Identification of serum biomarkers and therapeutic targets for aortic diseases in obesity through multi-omics analysis
Highlight box
Key findings
• Serum component 5 (C5) and apolipoprotein D (apoD) levels demonstrated higher sensitivity and specificity in predicting obesity-related aortic dissection (AD).
What is known and what is new?
• Serum C5a levels were found to be significantly higher in patients with abdominal aortic aneurysm compared to healthy individuals.
• Serum C5 levels were significantly lower in AD patients with body mass index (BMI) >24 kg/m2 compared to those with BMI ≤24 kg/m2 and healthy controls with BMI >24 kg/m2.
What is the implication, and what should change now?
• High BMI is associated with an increased risk of aortic disease, especially AD.
• Further research is necessary to explore the pathophysiological pathways associated with serum C5 and apoD, as well as their potential clinical applications.
Introduction
Background
The global prevalence of obesity is on the rise, affecting both children and adults, and leading to approximately 4 million deaths worldwide each year (1). Nearly 70% of deaths related to a high body mass index (BMI) are caused by cardiovascular diseases, making obesity a significant risk factor for such conditions (1). Obesity increases the risk of abdominal aortic aneurysm (AAA) (2), and obese patients with AAA may face higher operative risks (3). Elderly patients who are obese and present with acute aortic dissection (AD) are particularly susceptible to in-hospital mortality during undergoing open surgical repair (4).
Rationale and knowledge gap
Aortic diseases include aneurysms, dissections, intramural hematoma (IMH), and several other pathologies. Thoracic and abdominal aortic diseases are life-threatening conditions (5). In particular, AD often leads to catastrophic complications or death (6). Currently there are no effective drugs to prevent the occurrence and progression of aortic aneurysm (AA) or AD. Therefore, early detection and intervention are the most effective ways for reducing mortality.
Objective
A transcriptomics or proteomics approach is effective for analyzing the mechanisms and identifying of new biomarkers involved in cardiovascular diseases (7). In this study, we integrated transcriptomic analysis of aortic tissue with proteomic analysis of serum samples to identify potential biomarkers that could serve as early indicators and therapeutic targets for aortic diseases related to obesity. We present this article in accordance with the MDAR and ARRIVE reporting checklists (available at https://jtd.amegroups.com/article/view/10.21037/jtd-24-1113/rc).
Methods
Patient population
This study was performed at The First Affiliated Hospital of Soochow University from January 2022 to March 2023. A total of 237 consecutive patients with aortic disease and 72 patients with valvulopathy were enrolled based on findings from computed tomography angiography (CTA), magnetic resonance angiography, or ultrasound. AD is defined as a clear rupture in the aorta accompanied by the presence of a false lumen, as observed in CTA. Intramural aortic hematoma refers to the presence of a hematoma within the aortic media, without any evident rupture in the intima or the aortic vascular lumen. AA is identified by an aortic diameter that exceeds 1.5 times the normal size. Specifically, the diameter of the ascending aorta is greater than 5 cm, while the diameter of the descending aorta is greater than 4 cm. Patients with valvulopathy were identified as those with dysfunction of the mitral, tricuspid, or aortic valve resulting from rheumatic heart disease.
The following patients with aortic disease were excluded: (I) patients under 18 years of age; (II) family history of aortic disease or significant familial inherited aortic disease; (III) malignancy; (IV) traumatic dissection; (V) pregnant women.
According to the recommendations of the Chinese Obesity Working Group, overweight in adults is defined as a BMI of 24.0–28.0 kg/m2 and obesity as a BMI of 28 kg/m2 or higher (8,9). Patients were divided into three groups according to BMI: normal weight (BMI <24 kg/m2), overweight (BMI 24 to <28 kg/m2), and obese (BMI ≥28 kg/m2).
Postoperative hospital stay refers to the number of days following surgery during which a patient’s vital signs stabilize and symptoms significantly improve or resolve, allowing them to meet the criteria for discharge.
Data collection
All data were collected from electronic medical records, including baseline characteristics, preoperative characteristics, and postoperative data.
Blood sample collection and enzyme-linked immunosorbent assay (ELISA)
The study was conducted in accordance with the Declaration of Helsinki (as revised in 2013). The experimental protocol for the collection and use of human serum samples was approved by the Ethics Committee of The First Affiliated Hospital of Soochow University (No. 2021-274). Written informed consent was obtained from the patients or their families. Blood samples were obtained from patients diagnosed with AD before surgery and from the control group. The samples from patients with AD were stratified based on whether their BMI exceeded 24 kg/m2. The samples were centrifuged at 3,000 rpm for 20 min to extract serum, which was then stored at −80 ℃ for ELISA. The concentrations of serum complement component 5 (C5; catalog no: E-EL-H0810, Elabscience, Wuhan, China), human apolipoprotein D (apoD; catalog no: EK1223, Multi Sciences, Hangzhou, China), and polymeric immunoglobulin receptor (PIGR; catalog no: SEKH-0379, Solarbio, Beijing, China) were measured using the respective human ELISA kits according to the manufacturer’s instructions. All serum samples were analyzed by a researcher who was blinded to the patients’ clinical data.
Animals
Male C57BL/6 mice (4 weeks old) were purchased from Gempharmatech Company (Nanjing, China) and housed under a 12/12-hour light/dark cycle in a specific pathogen-free animal facility at Soochow University. All animal experiments were ethically approved by the Ethics Committee of Soochow University (No. 202207A0081), in compliance with Soochow University guidelines for the care and use of animals. A protocol was prepared before the study without registration.
