A LASSO-derived model for the prediction of coagulation disorders after coronary artery bypass grafting
Highlight box
Key findings
• A prediction model for assessing the risk of coagulation disorders after coronary artery bypass grafting (CABG) was developed and demonstrated good prediction performance in elderly individuals, employing the least absolute shrinkage and selection operator (LASSO) regression.
What is known and what is new?
• Preoperative variables associated with coagulation disorders included weight, baseline estimated glomerular filtration rate, B-type natriuretic peptide, platelet count, preoperative use of heparin and angiotensin receptor-neprilysin inhibitor.
• Intraoperative variables associated with coagulation disorders included utilization of epinephrine, norepinephrine, dopamine, cephalosporins, cardiopulmonary bypass, intra-aortic balloon pump, extracorporeal membrane oxygenation, operation duration, and total intraoperative fluid input.
What is the implication, and what should change now?
• This LASSO-derived model exhibited a good predictive performance and is helpful for early detection of elderly Chinese patients at high risk for coagulation disorders post-CABG surgery.
Introduction
Coronary artery bypass grafting (CABG) is a standard treatment option for coronary heart disease (CHD). As the incidence of CHD continues to rise, the number of CABG procedures performed has also gradually increased. CABG surgery can activate vascular endothelial cells, which can lead to vasoconstriction, coagulation disorders, and inflammatory responses, ultimately causing dysfunction of vascular endothelial cells (1). Postoperative bleeding is one of the most common complications of CABG and is positively correlated with increased risks of stroke, acute kidney injury (AKI), perioperative myocardial infarction, and mortality. Furthermore, postoperative red blood cell transfusions also elevate the risks of infection, organ damage, and mortality among patients (2,3). Therefore, reducing the risk of postoperative bleeding in CABG patients can help shorten hospital stays, decrease hospitalization costs, and ultimately enhance patient outcomes and benefits.
A retrospective study showed that surgical factors and coagulation dysfunction were the main causes of postoperative bleeding after CABG, among which 66% were caused by surgery and 34% by coagulation dysfunction (4). Compared with patients with surgical bleeding, patients with bleeding caused by coagulation disorders had a longer postoperative hospital stay, higher morbidity and mortality, and significantly increased use of blood products. However, several factors affect the postoperative coagulation disorders of the patients, such as the duration of cardiopulmonary bypass (CPB), CABG combined with valve replacement, blood product infusion volume, and the demographic characteristics of the patients, comorbidities (such as chronic kidney disease, liver disease or diabetes), preoperative platelet count, use of antiplatelet drugs (5,6). Elderly patients who undergo CABG surgery are particularly susceptible to postoperative coagulation disorders due to factors like impaired liver and kidney function, advanced age, and the presence of comorbidities. The purpose of this study was to establish and validate a risk prediction model for postoperative coagulation disorders in elderly patients after CABG surgery, effectively identify high-risk patients prone to coagulation disorders, reduce the risk of postoperative bleeding by preventive treatment measures, and strengthen the postoperative treatment monitoring for these patients. We present this article in accordance with the TRIPOD reporting checklist (available at https://jtd.amegroups.com/article/view/10.21037/jtd-24-1321/rc).
Methods
Study population
Patients who underwent CABG from February 2019 to December 2020 were retrospectively enrolled based on the inclusion and exclusion criteria. Inclusion criteria were: (I) age ≥65 years; (II) confirmed diagnosis of CHD; (III) underwent CABG surgery. Exclusion criteria were: (I) no preoperative coagulation function tests performed; (II) preoperative platelet count <100×109/L; (III) preoperative prothrombin time (PT) >12.8 seconds; (IV) preoperative liver insufficiency (Child-Pugh class B or C) or renal insufficiency [estimated glomerular filtration rate (eGFR) <30 mL/min/1.73 m2]; (V) oral anticoagulants (warfarin, dabigatran etexilate, or rivaroxaban) were used after surgery; (VI) myocardial infarction within one month prior to surgery; (VII) concurrent use of oral anticoagulants or antiplatelet drugs other than aspirin within 7 days prior to surgery; (VIII) patients undergoing secondary CABG; (IX) missing case information; (X) death during the operation. This study was approved by the Ethics Committee of Beijing Anzhen Hospital, Capital Medical University (approval No. 2021-069). This study was conducted in accordance with the principles of the Declaration of Helsinki (as revised in 2013) and informed consent was taken from all the patients.
