High-resolution computed tomography with 1,024-matrix for artificial intelligence-based computer-aided diagnosis in the evaluation of pulmonary nodules
Highlight box
Key findings
• 1,024-matrix high-resolution computed tomography (CT) scans outperform 512-matrix in computer-aided diagnosis.
What is known and what is new?
• Lung cancer is the leading cause of cancer-related deaths worldwide, and chest CT plays an important role in the diagnosis of lung nodules and early screening of lung cancer.
• The 1,024 matrix improves image quality, but may also decrease diagnostic efficiency by increasing the radiologist’s reading time due to additional image detail.
• Artificial intelligence (AI) has achieved remarkable results in lung nodule assessment. However, there are not many studies on AI assessment of lung nodules in 1,024 matrix scans.
What is the implication, and what should change now?
• The 1,024-matrix scanning scheme performed significantly better than the 512-matrix in terms of subjective image quality and lung nodule AI detection rate for all CT images. The advantages of the 1,024 matrix scanning program in computer-aided diagnosis are demonstrated, adding evidence and confidence to its widespread use.
Introduction
Lung cancer, the leading cause of cancer-related deaths worldwide, continues to have a grim overall prognosis (1,2). Early detection of lung cancer can greatly improve the survival chances of patients; therefore, early diagnosis of lung cancer plays a pivotal role and has irreplaceable value in clinical practice (3). In particular, as a common manifestation of early lung cancer, the increase in the detection rate of lung nodules has attracted widespread attention from the medical community (4). Pathologic puncture biopsy is a reliable method for preoperative diagnosis of lung nodules. However, on the one hand, the pathological features of pulmonary nodules usually show diversity and complexity, posing a diagnostic challenge to clinicians (5-7). On the other hand, it suffers from the problem of invasive sampling, which may lead to complications such as pulmonary atrophy and pneumothorax, limiting its use in clinical practice (8,9). Computed tomography (CT) examination, as a non-invasive examination method, is able to obtain a large amount of information in a shorter period of time while at the same time reducing the patient’s pain and recovery time, providing timely diagnostic results for doctors. Therefore, CT has an important role in the diagnosis of lung nodules and early screening of lung cancer.
Image quality plays an important role in the diagnosis of lung nodules. Various factors, including matrix, layer thickness, tube voltage, and tube current, affect medical image quality and diagnostic accuracy (10). Among them, high-resolution chest CT (HRCT) with 1,024×1,024 matrix scanning has proven to be a key technology, showing significant advantages over existing chest imaging modalities (11-13). Studies have shown that 1,024-matrix can significantly improve image quality and provide more detailed information (12). While better image quality may improve the precision of lung nodule assessment, it may also increase reading time for radiologists due to additional image details, which may reduce diagnostic efficiency, and bring new challenges to the application of HRCT in clinical work.
The utilization of artificial intelligence-based computer-aided diagnosis (AI-CAD) in the medical field has revolutionized the progress of medical technology (14,15). It has a wide range of applications, including CT imaging and post-processing. More importantly, Artificial intelligence (AI) technology assists radiologists in multiple aspects of lesion detection and diagnosis (16,17). So far, AI has achieved remarkable results in the detection, classification, as well as benign and malignant diagnosis of pulmonary nodule evaluation, thus improving the accuracy and efficiency of diagnosis (18-20). However, there are not many studies related to AI-CAD assessment of pulmonary nodules in clinical 1,024 matrix scans.
This study aimed to investigate the effectiveness of 1,024 and 512 matrix for an AI-CAD in the detection of lung nodules. We present this article in accordance with the STARD reporting checklist (available at https://jtd.amegroups.com/article/view/10.21037/jtd-24-1311/rc).
