscoring their potential as valuable biomarkers for CSA-AKI.
Patients undergoing cardiac surgery exhibit significant heterogeneity due to variations in preoperative cardiac function, medical history, and the specific type of surgery. The etiology and pathogenesis of CSA-AKI involve a multitude of factors, prompting the Acute Dialysis Quality Initiative Working Group to recommend the combination of biomarkers of different natures to enhance the effectiveness of early prediction models (7). Previous studies have combined different biomarkers to establish AKI prediction models. For example, Basu et al. combined urinary neutrophil gelatinase-associated lipocalin with sCysC to predict AKI after extracorporeal circulation in children (35). Yang et al. suggested that sCysC in combination with urinary renal injury molecule-1 could improve the identification of AKI in patients with heart failure (36). A study conducted in medical cardiac intensive care units suggested that both urinary liver-type fatty-acid binding protein (P<0.0001) and NT-proBNP (P=0.006) were independently associated with the development of AKI, when used in combination, improve early prediction of AKI (37). These studies suggest that combining different specimens or specimens from different organ sources could improve the ability to predict heart-related AKI.
Previous prediction models for CSA-AKI have mostly used acute renal failure requiring RRT as the endpoint. For example, the Cleveland Clinical Score (4), the Mehta Score (5), and the Simplified Renal Index Score (6), are all composed of clinical characteristics and independent risk factors. However, the external validation of these models showed that their predictive values were not as expected (38). Several factors contribute to this discrepancy. Firstly, the diagnostic criteria for CSA-AKI have been inconsistent, and the timing of RRT initiation has varied across studies. Secondly, the heterogeneity in surgical approaches, patient populations, and geographic regions has compromised the effectiveness of these prediction models.
In our present study, we integrated biomarkers indicative of glomerular and tubular injury, as well as biomarkers reflecting cardiac injury. While integrating these biomarkers enhances predictive accuracy, it’s crucial to consider their cost-effectiveness. Fortunately, detection methods for these biomarkers are well-established in most hospitals, making the costs relatively low. Moreover, these biomarker data were collected only once after surgery, avoiding repeated tests and further reducing expenses. The model developed in this study has undergone analysis for differentiation, calibration, clinical applicability, and external validation. However, a prediction model alone may not be convenient for clinical applications. Therefore, a risk nomogram was also developed to facilitate practical implementation. Integrating this tool with electronic health systems could further improve its usability and impact.
This study has several limitations. First, it is a single-center, prospective study, and although efforts were made to ensure the cases were representative, there may still be selective bias due to factors such as geographic population characteristics, surgical protocols, and technical differences. To address this limitation, we aim to propose a cross-regional and multicenter study in the future to externally validate the results obtained in this study. Second, the data used for modeling in this study were derived solely from the population in southern China, and there is a lack of analysis on other populations. Therefore, further research is necessary to validate the applicability of the model to other racial and ethnic groups. The third limitation is the lack of long-term follow-up data beyond hospital discharge. Although our study focused on early prediction, we recognize the importance of evaluating long-term outcomes. Future research will incorporate extended follow-up to address this issue. Fourth, there is a possibility of residual confounding in this study, although we used rigorous methods to minimize this effect. Fifth, the selection of variables in this study was based on the available literature and our clinical experience, and it is possible that some variables, such as new biomarkers of inflammation (39), may have been overlooked. Finally, all biomarkers were collected immediately after cardiac surgery, and their concentrations may vary with eGFR following the onset of CSA-AKI. A single measurement may limit the reference value of these biomarkers. However, it is important to note that continuous sampling is not recommended by KDIGO due to the low feasibility and high cost of repeated sampling. Therefore, the results obtained in this study are not undermined by this limitation.
In the future, the study will be expanded to multiple centers to further validate or refine the model. Long-term follow-up data will be incorporated to evaluate outcomes beyond the immediate postoperative period. Additionally, the inclusion of new biomarkers may be explored to improve predictive accuracy.
Conclusions
In summary, CSA-AKI is a common complication with significant adverse outcomes. The early prediction model for CSA-AKI using immediate postoperative renal biomarkers (sCysC and uNAG) and cardiac biomarkers (NT-proBNP and cTnI) is a potential approach. Our findings suggest that biomarkers from multiple sources might help in predicting complex clinical complications.
Acknowledgments
Funding: This work was supported by
Footnote
Reporting Checklist: The authors have completed the TRIPOD reporting checklist. Available at https://jtd.amegroups.com/article/view/10.21037/jtd-24-1185/rc
Data Sharing Statement: Available at https://jtd.amegroups.com/article/view/10.21037/jtd-24-1185/dss
Peer Review File: Available at https://jtd.amegroups.com/article/view/10.21037/jtd-24-1185/prf
Conflicts of Interest: All authors have completed the ICMJE uniform disclosure form (available at https://jtd.amegroups.com/article/view/10.21037/jtd-24-1185/coif). The authors have no conflicts of interest to declare.