Definition of postoperative coagulation disorders
Previous studies have predominantly relied on a single coagulation indicator to assess prognosis, neglecting the exploration of combined indicators. Furthermore, there is a lack of consensus regarding the definition criteria for coagulation disorders. This study adopted the disseminated intravascular coagulation (DIC) scoring system formulated by the International Society of Thrombosis and Haemostasis (ISTH) (Table 1) to define coagulation disorders, and DIC score ≥2 was defined as coagulation disorders (7).
Table 1
DIC score | PLT (×109/L) | D-dimer (mg/L) | FIB (g/L) | PT (s) |
---|---|---|---|---|
0 | >100 | ≤1.0 | ≥1.0 | ≤3.0 |
1 | 50–100 | >1.0–5.0 | <1.0 | >3.0–6.0 |
2 | <50 | >5.0 | – | >6.0 |
DIC, disseminated intravascular coagulation; ISTH, International Society of Thrombosis and Haemostasis; PLT, platelet; FIB, fibrinogen; PT, prothrombin time.
Statistical analysis
Statistical analyses were conducted using R software version 4.2.2. The entire cohort was randomly divided into a derivation set and a validation set at a ratio of 7:3. The Kolmogorov-Smirnov test was employed to assess the normality of continuous variables. For non-normally distributed continuous variables, medians and interquartile ranges (IQRs) were reported, and the Mann-Whitney U-test was applied. Categorical variables were presented as counts and percentages, with Chi-squared tests or Fisher’s exact tests performed as appropriate. P value <0.05 was considered statistically significant. Variables with missing values exceeding 20% were excluded, and the remaining missing values were imputed using mean values.
In the multivariate analysis of the derivation set, all predictor variables were included in a logistic regression model. The least absolute shrinkage and selection operator (LASSO) regression was implemented using the glmnet package in R for variable selection. The occurrence of coagulation disorder events served as the dependent variable, with patients experiencing the event coded as 1 and those without coded as 0. Ten-fold cross-validation was utilized to select the optimal penalty parameter Lambda (λ), with Lambda + 1se chosen to prevent model overfitting. The model’s performance was evaluated using confusion matrices and receiver operating characteristic (ROC) curves.
The predictive performance of the model for coagulation disorders was separately validated in both the derivation and validation sets. The area under the ROC curve (AUC) was calculated to assess the predictive accuracy of the model. Model calibration was performed using calibration curves based on 1,000 bootstrap samples.
Results
Patient characteristics
This study ultimately enrolled 1,266 patients, among whom 157 (12.4%) developed postoperative coagulation disorders. In the overall population, the median age was 69.0 years (range, 65.1–87.2 years), and 68.3% of the patients were male. Hypertension (66.8%) was the most prevalent comorbidity, followed by hyperlipidemia (56.8%) and type 2 diabetes (39.4%). The entire cohort was randomly divided into a derivation set (886 cases) and a validation set (380 cases) at a ratio of 7:3. As presented in Table 2, there were no statistically significant differences between the two groups in terms of baseline information and clinical characteristics (P<0.05). Within the derivation set, 110 patients (12.4%) were categorized into the coagulation disorder group, while 776 (87.6%) belonged to the normal coagulation group. The study selection flowchart is shown in Figure 1.