The mice were randomly assigned to four groups and fed a normal diet (ND; 10% kcal fat, Research diets D12450J), high fat diet (HFD; 60% fat, Research diets D12492), HFD-human angiotensin II (AngII), and ND-AngII for 8 weeks. After this period, mice in the HFD-AngII or ND-AngII groups were anesthetized with 2% isoflurane/oxygen and implanted under the skin with osmotic minipumps (Alzet model 2004, Durect Corporation, Cupertino, CA, USA) containing human AngII (1,000 ng/min/kg, dissolved in saline, MedChemExpress, Monmouth Junction, NJ, USA) for 4 weeks (10). The mice in the ND or ND-AngII groups were fed either a ND or an HFD diet for the final 4 weeks.
At the end of the experiment, blood was collected from the orbital plexus, and the mice were euthanized. The serum was isolated by centrifugation and stored at −80 ℃. Detailed study design can be found in Appendix 1.
Measurement of mean blood pressure and echocardiography
Systolic blood pressure (SBP) and diastolic blood pressure (DBP) in conscious mice were non-invasively measured by tail-cuff plethysmography (MRBP system, IITC Life Science, Woodland Hills, CA, USA). The average SBP and DBP was calculated based on a minimum of 5 measurements per mouse.
Vascular ultrasound imaging was performed using a FUJIFILM VisualSonics Vevo 2100 System (Toronto, Canada) with an MS-400 transducer (18–38 MHz). Mice were anesthetized with 2% isoflurane/oxygen and the aortic diameter was recorded during end-diastole at various locations. AAA was defined as a 1.5-fold increase in aortic diameter compared to the ND group.
The data describes biological replicates. Biological replicates involve using different biological samples to repeat the experiment, in this study, 6–10 biological replicates were used to ensure the robustness and reproducibility of the results.
Hematoxylin and eosin (HE) staining and elastic fiber staining
The aorta was harvested, perfused with saline, and fixed with 4% paraformaldehyde. Paraffin-embedded sections (5 µm thick) were prepared for HE staining as well as elastin van Gieson (EVG) staining to observe the general morphology and elastin degradation. Images were captured using an inverted optical microscope and analyzed with ImageJ software.
Transcriptomics analysis
The total RNA of thoracic aorta tissues from three different groups (ND, n=3; HFD, n=4; and HFD-AngII, n=3) was extracted using TRIzol reagent (Invitrogen, Carlsbad, CA, USA). Subsequently, cDNA library construction and sequencing were performed using Illumina Novaseq6000 at Gene Denovo Biotechnology Co., Ltd. (Guangzhou, China).
Principal component analysis was carried out using the R package models (http://www.r-project.org/). Differentially expressed genes (DEGs) were analyzed with DESeq2 software (11) to compare two different groups, with thresholds set at an absolute fold change (FC) ≥2 and P value <0.05. Pathway enrichment analysis was then performed to identify significantly enriched metabolic or signal transduction pathways based on the Kyoto Encyclopedia of Genes and Genomes (KEGG) database in DEGs compared to the whole genome background. Finally, the intersection between upregulated and downregulated transcripts was determined to elucidate the pathophysiological changes in the mouse model.
Series test of cluster (STC) analysis for DEGs was performed to uncover the trends in gene expression and cluster the genes with the same change characteristics in a trend profile, with a threshold set at a P value of <0.05 (12).
Proteomics analysis
Sample preparation involved a series of steps including protein denaturation, reduction, alkylation, tryptic digestion, and peptide cleanup before mass spectrometry detection. Initially, approximately 100 µL of serum was extracted from mice and transferred into a lysis buffer containing 1% sodium dodecyl sulfate, urea (8 M), and a protease inhibitor cocktail (1 mg/mL). The mixture was vortexed and lysed for 30 min on ice, followed by homogenization for 2 min using an ultrasonic homogenizer. Subsequently, the homogenate was centrifuged at 12,000 rpm for 15 min at 4 ℃, and the supernatant was collected. The proteins were digested overnight at 37 ℃, and the resulting peptide mixture was labeled with the iTRAQ-8Plex Isobaric Mass Tag Labeling Kit (Thermo Fisher Scientific, MA, USA). The labeled peptides were then redissolved in 0.1% formic acid for nano-LC-MS analysis by Gene Denovo Biotechnology Co., Ltd.
Data analysis was performed using PEAKS Studio version X+ (Bioinformatics Solutions Inc., Waterloo, Canada). The PEAKS database was searched with a fragment ion mass tolerance of 0.02 Da and a parent ion tolerance of 10 ppm.
Peptides were filtered based on a 1% false discovery rate and 1 unique hit criteria. Subsequently, differentially expressed proteins (DEPs) exhibiting significant changes were identified in ND vs. HFD, ND vs. HFD-AngII, and HFD vs. HFD-AngII groups, with a FC ratio of >1.20 or <0.83 in expression and P value <0.05 serving as the cut-off criteria. Protein functions and classification were analyzed using the KEGG database. Additionally, STC analysis was performed to analyze DEPs.
The raw sequence data reported in this article have been deposited in the Genome Sequence Archive (Genomics, Proteomics & Bioinformatics 2021) and OMIX in National Genomics Data Center (Nucleic Acids Res 2022), China National Center for Bioinformation/Beijing Institute of Genomics, Chinese Academy of Sciences that are publicly accessible at https://ngdc.cncb.ac.cn (13,14).