Ethical Statement: The authors are accountable for all aspects of the work in ensuring that questions related to the accuracy or integrity of any part of the work are appropriately investigated and resolved. The study was conducted in accordance with the Declaration of Helsinki (as revised in 2013). Informed consent was waived due to the use of de-identified and anonymized data, ensuring confidentiality and privacy for statistical research purposes only. The study was approved by the Ethics Committee of Guangdong Provincial People’s Hospital [approval No. GDREC2015396H(R1)].
Open Access Statement: This is an Open Access article distributed in accordance with the Creative Commons Attribution-NonCommercial-NoDerivs 4.0 International License (CC BY-NC-ND 4.0), which permits the non-commercial replication and distribution of the article with the strict proviso that no changes or edits are made and the original work is properly cited (including links to both the formal publication through the relevant DOI and the license). See: https://creativecommons.org/licenses/by-nc-nd/4.0/.
References
- Ortega-Loubon C, Fernández-Molina M, Carrascal-Hinojal Y, et al. Cardiac surgery-associated acute kidney injury. Ann Card Anaesth 2016;19:687-98. [Crossref] [PubMed]
- Bove T, Monaco F, Covello RD, et al. Acute renal failure and cardiac surgery. HSR Proc Intensive Care Cardiovasc Anesth 2009;1:13-21. [PubMed]
- Hobson CE, Yavas S, Segal MS, et al. Acute kidney injury is associated with increased long-term mortality after cardiothoracic surgery. Circulation 2009;119:2444-53. [Crossref] [PubMed]
- Thakar CV, Arrigain S, Worley S, et al. A clinical score to predict acute renal failure after cardiac surgery. J Am Soc Nephrol 2005;16:162-8. [Crossref] [PubMed]
- Mehta RH, Grab JD, O'Brien SM, et al. Bedside tool for predicting the risk of postoperative dialysis in patients undergoing cardiac surgery. Circulation 2006;114:2208-16; quiz 2208. [Crossref] [PubMed]
- Wijeysundera DN, Karkouti K, Dupuis JY, et al. Derivation and validation of a simplified predictive index for renal replacement therapy after cardiac surgery. JAMA 2007;297:1801-9. [Crossref] [PubMed]
- Ostermann M, Joannidis M. Acute kidney injury 2016: diagnosis and diagnostic workup. Crit Care 2016;20:299. [Crossref] [PubMed]
- Dharnidharka VR, Kwon C, Stevens G. Serum cystatin C is superior to serum creatinine as a marker of kidney function: a meta-analysis. Am J Kidney Dis 2002;40:221-6. [Crossref] [PubMed]
- Hou Y, Deng Y, Hu L, et al. Assessment of 17 clinically available renal biomarkers to predict acute kidney injury in critically ill patients. J Transl Int Med 2021;9:273-84. [Crossref] [PubMed]
- Strack C, Bauer S, Hubauer U, et al. N-acetyl-ß-D-glucosaminidase is predictive of mortality in chronic heart failure: a 10-year follow-up. Biomark Med 2021;15:1143-53. [Crossref] [PubMed]
- Deng Y, Ma J, Hou Y, et al. Combining Serum Cystatin C and Urinary N-Acetyl-Beta-D-Glucosaminidase Improves the Precision for Acute Kidney Injury Diagnosis after Resection of Intracranial Space-Occupying Lesions. Kidney Blood Press Res 2020;45:142-56. [Crossref] [PubMed]
- Deng Y, Chi R, Chen S, et al. Evaluation of clinically available renal biomarkers in critically ill adults: a prospective multicenter observational study. Crit Care 2017;21:46. [Crossref] [PubMed]
- Fang M, Li J, Fang H, et al. Prediction of acute kidney injury after total aortic arch replacement with serum cystatin C and urine N-acetyl-β-d-glucosaminidase: A prospective observational study. Clin Chim Acta 2023;539:105-13. [Crossref] [PubMed]
- Daniels LB, Maisel AS. Natriuretic peptides. J Am Coll Cardiol 2007;50:2357-68. [Crossref] [PubMed]
- Kim JS, Kim M, Kim YJ, et al. Troponin Testing for Assessing Sepsis-Induced Myocardial Dysfunction in Patients with Septic Shock. J Clin Med 2019;8:239. [Crossref] [PubMed]
- Haines R, Crichton S, Wilson J, et al. Cardiac biomarkers are associated with maximum stage of acute kidney injury in critically ill patients: a prospective analysis. Crit Care 2017;21:88. [Crossref] [PubMed]
- Bucholz EM, Whitlock RP, Zappitelli M, et al. Cardiac biomarkers and acute kidney injury after cardiac surgery. Pediatrics 2015;135:e945-56. [Crossref] [PubMed]