Table 2
Variables | The derivation set (N=886) | The validation set (N=380) | P value |
---|---|---|---|
Demographics | |||
Age (years)* | 69 (67, 73) | 69 (67, 73) | 0.34 |
Male sex | 599 (67.6) | 266 (70.0) | 0.40 |
Smoker | 177 (20.0) | 81 (21.3) | 0.59 |
Drinker | 163 (18.4) | 60 (15.8) | 0.26 |
BMI (kg/m2)* | 25.1 (22.9, 27.3) | 25.4 (23.9, 27.3) | 0.01 |
Complication | |||
Hypertension | 588 (66.4) | 258 (67.9) | 0.60 |
T2DM | 335 (37.8) | 164 (43.2) | 0.07 |
Hyperlipemia | 496 (56.0) | 223 (58.7) | 0.37 |
Prior MI | 113 (12.8) | 63 (16.6) | 0.07 |
Prior cerebral infarction | 107 (12.1) | 47 (12.4) | 0.88 |
Prior PCI | 86 (9.7) | 36 (9.5) | 0.90 |
NYHA cardiac functional class | |||
Class III/IV | 145 (16.4) | 63 (16.6) | 0.93 |
Laboratory examinations at admission* | |||
eGFR (mL/min) | 76.1 (64.2, 90.7) | 78.3 (65.9, 92.1) | 0.15 |
SCr (μmol/L) | 73.05 (62.5, 84.1) | 72.2 (63.2, 84.4) | 0.94 |
UA (μmol/L) | 325.3 (270.6, 389.6) | 325.2 (270.3, 388.6) | 0.91 |
BNP (pg/mL) | 155.5 (60.0, 255.0) | 158.5 (70.3, 261.3) | 0.30 |
PLT (×109/L) | 221 (186, 262) | 224.5 (186, 262) | 0.99 |
ALT (U/L) | 20 (14, 31) | 21 (15, 31) | 0.15 |
AST (U/L) | 21 (17, 27) | 21 (17.25, 28) | 0.045 |
Preoperative concomitant medication | |||
Aspirin | 230 (26.0) | 106 (27.9) | 0.48 |
Statins | 181 (20.4) | 84 (22.1) | 0.50 |
ACEI/ARB | 156 (17.6) | 55 (14.5) | 0.17 |
ARNI | 26 (2.9) | 9 (2.4) | 0.57 |
Heparin | 376 (42.4) | 166 (43.7) | 0.68 |
Preoperative use of IABP# | 2 (0.2) | 3 (0.8) | 0.16 |
Intraoperative | |||
Use of IABP | 67 (7.6) | 27 (7.1) | 0.78 |
Use of ECMO | 3 (0.3) | 2 (0.5) | 0.63 |
Use of CPB | 74 (8.4) | 36 (9.5) | 0.52 |
Use of epinephrine | 147 (16.6) | 80 (21.1) | 0.058 |
Use of norepinephrine | 489 (55.2) | 213 (56.1) | 0.78 |
Use of isoprenaline | 40 (4.5) | 22 (5.8) | 0.34 |
Use of dopamine | 749 (84.5) | 332 (87.4) | 0.19 |
Use of cephalosporin | 716 (80.8) | 323 (85.0) | 0.08 |
Operation time (h)* | 4 (4, 5) | 4 (4, 5) | 0.83 |
Operation urine volume (×100 mL) | 12.0 (6.4, 20.0) | 10.5 (6.0, 17.0) | 0.09 |
Operation total fluid input (×100 mL) | 25.0 (20.5, 30.0) | 25.8 (20.5, 30.0) | 0.62 |
Categorical data were presented as count (percentage) and were calculated by Chi-squared test. *, continuous data are expressed as median (interquartile range) and were calculated by Mann-Whitney U-test. #, Fisher’s exact test was used. BMI, body mass index; T2DM, type 2 diabetes mellitus; MI, myocardial infarction; PCI, percutaneous coronary intervention; NYHA, New York Heart Association; eGFR, estimated glomerular filtration rate; SCr, serum creatinine; UA, uric acid; BNP, B-type natriuretic peptide; PLT, platelet; ALT, alanine transaminase; AST, aspartate amino transferase; ACEI, angiotensin converting enzyme inhibitor; ARB, angiotensin II receptor blocker; ARNI, angiotensin receptor-neprilysin inhibitor; IABP, intra-aortic balloon pump; ECMO, extracorporeal membrane oxygenation; CPB, cardiopulmonary bypass.