Statistical analysis
Randomisation was performed using a computer-generated random number list to allocate subjects into different groups. The researchers conducting the study were blinded to the treatment groups during data collection and analysis. Continuous variables were presented as mean ± standard deviation or median (interquartile range), and categorical variables are presented as numbers/percentages. For continuous data, the normality of data distributions was assessed using the Shapiro-Wilk test, and equality of variances was assessed using the Brown-Forsythe test. Continuous variables were analyzed using one-way analysis of variance or the Wilcoxon rank-sum test, and categorical variables were assessed using the Chi-squared test or Fisher’s exact test. The specific statistical methods and P value could be found in the table available at https://cdn.amegroups.cn/static/public/jtd-24-1113-1.docx. Multivariable logistic regression analyses were performed to examine the relationship between BMI and in-hospital outcomes, including variables with a significance level of P<0.05 from univariate analysis and guided by expert knowledge. Clinical data were analyzed using SPSS 23.0 (IBM Corp., Armonk, NY, USA), and data from animal experiments and ELISA were analyzed using GraphPad Prism 8.0 (GraphPad Software Corp., San Diego, CA, USA). A significant level of P<0.05 was considered to indicate a statistically significant difference.
Results
Participants and characteristics
This study analyzed a total of 237 patients with aortic disease and 72 patients with valvulopathy. The mean age of patients in the aortic disease group was 55.64±14.13 years, with 83% (197 of 237) being male. In contrast, the valvulopathy group had a mean age of 58.67±11.07 years, with 75% (54 of 72) being male. Patients with aortic disease exhibited a significantly higher BMI and a lower mean age compared to those with valvulopathy (Table 1). Among the aortic disease group, 34.6% (n=82) had a BMI of less than 24 kg/m2, while 63.7% (n=151) had a BMI of 24 kg/m2 or greater. Furthermore, 56.1% of patients reported a history of hypertension, with AD present in 148 patients (62.4%), IHM in 42 patients (17.7%), and AA in 47 patients (19.8%). The baseline characteristics of the study cohort are detailed in Table S1.
Table 1
Characteristics | Aortic disease (n=237) | Valvulopathy (n=72) | t/χ2 | P |
---|---|---|---|---|
Gender (female/male) | 40/197 | 18/54 | 2.389 | 0.12 |
Age (years) | 55.64±14.13 | 58.67±11.07 | −1.896 | 0.06 |
BMI (kg/m2) | 25.60±3.92 | 23.36±3.39 | 4.326 | <0.001 |
SBP (mmHg) | 141.50±24.64 | 123.57±20.65 | 5.604 | <0.001 |
DBP (mmHg) | 77.74±16.84 | 76.13±14.72 | 0.734 | 0.46 |
Smoking | 74 (31.22) | 18 (25.00) | 1.023 | 0.19 |
Drinking | 49 (20.68) | 15 (20.83) | 1.857 | 0.11 |
Diabetes | 17 (7.17) | 7 (9.72) | 0.501 | 0.47 |
PHS (days) | 23.16±11.34 | 22.03±16.18 | 0.614 | 0.54 |
Death | 16 (6.75) | 5 (6.94) | 0.003 | 0.56 |
Data are shown as n, mean ± standard deviation, or n (%). BMI, body mass index; SBP, systolic blood pressure; DBP, diastolic blood pressure; PHS, postoperative hospital stay.
A correlation analysis was performed on the general characteristics of patients with aortic disease or valvulopathy (Figure S1) to identify significant associations between variables (P<0.05). BMI exhibited a positive correlation with aortic disease, gender, SBP, DBP, and postoperative hospitalization stays, whereas it showed a significant negative correlation with age.
BMI-related changes
After excluding four patients with missing BMI data and one patient classified as emaciated (BMI <18.5 kg/m2), the remaining 232 patients with aortic disease were categorized into different BMI groups. Significant differences were observed in the proportion of males, SBP, DBP and age among the groups (Table 2 and Table S2). In the normal BMI group, the mean age of patients was 60.07 years. As BMI increased, the age of onset tended to be younger, with the average age in the obese group being 47.98 years (Table 2). No significant differences were found in the proportion of patients with diabetes, smoking, and drinking (Table S2). Variables with significant differences, included albumin, triglyceride, high-density lipoprotein cholesterol (HDL-C), uric acid, hemoglobin, brain natriuretic peptide and the proportion of leukocytes, neutrophil and monocyte, as detailed in Table 2. Other variables are presented in Table S2.