Methods
Patients
The study was conducted in accordance with the Declaration of Helsinki (as revised in 2013). The study was approved by the Ethics Committee of Shanghai Changzheng Hospital (No. 2022SL070). Due to the retrospective nature of the analysis and the anonymity of the data, the requirement for patients’ informed consent was waived. The other center was informed and agreed with the study. 344 patients from Changzheng Hospital (Center 1) and Beijing Water Resources Hospital (Center 2) between January 2020 and November 2023 were included in this retrospective analysis. The study was conducted based on the following criteria. Our inclusion criteria were: (I) patients who have undergone a 1,024×1,024 matrix chest CT examination with both 1,024-matrix and 512-matrix reconstructed images; (II) patients with at least one pulmonary nodule possessing an average diameter ≤30 mm; (III) complete and clear CT images. The exclusion criteria were: (I) patients with underlying chronic lung disease (e.g., interstitial lung diseases, emphysema, or pneumonia), or difficulty in clearly depicting the border of pulmonary nodules due to collapsed or obstructive changes in the lung parenchyma surrounding the nodule; (II) severe artifacts in the image due to misalignment, respiratory motion, etc.; and (III) incomplete images in Digital Imaging and Communications in Medicine (DICOM) format. The specific flow chart of the study is shown in Figure 1.
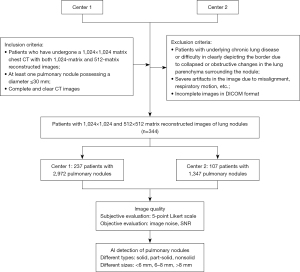
Image acquisition
All patients were scanned in supine position at the end of normal inspiration. All chest CT examinations used in hospital 1 were performed on a Philips iCT 256 (Center 1, n=237) and all chest CT examinations in hospital 2 (Center 2, n=107) were performed on a Philips Incisive CT. The parameters were as follows: layer thickness 1mm, scan matrix 1,024×1,024, tube voltage 120 kV, tube current modulation, gantry rotation 0.5 seconds per rotation. And iDose reconstruction algorithm was used for both schemes. Images of patients were reconstructed from the raw data according to 2 different protocols: 512×512 matrix and 1,024×1,024 matrix. The scan range covered from the lung apex to the lower margin of the posterior costophrenic angle.
Image quality analysis
Objective evaluation
Set 1,024-matrix images and 512-matrix images to linked status, and a circular region of interest (ROI) was placed in the air density of each patient’s chest CT examination at the same location of the two protocol images, avoiding the airways and blood vessels, as outlined in detail in the Figure S1. A total of three ROIs were outlined, and the average of the measured values at the three locations was used as the final value. Image noise, the average CT value, and the signal-to-noise ratio (SNR) were recorded. The image noise was defined as the standard deviation (SD) of the CT value, and the SNR was calculated as follows: SNR = CT/SD.
Subjective evaluation
Two radiologists (with 3, and 5 years of experience in CT diagnosis of cardiothoracic diseases, respectively) independently assessed the 1,024-matrix and 512-matrix images. Before the image quality assessment, two radiologists were trained in image quality assessment of 20 randomly selected patients who were not included in the study and reached a consensus on rating scales. The 512×512 matrix and 1,024×1,024 matrix images of each patient were displayed in a randomized order, side by side, on an 8.3-megapixel liquid crystal display (4K resolution). Images were displayed using the lung window setting [window width: 1,600 Hounsfield unit (HU); window level: −600 HU]. Two radiologists with different seniority evaluated the 512-matrix, and 1024-matrix images for noise, streak artifacts, pulmonary nodule edges, clarity of small blood vessels, homogeneity of normal lung parenchyma, and overall image quality. The results of subjective evaluations are rated on a 5-point Likert scale (1—bad, 2—medium to bad, 3—medium, 4—good, and 5—very good image quality). Record the average of the results. Figure 2 shows the images and lesions displayed in 512-matrix and 1,024-matrix images for patients.
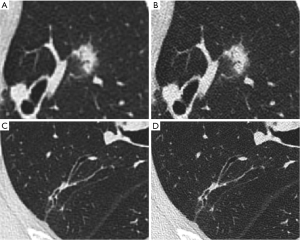
Lung nodule detection
Lung nodule detection and classification were automatically conducted on a dedicated AI-CAD (LungDoc, Beijing, China). The core design of the AI-CAD was the deep learning model, which was divided into three primary components: feature pyramid network (FPN), UNet and ResNet. The modified FPN was employed to simultaneously detect lung and lung nodules present in processed CT scans that were normalized and resampled into the same size. UNet was responsible for lung and nodule segmentation, while ResNet classified nodule types.