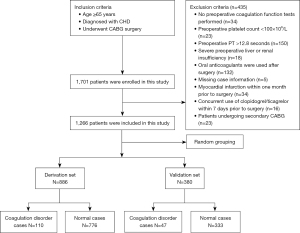
LASSO regression analysis
Due to the large number of variables and relatively small number of cases, LASSO regression was employed for variable selection. Figure 2A displays the variables selected by LASSO regression, while Figure 2B indicated the optimal lambda + 1se position and 15 variables identified. The findings of our study indicated that various risk factors were associated with postoperative coagulation disorders. These factors encompassed patient weight, preoperative baseline estimated glomerular filtration rate (eGFR), B-type natriuretic peptide (BNP), and platelet count (PLT). Additionally, preoperative administration of heparin and angiotensin receptor-neprilysin inhibitor (ARNI), as well as intraoperative utilization of epinephrine, norepinephrine, dopamine, cephalosporins, CPB, intra-aortic balloon pump (IABP), extracorporeal membrane oxygenation (ECMO), operation duration, and total intraoperative fluid input were identified as significant contributors. A detailed enumeration of these factors is presented in Table 3.
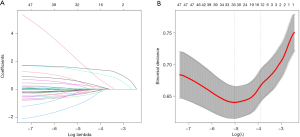
Table 3
Variables | Risk factors | Coefficient |
---|---|---|
X1 | Weight | −0.00095 |
X2 | eGFR | −0.00005 |
X3 | BNP | 0.00003 |
X4 | PLT | −0.00042 |
X5 | Preoperative use of heparin | −0.00391 |
X6 | Preoperative use of ARNI | −0.00020 |
X7 | Intraoperative use of epinephrine | 0.01513 |
X8 | Intraoperative use of norepinephrine | 0.01523 |
X9 | Intraoperative use of dopamine | −0.01492 |
X10 | Intraoperative use of cephalosporin | −0.00162 |
X11 | Intraoperative use of CPB | 0.15075 |
X12 | Intraoperative use of IABP | 0.21603 |
X13 | Intraoperative use of ECMO | 0.20504 |
X14 | Operation time | 0.00299 |
X15 | Operation total fluid input | 0.00055 |
Intercept | 0.23076 |
CABG, coronary artery bypass grafting; eGFR, estimated glomerular filtration rate; BNP, B-type natriuretic peptide; PLT, platelet; ARNI, angiotensin receptor-neprilysin inhibitor; CPB, cardiopulmonary bypass; IABP, intra-aortic balloon pump; ECMO, extracorporeal membrane oxygenation.
Model validation and calibration
To evaluate the predictive performance of the model, ROC curves for both the derivation and validation sets were plotted (Figure 3). The AUC for the derivation set was 0.818 [95% confidence interval (CI): 0.775−0.862] (Figure 3A), while the AUC for the validation set was 0.827 (95% CI: 0.755−0.898) (Figure 3B). The confusion matrix plots and validation results for both sets are presented in Tables 4,5, respectively. The model achieved a sensitivity of 80.0% and a specificity of 70.0% in the derivation set, and a sensitivity of 76.6% and a specificity of 81.7% in the validation set. Calibration curves were plotted to assess the agreement between the predicted and observed coagulation disorders in the derivation set (Figure 4A) and the validation set (Figure 4B). The results indicated that the model exhibited good predictive performance.