Table 2
Characteristics | Normal BMI (n=82) | Overweight (n=90) | Obesity (n=60) | χ2/F/H | P |
---|---|---|---|---|---|
Age (years) | 60.07±13.27 | 56.18±12.89 | 47.98±13.09 | 15.045 | <0.001 |
SBP (mmHg) | 133.55±25.04 | 143.97±20.93* | 149.03±26.31* | 8.006 | <0.001 |
DBP (mmHg) | 73.22±17.08 | 79.66±13.76* | 81.40±19.83* | 5.047 | 0.007 |
Gender (female/male) | 24/58 | 8/82 | 7/53 | 14.274 | 0.001 |
PHS (days) | 18.500 (13.0, 27.0) | 22.000 (16.0, 30.0) | 24.000 (18.0, 30.3)* | 8.097 | 0.01 |
ALB (g/L) | 37.550 (34.6, 40.4) | 38.900 (35.6, 41.4) | 39.400 (36.6, 42.9)* | 6.304 | 0.043 |
TG (mmol/L) | 0.920 (0.7, 1.3) | 1.215 (0.9, 1.6)* | 1.195 (1.0, 1.9)* | 19.138 | <0.001 |
HDL-C (mmol/L) | 1.100 (0.9, 1.3) | 0.975 (0.8, 1.1)* | 0.880 (0.8, 1.0)* | 16.411 | <0.001 |
UA (mmol/L) | 324.100 (280.6, 405.8) | 390.900 (318.4, 480.0)* | 424.950 (332.2, 483.1)* | 16.95 | <0.001 |
Hb (g/L) | 122.000 (112.8, 136.0) | 131.000 (116.8, 138.0)* | 136.000 (124.0, 148.0)*,** | 16.963 | <0.001 |
WBC (109/L) | 8.395 (6.0, 12.2) | 10.765 (7.9, 13.0)* | 10.795 (8.4, 15.4)* | 11.243 | 0.004 |
NE (109/L) | 6.850 (4.0, 10.8) | 9.425 (5.8, 11.6)* | 9.335 (6.5, 13.5)* | 9.453 | 0.009 |
MO (109/L) | 0.445 (0.3, 0.6) | 0.620 (0.4, 0.9) | 0.580 (0.4, 0.8)* | 8.281 | 0.01 |
BNP (pg/mL) | 219.700 (124.5, 444.9) | 176.800 (75.1, 426.7)* | 115.400 (55.2, 390.5)* | 6.895 | 0.03 |
Data are shown as n, mean ± standard deviation or median (Q1, Q3). Normal weight (BMI <24 kg/m2), overweight (BMI 24 to <28 kg/m2), and obesity (BMI ≥28 kg/m2). F means using Fisher exact test. H means using Kruskal-Wallis H test. *, represents a significant difference (P<0.05) comparing to the normal BMI group; **, represents a significant difference (P<0.05) comparing to the overweight group. BMI, body mass index; SBP, systolic blood pressure; DBP, diastolic blood pressure; PHS, postoperative hospital stay; ALB, albumin; TG, triglyceride; HDL-C, high-density lipoprotein cholesterol; UA, uric acid; Hb, hemoglobin; WBC, leukocytes (white blood cell); NE, neutrophil; MO, monocyte; BNP, brain natriuretic peptide.
Effects of obesity-hypertension on behaviors and vascular morphology of mice
Clinical data indicated that 66.8% of patients with aortic disease were overweight or obese, a factor strongly linked to poor clinical outcomes. To investigate the direct impact of obesity on aortic disease, we established three mouse models: one for obesity, one for obesity-related hypertension, and one for hypertension alone. At the end of the experiment, the weight, blood pressure, serum lipid profiles, and aortic diameters of mice were measured.
Mice in the HFD or HFD-AngII groups exhibited a significant increase in body weight compared to the ND group (Figure 1A). Treatment with AngII did not lead to noticeable changes in food intake or body weight. Additionally, HFD contributed to elevated blood pressure levels in the mice, with SBP and DBP slightly higher in model mice than the control ND-fed mice (Figure 1B,1C). Blood pressure increased more in the HFD-AngII group than in the HFD alone group. Moreover, subcutaneous infusion of AngII significantly increased the SBP and DBP.
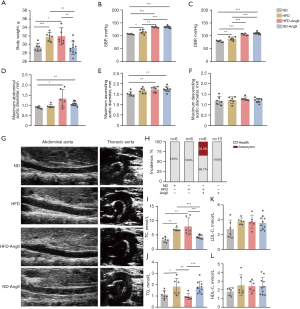
The AngII infusion model is commonly used for studying AA (15). To investigate the effects of obesity on aortic injury, echocardiography was performed to measure the diameter of the thoracic and abdominal aorta. Subsequently, the aortas were isolated and fixed for HE and EVG staining to assess aortic elastic fiber tears and dilation. After 4 weeks of AngII treatment, there was an increase in the maximal diameters of the ascending and abdominal aorta (Figure 1D,1E) and not significant difference in the maximal diameters of the descending aorta (Figure 1F). AAAs only developed under HFD-AngII treatment (Figure 1G,1H).
The serum lipid profiles of mice were significantly influenced by HFD. In comparison to the ND group, TC levels were notably elevated in both the HFD and HFD-AngII groups (Figure 1I). Conversely, the TG level decreased in the HFD-AngII group compared to the HFD and ND-AngII groups (Figure 1J). Interestingly, there were no significant differences in low-density lipoprotein cholesterol (LDL-C) and HDL-C levels among all groups (Figure 1K,1L).
Representative macroscopic histological images of the aorta demonstrated the induction of AAAs by HFD-AngII treatment (Figure 2A). HE staining of the abdominal aorta revealed thickening of the aortic media and infiltration of inflammatory cells in the arterial adventitia. EVG staining showed disruption of the elastic lamina (Figure 2B,2C).
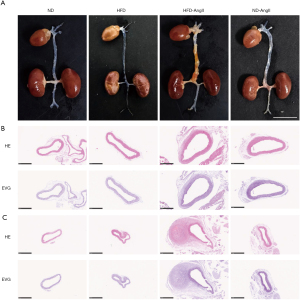
Identification of DEGs in obesity-hypertension mice
A non-target global gene expression analysis was conducted to investigate the impact of obesity and hypertension on aortic tissue messenger RNA (mRNA) expression in mouse models. Heatmap analysis based on normalized Z score was performed to assess the clustering patterns among samples (Figure S2A,S2B). DEGs were identified, and principal component analysis demonstrated distinct variations among the three experimental model groups (Figure S3A). Genes meeting the criteria of log2|FC| >1 and P<0.05 were considered significantly differentially expressed between groups, with detailed information provided in Figure S3B.