Due to the size difference between lung nodules, the modified FPN was meticulously dedicated and optimized for the detection of tiny lung nodules. Small targets occupy fewer pixels in feature maps with high resolution. Additionally, their information may disappear after multiple convolutions and pooling layers. Therefore, we proposed a multi-level feature fusion strategy to merge target details, such as location, from high-resolution feature maps and semantic information from low-resolution feature maps, which utilizes various levels of features thoroughly and enhances detection accuracy. Since lung nodules are sparsely located in CT images, an attention mechanism (21) was introduced to assist the model in focusing on regions that potentially harbor nodules, thereby reducing omissions. Then, UNet was implemented to segment nodules in regions that were detected through the modified FPN. Learning features from both input regions and patches extracted, Coarse2Fine (22) was used to improve segmentation accuracy. Based on segmented masks, we calculated the size of pulmonary nodules. Together with the original CT scans, masks were input into a ResNet to classify the type of nodules.
The number, size, and type of each pulmonary nodule detected were recorded for further analysis. For nodule size, the Lung-RADS guideline of 2022 (23) shows that nodule diameters of 6 and 8 mm have a significant impact on the management strategy for lung nodules. For nodules less than 6 mm, the primary follow-up strategy was to take no further intervention or treatment; 8 mm was the demarcation criterion between categories 3 and 4 for solid nodules, and those >8 mm were classified as category 4 nodules and were followed up at 3-month intervals rather than the 6-month follow-up interval for category 3 management. Two experienced radiologists independently reevaluated all subjects to confirm the presence of any misdiagnosed or missed nodules. The total detection rate of AI-CAD on lung nodules was calculated, and its detection rate in different types and sizes of lung nodules was counted separately. The accuracy, precision and recall of AI-CAD in the detection of lung nodules were counted using the manual judgment results of two radiologists as the standard. In our study, accuracy measures the overall correctness of the classification model by dividing the sum of true positives (nodules) and true negatives (non-nodules) by the total number of predictions. Precision assesses the accuracy of positive predictions, expressed as the ratio of true positives to all predicted positives. Recall, or sensitivity, evaluates the model’s ability to identify all actual positive nodules, calculated as the ratio of true positives to the total number of actual positive instances.
Statistical methods
All statistical analyses were performed using SPSS (IBM SPSS, version 26.0). Spearman rank correlation analysis was used to assess associations between two radiologists’ subjective ratings. Six evaluation indexes of image noise, streak artifacts, nodule edges, small vessel definition, homogeneity of normal lung parenchyma and overall image quality were compared between 1,024×1,024 matrix and 512×512 matrix by using t-test. Statistically significant at P<0.05.
Results
Image quality
A total of 344 patients with 4,319 nodules were included in this study. The overall characteristics of the nodules are detailed in Table S1. Spearman’s correlation coefficients at the 512×512 and 1,024×1,024 matrix were 0.838 and 0.828, respectively. The image quality evaluation scores for the two scanning matrices are recorded in Table 1. In subjective evaluation, the 1,024×1,024 matrix achieved superior performance at pulmonary nodule edges, clarity of small blood vessels, homogeneity of normal lung parenchyma, and overall image quality. And performed lower than the 512×512 matrix in terms of image noise and streak artifacts. In objective metrics, compared to 512 matrix, image noise was higher and SNR was lower in 1,024 matrix scans (P<0.05).
Table 1
Evaluation indicators | 512 matrix | 1,024 matrix | P |
---|---|---|---|
Subjective evaluation | |||
Image noise | 4.21 | 3.59 | <0.001 |
Streak artifacts | 3.70 | 3.32 | <0.001 |
Pulmonary nodule edges | 3.43 | 4.53 | <0.001 |
Clarity of small blood vessels | 3.61 | 4.03 | <0.001 |
Homogeneity of normal lung parenchyma | 3.36 | 4.25 | <0.001 |
Overall image quality | 3.63 | 4.18 | <0.001 |
Objective evaluation | |||
Image noise | 33.50 | 36.65 | <0.001 |
SNR | 26.08 | 23.70 | <0.001 |
SNR, signal-to-noise ratio.