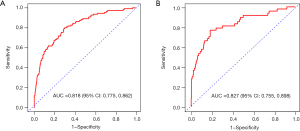
Table 4
Data set | Values | Actual value (positive) | Actual value (negative) |
---|---|---|---|
The derivation set | Predict value (positive) | 88 | 233 |
Predict value (negative) | 22 | 543 | |
The validation set | Predict value (positive) | 36 | 61 |
Predict value (negative) | 11 | 272 |
Table 5
Data set | Sensitivity (%) | Specificity (%) | Accuracy (%) |
---|---|---|---|
The derivation set | 80.0 | 70.0 | 71.2 |
The validation set | 76.6 | 81.7 | 81.1 |
Bleeding complications
Based on the Universal Definition of Perioperative Bleeding in Adult Cardiac Surgery for defining post-cardiac surgery bleeding events (8), we compared the differences in bleeding between patients with coagulation disorders and those with normal coagulation function (Table 6). Our findings revealed that for Class 0 (insignificant) and Class 1 (mild) bleeding, the proportion of patients in the normal coagulation group was significantly higher than that in the coagulation disorders group. Conversely, for Class 3−4 (severe-massive) bleeding, the incidence rate was significantly elevated in the coagulation disorders group relative to the normal coagulation group.
Table 6
Bleeding class | Coagulation disorder group (N=157) | Normal group (N=1,109) | P value |
---|---|---|---|
Class 0 (insignificant) | 38 (24.2) | 500 (45.1) | <0.001 |
Class 1 (mild) | 25 (15.9) | 278 (25.1) | 0.01 |
Class 2 (moderate) | 43 (27.4) | 212 (19.1) | 0.02 |
Class 3–4 (severe-massive) | 51 (32.5) | 119 (10.7) | <0.001 |
Total significant bleeding | 119 (75.8) | 609 (54.9) | <0.001 |
Discussion
This study focused on identifying risk factors for coagulation disorders in patients after CABG surgery, including preoperative baseline variables and intraoperative variables. Based on these risk factors, a prediction model with robust predictive performance was established.
The role of CPB
The classic CABG procedure involves the use of CPB, which, while necessary, has detrimental effects on patients’ coagulation mechanisms. CPB triggers the activation of the coagulation system due to blood contact with artificial surfaces, mechanical injury to blood cells, and systemic inflammatory responses. This activation disrupts the balance of anticoagulant mechanisms, contributing significantly to postoperative coagulation disorders. Additionally, anticoagulants like heparin, used during CPB, inhibit thrombin activity but may also lead to postoperative coagulation issues and increased bleeding risks.
A retrospective study found that age over 60 years, low BMI, preoperative thrombocytopenia, and prolonged CPB duration were significant predictors of thrombocytopenia after cardiac surgery with CPB (9). Furthermore, the impact of CPB on coagulation function is well-documented and results from a combination of hemodilution, hypothermia and rewarming effects, and exposure of plasma and cellular blood components to non-physiological flow patterns. This leads to the activation, consumption, and dilution of platelets and coagulation factors, as well as the activation of inflammatory cascades (10).
The influence of IABP and ECMO
IABP and ECMO are crucial life-support technologies. However, our study revealed that both IABP and ECMO are risk factors for coagulation disorders following CABG. The underlying mechanisms may involve vascular endothelial injury and inflammatory responses.
Patient-specific and surgical factors
Platelets play a crucial role in coagulation function, and thrombocytopenia after CABG is influenced by multiple factors, including age, preoperative platelet count, comorbidities (such as chronic kidney disease and diabetes mellitus), and medications (like antiplatelet drugs) (9,11). Surgical factors, such as the complexity of the procedure, the use of IABP or ECMO, and the duration of surgery and CPB, also play significant roles.
Our findings reconfirm the impact of coagulation disorders, the use of CPB, IABP, and ECMO, as well as surgical duration on coagulation function. Additionally, studies suggest that shear stress and the high tensile state imposed on bypass vessels stimulate endothelial cells to generate reactive oxygen species (ROS), leading to oxidative stress, inflammatory responses, autoimmune platelet clearance, and endothelial dysfunction (12,13). This dysfunctional state exacerbates lipid peroxidation and perpetuates coagulation dysfunction.