A progressive trend toward compounded injury due to obesity and hypertension was observed across the ND, HFD, and HFD-AngII groups. Trend analysis (STC) was employed to identify profiles in gene abundance across gradient-based samples, revealing eight distinct trends in gene expression (Figure 3A).
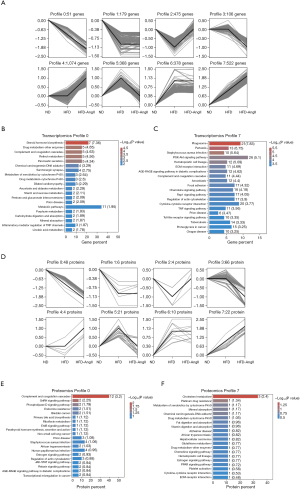
Various genes were included in these significant profiles. Genes exhibiting continuous increase or decrease in profile 0 (51 genes) or profile 7 (522 genes) were further analyzed for functional enrichment using KEGG to elucidate their properties and potential biological relevance. Notably, two common pathways related to complement and coagulation cascades and prion disease were among the top 20 enrichment pathways (Figure 3B,3C and Table S3).
Proteomics analysis
To investigate differences in serum protein expression in response to obesity or hypertension, we adopted a Data-Independent Acquisition Mass Spectrometry (DIA-MS)-based quantitative proteomics approach to analyze changes in ND, HFD, or HFD-AngII groups. A total of 611 quantified proteins were identified across all three groups for differential abundance analysis. Applying a screening threshold for DEPs, we found 123 DEPs (53 upregulated and 70 downregulated) in HFD and 171 DEPs (51 upregulated and 120 downregulated) in HFD-AngII compared to the ND group. Additionally, 69 DEPs (54 upregulated and 15 downregulated) were identified in HFD-AngII compared to HFD (Figure S3C).
Trend analysis revealed eight distinct patterns in protein abundance (Figure 3D). Further analysis focused on proteins in profile 0 (48 proteins) and profile 7 (22 proteins) for functional enrichment using KEGG. Notably, two common pathways i.e., African trypanosomiasis and estrogen signaling pathway, were among the top 20 enrichment pathways (Figure 3E,3F and Table S4).
Integrated KEGG pathway enrichment analysis of aortic DEGs and serum DEPs
To investigate the molecular markers in the aortic tissue and serum of mice, we analyzed the top 20 enrichment pathways from transcriptomics profile 0 and proteomics profile 0, revealing 16 common DEGs/DEPs involved in complement and coagulation cascade signaling. These included genes such as C5, C6, C8a, and F7 (Table 3). Subsequent integrated analysis of transcriptomics profile 7 and proteomics profile 7 identified 4 shared enrichment KEGG pathways, such as hematopoietic cell lineage and chemokine signaling pathway (Table 4). Further examination of the intersection of DEGs and DEPs in the aorta and serum led to the identification of 4 common genes with similar expression trends: C5, PIGR, apoD, and RIKEN cDNA 2610524H06 gene (env) (Figure 4A).
Table 3
Pathway | Transcriptomics profile 0 | Proteomics profile 0 |
---|---|---|
Complement and coagulation cascades | Fgg; C9; C4bpa; C5; F13b | Itgam; C1sa; Kng1; C8a; Cfi; Serpinc1; Serpina1e; F7; C5; Vwf; C6; A2m |
Prion disease | C9; C5 | C8a; C5; C6 |
Table 4
Pathway | Transcriptomics profile 7 | Proteomics profile 7 |
---|---|---|
Hematopoietic cell lineage | Pik3cd; Arrb2; Ncf1; Gng4; Prkcb; Pak1; Cxcl1; Cx3cl1; Shc3; Vav2; Cxcr3; Ccr1; Ccl12; Ccl2; Ccl7; Cxcr6 | Gp5 |
ECM-receptor interaction | Cd44; Col2a1; Spp1; Fn1; Col1a1; Col1a2; Itga2; Thbs2; Comp; Sv2a; Itga11 | Gp5 |
Chemokine signaling pathway | Pik3cd; Arrb2; Ncf1; Gng4; Prkcb; Pak1; Cxcl1; Cx3cl1; Shc3; Vav2; Cxcr3; Ccr1; Ccl12; Ccl2; Ccl7; Cxcr6 | Pf4 |
Cytokine-cytokine receptor interaction | Csf1r; Lif; Il18r1; Il6; Tnfsf13b; Il7r; Gdf3; Cxcl1; Cx3cl1; Tnfsf8; Cxcr3; Ccr1; Csf2ra; Ccl12; Ccl2; Tnfrsf1b; Ccl7; Cxcr6; Ngfr; Gdf10 | Pf4 |
ECM, extracellular matrix.