Lung nodules detection
The detection accuracy rate, precision, and recall of the 512 and the 1,024 matrix, when considering pulmonary nodules as a unit, were 91.63% vs. 98.32%, 95.68% vs. 98.32%, and 95.59% versus 100%, respectively. The lung nodules were detected with higher accuracy, precision, and recall rates in 1,024×1,024. The detection in different types (solid, part-solid, and nonsolid nodules) and different sizes (<6, 6–8, and >8 mm) of pulmonary nodules were counted separately and the results are shown in Table 2. First, among different sizes of nodules, AI-CAD achieved 97.87%, 99.04%, and 99.36% accuracy for the detection of lung nodules with a long diameter <6, 6–8, and >8 mm in 1,024 matrix, respectively, which were higher than that in 512 matrix. In addition, the detection rate of AI-CAD was low for lung nodules <6 mm in either scheme, 90.58% in 512 and 97.87% in 1024. For nodules of different types, the accuracy of detecting all types of nodules in the 1,024 matrix was not less than 97.74%, representing a high detection efficacy. While in the 512 matrix, the AI-CAD detection rates for solid, part-solid, and nonsolid nodules were 91.3%, 94.63%, and 94.71%, respectively, which is slightly less effective in detecting solid nodules.
Table 2
Variable | 512 matrix | 1,024 matrix | |||||
---|---|---|---|---|---|---|---|
Accuracy, % | Precision, % | Recall, % | Accuracy, % | Precision, % | Recall, % | ||
All nodules | 91.63 | 95.68 | 95.59 | 98.32 | 98.32 | 100 | |
Nodule size | |||||||
<6 mm | 90.58 | 95.17 | 94.95 | 97.87 | 97.87 | 100.00 | |
6–8 mm | 96.64 | 98.29 | 98.29 | 99.04 | 99.04 | 100.00 | |
>8 mm | 93.68 | 96.62 | 96.85 | 99.36 | 99.36 | 100.00 | |
Nodule type | |||||||
Solid | 91.30 | 95.56 | 95.34 | 98.34 | 98.34 | 100.00 | |
Part-solid | 94.63 | 97.49 | 97.00 | 98.50 | 98.50 | 100.00 | |
Nonsolid | 94.71 | 95.98 | 98.62 | 97.74 | 97.74 | 100.00 |
Discussion
Key findings
In this study, we compared the performance of 1,024 and 512 matrix in terms of both image quality and AI-CAD detection of lung nodules. Higher overall subjective image quality scores were obtained with the 1,024 matrix although some degree of sacrifice in image noise was made. The lung nodules were detected by AI-CAD with higher accuracy, precision, and recall in 1,024×1,024 matrix. Compared to 512 matrix, 1,024 matrix can improve the image quality and accuracy of AI-CAD evaluation of lung nodules.
Comparison with similar researches and explanations of findings
In terms of image quality, Hata et al. (21) compared the subjective image quality of different matrix CT scanning protocols (512×512, 1,024×1,024, 2,048×2,048) on 11 cadaveric lungs for overall image quality, noise, streak artifacts, vascularity, bronchiality, and image findings, and showed that except for noise and streak artifacts, both the 2,048 and 1,024 matrices performed significantly better than the 512 matrix. Later, Tsubamoto et al. (12) performed a prospective CT image quality assessment of lung nodules and showed that despite the increase in noise, lung nodules could be better assessed using the 1,024 matrix scanning protocol. This is the same as the results of our study. Moreover, in this study, the image quality and AI-CAD detection efficiency of 512×512 and 1,024×1,024 under the same conditions (scanning conditions and post-processing conditions) were investigated, so no post-processing algorithms to improve image quality were used specifically for 1,024. However, in the studies of Jiang et al. (24,25), it has been confirmed that image noise can be significantly reduced by deep learning image post-processing methods so that the images can meet the needs of clinical diagnosis.