Vascular and temperature factors
During vascular injury, stressed and compromised endothelial cells emit a cascade of vasoactive substances, leading to vasodilation and disrupting microcirculatory homeostasis. Furthermore, there is a notable elevation in the release of tissue plasminogen activator, resulting in abnormal vasomotor and fibrinolytic function (14). In addition to vascular factors, patient-specific factors also increase the risk of postoperative coagulation disorders. A study focusing on endothelial function within bypass vessels of CABG patients revealed that advanced age and overweight stimulated ROS production from endothelial cells, contributing to endothelial dysfunction (15).
Hypothermia can reduce the enzymatic activity of coagulation factors, impair platelet function, and disrupt the fibrinolytic system. Under mild hypothermia, the production of thrombinase is slightly delayed, and platelet adhesion and aggregation functions are reduced. When body temperature falls below 33 ℃, platelet activation and enzyme activity are also affected. Additionally, hypothermia can trigger the release of heparin-like anticoagulant substances into circulation, further contributing to coagulation disorders (16-18). Unfortunately, our study did not incorporate data on intraoperative body temperature variations, which limited our exploration of the impact of hypothermia on postoperative coagulation disorders.
Blood dilution and vasoconstrictors
Blood dilution after CABG can cause a decrease in fibrinogen concentration, leading to coagulation disorders. A study by Gielen et al. showed that blood dilution could cause a decrease in fibrinogen concentration after CABG, leading to coagulation disorders (19). Similarly, intraoperative massive infusions of fluid can reduce coagulation factor concentrations and platelet counts.
Administration of vasoconstrictors during surgery also contributes to postoperative coagulation disorders. This is attributed to the tendency to increase the types and doses of vasoconstrictors when patients experience hemodynamic instability. In addition, the use of vasoconstrictors may also cause microcirculation disorders and changes in blood viscosity, thereby affecting the normal function of coagulation factors and platelet aggregation, and ultimately leading to coagulation disorders.
Preoperative baseline factors
Furthermore, our results also indicated that patients’ preoperative baseline cardiac and renal functions were significant factors influencing coagulation function following cardiac surgery. Cardiac insufficiency may lead to impaired blood circulation and abnormal platelet function, while renal insufficiency may affect the metabolism and excretion of anticoagulants as well as cause electrolyte disturbances.
Development and application of model
Through the exploration of risk factors associated with coagulation disorders, we have developed a model for predicting post-CABG coagulation disorders, with the aim of optimizing postoperative coagulation management in elderly patients. The primary objective of this model is to identify high-risk patients undergoing CABG surgery, thereby reducing the risk of coagulation disorders. This is embodied in several key aspects: Firstly, the model integrates multifaceted information including patients’ baseline characteristics, surgical procedures, and laboratory indicators to comprehensively assess their postoperative coagulation status, thereby assisting physicians in gaining a more accurate understanding of patients’ coagulation recovery. Secondly, for the identified high-risk patients, the model aids physicians in initiating targeted preventive measures proactively. For instance, intensifying monitoring of postoperative drainage volume and pericardial effusion, timely adjustment of anticoagulant dosages, and enhancing wound care, all of which effectively decrease the incidence of bleeding complications. Lastly, by predicting patients’ postoperative coagulation needs and potential complication risks, the model facilitates a more rational allocation of medical resources, including postoperative care teams and rehabilitation facilities, ultimately enhancing the overall efficiency and quality of medical service.
Distinctiveness of the study
The distinctiveness of this study compared to previous research are reflected the following aspects: (I) previous studies mainly focused on thrombocytopenia or postoperative hemorrhage, and there was lack of research and prediction models for coagulation disorders (20-22); (II) this study focused on elderly patients aged 65 years and older who underwent CABG surgery. Due to the influence of liver and kidney function impairment, advanced age, and comorbidities, this population is more prone to postoperative coagulation disorders; (III) previous research mostly relied on a single coagulation indicator to assess prognosis, and there was a lack of research on the combination of indicators, lacking representative significance and with inconsistent definition criteria for coagulation dysfunction. In this study, we adopted the DIC scoring criteria formulated by the International Society on Thrombosis and Haemostasis (ISTH) in 2001 (as shown in Table 1), and DIC score of ≥2 was defined coagulation dysfunction; (IV) to minimize the risk of overfitting, we employed LASSO regression to select variables with significant predictive value for the outcome.