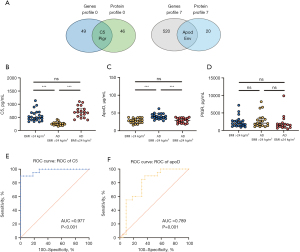
Validation of the candidate marker proteins in serum
To evaluate the clinical relevance of common DEPs, C5, PIGR, and apoD expression levels were examined in serum samples from individuals with AD and healthy controls using ELISA. Env, a group of predictive genes, has not yet been studied in humans. AD patients were divided based on BMI over or under 24 kg/m2. Serum C5 levels were significantly lower in AD patients with BMI >24 kg/m2 compared to those with BMI ≤24 kg/m2 and healthy controls with BMI >24 kg/m2. No significant difference was observed between healthy controls with BMI >24 kg/m2 and AD patients with BMI ≤24 kg/m2 (Figure 4B). Conversely, apoD levels were higher in AD patients with BMI >24 kg/m2 compared to those with BMI ≤24 kg/m2 and healthy controls with BMI >24 kg/m2. There was no significant difference between healthy controls with BMI >24 kg/m2 and AD patients with BMI ≤24 kg/m2 (Figure 4C). PIGR levels did not exhibit significant differences among the groups (Figure 4D). Receiver operating characteristic (ROC) curve analysis indicated that C5 and apoD could predict AD in patients with a BMI over 24 kg/m2 (Figure 4E,4F). The optimal cut-off for C5 was 315, with a sensitivity of 90% and specificity of 100% [area under the curve (AUC) =0.977; 95% confidence interval (CI): 0.941–1.000; P<0.001] (Table 5). ApoD had a cut-off of 31.4, with a sensitivity of 90% and specificity of 63.6% (AUC =0.789; 95% CI: 0.646–0.931; P=0.001) (Table 5). Both C5 and apoD serum levels displayed promising clinical predictive value and potential applications.
Table 5
Variables | AUC (95% CI) | Sensitivity (%) | Specificity (%) | Cut-off |
---|---|---|---|---|
C5 | 0.977 (0.941–1.000) | 90 | 100 | 315 |
apoD | 0.789 (0.646–0.931) | 90 | 63.6 | 31.4 |
C5, component 5; apoD, apolipoprotein D; AUC, area under the curve; CI, confidence interval.
Discussion
In this study, we found that 64.9% of patients diagnosed with aortic disease were overweight or obese, and 62.4% had hypertension. Among those with hypertension, 79.4% had a BMI ≥24 kg/m2. It was noted that a higher BMI was associated with an earlier onset of aortic disease, particularly AD. Additionally, BMI had a significant positive correlation with blood pressure, length of hospital stay, and mortality rate, indicating that obesity increases the risk of aortic disease. Currently, there are no effective drug therapies available for preventing the progression of AA or AD.
Obesity is a known risk factor for various aortic diseases (16), including aortic valve stenosis (17), acute AD (18), and AA (19). The vasculopathy associated with obesity is believed to be primarily due to excessive lipid deposition, leading to macrophage infiltration of the adipose tissue, increased expression of pro-inflammatory cytokines, and impaired sphingolipid metabolism, which are all implicated in the development of AAs (2,20). Additionally, obesity contributes to high blood pressure through mechanisms such as physical compression of the kidneys by surrounding fat, activation of the renin-angiotensin-aldosterone system, and increased sympathetic nervous system activity (21). Both obesity and hypertension independently increase the risk of AAs and ADs, with obesity placing mechanical stress on the aortic wall and hypertension further escalating this stress by raising internal pressure (22). The coexistence of adiposity and hypertension can synergistically worsen aortic conditions, as the combined impact of inflammation, endothelial dysfunction, and hemodynamic stress can hasten disease progression.
To investigate the impact of obesity and obesity-related hypertension on aortic disease, three mouse models were established: HFD, HFD-AngII, and ND-AngII groups. The results revealed that obesity induced by HFD feeding did not lead to aortic dilation or aneurysm, despite raising SBP and DBP. Aortic dilatation was observed in the ascending and abdominal aorta of the ND-AngII group and HFD-AngII group mice, with 33.3% of AAAs specifically occurring in the HFD-AngII group. The AngII mouse model is commonly used to assess AA or AD incidence, severity, and related mortality. Most AngII infusion studies utilize hyperlipidemic mice, such as LDLR−/−, ApoE−/− mice, or those fed an HFD. The incidence of AAAs is reported to be lower in C57BL/6 mice than in hyperlipidemic mice. C57BL/6 mice co-infused with AngII and BAPN have a similar incidence rate to AngII-infused ApoE−/− mice (23). While hyperlipidemia is not a prerequisite for AngII-induced AAA development, its presence does increase the likelihood. A meta-analysis suggests that a lower dose of AngII (750 ng/min/kg) can reduce AAA incidence and mortality, whereas the dose increase plays a less significant role, even at high levels (2,000 ng/min/kg) (24). Overall, the combined effect of obesity and hypertension appears to be crucial for the development of AAs or ADs.
The combined mechanism of obesity and hypertension in aortic disease remains unclear. In this study, we first described the trends seen among DEGs and DEPs in aortic transcriptomic and serum proteomic data from ND, HFD, and HFD-AngII groups through STC analysis. To further investigate the functions of these DEGs or DEPs, KEGG enrichment was performed in profiles 0 and 7. The results revealed that obesity and hypertension impact common KEGG pathways, such as complement and coagulation cascades, and prion disease in transcriptomics profiles 0 and 7, as well as African trypanosomiasis and the estrogen signaling pathway in protein profiles 0 and 7. An integrated analysis of transcriptomic and protein profiles identified C5 and apoD as potential biomarkers for the adverse effects of obesity, which were subsequently validated in human serum through ELISA.