We not only counted the overall nodule detection rate but further analyzed the nodules of different sizes and types in groups. First, it can be seen that the overall AI-CAD detection of nodules in 1,024 is better than the 512 matrix, which increases the confidence and necessity for the popularization of 1,024. With the same settings of the AI-CAD, the AI still had leakage in the 512×512 matrix, whereas the 1,024×1,024 matrix achieved the detection of all lung nodules. In the daily use of AI-CAD, radiologists prefer AI-CAD to help detect lung nodules, and can tolerate the slight lack of specificity. In this regard, 1,024 can better meet the corresponding diagnostic needs. Second, among nodules of different sizes, the accuracy of AI-CAD detection of lung nodules <6 mm in both schemes was lower in the other two groups, 90.58% in 512 and 97.87% in 1,024. In clinical practice, the detection of small pulmonary nodules by manual judgment of radiologists and AI-CAD is still challenging. In the study by Agnes et al. (26), the AI-CAD was slightly less sensitive in detecting smaller lung nodules, with a sensitivity of 0.92 for nodules in the size range of 5–9 mm and 0.93 in nodules >10 mm. In particular, some computer-aided diagnosis introduce a filtering step to remove irrelevant images from the dataset to improve the efficiency of detecting lung nodules and to reduce the false positive rate. However, this method may miss some very small nodules or fail to detect certain types of nodules (27-29). For this problem, our study employs improved FPN, multilevel feature fusion strategy, and introduction of attention mechanism to fully utilize the features at all levels and to improve the detection accuracy and reduce the omissions, which obtains excellent results in 1,024 matrices. In addition, for different types of nodules, unlike the manualization of lung nodules that tends to miss pure ground-glass nodules, AI-CAD judgment has the highest misdiagnosis rate for solid nodules in 512×512 matrix. Lung nodule detection is important as the first step in the diagnosis of lung nodules, but few of the studies that have been done on lung nodule detection have analyzed different types of lung nodules in groups. The results of this study are of reference value to diagnostic radiologists, who can rationally apply AI-CAD in their clinical work.
Strengths and limitations
Previous comparative studies between 1,024×1,024 matrix and 512×512 matrix are mostly limited to the subjective evaluation and objective parameter evaluation of image quality (30-32), whereas with the current application of AI-assisted diagnostic systems (33,34), more and more attention has been paid to the comparison of the performance of AI-CAD in evaluating lung nodules between 1,024 matrix and 512 matrix. In the case of different hospitals with different scanning equipment, we explored the results of 512×512 and 1,024×1,024 matrix with the same AI-CAD for performing lung nodule detection and further verified the difference analysis of 1,024×1,024 and 512×512 matrix in real-world lung nodules evaluation applications, which has strong practical significance.
There are several limitations to this study. First, the sample size in this study was small, and a larger database is expected for further exploration in the future. Second, in addition to the overall quality of the images, we only focused on lung nodules, and further study is needed to determine whether HRCT images can improve the assessment of diffuse lung diseases so that more patients can benefit from it. Finally, the subjective assessment did not include the diagnosis of benign and malignant lung nodules and comparison with histopathology, and whether the accuracy of malignancy diagnosis increased was not assessed; further studies are required to fully evaluate the clinical utility of the ultra-high-resolution model of deep learning images.
Implications and actions needed
The 1,024-matrix scanning scheme performed significantly better than the 512-matrix in terms of subjective image quality and lung nodule AI detection rate for all CT images. The advantages of the 1,024 matrix scanning program in computer-aided diagnosis are demonstrated, adding evidence and confidence to its widespread use.
Conclusions
In conclusion, the 1,024-matrix scanning scheme performed significantly better than the 512-matrix in terms of subjective image quality and lung nodule AI detection rate for all CT images. For AI-CAD evaluation of lung nodules, the adoption of a 1,024×1,024 reconstruction matrix enhanced image quality, enhanced detail, and led to more accurate results.
Acknowledgments
None.
Funding: This study was funded by
Footnote
Reporting Checklist: The authors have completed the STARD reporting checklist. Available at https://jtd.amegroups.com/article/view/10.21037/jtd-24-1311/rc
Data Sharing Statement: Available at https://jtd.amegroups.com/article/view/10.21037/jtd-24-1311/dss
Peer Review File: Available at https://jtd.amegroups.com/article/view/10.21037/jtd-24-1311/prf
Conflicts of Interest: All authors have completed the ICMJE uniform disclosure form (available at https://jtd.amegroups.com/article/view/10.21037/jtd-24-1311/coif). C.Z., X.M., and Y.H. report full-time employees of Shukun (Beijing) Network Technology Co., Ltd. The other authors have no conflicts of interest to declare.
Ethical Statement: The authors are accountable for all aspects of the work in ensuring that questions related to the accuracy or integrity of any part of the work are appropriately investigated and resolved. The study was conducted in accordance with the Declaration of Helsinki (as revised in 2013). The study was approved by the Ethics Committee of Shanghai Changzheng Hospital (No. 2022SL070). The other center was informed and agreed with the study. Due to the retrospective nature of the analysis and the anonymity of the data, the requirement for patients’ informed consent was waived.