Limitations
The limitations of this study are as follows: (I) this is a retrospective single-center study, including data from only one center. The impact of risk factors and the performance of the model may differ in centers with varying demographic characteristics. Thus, external validation is required; (II) the proportion of patients receiving CPB in this study is low, and the predictive effect of this model on CPB patients may be biased, which needs to be verified and optimized by future studies with larger data; (III) it is acknowledged that there may be additional risk factors not being included in this study, such as intraoperative temperature, which could potentially influence the predictive performance of the model, and prospective in-depth studies are needed to determine its predictive effectiveness in the future.
Conclusions
In this study, LASSO regression was used to select variables to develop and validate a risk prediction model for postoperative coagulation disorders in elderly patients after CABG. The model exhibited good predictive performance in both the derivation and validation sets, which can help clinicians identify high-risk individuals with coagulation disorders following CABG.
Acknowledgments
We would like to thank Beijing Anzhen Hospital and all the participants included in this study.
Funding: None.
Footnote
Reporting Checklist: The authors have completed the TRIPOD reporting checklist. Available at https://jtd.amegroups.com/article/view/10.21037/jtd-24-1321/rc
Data Sharing Statement: Available at https://jtd.amegroups.com/article/view/10.21037/jtd-24-1321/dss
Peer Review File: Available at https://jtd.amegroups.com/article/view/10.21037/jtd-24-1321/prf
Conflicts of Interest: All authors have completed the ICMJE uniform disclosure form (available at https://jtd.amegroups.com/article/view/10.21037/jtd-24-1321/coif). The authors have no conflicts of interest to declare.
Ethical Statement: The authors are accountable for all aspects of the work in ensuring that questions related to the accuracy or integrity of any part of the work are appropriately investigated and resolved. This study was conducted in accordance with the Declaration of Helsinki (as revised in 2013). This study protocol was approved by the Ethics Committee of Beijing Anzhen Hospital, Capital Medical University (No. 2021-069) and informed consent was taken from all the patients.
Open Access Statement: This is an Open Access article distributed in accordance with the Creative Commons Attribution-NonCommercial-NoDerivs 4.0 International License (CC BY-NC-ND 4.0), which permits the non-commercial replication and distribution of the article with the strict proviso that no changes or edits are made and the original work is properly cited (including links to both the formal publication through the relevant DOI and the license). See: https://creativecommons.org/licenses/by-nc-nd/4.0/.
References
- Nazari-Shafti TZ, Thau H, Zacharova E, et al. Endothelial damage inhibitor preserves the integrity of venous endothelial cells from patients undergoing coronary bypass surgery. Eur J Cardiothorac Surg 2023;64:ezad327. [Crossref] [PubMed]
- Ngufor C, Murphree D, Upadhyaya S, et al. Effects of Plasma Transfusion on Perioperative Bleeding Complications: A Machine Learning Approach. Stud Health Technol Inform 2015;216:721-5. [PubMed]
- Crawford TC, Magruder JT, Fraser C, et al. Less is more: results of a statewide analysis of the impact of blood transfusion on coronary artery bypass grafting outcomes. Ann Thorac Surg 2018;105:129-36. [Crossref] [PubMed]