ApoD, an atypical apolipoprotein from the lipocalin superfamily, is widely distributed in the body with high expression in the kidney, spleen, and lung tissues (25). In plasma, apoD is primarily found in HDL particles, with a smaller portion bound to low-density lipoproteins (LDLs) and very low-density lipoproteins (VLDLs) (25). Functioning as a multi-ligand, multi-functional protein, apoD plays roles in lipid trafficking, food intake, inflammation, and antioxidative response. Genetic variations in apoD have been linked to abnormal lipid metabolism and an increased susceptibility to cardiovascular diseases (26). Increased apoD deposition is observed in atherosclerotic lesions of both humans with cardiovascular disease and mice with premature atherosclerosis. However, apoD has not been identified in AA or AD. Our study found that apoD levels were higher in patients with AD (BMI >24 kg/m2) compared to those with AD (BMI ≤24 kg/m2) and healthy individuals with BMI >24 kg/m2. ApoD showed promising clinical predictive value in individuals with AD who were overweight. Elevated apoD levels are associated with improved metabolic health and reduced inflammation in the round ligament of morbidly obese women (27). Mice overexpressing apoD in the liver and fed HFD showed increased anti-inflammatory lipid mediators and reduced inflammation markers such as tumor necrosis factor-α and interleukin-6 in the blood, liver, and adipose tissues (28). Given that apoD is involved in various pathologies and our understanding of the underlying mechanisms is limited, exploring the therapeutic potential of apoD in aortic diseases is warranted.
The complement system is a crucial component of the innate immune response that plays a key role in defending the body and maintaining tissue balance. Dysregulation of the complement system is linked to various rare diseases such as paroxysmal nocturnal hemoglobinuria and atypical hemolytic uremic syndrome, as well as common conditions such as rheumatic diseases, ischemia-reperfusion injuries, and atherosclerosis (29). More and more diseases are being known to be caused by complement dysregulation. In an observational case-control study, serum C5a levels were significantly higher in patients with AAA than in healthy individuals and were associated with an increase in the maximum diameter of AAAs (30). This study is the first to identify C5 as a distinguishing risk factor in samples with overlapping obesity and obesity-related hypertension. The results showed a significant decrease in C5 concentration in AD patients with a BMI >24 kg/m2 compared to healthy individuals with a BMI >24 kg/m2. Serum C5 was found to have a high predictive value for AD patients with a BMI greater than 24 kg/m2, highlighting its importance as a biological marker for predicting the development of AD in overweight individuals.
Limitations of this study include the difference between humans and mice, as well as the fact that the HFD-AngII mouse model induced aortic dilatation and AAA but not AD. Currently, there is a lack of established models for AD directly linked to obesity and hypertension. Additionally, apoD, a protective lipocalin protein, is found to be elevated in AD patients with overweight, with the underlying mechanism requiring further elucidation. Both C5 and apoD serum levels show promise as clinical predictors of AD, but their downstream targets and functions in AD development are currently only predicted through bioinformatics analysis. Further biological experiments are necessary to validate their precise roles.
Conclusions
This comprehensive analysis of 237 individuals provides evidence that a higher BMI is associated with an increased risk of aortic disease, especially AD. Results from cluster analysis of transcriptomics and proteomics data revealed a trend in gene and protein expression in a mouse model with overlapping factors of obesity and hypertension. Bioinformatics analysis highlighted the involvement of the complement and coagulation cascades signaling pathway. ApoD and C5 levels were identified as potential biomarkers for assessing the risk of aortic disease in obese individuals.
Acknowledgments
Funding: The research was funded by
Footnote
Reporting Checklist: The authors have completed the MDAR and ARRIVE reporting checklists. Available at https://jtd.amegroups.com/article/view/10.21037/jtd-24-1113/rc
Data Sharing Statement: Available at https://jtd.amegroups.com/article/view/10.21037/jtd-24-1113/dss
Peer Review File: Available at https://jtd.amegroups.com/article/view/10.21037/jtd-24-1113/prf
Conflicts of Interest: All authors have completed the ICMJE uniform disclosure form (available at https://jtd.amegroups.com/article/view/10.21037/jtd-24-1113/coif). The authors have no conflicts of interest to declare.
Ethical Statement: The authors are accountable for all aspects of the work in ensuring that questions related to the accuracy or integrity of any part of the work are appropriately investigated and resolved. The experimental protocol for the collection and use of human serum samples was approved by the Ethics Committee of The First Affiliated Hospital of Soochow University (No. 2021-274). The study was conducted in accordance with the Declaration of Helsinki (as revised in 2013). Written informed consent was obtained from the patients or their families. All animal experiments were ethically approved by the Ethics Committee of Soochow University (No. 202207A0081), in compliance with Soochow University guidelines for the care and use of animals.
Open Access Statement: This is an Open Access article distributed in accordance with the Creative Commons Attribution-NonCommercial-NoDerivs 4.0 International License (CC BY-NC-ND 4.0), which permits the non-commercial replication and distribution of the article with the strict proviso that no changes or edits are made and the original work is properly cited (including links to both the formal publication through the relevant DOI and the license). See: https://creativecommons.org/licenses/by-nc-nd/4.0/.
References
- GBD 2015 Obesity Collaborators; Afshin A, Forouzanfar MH, et al. Health Effects of Overweight and Obesity in 195 Countries over 25 Years. N Engl J Med 2017;377:13-27.