- Skrivankova VW, Richmond RC, Woolf BAR, et al. Strengthening the Reporting of Observational Studies in Epidemiology Using Mendelian Randomization: The STROBE-MR Statement. JAMA 2021;326:1614-21. [Crossref] [PubMed]
- Bossuyt PM, Reitsma JB, Bruns DE, et al. Toward complete and accurate reporting of studies of diagnostic accuracy: the STARD initiative. Acad Radiol 2003;10:664-9. [Crossref] [PubMed]
- Bihorac A, Chawla LS, Shaw AD, et al. Validation of cell-cycle arrest biomarkers for acute kidney injury using clinical adjudication. Am J Respir Crit Care Med 2014;189:932-9. [Crossref] [PubMed]
- Thomas ME, Blaine C, Dawnay A, et al. The definition of acute kidney injury and its use in practice. Kidney Int 2015;87:62-73. [Crossref] [PubMed]
- Endre ZH, Walker RJ, Pickering JW, et al. Early intervention with erythropoietin does not affect the outcome of acute kidney injury (the EARLYARF trial). Kidney Int 2010;77:1020-30. [Crossref] [PubMed]
- Sauerbrei W, Royston P, Binder H. Selection of important variables and determination of functional form for continuous predictors in multivariable model building. Stat Med 2007;26:5512-28. [Crossref] [PubMed]
- Vickers AJ, Elkin EB. Decision curve analysis: a novel method for evaluating prediction models. Med Decis Making 2006;26:565-74. [Crossref] [PubMed]
- Zhou H, Wang G, Yang L, et al. Acute Kidney Injury After Total Arch Replacement Combined With Frozen Elephant Trunk Implantation: Incidence, Risk Factors, and Outcome. J Cardiothorac Vasc Anesth 2018;32:2210-7. [Crossref] [PubMed]
- Jain A, Tracci MC, Coleman DM, et al. Renal malperfusion: spontaneous renal artery dissection and with aortic dissection. Semin Vasc Surg 2013;26:178-88. [Crossref] [PubMed]
- Pickering JW, James MT, Palmer SC. Acute kidney injury and prognosis after cardiopulmonary bypass: a meta-analysis of cohort studies. Am J Kidney Dis 2015;65:283-93. [Crossref] [PubMed]
- Nohria A, Hasselblad V, Stebbins A, et al. Cardiorenal interactions: insights from the ESCAPE trial. J Am Coll Cardiol 2008;51:1268-74. [Crossref] [PubMed]
- Palazzuoli A, Masson S, Ronco C, et al. Clinical relevance of biomarkers in heart failure and cardiorenal syndrome: the role of natriuretic peptides and troponin. Heart Fail Rev 2014;19:267-84. [Crossref] [PubMed]
- Patel UD, Garg AX, Krumholz HM, et al. Preoperative serum brain natriuretic peptide and risk of acute kidney injury after cardiac surgery. Circulation 2012;125:1347-55. [Crossref] [PubMed]
- McDougal WS. Early detection of acute renal failure by serum cystatin C. J Urol 2005;174:1024-5. [Crossref] [PubMed]
- Haase-Fielitz A, Bellomo R, Devarajan P, et al. Novel and conventional serum biomarkers predicting acute kidney injury in adult cardiac surgery--a prospective cohort study. Crit Care Med 2009;37:553-60. [Crossref] [PubMed]
- Li J, Li QX, Xie XF, et al. Differential roles of dihydropyridine calcium antagonist nifedipine, nitrendipine and amlodipine on gentamicin-induced renal tubular toxicity in rats. Eur J Pharmacol 2009;620:97-104. [Crossref] [PubMed]
- Charlton JR, Portilla D, Okusa MD. A basic science view of acute kidney injury biomarkers. Nephrol Dial Transplant 2014;29:1301-11. [Crossref] [PubMed]
- Basu RK, Wong HR, Krawczeski CD, et al. Combining functional and tubular damage biomarkers improves diagnostic precision for acute kidney injury after cardiac surgery. J Am Coll Cardiol 2014;64:2753-62. [Crossref] [PubMed]
- Yang CH, Chang CH, Chen TH, et al. Combination of Urinary Biomarkers Improves Early Detection of Acute Kidney Injury in Patients With Heart Failure. Circ J 2016;80:1017-23. [Crossref] [PubMed]
- Naruse H, Ishii J, Takahashi H, et al. Predicting acute kidney injury using urinary liver-type fatty-acid binding protein and serum N-terminal pro-B-type natriuretic peptide levels in patients treated at medical cardiac intensive care units. Crit Care 2018;22:197. [Crossref] [PubMed]
- Jiang W, Xu J, Shen B, et al. Validation of Four Prediction Scores for Cardiac Surgery-Associated Acute Kidney Injury in Chinese Patients. Braz J Cardiovasc Surg 2017;32:481-6. [Crossref] [PubMed]
- Karauzum I, Karauzum K, Acar B, et al. Predictive Value of Lymphocyte-to-monocyte Ratio in Patients with Contrast-induced Nephropathy After Percutaneous Coronary Intervention for Acute Coronary Syndrome. J Transl Int Med 2021;9:123-30. [Crossref] [PubMed]