Open Access Statement: This is an Open Access article distributed in accordance with the Creative Commons Attribution-NonCommercial-NoDerivs 4.0 International License (CC BY-NC-ND 4.0), which permits the non-commercial replication and distribution of the article with the strict proviso that no changes or edits are made and the original work is properly cited (including links to both the formal publication through the relevant DOI and the license). See: https://creativecommons.org/licenses/by-nc-nd/4.0/.
References
- Zhang Y, Luo G, Etxeberria J, et al. Global Patterns and Trends in Lung Cancer Incidence: A Population-Based Study. J Thorac Oncol 2021;16:933-44. [Crossref] [PubMed]
- Sung H, Ferlay J, Siegel RL, et al. Global Cancer Statistics 2020: GLOBOCAN Estimates of Incidence and Mortality Worldwide for 36 Cancers in 185 Countries. CA Cancer J Clin 2021;71:209-49. [Crossref] [PubMed]
- Li N, Tan F, Chen W, et al. One-off low-dose CT for lung cancer screening in China: a multicentre, population-based, prospective cohort study. Lancet Respir Med 2022;10:378-91. [Crossref] [PubMed]
- Yankelevitz DF, Yip R, Smith JP, et al. CT Screening for Lung Cancer: Nonsolid Nodules in Baseline and Annual Repeat Rounds. Radiology 2015;277:555-64. [Crossref] [PubMed]
- Nicholson AG, Tsao MS, Beasley MB, et al. The 2021 WHO Classification of Lung Tumors: Impact of Advances Since 2015. J Thorac Oncol 2022;17:362-87. [Crossref] [PubMed]
- MacMahon H, Naidich DP, Goo JM, et al. Guidelines for Management of Incidental Pulmonary Nodules Detected on CT Images: From the Fleischner Society 2017. Radiology 2017;284:228-43. [Crossref] [PubMed]
- Christensen J, Prosper AE, Wu CC, et al. ACR Lung-RADS v2022: Assessment Categories and Management Recommendations. Chest 2024;165:738-53. [Crossref] [PubMed]
- Qin W, Ge J, Gong Z, et al. The incidence and risk factors of acute pain after preoperative needle localization of pulmonary nodules: a cross-sectional study. Transl Lung Cancer Res 2022;11:1667-77. [Crossref] [PubMed]
- He C, Zhao L, Yu HL, et al. Incidence and risk factors for pulmonary hemorrhage after percutaneous CT-guided pulmonary nodule biopsy: an observational study. Sci Rep 2024;14:7348. [Crossref] [PubMed]
- Bankier AA, MacMahon H, Goo JM, et al. Recommendations for Measuring Pulmonary Nodules at CT: A Statement from the Fleischner Society. Radiology 2017;285:584-600. [Crossref] [PubMed]
- Zhu Y, Hou D, Lan M, et al. A comparison of ultra-high-resolution CT target scan versus conventional CT target reconstruction in the evaluation of ground-glass-nodule-like lung adenocarcinoma. Quant Imaging Med Surg 2019;9:1087-94. [Crossref] [PubMed]
- Tsubamoto M, Hata A, Yanagawa M, et al. Ultra high-resolution computed tomography with 1024-matrix: Comparison with 512-matrix for the evaluation of pulmonary nodules. Eur J Radiol 2020;128:109033. [Crossref] [PubMed]
- Zhao Y, Hernandez AM, Boone JM, et al. Quantification of airway dimensions using a high-resolution CT scanner: A phantom study. Med Phys 2021;48:5874-83. [Crossref] [PubMed]
- Nam JG, Hwang EJ, Kim J, et al. AI Improves Nodule Detection on Chest Radiographs in a Health Screening Population: A Randomized Controlled Trial. Radiology 2023;307:e221894. [Crossref] [PubMed]
- Bennani S, Regnard NE, Ventre J, et al. Using AI to Improve Radiologist Performance in Detection of Abnormalities on Chest Radiographs. Radiology 2023;309:e230860. [Crossref] [PubMed]
- Wang P, Liu X, Berzin TM, et al. Effect of a deep-learning computer-aided detection system on adenoma detection during colonoscopy (CADe-DB trial): a double-blind randomised study. Lancet Gastroenterol Hepatol 2020;5:343-51. [Crossref] [PubMed]