- Eckstein HH, Maegdefessel L. Linking obesity with abdominal aortic aneurysm development. Eur Heart J 2020;41:2469-71. [Crossref] [PubMed]
- Shimizu T, Kimura N, Mieno M, et al. Effects of Obesity on Outcomes of Acute Type A Aortic Dissection Repair in Japan. Circ Rep 2020;2:639-47. [Crossref] [PubMed]
- Pan X, Xing Z, Yang G, et al. Obesity Increases In-Hospital Mortality of Acute Type A Aortic Dissection Patients Undergoing Open Surgical Repair: A Retrospective Study in the Chinese Population. Front Cardiovasc Med 2022;9:899050. [Crossref] [PubMed]
- Wortmann M, Peters AS, Erhart P, et al. Inflammasomes in the Pathophysiology of Aortic Disease. Cells 2021;10:2433. [Crossref] [PubMed]
- Hibino M, Otaki Y, Kobeissi E, et al. Blood Pressure, Hypertension, and the Risk of Aortic Dissection Incidence and Mortality: Results From the J-SCH Study, the UK Biobank Study, and a Meta-Analysis of Cohort Studies. Circulation 2022;145:633-44. [Crossref] [PubMed]
- Jiang S, Liu G, Yuan H, et al. Changes on proteomic and metabolomic profile in serum of mice induced by chronic exposure to tramadol. Sci Rep 2021;11:1454. [Crossref] [PubMed]
- Pan XF, Wang L, Pan A. Epidemiology and determinants of obesity in China. Lancet Diabetes Endocrinol 2021;9:373-92. [Crossref] [PubMed]
- Zeng Q, Li N, Pan XF, et al. Clinical management and treatment of obesity in China. Lancet Diabetes Endocrinol 2021;9:393-405. Erratum in: Lancet Diabetes Endocrinol 2021;9:e2. [Crossref] [PubMed]
- Piek A, Koonen DPY, Schouten EM, et al. Pharmacological myeloperoxidase (MPO) inhibition in an obese/hypertensive mouse model attenuates obesity and liver damage, but not cardiac remodeling. Sci Rep 2019;9:18765. [Crossref] [PubMed]
- Love MI, Huber W, Anders S. Moderated estimation of fold change and dispersion for RNA-seq data with DESeq2. Genome Biol 2014;15:550. [Crossref] [PubMed]
- Luan S, Li P, Yi T. Series test of cluster and network analysis for lupus nephritis, before and after IFN-K-immunosuppressive therapy. Nephrology (Carlton) 2018;23:997-1006. [Crossref] [PubMed]
- Chen T, Chen X, Zhang S, et al. The Genome Sequence Archive Family: Toward Explosive Data Growth and Diverse Data Types. Genomics Proteomics Bioinformatics 2021;19:578-83. [Crossref] [PubMed]
- Database Resources of the National Genomics Data Center. China National Center for Bioinformation in 2022. Nucleic Acids Res 2022;50:D27-38. [Crossref] [PubMed]
- Tedjawirja VN, de Waard V. Which Mouse Model of Abdominal Aortic Aneurysm Deserves Triple A Status? Eur J Vasc Endovasc Surg 2019;58:777-8. [Crossref] [PubMed]
- Powell-Wiley TM, Poirier P, Burke LE, et al. Obesity and Cardiovascular Disease: A Scientific Statement From the American Heart Association. Circulation 2021;143:e984-1010. [Crossref] [PubMed]
- Kaltoft M, Langsted A, Nordestgaard BG. Obesity as a Causal Risk Factor for Aortic Valve Stenosis. J Am Coll Cardiol 2020;75:163-76. [Crossref] [PubMed]
- Liu Y, Zhang B, Liang S, et al. Impact of body mass index on early and mid-term outcomes after surgery for acute Stanford type A aortic dissection. J Cardiothorac Surg 2021;16:179. [Crossref] [PubMed]
- Stoll S, Sowah SA, Fink MA, et al. Changes in aortic diameter induced by weight loss: The HELENA trial- whole-body MR imaging in a dietary intervention trial. Front Physiol 2022;13:976949. [Crossref] [PubMed]
- Okrzeja J, Karwowska A, Błachnio-Zabielska A. The Role of Obesity, Inflammation and Sphingolipids in the Development of an Abdominal Aortic Aneurysm. Nutrients 2022;14:2438. [Crossref] [PubMed]
- Hall JE, do Carmo JM, da Silva AA, et al. Obesity-induced hypertension: interaction of neurohumoral and renal mechanisms. Circ Res 2015;116:991-1006. [Crossref] [PubMed]
- Humphrey JD. Mechanisms of Vascular Remodeling in Hypertension. Am J Hypertens 2021;34:432-41. [Crossref] [PubMed]
- Daugherty A, Cassis LA. Mouse models of abdominal aortic aneurysms. Arterioscler Thromb Vasc Biol 2004;24:429-34. [Crossref] [PubMed]
- Trachet B, Fraga-Silva RA, Jacquet PA, et al. Incidence, severity, mortality, and confounding factors for dissecting AAA detection in angiotensin II-infused mice: a meta-analysis. Cardiovasc Res 2015;108:159-70. [Crossref] [PubMed]
- Rassart E, Desmarais F, Najyb O, et al. Apolipoprotein D. Gene 2020;756:144874. [Crossref] [PubMed]
- Yang HH, Wang X, Li S, et al. Lipocalin family proteins and their diverse roles in cardiovascular disease. Pharmacol Ther 2023;244:108385. [Crossref] [PubMed]
- Fyfe-Desmarais G, Desmarais F, Rassart É, et al. Apolipoprotein D in Oxidative Stress and Inflammation. Antioxidants (Basel) 2023;12:1027. [Crossref] [PubMed]
- Kurano M, Tsukamoto K, Kamitsuji S, et al. Apolipoprotein D modulates lipid mediators and osteopontin in an anti-inflammatory direction. Inflamm Res 2023;72:263-80. [Crossref] [PubMed]
- West EE, Woodruff T, Fremeaux-Bacchi V, et al. Complement in human disease: approved and up-and-coming therapeutics. Lancet 2024;403:392-405. [Crossref] [PubMed]
- Bhak RH, Wininger M, Johnson GR, et al. Factors associated with small abdominal aortic aneurysm expansion rate. JAMA Surg 2015;150:44-50. [Crossref] [PubMed]