- Hall TS, Brevetti GR, Skoultchi AJ, et al. Re-exploration for hemorrhage following open heart surgery differentiation on the causes of bleeding and the impact on patient outcomes. Ann Thorac Cardiovasc Surg 2001;7:352-7. [PubMed]
- Demal TJ, Fehr S, Mariscalco G, et al. Coronary Artery Bypass Grafting in Patients With High Risk of Bleeding. Heart Lung Circ 2022;31:263-71. [Crossref] [PubMed]
- Scrascia G, Rotunno C, Nanna D, et al. Pump blood processing, salvage and re-transfusion improves hemoglobin levels after coronary artery bypass grafting, but affects coagulative and fibrinolytic systems. Perfusion 2012;27:270-7. [Crossref] [PubMed]
- Bakhtiari K, Meijers JC, de Jonge E, et al. Prospective validation of the International Society of Thrombosis and Haemostasis scoring system for disseminated intravascular coagulation. Crit Care Med 2004;32:2416-21. [Crossref] [PubMed]
- Dyke C, Aronson S, Dietrich W, et al. Universal definition of perioperative bleeding in adult cardiac surgery. J Thorac Cardiovasc Surg 2014;147:1458-1463.e1. [Crossref] [PubMed]
- Yan S, Gao S, Lou S, et al. Risk factors of thrombocytopenia after cardiac surgery with cardiopulmonary bypass. Braz J Cardiovasc Surg 2023;38:389-97. [Crossref] [PubMed]
- Besser MW, Klein AA. The coagulopathy of cardiopulmonary bypass. Crit Rev Clin Lab Sci 2010;47:197-212. [Crossref] [PubMed]
- Ibrahim H, Vapheas E, Shah B, et al. Preprocedural P2Y(12) inhibition and decrease in platelet count following transcatheter aortic valve replacement. Catheter Cardiovasc Interv 2019;94:812-7. [Crossref] [PubMed]
- Li J, Yu D, Song Y, et al. Association Between Postoperative Thrombocytopenia and Outcomes After Coronary Artery Bypass Grafting Surgery. Front Surg 2021;8:747986. [Crossref] [PubMed]
- Girão-Silva T, Fonseca-Alaniz MH, Ribeiro-Silva JC, et al. High stretch induces endothelial dysfunction accompanied by oxidative stress and actin remodeling in human saphenous vein endothelial cells. Sci Rep 2021;11:13493. [Crossref] [PubMed]
- Qureshi SH, Patel NN, Murphy GJ. Vascular endothelial cell changes in postcardiac surgery acute kidney injury. Am J Physiol Renal Physiol 2018;314:F726-35. [Crossref] [PubMed]
- Saemann L, Wernstedt L, Pohl S, et al. Impact of Age on Endothelial Function of Saphenous Vein Grafts in Coronary Artery Bypass Grafting. J Clin Med 2023;12:5454. [Crossref] [PubMed]
- Mitrophanov AY, Rosendaal FR, Reifman J. Computational analysis of the effects of reduced temperature on thrombin generation: the contributions of hypothermia to coagulopathy. Anesth Analg 2013;117:565-74. [Crossref] [PubMed]
- Gong P, Zhang MY, Zhao H, et al. Effect of mild hypothermia on the coagulation-fibrinolysis system and physiological anticoagulants after cardiopulmonary resuscitation in a porcine model. PLoS One 2013;8:e67476. [Crossref] [PubMed]
- Ise H, Kitahara H, Oyama K, et al. Hypothermic circulatory arrest induced coagulopathy: rotational thromboelastometry analysis. Gen Thorac Cardiovasc Surg 2020;68:754-61. [Crossref] [PubMed]
- Gielen CL, Grimbergen J, Klautz RJ, et al. Fibrinogen reduction and coagulation in cardiac surgery: an investigational study. Blood Coagul Fibrinolysis 2015;26:613-20. [Crossref] [PubMed]
- Yang M, Zhan S, Gao H, et al. Construction and validation of risk prediction model for gastrointestinal bleeding in patients after coronary artery bypass grafting. Sci Rep 2023;13:21909. [Crossref] [PubMed]
- Zhu J, Wu Z, Huang G, et al. Nomogram for predicting major bleeding after off-pump coronary artery bypass grafting. J Cardiothorac Surg 2024;19:23. [Crossref] [PubMed]
- Zapata D, Binongo J, Lasanajak Y, et al. Heparin-Induced Thrombocytopenia in Patients Undergoing Valvular and Aortic Surgery: A Modern Assessment of Risk. Innovations (Phila) 2020;15:229-34. [Crossref] [PubMed]