- Beede E, Baylor E, Hersch F, et al. A human-centered evaluation of a deep learning system deployed in clinics for the detection of diabetic retinopathy. Proceedings of the 2020 CHI conference on human factors in computing systems; 2020. doi:
10.1145/3313831.337671 . - Lancaster HL, Zheng S, Aleshina OO, et al. Outstanding negative prediction performance of solid pulmonary nodule volume AI for ultra-LDCT baseline lung cancer screening risk stratification. Lung Cancer 2022;165:133-40. [Crossref] [PubMed]
- Chen L, Gu D, Chen Y, et al. An artificial-intelligence lung imaging analysis system (ALIAS) for population-based nodule computing in CT scans. Comput Med Imaging Graph 2021;89:101899. [Crossref] [PubMed]
- Adams SJ, Mondal P, Penz E, et al. Development and Cost Analysis of a Lung Nodule Management Strategy Combining Artificial Intelligence and Lung-RADS for Baseline Lung Cancer Screening. J Am Coll Radiol 2021;18:741-51. [Crossref] [PubMed]
- Hata A, Yanagawa M, Honda O, et al. Effect of Matrix Size on the Image Quality of Ultra-high-resolution CT of the Lung: Comparison of 512 × 512, 1024 × 1024, and 2048 × 2048. Acad Radiol 2018;25:869-76. [Crossref] [PubMed]
- Eshratifar AE, Eigen D, Gormish M, et al. Coarse2Fine: a two-stage training method for fine-grained visual classification. Machine Vision and Applications 2021;32:49. [Crossref]
- American College of Radiology.Lung CT Screening Reporting & Data System (Lung-RADS®). 2022. Available online: https://www.acr.org/Clinical-Resources/Reporting-and-Data-Systems/Lung-Rads
- Jiang B, Li N, Shi X, et al. Deep Learning Reconstruction Shows Better Lung Nodule Detection for Ultra-Low-Dose Chest CT. Radiology 2022;303:202-12. [Crossref] [PubMed]
- Jiang Q, Sun H, Deng W, et al. Super Resolution of Pulmonary Nodules Target Reconstruction Using a Two-Channel GAN Models. Acad Radiol 2024;31:3427-37. [Crossref] [PubMed]
- Akila Agnes S, Anitha J, Arun Solomon A. Two-stage lung nodule detection framework using enhanced UNet and convolutional LSTM networks in CT images. Comput Biol Med 2022;149:106059. [Crossref] [PubMed]
- Liang J, Ye G, Guo J, et al. Reducing False-Positives in Lung Nodules Detection Using Balanced Datasets. Front Public Health 2021;9:671070. [Crossref] [PubMed]
- Setio AA, Ciompi F, Litjens G, et al. Pulmonary Nodule Detection in CT Images: False Positive Reduction Using Multi-View Convolutional Networks. IEEE Trans Med Imaging 2016;35:1160-9. [Crossref] [PubMed]
- Huang X, Shan J, Vaidya V, editors. Lung nodule detection in CT using 3D convolutional neural networks. 2017 IEEE 14th International Symposium on Biomedical Imaging (ISBI 2017); 2017: IEEE.
- Ashish V. Attention is all you need. Advances in Neural Information Processing Systems 30 (NIPS 2017) 2017;30:6000-10.
- Ogawa K, Onishi H, Hori M, et al. Visualization of small visceral arteries on abdominal CT angiography using ultra-high-resolution CT scanner. Jpn J Radiol 2021;39:889-97. [Crossref] [PubMed]
- Ucar FA, Frenzel M, Kronfeld A, et al. Improvement of Neurovascular Imaging Using Ultra-High-Resolution Computed Tomography Angiography. Clin Neuroradiol 2024;34:189-99. [Crossref] [PubMed]
- Homayounieh F, Digumarthy S, Ebrahimian S, et al. An Artificial Intelligence-Based Chest X-ray Model on Human Nodule Detection Accuracy From a Multicenter Study. JAMA Netw Open 2021;4:e2141096. [Crossref] [PubMed]
- Singh R, Kalra MK, Homayounieh F, et al. Artificial intelligence-based vessel suppression for detection of sub-solid nodules in lung cancer screening computed tomography. Quant Imaging Med Surg 2021;11:1134-43. [Crossref] [PubMed]